Comprehensive analysis of competitive endogenous RNA network in colorectal cancer
Introduction
Colorectal cancer (CRC), also known as bowel or colon cancer or rectal cancer, is an aggressive human tumor (1,2). According to the statistics, more than 51,020 people will die from this disease in 2019, accounting for about 8% of all cancer deaths. Moreover, it has been estimated that, as of January 1, 2019, more than 1.5 million people in the United States received a diagnosis of CRC, and 145,600 new cases are predicted to be diagnosed in 2019 (3,4). With the rise of the Human Development Index (HDI), the incidence of CRC has gradually increased worldwide. Consistently, the incidence and mortality of CRC in China have grown in the past decade (5). Therefore, understanding CRC carcinogenesis at the molecular level is a pivotal step for improving early diagnosis and prognosis, as well as for developing effective therapeutics.
Although transcriptionally inactive, noncoding RNAs (ncRNAs) regulate the expression of target genes (6,7). Long noncoding RNAs (lncRNAs) are a class of ncRNAs with a length of >200 nucleotides, affecting many cellular processes. Recent studies demonstrated that many lncRNAs play an essential role in cancer pathogenesis, including tumor formation and progression (8). Unlike linear RNAs, circular RNAs (circRNAs) have a unique circular covalently bonded structure, which confers them a high resistance to exonucleases. Due to their conservation, abundance, and tissue specificity, accumulating evidence indicates that circRNAs may serve as specific molecular markers in multiple cancers, including gastric cancer, hepatocellular carcinoma, and lung cancer (9-11). MicroRNAs (miRNAs) are a variety of small ncRNA molecules ranging in size between 15 and 21 nucleotides and exerting post-transcriptional regulation of gene expression and RNA silencing by binding to the 3'UTR regions of target genes. In the past few decades, significant achievements have been made about the correlations between miRNAs and cancers (12-14). A competitive endogenous RNA (ceRNA) hypothesis was first proposed by Salmena et al. to describe the interactions between different types of ncRNAs and postulated that such networks regulate the expression of downstream target genes in multiple malignancies (15). Numerous studies support this hypothesis, confirming the involvement of lncRNA-miRNA-messenger RNA (mRNA) and circRNA-miRNA-mRNA ceRNA networks in various cancers, including CRC (16-18). However, the mechanisms by which these networks may affect CRC progression and prognosis are as yet unclear.
In light of the ceRNA theory, we reconstructed a molecular network potentially implicated in CRC using online data from the National Center for Biotechnology Information Gene Expression Omnibus (NCBI-GEO), which contained 44 circRNAs, 2 lncRNAs, 5 miRNAs, and 36 mRNAs. Following this, based on the ceRNA network, we performed functional enrichment analysis and core gene speculation, for the further construction of a core gene-centered RNA interaction network. Indeed, several differentially expressed RNAs identified have been reported in CRC previously, but the majority have mainly focused on lncRNA or circRNA mediated networks. In our research, we considered the interaction between lncRNAs and circRNAs and constructed a multi-molecule co-mediated ceRNA network. Besides, to specifically explore the molecular mechanisms associated with CRC in the Chinese population, only tumor specimens from Chinese patients were analyzed in all datasets. Our data may inspire new approaches to treatment and prevention.
Methods
Data source
Gene expression profiles of CRC patients and healthy individuals (GSE108153, GSE126093, GSE126094, GSE126092, and GSE41657) were obtained from NCBI-GEO, a free database of microarray/gene profile and next-generation sequencing data. The GSE108153 and GSE126093 datasets contained miRNA microarray data of 21 and 10 paired samples from patients with CRC and healthy individuals, respectively. GSE126094 contained circRNA microarray data of 10 paired samples from CRC patients and 10 healthy individuals. GSE126092 contained data of 10 paired samples from CRC patients and 10 healthy individuals and was used to analyze the lncRNAs. Finally, mRNA profiles were analyzed in the GSE41657 dataset, comprising 25 paired CRC patients and 12 healthy individuals.
