Individual- and county-level determinants of high breast cancer incidence rates
Introduction
Breast cancer is the most commonly diagnosed cancer in women in the United States, with an estimated 246,660 new cases diagnosed in 2016 (1). Breast cancer incidence varies dramatically across and within states (2,3). Reducing breast cancer disparities, including geographic disparities, is an overarching goal of the Healthy People 2020 initiative (4).
Progress has been made in reducing geographic disparities in breast cancer outcomes, but disparities remain (5,6). Understanding the complex and multilevel factors that influence these disparities is essential in order to design and implement effective interventions. Complex multilevel factors include individual factors; family, friends, and social support factors; healthcare provider and organizational factors; and policy and community factors (7). Because public health programs and policies are frequently designed and implemented at the county-level, there is value to examine disparities at this level. Such local information can also be used by hospitals and healthcare systems to understand local needs for medical care and to improve population health management as part of the Affordable Care Act. Identifying determinants at the individual- and area-levels may help explain geographic disparities, namely why some areas experience higher breast cancer incidence rates while other areas experience lower rates. Individual-level determinants include both modifiable (e.g., being overweight, use of hormones, physical inactivity, alcohol consumption) and non-modifiable risk factors for breast cancer (e.g., age, longer menstrual history, family history of breast cancer) (8). Theoretical models also suggest that population health is affected by population/area-level determinants (9-12), which are factors that influence breast cancer incidence on a wider scale. Examples include access to medical care, local socioeconomic conditions, and racial segregation (13,14), which act on all individuals in a population including women at risk for breast cancer. While prior research has focused predominantly on either individual-level determinants of individual breast cancer risk or examined population-level determinants of area-level breast cancer incidence, there is little evidence of the relative impact of both types of determinants on breast cancer incidence at the population level. Identifying reasons for elevated breast cancer incidence will allow for development and implementation of evidence-based, multilevel interventions to reduce geographic disparities. If population-level determinants are driving disparities over and above individual-level determinants, then this will help identify which types of interventions would be most beneficial (15).
We focused on the State of Arkansas because of the large geographic disparities and burden of breast cancer across counties that exist. Arkansas is a primarily rural state in the Midwest part of the United States, with areas of greater population density surrounding its larger cities in the central, northwest, northeast, and southwest areas of the state. White non-Hispanic residents are the majority racial group, with about one in six residents being African American. About 75 percent of Arkansas residents have completed high school or above. In 2018, 16 percent of Arkansas residents lived below the federal poverty line (16). While unemployment in Arkansas is typically similar to that in the United States, it varies substantially across Arkansas with higher rates in the eastern part of Arkansas. Extensive racial disparities exist in health outcomes with African Americans having higher rates of diabetes, risk factors for chronic diseases, and incidence and mortality following chronic disease diagnosis compared to white residents (17-20). According to countyhealthrankings.org, health behaviors, access to high-quality medical care, social and economic factors, and health outcomes appear to be worse in the eastern part of Arkansas.
During 2008–2012, 11,556 women were diagnosed with ductal carcinoma in-situ or invasive breast cancer across Arkansas’ 75 counties. The overall age-adjusted rate was 132.1 (95% CI: 129.6–134.6) per 100,000 population. Of 11,556 breast cancers, 1,429 (12.4%) were among African Americans and 9,837 (85.1%) among whites. Invasive cancers accounted for 81.7 percent of breast cancers. Breast cancer incidence varied across counties and ranged from 80.9 to 161.6 per 100,000 population (Figure 1). The number of breast cancer cases by county ranged from 16 to 1,762. Incidence appeared to be higher in the central counties, although some counties with high rates were bordered by counties with low rates.
We used a novel micro-macro statistical approach in public health (21), which adjusts aggregated individual-level data to account for aggregation-induced biases, to identify determinants of county-level breast cancer incidence rates at both the individual and county level.
Methods
Breast cancer incidence data
County-level breast cancer incidence data from 2008 to 2012 was obtained from the Arkansas Central Cancer Registry (ACCR). Specifically, the ACCR provided county-level age-adjusted breast cancer incidence rates for women diagnosed with ductal carcinoma in situ or invasive disease during 2008–2012. The ACCR is certified by the North American Association of Central Cancer Registries, and is a population-based registry financially supported by the Centers for Disease Control and Prevention (CDC) through their National Program of Cancer Registries and collects data on all cancers of Arkansas residents. Mandated reporters are required by Arkansas law (20-15-202) to submit all cancer-related diagnoses. Additionally, the ACCR has a case-sharing agreement with 18 other states to capture cancer cases among Arkansas residents who may have been diagnosed or treated elsewhere. The ACCR is gold certified by the North American Association of Central Cancer Registries, which means that the Registry was estimated to capture at least 95% of the expected number of cancer cases.