Screening of DEmiRNAs, circRNAs, lncRNAs, and mRNAs
R software (version 3.5.2) was utilized to select DEmiRNAs from the GSE108153 and GSE126093 datasets separately. The two series of data were merged, and miRNAs with the same seed sequence and expression pattern were selected as co-expressed miRNAs. The R software was also used to identify DEcircRNAs, lncRNAs, and mRNAs in the GSE126094, GSE126092, and GSE41657 datasets, respectively. For all analyses, we applied a log2FC >2, and an adjusted P value <0.05 was the cut-off for statistical significance. Volcano maps were generated based on the DEcircRNAs, lncRNAs, and mRNAs using the ggplots packages in the R platform.
Construction of the ceRNA network
The Starbase database (http://starbase.sysu.edu.cn/) was used to predict interactions between lncRNA/circRNA and miRNAs. Starbase is an open-source platform for studying miRNA-ncRNA or miRNA-mRNA interaction and contains gene expression data of 32 types of cancers from 10,882 RNA-Seq and 10,546 miRNA-Seq datasets. Moreover, Starbase includes 10 different programs to predict miRNA targets (PITA, RNA22, miRmap, microT, miRanda, PicTar, TargetScan, AgoExpNum, CleaveExpNum, and Pan-Cancer) and was used to identify mRNA targets of the identified miRNAs. The genes that were recognized as targets of the selected miRNAs by more than five prediction programs were considered for further analysis.
Functional enrichment analysis
The Database for Annotation, Visualization, and Integrated Discovery (DAVID) is an online resource platform (available at https://david.ncifcrf.gov/), which allows for systematic and comprehensive analysis of large gene lists. To assess the functional enrichment of the DEmRNAs, GO terms, and KEGG pathways were analyzed using DAVID. P values <0.05 were considered indicative of statistically significant DEmRNA enrichment in KEGG pathways or GO terms.
Construction and analysis of a PPI network
The STRING database (http://stringdb.org) was used to predict potential interactions between DEmRNAs. The results were visualized by Cytoscape 3.7.1. In addition, the CytoHubba tool was used to identify hub genes belonging to the obtained PPI network. Finally, we analyzed PPI subnetworks based on the identified hub-genes.
Data availability
The datasets analyzed during the current study are available in the Gene Expression Omnibus (GEO) repository, http://www.ncbi.nlm.nih.gov/geo/.
Results
Differentially expressed ncRNAs in CRC
DEmiRNAs in CRC patients and paired healthy controls from all datasets were selected, using a P value <0.05 and a log2FC >2 as cut-off criteria. Eight and 103 DEmiRNAs were extracted from the GSE108153 and GSE126093 microarray datasets, respectively. Five of these DEmiRNAs, 3 up-regulated and 2 downregulated in CRC compared to healthy subjects, were common to both datasets (Figure 1).
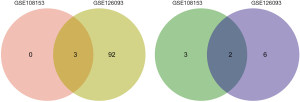
In the GSE126094 dataset, 245 differentially expressed circRNAs (DEcircRNAs) were found, of which 237 were up-regulated and 8 downregulated in CRC patients compared to healthy individuals. Then, GSE126092 microarray data were used to identify differentially expressed lncRNAs (DElncRNAs). As a result, 555 up-regulated and 1,111 downregulated lncRNAs were selected. Further, the GSE41657 microarray dataset was used to identify differentially expressed mRNAs (DEmRNAs), revealing 260 up-regulated and 674 downregulated mRNAs in CRC patients. The selected variations had a log2FC >2 and an adjusted P value <0.05 (Figure 2). At the same time, the differentially expressed RNAs were listed in supplementary (Figure S1).
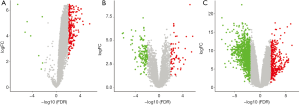
Construction of a ceRNA regulatory network in CRC
To explore pathogenetically relevant interactions between DEcircRNAs, DElncRNAs, DEmiRNAs, and DEmRNAs, we constructed a lncRNA/circRNA-miRNA-mRNA ceRNA regulatory network for CRC. First, we predicted the interaction pairs between the five selected DEmiRNAs and the DEcircRNAs, lncRNAs, and mRNAs using the StarBase database. By merging the three datasets, 44 overlapping DEcircRNAs (43 up-regulated and 1 downregulated), 2 overlapping DElncRNAs, and 36 overlapping DEmRNAs (13 up-regulated and 23 downregulated) were obtained and imported into Cytoscape for analysis. The constructed network is shown in Figure 3.
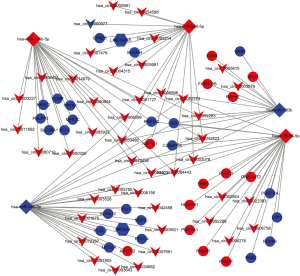
Functional analysis of DEmRNAs
To investigate the biological function of the 36 DEmRNAs, data were clustered by Gene Ontology (GO) and Kyoto Encyclopedia of Genes and Genomes (KEGG) pathway analyses using the DAVID software. P values <0.05 were considered to be statistically significant (Figure 4). DEmRNAs were classified based on three functional criteria: biological process (BP), molecular function (MF), and cellular component (CC). According to BP ontology, DEmRNAs were mainly involved in the repression of transcription from the RNA polymerase II promoter. Based on CC ontology, DEmRNAs were mainly enriched in the nuclear compartment. According to MF ontology, DEmRNAs were enriched in sequence-specific DNA binding and RNA polymerase II core promoter proximal region sequence-specific binding. Furthermore, according to the KEGG pathway enrichment analysis, DEmRNAs were implicated in the oxytocin signaling pathway.
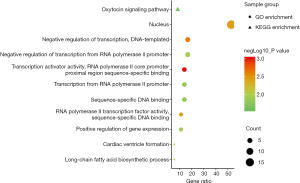
Construction of a protein-protein interaction (PPI) network based on DEmRNAs
To discover the core molecules of cellular processing at the protein level, we used the Search Tool for the Retrieval of Interacting Genes (STRING), a database of known and predicted protein interactions, to identify functional PPIs based on the 36 DEmRNAs. A network consisting of 32 nodes and 76 edges, with an enrichment P value of 1.3×10−6, was obtained. Proteins with essential roles in the network were identified based on the scores assigned by Cytoscape to the nodes and edges (https://cytoscape.org/) (Figure 5). Both the size and color shade of nodes reflect the degree of centrality. In particular, the larger and darker a node, the higher the number of interactions. The thickness of edges between nodes represents the statistical significance of the interactions. In our network, several node proteins, including KLF4, SOX4, PDGFRA, MEF2C, FOXQ1, FOXP2, TRIB3, NAPAL1, and QKI, showed a strong association with other node proteins, reflecting high hub degrees. Therefore, they may participate in the core role of PPIs.
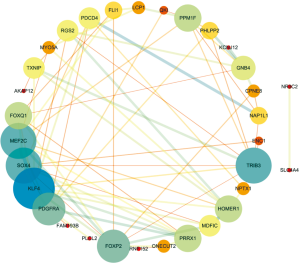
An in-depth analysis of the PPI network was performed using the Cytoscape plugin CytoHubba, which provides 12 topological methods for ranking the properties of nodes in the network. As a result, potential functionally relevant proteins can be accurately selected based on the scores assigned to node proteins using different algorithms (Figure 6). The proteins assigned high scores with all algorithms were considered to be potentially relevant in CRC. Ten genes (KLF4, SOX4, PDGFRA, MEF2C, FOXQ1, FOXP2, TRIB3, PRRX1, PPM1F, and HOMER1) were found to be crucial for PPIs and were named “hub genes”. Based on these results, we established a circRNA/lncRNA-miRNA-hub gene subnetwork, including 28 ceRNA regulatory modules (Figure 7). This network highlighted hub genes with likely involvement in CRC pathogenesis, which may serve as a useful guide for further targeted research into identifying new therapeutic targets or biomarkers. At the same time, these highlighted genes will also become the target genes for further experimental verification.