Individual-level breast cancer risk factors
Individual-level sociodemographic information and breast cancer risk factors were obtained from the cross-sectional Arkansas Rural Community Health (ARCH) study (described in more detail elsewhere) (22,23). Briefly, the ARCH study recruited women during community events designed to increase breast cancer awareness, as well as non-cancer related community events. After providing written consent, women completed a questionnaire about breast cancer risk factors using validated instruments (22). We limited the study participants for this analysis to those who were between the ages of 35 and 85 at the time of enrollment, were white or African American, did not have a prior diagnosis of breast cancer, were enrolled in the study between September 2007 and December 2012, and resided in Arkansas at the time of enrollment. The self-reported residential street address of each study participant was geocoded using ArcGIS version 10.2.2 to obtain the county of residence for linking with the corresponding county-level measurements. Predicted breast cancer risks were estimated using the Gail model (24) for white women and the Women’s Contraceptive and Reproductive Experiences study (CARE) model for African American women (25). The Gail model uses a woman’s personal medical and reproductive history and the history of breast cancer among her first-degree relatives (mother, sisters, or daughters) to estimate absolute breast cancer risk. The CARE model uses age at menarche, number of affected mother or sisters, and number of previous benign biopsy examinations to estimate risk. Because the number of biopsies collected per woman was not measured, women who reported having a biopsy were considered as having had one biopsy for the purpose of risk prediction. Likewise, the questionnaire did not collect data on atypical hyperplasia, so this was set to missing for all women. Five-year and lifetime breast cancer risk were estimated using the SAS macro programs obtained from the National Cancer Institute for the Gail model (26) and for the CARE model (27).
Self-reported height and weight were used to calculate body mass index (BMI) both at the time of completion of the survey and at age 18. Alcohol consumption in grams per day was calculated as the sum of the daily number of drinks multiplied by the average alcohol content per type of alcoholic beverage (13 g of alcohol per serving). Daily alcohol use was categorized as <10 or ≥10 g/day based on its association with breast cancer risk in women aged 40 or older (28). Breast feeding was measured as the duration (if any) of breast feeding (22). Physical activity was categorized as highly active, active, insufficiently active, or inactive based on CDC guidelines of ≥30 minutes of moderate physical activity 5 or more days per week or vigorous physical activity for ≥20 minutes 3 or more days per week) (29).
County-level breast cancer determinants
County-level determinants were obtained from multiple data sources (e.g., Behavioral Risk Factor Surveillance System, Area Resource File, American Community Survey). We used the County Health Rankings model to classify the county-level determinants into four broad exposure categories (Health Behaviors, Clinical Care, Social and Economic Environment, and Physical Environment) (30). The County Health Rankings model was augmented by adding a Population Health Status category (31). Because county data from the Arkansas Behavioral Risk Factor Surveillance System (BRFSS) yields reliable data for only one county (Pulaski), the Arkansas Department of Health has estimated county-level prevalence using survey data from adjacent counties with subsequent adjustment to the age, race, and gender distribution of the county (32).
Health behavior determinants consisted of: (I) breast cancer screening prevalence (percentage of women aged ≥40 who reported not having had a mammogram during the past 2 years); and (II) prevalence of the population meeting the CDC’s physical activity guidelines.
Clinical care determinants consisted of access to and quality of medical care, which included: (I) the population per primary care physician; (II) the hospitalization rate for ambulatory-care sensitive conditions (preventable hospitalizations); and (III) the population aged <65 without health insurance (uninsured rate).
Social and economic determinants consisted of: (I) the Theil index of racial segregation (33); (II) poverty rate (percentage of the population below the federal poverty line); (III) percentage of adults without social/emotional support; (IV) the violent crime rate (per 100,000 population); and (V) the high school graduation rate. We obtained the Theil index to estimate racial inequality from CommunityCommons.org, which measures the "evenness" of all races across a county based on the racial composition of the population at census blocks. For any given county, the index measures the average difference between each census block’s racial distribution (entropy), and the racial distribution (entropy) of the county as a whole. Values range from 0 to 1. Areas with higher values have less uniform racial distributions and areas with lower values have more uniform ethnic distributions. The population groups used in the measurement were non-Hispanic White, non-Hispanic Black, non-Hispanic Asian, non-Hispanic American Indian/Alaska Native, non-Hispanic Native Hawaiian/Pacific Islander, and Hispanic or Latino.
Physical environment determinants consisted of: (I) lead emissions and (II) population density per square mile. County-level estimates of lead emissions were obtained from the Environmental Protection Agency’s Toxic Release Inventory data that contains facility location and onsite lead release (in pounds). Lead has been shown to increase breast cancer risk (34-36). The Toxic Release Inventory is publicly available data that contains detailed information on selected chemical releases and waste management activities reported annually (37).
Population Health Status comprised: (I) diabetes prevalence (percentage of the population who reported having been diagnosed with diabetes, excluding gestational diabetes); (II) infant mortality rate; and (III) prevalence of fair or poor health status. Infant mortality was based on the number of infant deaths <1 year old per 1,000 live births obtained from the Area Health Resource File. Diabetes and infant mortality are often used as an indicator of the level of health in a county (38,39). The Area Health Resource file suppresses data for counties with <10 infant deaths between 2008 and 2012, therefore we compared these data with the 2006 to 2010 infant mortality rate estimates from the Area Health Resource file for which such data were not suppressed. We found a correlation of 0.94 suggesting that infant mortality rates for counties with ≥10 infant deaths during 2008-2012 were stable.
Statistical analysis
Data for county-level mean lead exposure were missing for 26 of the 75 counties. Missing values were imputed by regressing the log mean lead measurement on all other county-level predictors. The antilog of the fitted values from the regression was then imputed for the 26 counties with missing lead measurements.