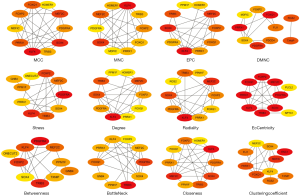
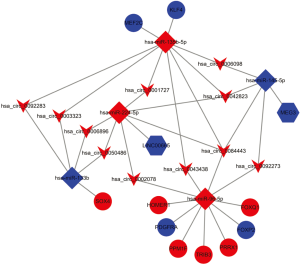
Discussion
In recent years, owing to the ceRNA theory and the use of TCGA or NCBI-GEO databases, growing knowledge has been gained on the interactions between mRNAs, lncRNAs, circRNAs, and miRNAs with differential expression in tumor and adjacent non-tumor tissues. These studies mainly focused on lncRNA- or circRNA-based ceRNA networks. The mechanism by which lncRNAs and circRNAs affect miRNA action on downstream target genes in CRC is not clear. In this study, we identified distinct circRNAs, lncRNAs, mRNAs, and miRNAs implicated in CRC, providing novel insights into tumor prognosis. Moreover, a circRNA/lncRNA-miRNA-mRNA regulatory network was constructed by integrated bioinformatics analysis.
circRNAs are a new class of endogenous ncRNAs, extracted initially from RNA viruses in 1976 and initially considered as the result of splicing errors (19). Later on, owing to deep RNA sequencing technology, as well as advancements in bioinformatic analysis, circRNAs have become a research hotspot. Multiple functions have been attributed to circRNAs, including the regulation of transcription (20), competition with pre-mRNA splicing, miRNA sponging (21-23), and formation of proteins involved in various BPs (24). Recent studies have revealed that some circRNAs are associated with tumor initiation, progression, and metastasis (25,26). In particular, increasing evidence indicates a role of circRNAs in the initiation and progression of CRC. Tian et al. analyzed 192 pairs of CRC tissues and blood samples and found that hsa_circ_0004585 is significantly up-regulated in both tumors and peripheral blood of CRC patients (27). Moreover, the latter study showed that hsa_circ_0004585 is positively correlated with tumor size, indicating a role of this circRNA in CRC carcinogenesis and metastasis. Furthermore, Li et al. found that the expression of hsa_circ_102958 significantly increased in CRC tissues, compared to adjacent control tissues. Moreover, CCK8, colony formation, and Transwell assays revealed that hsa_circ_102958 promotes CDC25B expression by sponging miR-585 in CRC (28). In our study, we analyzed data from CRC and normal colorectal tissue samples and found that 44 circRNAs were involved in the ceRNA network, based on differential expression of circRNAs and on the identification of circRNA-miRNA interaction pairs. In addition, 11 circRNAs were selected after establishing a circRNA/lncRNA-miRNA-hub gene network, and it was found that none of them was previously described to be associated with CRC.
Recently, lncRNAs have received attention in relation to various cancers, including CRC. For example, lncRNA-MEG3, a maternally imprinted gene containing ten exons and encoding an approximately 1.6-Kb long ncRNA (29), is abundantly expressed in many tissues but is downregulated in a variety of primary human cancers (30). Another study found that MEG3 levels are decreased in CRC tissues and evidently associated with histological grade, tumor invasion depth, and TNM stage (31). Our data on differential lncRNA expression are consistent with that of the above study. In addition, Wang et al. found that MEG3 expression was downregulated in CRC tissues and cell lines, especially in oxaliplatin-resistant tissues and cells. Moreover, the latter study revealed a novel MEG3/miR-141/PDCD4 regulatory axis that could restrain oxaliplatin resistance in CRC (32). In this study, we identified a possible regulatory interaction between MEG3 and hsa-miR-145-5p. However, due to a lack of experimental validation and regulation of circRNA upstream, the target mRNA is temporarily undetermined. A previous study had identified another lncRNA, LINC00665, as a regulator of viability, apoptosis, and autophagy via the miR-186-5p/MAP4K3 axis in hepatocellular carcinoma (33). We reasoned that LINC00665 could also affect the expression of target genes in CRC by interacting with miR-224-5p. On the other hand, the mechanism by which lncRNAs and circRNAs together affect downstream target genes has not been clarified.