We examined the univariate association of each individual-level and county-level determinant with breast cancer incidence. Nonlinear functions of the predictors were examined by visually investigating scatterplots and including logarithmic transformations in the univariate models. Next, we investigated multivariable linear models for county-level age-adjusted breast cancer incidence rates including: (I) all county-level predictors; (II) all individual-level predictors; and (III) all county-level and individual-level predictors. For models including individual-level predictors, we aggregated individual data to the county level using a micro-macro model to adjust for bias due to group-level aggregation (21). The micro-macro model has been applied to fields such as education and organizational psychology and management (40-45), but to our knowledge has not yet been implemented in public health research. Ordinary least squares (OLS) regression using aggregated individual data to predict a group-level outcome will result in biased estimates of regression coefficients, a phenomenon sometimes called the atomistic fallacy. The micro-macro model adjusts the group-aggregated average to provide an unbiased estimate of the relationship between the aggregated predictor and the outcome. The adjustment comes from a linear combination of: (I) the group-aggregated average; (II) the full sample mean of the individual-level values; and (III) the deviation from the overall average of included group-level predictors. The model also adjusts standard errors to account for imprecision in the newly created predictor. We also examined interactions between 5-year predicted breast cancer risk and each of the county-level determinants, hypothesizing that higher breast cancer incidence rates were due to the synergistic effects of individual- and county-level determinants.
We used a backward stepwise selection with BIC criteria to arrive at a final model for predicting county-level breast cancer incidence based on individual- and county-level determinants, with lower BIC values indicating better model fit. Beginning with the full model including all individual- and group-level predictors, predictor variables were removed until the model with lowest BIC was reached (i.e., removing any variable from the model would increase BIC). Since the BIC criteria are a direct numerical comparison without a formal hypothesis test, it is possible for the best fit model to contain predictors with non-significant coefficients.
The stability of our results may be affected by the standard error of the county breast cancer rates. We examined the robustness of our findings by performing a sensitivity analysis regressing the upper and lower bounds of the 95% confidence intervals of the county rate using the variables in our best-fit model. We also joined the residuals of our best-fit model with a map of the Arkansas counties and calculated Moran's I (both an empirical method and a Bayesian method) to determine the need for a spatial model. Analyses were performed using R (version 3.3.1) (46).
Results
Study population
In all, 20,007 women in the ARCH study completed questionnaires, 13,554 of whom met the study’s inclusion criteria. The number of completed questionnaires ranged from 8 to 4,166 across Arkansas counties. In our study sample, 12.5% were age 65 or older, 20.8% were African American, and 75.2% had attended at least some college (Table 1). Nearly 40% of participants had a BMI considered to be obese (BMI ≥30). Many of the sociodemographic characteristics of the participants varied across counties.
Table 1
Risk factors | Percentage | County range (%) |
---|---|---|
Age (years) | ||
35–49 | 50.4 | − |
50–64 | 37.1 | − |
65 or older | 12.5 | 4.4–47.8 |
Race | ||
African American | 20.8 | 0.0–75.9 |
White | 79.2 | − |
Education | ||
Less than high school | 3.5 | 0.0–23.6 |
High school or GED | 21.2 | − |
At least some college | 75.2 | − |
Unknown | 0.1 | − |
Age at menarche (years) | ||
<12 | 24.0 | 16.7–40.0 |
12–13 | 52.3 | − |
14 or older | 23.7 | − |
Body mass index at time of survey (kg/m2) | ||
<18.5 | 1.3 | − |
18.5–24.9 | 28.3 | − |
25.0–29.9 | 29.8 | − |
30 or more | 39.7 | 0.0–63.2 |
Unknown | 1.0 | − |
Body mass index at age 18 (kg/m2) | ||
<18.5 | 22.5 | − |
18.5–24.9 | 63.1 | − |
25.0–29.9 | 8.5 | − |
30 or more | 4.4 | 0.0–12.5 |
Unknown | 1.5 | − |
Lactation | ||
No (no child birth, duration 0–6 months) | 76.9 | − |
Yes (6 or more months) | 21.7 | 0.0–50.0 |
Unknown | 1.4 | − |
Alcohol use | ||
0–<10 g/day | 86.8 | − |
10 g/day or more | 12.4 | 0–19.5 |
Unknown | 0.1 | − |
Physical activity | ||
Inactive | 6.4 | 0–18.2 |
Insufficiently active | 14.9 | − |
Active | 16.9 | − |
Highly active | 61.8 | − |
Mean 5-year predicted breast cancer risk, % (st dev) | 1.3 (1.0) | 1.0–1.9 |
Mean lifetime predicted breast cancer risk, % (st dev) | 9.9 (5.2) | 7.5–11.9 |
GED, graduate equivalency degree.
Univariate models of individual- and county-level determinants
For many characteristics, the variation across Arkansas counties was large (Table 2). In many instances, the counties with the maximum values for some of these adverse county-level factors were more than double those of the counties with the minimum values. Several individual- and county-level factors were associated with higher breast cancer incidence in univariate models (Table S1). The explained variance of any determinant was highest for infant mortality rate (R-squared =16.4%).