In the ceRNA network constructed in this study, 5 miRNAs were differentially expressed in CRC patients compared to healthy individuals. Previous research showed that aberrantly expressed miRNAs regulate the expression of multiple oncogenes and tumor suppressors and have been widely associated with cancer development (34). Sun et al. demonstrated that lincRNA-SNHG15 promotes the development of colorectal carcinoma by functioning as a ceRNA controlling the miR-141/SIRT1/Wnt/β-catenin axis (35). Another study found that LINC00858 promotes tumor cell proliferation, migration, and invasion by acting as a ceRNA for miR-22-3p in CRC (36). Of the five selected DEmiRNAs involved in our ceRNA network, four have been reported to play important roles in the initiation and development of CRC, namely hsa-miR-135b-5p, hsa-miR-145-5p, hsa-miR-224-5p, and hsa-miR-133b (37-40). However, to date, a role for hsa-miR-96-5p in CRC has not been reported. One study showed that hsa-miR-96-5p may serve as a marker to distinguish human ovarian cancer tissues from their normal counterparts, with a 97% sensitivity and a 92% specificity, suggesting that this miRNA is involved in ovarian cancer development (41). In addition, Piotto et al. indicated that hsa-miR-96-5p directly regulates the expression of target genes involved in homologous recombination and the non-homologous end-joining pathway (i.e., RAD51, BRCA2, PRKDC, XRCC5, and LIG1), thus affecting the efficacy of radiotherapy in non-small cell lung cancer (42). This evidence highlights the importance of hsa-miR-96-5p in pan-cancer events and indicates that it is inextricably linked to CRC initiation and development.
To further explore the core genes participating in the regulatory network, functional enrichment analyses were performed, and a PPI network was established. Finally, a circRNA/lncRNA-miRNA-hub gene network, including 28 ceRNA regulatory modules, was constructed, and 10 hub genes were identified. The results showed that the selected DEmRNAs were significantly enriched in the oxytocin signaling pathway. Deiner et al. found that mice lacking the netrin-1 receptor, which is deleted in CRC, exhibited abnormalities in hypothalamic-controlled neuroendocrine pathways, such as oxytocin secretion, compared to normal mice (43). Also, Cassoni et al. showed that oxytocin and the oxytocin-analog F314 inhibit tumor cell proliferation and growth in colon and mammary carcinomas in mice (44). Therefore, the identified DEmRNAs are involved in many important CRC-associated biological functions and metabolic pathways. Moreover, except for HOMER1, PPM1F, and TRIB3, on which no evidence has been previously published, many reports have shown that the hub genes identified in this study are abnormally expressed in CRC, due to upstream lncRNA- or circRNA-mediated miRNA changes, ultimately affecting cell proliferation, tumor metastasis, and drug resistance (45-49).
However, our study presents several limitations. First, as we analyzed pre-existing data, our conclusions may be affected by certain errors and uncontrollable factors, such as sample age classification and tumor staging, and need to be experimentally verified, with particular regard to previously unreported hub genes. Second, it is still unclear how lncRNAs and circRNAs may act in combination to control miRNA expression within the constructed lncRNA/circRNA-miRNA-hub gene network. To validate these findings, we will perform in vitro and in vivo experiments focusing on a larger number of clinical samples. Finally, our experimental data can be combined with the clinical data of patients with CRC to explore further the effects of hub genes on the prognosis and treatment of patients. All data analyzed in this study were from Chinese subjects and may inspire future research on the mechanism underlying CRC development in the Chinese population.
Conclusions
We identified several noncoding RNAs with a possible pathogenetic role in CRC and built a CRC-specific ceRNA network based on our bioinformatics analysis. Our study provides novel insights into the molecular events implicated in CRC.
Acknowledgments
The authors would like to thank the NCBI-GEO, DAVID, STRING, and Starbase databases for valuable data. Similarly, we would like to thank Editage (www.editage.cn) for English language editing.
Funding: None.