Table 2
County-level factors | Median | Mean | Range | Data source |
---|---|---|---|---|
Health behaviors | ||||
Women ≥40 without mammogram in past 2 years (%) | 31.3 | 31.4 | 14.4–46.3 | BRFSS [2009] |
Meeting physical activity recommendations (%) | 46.8 | 46.8 | 33.7–63.1 | BRFSS [2009] |
Clinical care | ||||
Population per primary care physician | 1,419 | 2,152 | 673.9–14,130 | Area Health Resource file |
Hospitalization rate for ambulatory-care sensitive conditions (per 1,000 Medicare enrollees) | 81 | 86 | 51–145 | Dartmouth Atlas of Health Care from County Health Rankings [2011] |
Uninsured rate (age <65 years) (%) | 20 | 21 | 16–31 | Small Area Health Insurance Estimates [2011] |
Social & economic factors | ||||
Theil index of racial segregation | 0.455 | 0.452 | 0.285–0.633 | CommunityCommons.org [2010] |
Poverty rate (%) | 20.4 | 21.0 | 8.4–32.3 | American Community Survey [2010] |
Adults without social/emotional support (%) | 22 | 22 | 11–39 | BRFSS [2005-2010] |
Violent crime rate (per 100,000) | 270 | 352 | 30–1,724 | FBI Uniform Crime Reporting [2009-2011] |
High school graduation rate (%) | 84 | 84 | 66–96 | American Community Survey [2010] |
Physical environment | ||||
Lead (pounds) | 51.31 | 152.2 | 0–2,538 | Toxic Release Inventory |
Population density (per square mile) | 115.5 | 194.2 | 10.5–468.9 | Area Health Resource file |
Population health status | ||||
Diabetes (%) | 10.6 | 10.9 | 5.0–17.9 | BRFSS |
Infant mortality rate (per 1,000 live births) | 7.4 | 7.5 | 0.0–15.1 | Area Health Resource file |
Fair-poor health status (%) | 22 | 22 | 12–36 | BRFSS |
BRFSS, Behavioral Risk Factor Surveillance System.
Multivariable model of individual- and county-level determinants
Table 3 compares the variance explained (adjusted R-squared) and model fit (BIC) across four models: all county-level factors (Model 1), all individual-level factors (Model 2), all county- and individual-level factors (Model 3), and the model of best fit (Model 4). Model 1 had higher adjusted R-squared and better fit than Model 2. Although the adjusted R-squared was higher for the model with all predictors (Model 3), its fit was significantly worse than either Model 1 or 2. Model 4, the best fit model, contained the individual-level determinants (Gail/CARE predicted breast cancer risk) and county-level determinants [lead exposure (log transformed) and population density (log transformed)] and yielded an adjusted R-squared of 14.1%. As shown in Table 4, the county breast cancer incidence rate increases by 0.64 cases per 100,000 population for every percentage increase in a woman’s risk of breast cancer, controlling for other variables in the model. The county incidence rate increased by 6.8 per 100,000 population for every unit increase in the log-transformed population density. Although log-transformed county lead exposure was included because of the improvement in the model’s fit, it was not statistically associated with breast cancer incidence rate (P=0.090). The best fit model was checked for linear model assumptions and collinearity. The linear model passed visual inspection for violations of linearity, homoscedasticity, and normality via residual plots which can be found in Figures S1,S2. All variance inflation factors were less than 2, indicating minimal concerns about collinearity.
Table 3
Model | R-squared | Adjusted R-squared | BIC |
---|---|---|---|
Model 1: all county-level predictors | 0.287 | 0.102 | 647.5 |
Model 2: all individual-level predictors | 0.275 | 0.055 | 657.3 |
Model 3: all predictors | 0.628 | 0.338 | 672.5 |
Model 4: best fit model | 0.176 | 0.141 | 606.6 |
BIC, Bayesian Information Criterion.
Table 4
Variable | Beta | Standard error | P value |
---|---|---|---|
Individual-level factors | |||
5-year predicted breast cancer risk (Gail/CARE models) | 0.639 | 0.121 | <0.001 |
County-level factors | |||
Lead (log) | −0.667 | 0.396 | 0.097 |
Population density per square mile (log) (%) | 6.815 | 1.908 | 0.001 |
Generally, our conclusions were similar in sensitivity analyses modeling the upper and lower bounds of the 95% confidence intervals. Though there were numerical differences across the models, the results were qualitatively similar. Also, there was no clear evidence for the need for a spatial model using the empirical and Bayesian methods to calculate Moran’s I and the residuals of our best-fit model (P values >0.05).
Discussion
Because breast cancer incidence rates varied significantly across Arkansas counties, our purpose was to identify individual- and county-level determinants in an attempt to identify opportunities for intervention to reduce county variability in incidence rates. Using the County Health Rankings model as our guide, we identified two county-level determinants of breast cancer incidence, mean lead emission (log transformed) and population density (log transformed). In other words, breast cancer incidence rate differences reflect factors beyond those captured solely by the woman’s predicted breast cancer risk. This is evidenced by the fact that this model displayed much better fit than the model that considered only individual-level risk factors; it explained 14.1% of the variance in breast cancer incidence.