Footnote
Conflicts of Interest: Both authors have completed the ICMJE uniform disclosure form (available at http://dx.doi.org/10.21037/tcr-19-2973). The authors have no conflicts of interest to declare.
Ethical Statement: The authors are accountable for all aspects of the work in ensuring that questions related to the accuracy or integrity of any part of the work are appropriately investigated and resolved. The study was conducted in accordance with the Declaration of Helsinki (as revised in 2013).
Open Access Statement: This is an Open Access article distributed in accordance with the Creative Commons Attribution-NonCommercial-NoDerivs 4.0 International License (CC BY-NC-ND 4.0), which permits the non-commercial replication and distribution of the article with the strict proviso that no changes or edits are made and the original work is properly cited (including links to both the formal publication through the relevant DOI and the license). See: https://creativecommons.org/licenses/by-nc-nd/4.0/.
References
- Wang N, Zeng L, Li Z, et al. Serum miR-663 expression and the diagnostic value in colorectal cancer. Artif. Cells Nanomed Biotechnol 2019;47:2650-3. [Crossref] [PubMed]
- Leijssen LG, Dinaux AM, Kunitake H, et al. The impact of postoperative morbidity on survival in patients with metastatic colon and rectal cancer. J Surg Oncol 2019;120:460-72. [Crossref] [PubMed]
- Siegel RL, Miller KD, Jemal A. Cancer Statistics, 2019. CA Cancer J Clin 2019;69:7-34. [Crossref] [PubMed]
- Miller KD, Nogueira L, Mariotto AB, et al. Cancer treatment and survivorship statistics, 2019. CA Cancer J Clin 2019;69:363-85. [Crossref] [PubMed]
- Chen W, Sun K, Zheng R, et al. Cancer incidence and mortality in China, 2014. Chin J Cancer Res 2018;30:1-12. [Crossref] [PubMed]
- Pardini B, Sabo AA, Birolo G, et al. Noncoding RNAs in extracellular fluids as cancer biomarkers: the new frontier of liquid biopsies. Cancers (Basel) 2019;11:1170. [Crossref] [PubMed]
- Yu XH, Wang HF, Wu JB, et al. Noncoding RNAs derailed: the many influences on the fatty acid reprogramming of cancer. Life Sci 2019;231:116509. [Crossref] [PubMed]
- Maruyama R, Suzuki H. Long noncoding RNA involvement in cancer. BMB Rep 2012;45:604-11. [Crossref] [PubMed]
- Chen J, Li Y, Zheng Q, et al. Circular RNA profile identifies circPVT1 as a proliferative factor and prognostic marker in gastric cancer. Cancer Lett 2017;388:208-19. [Crossref] [PubMed]
- Shang X, Li G, Liu H, et al. Comprehensive circular RNA profiling reveals that hsa_circ_0005075, a new circular RNA biomarker, is involved in hepatocellular carcinoma development. Medicine (Baltimore) 2016;95:e3811. [Crossref] [PubMed]
- Wan L, Zhang L, Fan K, et al. Circular RNA-ITCH suppresses lung cancer proliferation via inhibiting the Wnt/β-catenin pathway. Biomed Res Int 2016;2016:1579490.