Our results support theoretical models that claim that population-level determinants of area-level disease are key drivers beyond individual risk (47,48). Thus, examining determinants of geographic variability in breast cancer incidence and opportunities for intervention should include individual-level as well as area-level determinants. Our results further suggest that reducing the variability of only individual-level risk factors cannot be reasonably expected to reduce variability in incidence rates among counties. Typically, interventions targeting individual-level determinants in the face of powerful population-level determinants are expected to have a minimal impact on population-level disease (11). Interventions focusing on multiple levels may have a larger impact than those focusing solely on individual-level determinants (15). Our results also suggest that strategies should incorporate various social determinants of health to better understand the impact of modifiable and non-modifiable risk factors that contribute to an individual’s risk of disease (breast cancer in this case). Failure to recognize this will perpetuate ignoring area-level (environmental/social contextual) factors (49).
Two county-level determinants were found to be associated with breast cancer incidence. First, our results of a positive association between higher population density and breast cancer incidence confirm observations that urban women had higher breast cancer risk than rural women (47,48). This suggests that targeting women in urban counties in Arkansas by reducing their risk may reduce the existing variability in breast cancer incidence. Their increased risk may be due, in part, to increased traffic-related air pollution in urban areas. A recent study showed increased premenopausal breast cancer incidence associated with residential air pollution (50). Second, mean lead emission (log transformed) was included in the best-fitting model. Lead exposure has been shown to increase a woman’s breast cancer risk (34-36). Our results suggest that county-level lead emission may be associated with breast cancer incidence rates, but additional research should be conducted to further delineate this association.
Third, just as important was our finding that county-level health behaviors (including mammography use), availability of medical care, social and economic determinants, and population health status were not associated with breast cancer incidence. This lack of association suggests that intervening on these determinants would not reduce the variability in breast cancer incidence at the county level. Moreover, the rate of in-situ and invasive breast cancer were very similar for white and African American women in Arkansas. County poverty rate, all too often associated with racial composition, and the Theil index of racial segregation were not associated with county breast cancer incidence rate. Thus, county racial composition is unlikely to explain higher breast cancer rates in some counties. Our results confirm for breast cancer incidence that the use of medical care provided to patients accounts for only a minor portion of population health status (12).
The only individual-level determinant associated with breast cancer incidence in our best-fitting model was predicted risk of breast cancer based on the Gail/CARE model, which consists of woman’s age, education, age at menarche, number of biopsies, number of first-degree relatives that have been diagnosed with breast cancer, age at first childbirth, and the presence of atypical hyperplasia (24,25). Although this predicted risk was associated with county-level breast cancer incidence, none of these variables are modifiable. While behavior represents the single most prominent domain of influence over health (12), interestingly, previously observed risk factors for breast cancers, such as BMI at age 18 or at the time of the survey, breast feeding, physical activity, and alcohol use, were not associated with breast cancer incidence in our best fitting model. This suggests that modifying these behaviors would have little direct impact on reducing geographic variability in breast cancer incidence at the county level. Other modifiable risk factors for breast cancer, including diet, body shape at menarche, use of hormone replacement therapy, and dietary patterns (51), may have played a role but were not assessed in our survey. Future studies should include these variables, building upon our best fitting model, recognizing that our model explained only 14.1% of the variance in the county breast cancer incidence rate.
Our findings should be interpreted in light of some limitations. First, our data were observational data and our results should be interpreted as reflecting statistical associations, not causal relationships. Because some data were at the county level, we were unable to address issues of cross-border receipt of medical care or exposures. Second, the use of county-level data based on sampling (e.g., BRFSS) is subject to uncertainty. Although the number of participants in the ARCH survey varied across counties, our micro-macro statistical model was able to negate this variability. Third, data from women who participated in the ARCH survey were typically of higher income and education. Controlling for educational status may have alleviated some of this limitation but perhaps not all of it. Fourth, because our understanding of risk factors for breast cancer is still incomplete (51,52), unmeasured and unknown risk factors may have played a role. Fifth, generalizability of our findings beyond the State of Arkansas may be limited because of the unique characteristics of the state. Sixth, we made no distinction between pre- or post-menopausal breast cancer, in situ or invasive breast cancer, nor among various molecular breast cancer types (e.g., triple negative breast cancer) because of the potentially small number of breast cancers in many counties which would have resulted in unstable rates. Seventh, the standard error of the breast cancer rates varied across counties based on the number of breast cancers. However, our sensitivity analyses regressing the upper and lower bounds of the 95% confidence intervals of the county rates showed our results to be qualitatively similar to our analysis of the county rates. Eighth, variable selection and model development is an inherently exploratory process. There is a tradeoff between explaining the largest proportion of variation in the outcome and excluding spurious relationships with the goal of producing replicable models. In our case the best fitting model produces a much lower adjusted R-squared than the full model as much of the variation is due to minor improvements from many variables. Removal of those variables yields a lower adjusted R-squared but a model in which we can be more confident about the relationships that were uncovered. Finally, genetic aspects of breast cancer beyond family history were not included, but this is expected to play only a minor role at the population level (53).
In conclusion, variability in breast cancer incidence rates reflects determinants beyond those captured by individual-level variables. Not considering upstream determinants assumes that traditional determinants (e.g., mammography use, breast cancer risk) play a large role in breast cancer incidence disparities. Additional research should be conducted to further explain county-level breast cancer incidence rates.
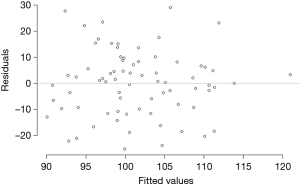
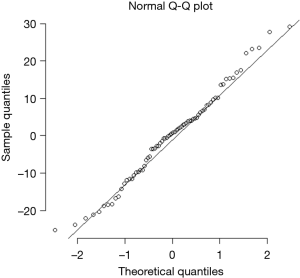
Table S1
Individual- and county-level factors | R-square | Beta | Standard error | P value |
---|---|---|---|---|
Individual-level factors | ||||
5-year predicted breast cancer risk | 0.000 | 0.066 | 1.510 | 0.965 |
Age (years) | 0.017 | −0.176 | 0.151 | 0.247 |
Current body mass index (vs. <18.5) | 0.026 | |||
18.5–24.9 | 0.017 | 0.051 | 0.738 | |
25.0–29.9 | 0.010 | 0.041 | 0.816 | |
30 or more | 0.049 | 0.040 | 0.222 | |
Age at menarche (vs. <12 years) | 0.030 | |||
12–13 years | −0.082 | 0.039 | 0.040 | |
14 years or older | −0.106 | 0.054 | 0.056 | |
Body mass index at age 18 (vs. <18.5) in kg/m2 | 0.072 | |||
18.5–24.9 | 0.056 | 0.037 | 0.136 | |
25.0–29.9 | −0.659 | 0.307 | 0.035 | |
30 or more | −0.074 | 0.073 | 0.315 | |
Lactation (yes vs. no) | 0.018 | 0.006 | 0.006 | 0.352 |
Education (vs. less than high school) | 0.020 | |||
High school or GED | 0.090 | 0.040 | 0.027 | |
At least some college | 0.071 | 0.030 | 0.021 | |
Alcohol use (≥10 vs. <10 g/day) | 0.010 | 0.070 | 0.055 | 0.205 |
Physical activity (vs. inactive) | 0.013 | |||
Insufficiently active | −0.132 | 0.213 | 0.539 | |
Active | −0.064 | 0.132 | 0.627 | |
Highly active | −0.164 | 0.235 | 0.488 | |
County-level factors | ||||
Health behaviors | ||||
Women ≥40 without mammogram in past 2 years (%) | 0.003 | 0.114 | 0.262 | 0.663 |
Meeting physical activity recommendations (%) | 0.021 | 0.342 | 0.279 | 0.224 |
Clinical care | ||||
Population per primary care physician | 0.071 | −6.367 | 2.723 | 0.022 |
Hospitalization rate for ambulatory-care sensitive conditions | 0.050 | −0.140 | 0.072 | 0.056 |
Uninsured rate (age <65 years) (%) | 0.009 | −0.494 | 0.611 | 0.422 |
Social & economic factors | ||||
Theil index (linear only) | 0.004 | −0.108 | 0.200 | 0.590 |
Theil (linear component) | 0.085 | −5.095 | 2.008 | 0.013 |
Theil (quadratic component) | 0.056 | 0.022 | 0.015 | |
Population living in the same house 1 year ago (%) | 0.000 | −0.012 | 0.448 | 0.979 |
Poverty rate (%) | 0.024 | −0.433 | 0.330 | 0.194 |
Adults without social/emotional support (%) | 0.014 | −0.369 | 0.364 | 0.314 |
Violent crime rate (per 100,000) | 0.024 | 0.007 | 0.006 | 0.189 |
High school graduation rate (%) | 0.017 | −0.348 | 0.307 | 0.262 |
Physical environment | ||||
Lead (natural log) | 0.018 | −0.448 | 0.391 | 0.256 |
Population density per square mile | 0.108 | 5.985 | 2.039 | 0.004 |
Population health status | − | |||
Diabetes (%) | 0.064 | −1.171 | 0.531 | 0.031 |
Infant mortality rate (linear only) | 0.008 | 0.443 | 0.583 | 0.450 |
Infant mortality rate (linear component) | 0.164 | 6.984 | 1.890 | 0.000 |
Infant mortality rate (quadratic component) | − | −0.413 | 0.114 | 0.001 |
Fair-poor health status (%) | 0.052 | −0.647 | 0.326 | 0.051 |
Acknowledgments
Funding: None.
Footnote
Provenance and Peer Review: This article was commissioned by the Guest Editors (Hui-Yi Lin, Tung-Sung Tseng) for the series “Population Science in Cancer” published in Translational Cancer Research. The article has undergone external peer review.
Conflicts of Interest: All authors have completed the ICMJE uniform disclosure form (available at http://dx.doi.org/10.21037/tcr.2019.06.08). The series “Population Science in Cancer” was commissioned by the editorial office without any funding or sponsorship. The authors have no other conflicts of interest to declare.
Ethical Statement: The authors are accountable for all aspects of the work in ensuring that questions related to the accuracy or integrity of any part of the work are appropriately investigated and resolved. The study was conducted in accordance with the Declaration of Helsinki (as revised in 2013). The study was approved by the Institutional Review Boards of the University of Arkansas Medical Sciences (No. 89071) and Saint Louis University (No. 26910) and written informed consent was obtained from all patients.