- Feng FY, Liang G, Lu WF, et al. Correlation of polymorphisms of UDP-glucuronosyltransferase 1A7 gene to genetic susceptibility of lung cancer. Ai Zheng 2005;24:1085-90. [PubMed]
- Gan TQ, Chen WJ, Qin H, et al. Clinical value and prospective pathway signaling of microRNA-375 in lung adenocarcinoma: a study based on the Cancer Genome Atlas (TCGA), Gene Expression Omnibus (GEO) and bioinformatics analysis. Med Sci Monit 2017;23:2453-64. [Crossref] [PubMed]
- Ganju A, Khan S, Hafeez BB, et al. miRNA nanotherapeutics for cancer. Drug Discov Today 2017;22:424-32. [Crossref] [PubMed]
- Salmena L, Poliseno L, Tay Y, et al. ceRNA hypothesis: the Rosetta Stone of a hidden RNA language? Cell 2011;146:353-8. [Crossref] [PubMed]
- Li R, Yang YE, Jin J, et al. Identification of lncRNA biomarkers in lung squamous cell carcinoma using comprehensive analysis of lncRNA mediated ceRNA network. Artif Cells Nanomed Biotechnol 2019;47:3246-58. [Crossref] [PubMed]
- Li Y, Ma B, Yin Z, et al. Competing endogenous RNA network and prognostic nomograms for hepatocellular carcinoma patients who underwent R0 resection. J Cell Physiol 2019;234:20342-53. [Crossref] [PubMed]
- Yan S, Yue Y, Wang J, et al. LINC00668 promotes tumorigenesis and progression through sponging miR-188-5p and regulating USP47 in colorectal cancer. Eur J Pharmacol 2019;858:172464. [Crossref] [PubMed]
- Sanger HL, Klotz G, Riesner D, et al. Viroids are single-stranded covalently closed circular RNA molecules existing as highly base-paired rod-like structures. Proc Natl Acad Sci USA 1976;73:3852-6. [Crossref] [PubMed]
- Li Z, Huang C, Bao C, et al. Exon-intron circular RNAs regulate transcription in the nucleus. Nat Struct Mol Biol 2015;22:256-64. [Crossref] [PubMed]
- Wang Y, Liu J, Ma J, et al. Exosomal circRNAs: biogenesis, effect and application in human diseases. Mol Cancer 2019;18:116. [Crossref] [PubMed]
- Granados-Riveron, JT, Aquino-Jarquin G. The complexity of the translation ability of circRNAs. Biochim Biophys Acta 2016;1859:1245-51.
- Ashwal-Fluss R, Meyer M, Pamudurti NR, et al. circRNA biogenesis competes with pre-mRNA splicing. Mol Cell 2014;56:55-66. [Crossref] [PubMed]
- Zhao X, Cai Y, Xu J. Circular RNAs: biogenesis, mechanism, and function in human cancers. Int J Mol Sci 2019;20:3926. [Crossref] [PubMed]
- Zhou B, Zheng P, Li Z, et al. CircPCNXL2 sponges miR-153 to promote the proliferation and invasion of renal cancer cells through up-regulating ZEB2. Cell Cycle 2018;17:2644-54. [Crossref] [PubMed]
- Lv C, Sun L, Guo Z, et al. Circular RNA regulatory network reveals cell-cell crosstalk in acute myeloid leukemia extramedullary infiltration. J Transl Med 2018;16:361. [Crossref] [PubMed]
- Tian J, Xi X, Wang J, et al. CircRNA hsa_circ_0004585 as a potential biomarker for colorectal cancer. Cancer Manag Res 2019;11:5413-23. [Crossref] [PubMed]
- Li R, Wu B, Xia J, et al. Circular RNA hsa_circRNA_102958 promotes tumorigenesis of colorectal cancer via miR-585/CDC25B axis. Cancer Manag Res 2019;11:6887-93. [Crossref] [PubMed]
- Zhang X, Rice K, Wang Y, et al. Maternally expressed gene 3 (MEG3) noncoding ribonucleic acid: isoform structure, expression, and functions. Endocrinology 2010;151:939-47. [Crossref] [PubMed]
- Balik V, Srovnal J, Sulla I, et al. MEG3: a novel long noncoding potentially tumour-suppressing RNA in meningiomas. J Neurooncol 2013;112:1-8. [Crossref] [PubMed]
- Yin DD, Liu ZJ, Zhang E, et al. Decreased expression of long noncoding RNA MEG3 affects cell proliferation and predicts a poor prognosis in patients with colorectal cancer. Tumour Biol 2015;36:4851-9. [Crossref] [PubMed]
- Wang H, Li H, Zhang L, et al. Overexpression of MEG3 sensitizes colorectal cancer cells to oxaliplatin through regulation of miR-141/PDCD4 axis. Biomed Pharmacother 2018;106:1607-15. [Crossref] [PubMed]
- Shan Y, Li P. Long intergenic non-protein coding RNA 665 regulates viability, apoptosis, and autophagy via the miR-186-5p/MAP4K3 axis in hepatocellular carcinoma. Yonsei Med J 2019;60:842-53. [Crossref] [PubMed]
- Zamani M, Hosseini SV, Mokarram P. Epigenetic biomarkers in colorectal cancer: premises and prospects. Biomarkers 2018;23:105-14. [Crossref] [PubMed]
- Sun X, Bai Y, Yang C, et al. Long noncoding RNA SNHG15 enhances the development of colorectal carcinoma via functioning as a ceRNA through miR-141/SIRT1/Wnt/β-catenin axis. Artif Cells Nanomed Biotechnol 2019;47:2536-44. [Crossref] [PubMed]
- Sha QK, Chen L, Xi JZ, et al. Long noncoding RNA LINC00858 promotes cells proliferation, migration and invasion by acting as a ceRNA of miR-22-3p in colorectal cancer. Artif Cells Nanomed Biotechnol 2019;47:1057-66. [Crossref] [PubMed]
- Moghadamnia F, Ghoraeian P, Minaeian S, et al. MicroRNA expression and correlation with mRNA levels of colorectal cancer-related genes. J Gastrointest Cancer 2020;51:271-9. [Crossref] [PubMed]
- Li J, Zhong Y, Cai S, et al. MicroRNA expression profiling in the colorectal normal adenoma carcinoma transition. Oncol Lett 2019;18:2013-8. [PubMed]
- Moreno EC, Pascual A, Prieto-Cuadra D, et al. Novel molecular characterization of colorectal primary tumors based on miRNAs. Cancers (Basel) 2019;11:346. [Crossref] [PubMed]
- Tang Y, Zhao Y, Song X, et al. Tumor‐derived exosomal miRNA‐320d as a biomarker for metastatic colorectal cancer. J Clin Lab Anal 2019;33:e23004. [Crossref] [PubMed]
- Wang L, Zhu MJ, Ren AM, et al. A ten-microRNA signature identified from a genome-wide microRNA expression profiling in human epithelial ovarian cancer. PLoS One 2014;9:e96472. [Crossref] [PubMed]
- Piotto C, Biscontin A, Millino C, et al. Functional validation of miRNAs targeting genes of DNA double-strand break repair to radiosensitize non-small lung cancer cells. Biochim Biophys Acta Gene Regul Mech 2018;1861:1102-18.
- Deiner MS, Sretavan DW. Altered midline axon pathways and ectopic neurons in the developing hypothalamus of netrin-1- and DCC-deficient mice. J Neurosci 1999;19:9900-12. [Crossref] [PubMed]
- Cassoni P, Sapino A, Papotti M, et al. Oxytocin and oxytocin-analogue F314 inhibit cell proliferation and tumor growth of rat and mouse mammary carcinomas. Int J Cancer 1996;66:817-20. [Crossref] [PubMed]
- Slattery ML, Mullany LE, Sakoda LC, et al. The MAPK-signaling pathway in colorectal cancer: dysregulated genes and their association with microRNAs. Cancer Inform 2018;17:1176935118766522. [Crossref] [PubMed]
- Lei X, Li L, Duan X. Long noncoding RNA ABHD11-AS1 promotes colorectal cancer development through regulation of miR-133a/SOX4 axis. Biosci Rep 2018;38:BSR20181386. [Crossref] [PubMed]
- Chen G, Han N, Li G, et al. Time course analysis based on gene expression profile and identification of target molecules for colorectal cancer. Cancer Cell Int 2016;16:22. [Crossref] [PubMed]
- Xie Y, Zhao J, Liang Y, et al. MicroRNA-10b controls the metastasis and proliferation of colorectal cancer cells by regulating Krüppel-like factor 4. Artif Cells Nanomed Biotechnol 2019;47:1722-9. [Crossref] [PubMed]
- Wang H, Deng Z, Chen X, et al. Downregulation of miR-222-3p reverses doxorubicin-resistance in LoVo cells through up-regulating forkhead box protein P2 (FOXP2) protein. Med Sci Monit 2019;25:2169-78. [Crossref] [PubMed]