Open Access Statement: This is an Open Access article distributed in accordance with the Creative Commons Attribution-NonCommercial-NoDerivs 4.0 International License (CC BY-NC-ND 4.0), which permits the non-commercial replication and distribution of the article with the strict proviso that no changes or edits are made and the original work is properly cited (including links to both the formal publication through the relevant DOI and the license). See: https://creativecommons.org/licenses/by-nc-nd/4.0/.
References
- American Cancer Society. Cancer facts & figures, 2015. Atlanta, GA: American Cancer Society, 2015.
- American Cancer Society. Cancer facts & figures, 2016. Atlanta, GA: American Cancer Society, 2016.
- Keller D, Guilfoyle C, Sariego J. Geographical influence on racial disparity in breast cancer presentation in the United States. Am Surg 2011;77:933-6. [PubMed]
- U.S. Department of Health and Human Services. Healthy People 2020. 2014. Available online: http://www.healthypeople.gov/2020/about/foundation-health-measures/Disparities.
- Schootman M, Lian M, Deshpande AD, et al. Temporal trends in geographic disparities in small-area breast cancer incidence and mortality, 1988-2005. Cancer Epidemiol Biomarkers Prev 2010;19:1122-31. [Crossref] [PubMed]
- Sighoko D, Murphy AM, Irizarry B, et al. Changes in the racial disparity in breast cancer mortality in the ten US cities with the largest African American populations from 1999 to 2013: The reduction in breast cancer mortality disparity in Chicago. Cancer Causes Control 2017;28:563-8. [Crossref] [PubMed]
- Purnell TS, Calhoun EA, Golden SH, et al. Achieving health equity: Closing the gaps in health care disparities, interventions, and research. Health Affairs 2016;35:1410-5. [Crossref] [PubMed]
- Markin A, Habermann EB, Chow CJ, et al. Rurality and cancer surgery in the United States. Am J Surg 2012;204:569-73. [Crossref] [PubMed]
- Glass TA, McAtee MJ. Behavioral science at the crossroads in public health: Extending horizons, envisioning the future. Soc Sci Med 2006;62:1650-71. [Crossref] [PubMed]
- Rose G. Sick individuals and sick populations. Int J Epidemiol 2001;30:427-32. [Crossref] [PubMed]
- Frohlich KL, Potvin L. Transcending the Known in Public Health Practice: The Inequality Paradox: The Population Approach and Vulnerable Populations. Am J Public Health 2008;98:216-21. [Crossref] [PubMed]
- McGinnis JM, Williams-Russo P, Knickman JR. The case for more active policy attention to health promotion. Health Affairs 2002;21:78-93. [Crossref] [PubMed]
- Lian M, Struthers J, Schootman M. Comparing GIS-based measures in access to mammography and their validity in predicting neighborhood risk of late-stage breast cancer. PLoS One 2012;7:e43000. [Crossref] [PubMed]
- Boscoe FP, Henry KA, Sherman RL, et al. The relationship between cancer incidence, stage and poverty in the United States. Int J Cancer 2016;139:607-12. [Crossref] [PubMed]
- Paskett E, Thompson B, Ammerman AS, et al. Multilevel interventions to address health disparities show promise in improving population health. Health Affairs 2016;35:1429-34. [Crossref] [PubMed]
- Center for American progress. Talk poverty. 2019. Available online: https://talkpoverty.org/state-year-report/arkansas-2018-report/. Accessed 5/24/2019.
- Govindarajan R, Shah RV, Erkman LG, et al. Racial differences in the outcome of patients with colorectal carcinoma. Cancer 2003;97:493-8. [Crossref] [PubMed]
- Sekikawa A, Kuller LH. Striking variation in coronary heart disease mortality in the United States among black and white women aged 45-54 by state. J Womens Health Gend Based Med 2000;9:545-58. [Crossref] [PubMed]
- Monzavi-Karbassi B, Siegel ER, Medarametla S, et al. Breast cancer survival disparity between African American and Caucasian women in Arkansas: A race-by-grade analysis. Oncol Lett 2016;12:1337-42. [Crossref] [PubMed]
- Mujib M, Zhang Y, Feller MA, et al. Evidence of a "heart failure belt" in the southeastern United States. Am J Cardiol 2011;107:935-7. [Crossref] [PubMed]
- Croon MA, van Veldhoven MJ. Predicting group-level outcome variables from variables measured at the individual level: a latent variable multilevel model. Psychol Methods 2007;12:45-57. [Crossref] [PubMed]
- Bondurant KL, Harvey S, Klimberg S, et al. Establishment of a southern breast cancer cohort. Breast J 2011;17:281-8. [Crossref] [PubMed]
- Lee JY, Klimberg S, Bondurant KL, et al. Cross-sectional study to assess the association of population density with predicted breast cancer risk. Breast J 2014;20:615-21. [Crossref] [PubMed]
- Gail MH, Brinton LA, Byar DP, et al. Projecting individualized probabilities of developing breast cancer for white females who are being examined annually. J Natl Cancer Inst 1989;81:1879-86. [Crossref] [PubMed]
- Gail MH, Costantino JP, Pee D, et al. Projecting individualized absolute invasive breast cancer risk in African American women. J Natl Cancer Inst 2007;99:1782-92. [Crossref] [PubMed]
- Breast Cancer Risk Assessment SAS Macro (Version 4, Gail Model). Available online: https://dceg.cancer.gov/tools/risk-assessment/bcrasasmacro. Accessed April 29, 2019.
- CARE Model SAS Macro: Breast Cancer Risk Assessment for African American Women. Available online: https://dceg.cancer.gov/tools/risk-assessment/care. Accessed April 29, 2019.
- Chen WY, Rosner B, Hankinson SE, et al. Moderate alcohol consumption during adult life, drinking patterns, and breast cancer risk. JAMA 2011;306:1884-90. [Crossref] [PubMed]
- U.S. Department of Health and Human Services. 2008 Physical activity guidelines for American. ODPHP Publication No. U0036 Washington, DC2008.
- University of Wisconsin Population Health Initiative. County health rankings and roadmaps. 2017. Available online: http://www.countyhealthrankings.org/our-approach. Accessed February 22, 2017.
- Patel SA, Ali MK, Narayan KM, et al. County-level variation in cardiovascular disease mortality in the United States in 2009-2013: Comparative assessment of contributing factors. Am J Epidemiol 2016;184:933-42. [Crossref] [PubMed]
- Arkansas Department of Health. Methodology for county BRFSS estimates. Little Rock, AR. 2017. Accessed February 22, 2017.
- Reardon SF, Firebaugh G. Measures of multigroup segregation. Sociol Methodol 2002;32:33-67. [Crossref]
- Poirier LA, Vlasova TI. The prospective role of abnormal methyl metabolism in cadmium toxicity. Environ Health Perspect 2002;110:793-5. [Crossref] [PubMed]
- Silbergeld EK, Waalkes M, Rice JM. Lead as a carcinogen: experimental evidence and mechanisms of action. Am J Ind Med 2000;38:316-23. [Crossref] [PubMed]
- Salnikow K, Costa M. Epigenetic mechanisms of nickel carcinogenesis. J Environ Pathol Toxicol Oncol 2000;19:307-18. [PubMed]
- Environmental Protection Agency. TRI national analysis archive. Washington, DC. 2017. Accessed February 1, 2017.
- Institute of Medicine. State of the USA Health Indicators: Letter Report. Washington, DC: The National Academies Press, 2009.
- Yankauer A. What infant mortality tells us. Am J Public Health 1990;80:653-4. [Crossref] [PubMed]
- Schweig J. Cross-Level Measurement Invariance in School and Classroom Environment Surveys: Implications for Policy and Practice. Educ Eval Policy Anal 2014;36:259-80. [Crossref]
- Marsh HW, Lüdtke O, Nagengast B, et al. Classroom Climate and Contextual Effects: Conceptual and Methodological Issues in the Evaluation of Group-Level Effects. Educ Psychol 2012;47:106-24. [Crossref]
- Wood S, Van Veldhoven M, Croon M, et al. Enriched job design, high involvement management and organizational performance: The mediating roles of job satisfaction and well-being. Human Relations 2012;65:419-45. [Crossref]
- Taris TW, Schreurs PJG. Well-being and organizational performance: An organizational-level test of the happy-productive worker hypothesis. Work Stress 2009;23:120-36. [Crossref]
- Zhang Z, Waldman DA, Wang Z. A multilevel investigation of leader- member exchange, informal leader emergence, and individual and team performance: personnel psychology. Pers Psychol 2012;65:49-78. [Crossref]
- Kostopoulos KC, Spanos YE, Prastacos GP. Structure and Function of Team Learning Emergence: A Multilevel Empirical Validation. J Manage 2013;39:1430-61. [Crossref]
- R Core Team. Computing RFfS. editor. R: A language and environment for statistical computing. Vienna, Austria: R Foundation for statistical Computing 2016;
- Akinyemiju TF, Genkinger JM, Farhat M, et al. Residential environment and breast cancer incidence and mortality: a systematic review and meta-analysis. BMC Cancer 2015;15:191. [Crossref] [PubMed]
- Robert SA, Strombom I, Trentham-Dietz A, et al. Socioeconomic risk factors for breast cancer: distinguishing individual- and community-level effects. Epidemiology 2004;15:442-50. [PubMed]
- Paskett ED. The new vital sign: Where do you live? Cancer Epidemiol Biomarkers Prev 2016;25:581-2. [Crossref] [PubMed]
- Villeneuve PJ, Goldberg MS, Crouse DL, et al. Residential exposure to fine particulate matter air pollution and incident breast cancer in a cohort of Canadian women. Env Epdemiol 2018;2:e021. [Crossref]
- Dartois L, Fagherazzi G, Baglietto L, et al. Proportion of premenopausal and postmenopausal breast cancers attributable to known risk factors: Estimates from the E3N-EPIC cohort. Int J Cancer 2016;138:2415-27. [Crossref] [PubMed]
- Coyle YM. The effect of environment on breast cancer risk. Breast Cancer Res Treat 2004;84:273-88. [Crossref] [PubMed]
- West KM, Blacksher E, Burke W. Genomics, health disparities, and missed opportunities for the nation's research agenda. JAMA 2017;317:1831-2. [Crossref] [PubMed]