Analysis of differentially expressed mRNAs and the prognosis of cholangiocarcinoma based on TCGA database
Introduction
Cholangiocarcinoma (CCA) is an abnormally aggressive malignant tumor originating in the bile duct epithelium and accounts for 10–20% of primary liver tumors. It is the second most common primary liver cancer, with a 5-year survival rate of only 5% (1). Recently, the incidence of CCA has increased each year. However, the incidence of CCA is occult, and when symptoms do appear, it is often impossible to operate (2-4). In most cases, the diagnosis of CCA in the early resectable stage is uncommon due to an insufficient understanding of the risk factors and the lack of accurate screening tools. CCA has a high incidence rate in many Asian countries, including China. At present, the carcinoembryonic antigen (CEA) and the carbohydrate antigens 19-9 (CA 19-9) and 125 (CA 125) are used clinically as serum markers for CCA; however, they have low sensitivity and specificity and are not adequate for providing an early detection method. For CA19-9, the most recent data resulting from a large meta-analysis that examined the distinction between CCA and healthy controls or patients with benign biliary disease, indicated pooled sensitivity and specificity of 72% and 84%, respectively (5). Similarly, the diagnostic sensitivity and specificity of CEA range from 42% to 85% and 70% to 89% respectively (6-8). In recent years, the research on non-coding RNAs as biomarkers have gradually increased. Circulating miR-21 is one of the most well-defined microRNAs and has the potential to be a biomarker for the diagnosis of CCA. Meanwhile, the study also showed that serum miR-21 levels were also positively correlated with tumor stage (TNM criteria) and poor survival. The level of serum miR-21 decreased after tumor resection, highlighting the value of circulating miR-21 as both a diagnostic tool and a putative prognostic biomarker (9). Non-coding RNAs with similar effects for CCA also include miR-26a, miR-106a and miR-150 (10,11). However, since most of the data on using circulating nucleic acids as biomarkers for diagnosis and prognosis of CCA come from conceptual experimental verification studies, further international assessment is urgently needed to confirm their potential clinical value. Other promising biomarkers for circulating diagnosis and prognosis of CCA include cytokeratin 19 fragment (CYFRA 21-1), matrix metalloproteinase 7 (MMP-7) and osteopontin. Patients with intrahepatic CCA have increased CYFRA 21-1 compared with patients with benign biliary tract disease, and CYFRA 21-1 has a higher diagnostic value than CA19-9 and CEA (12). Also, the serum level of CYFRA 21-1 is related to the stage of the disease and is an independent predictor of the survival of patients without recurrence (12,13). Serum levels of MMP-7 in patients with CCA were also increased compared with benign biliary diseases, but its correlation with survival prognosis is currently unclear (14,15). Also, studies have shown that high levels of osteopontin in patients with CCA before and after surgery are associated with a decrease in overall survival (OS) after tumor resection (16). Overall, CCA currently lacks sensitive early diagnostic markers and effective prognostic biomolecules. Furthermore, the research on biomarkers is still scarce and needs further exploration. Our study uses a combination of bioinformatics and basic experiments, in which a protein interaction network is constructed to further narrow the scope of the key mRNAs. The purpose is to screen out key node molecules that regulate the poor prognosis of patients with CCA, and serve as targets for further mechanism research and therapeutic intervention.
Methods
Datasets and materials
We downloaded the mRNA expression data of CCA from The Cancer Genome Atlas (TCGA) database: 44 cases with genomic and corresponding clinical information were obtained from TCGA, including 35 cases of cancer tissue and 9 cases of normal tissue. Clinical information included age, gender, grade, stage, Eastern Cooperative Oncology Group (ECOG) score, family cancer history, OS time, and survival status. The study was conducted in accordance with the Declaration of Helsinki (as revised in 2013). The study was approved by the Ethics Committee of the General Hospital of Northern Theater Command [No.: k(2017)12] and informed consent was taken from all the patients. The total number of samples was 36, including 27 cancer tissues and 9 adjacent tissues. PLK1 antibody (ab17056) and Aurora B antibody (ab2254) were purchased from Abcam to check the expression of the corresponding molecules in the tissue by immunochemistry and Western blot.
Differential gene analysis
The R package, “edgeR”, was used to integrate the two sets of expression profile data to perform differential expression gene analysis. The genes with | log2 FC | >2 and false discovery rate (FDR) <0.05 were considered differentially expressed genes.
Gene function enrichment analysis
Through the R package, “ClusterProfiler” The Gene Ontology (GO) and Kyoto Encyclopedia of Genes and Genomes (KEGG) functions enriched by genes in the module of interest were obtained.
Construction of protein interaction network and screening of Hub genes
Cytoscape was used to characterize the interaction network between the differential genes and disease genes and to map the key protein interaction networks (highest confidence, 0.900). CytoHubba was used to calculate the top 10 key genes for the score.
Survival analysis
We divided the mRNAs into high and low expression groups by using the median score of the top 10 differential mRNAs combined with clinical data as the cutoff value; the log-rank test was used to test the differences in survival curves between the two groups and to screen for prognostic differential genes.
Immunohistochemistry (IHC)
IHC and results judgment were performed strictly in accordance with the immunochemistry operation process and standard specifications. PLK1 and AURKB staining showed brownish-yellow particles, which were cytoplasmic or nuclear and cytoplasmic. The immunohistochemically stained sections were observed under a microscope. An imaging device was used to take pictures of the fields of each group, and we randomly selected five classic fields after the picture. Pathologists scored them on the percentage of positively stained cells (A) and the intensity of staining of stained cells (B). The scoring procedure is described below.
(A) The number of positively stained cells was determined by first observing 5 high-power fields (+200) on each section and calculating the percentage of positive cells. Scoring was performed according to the percentage of positive cells as follows: <5%=0 points; 5–25%=1 point; 26–50%=2 points; 51–75%=3 points; and 76–100%=4 points.
(B) The scoring for positive coloring intensity was performed a follows: colorless =0 points; light yellow =1 point; brown yellow =2 points; and tan =3 points. The score of the two groups were added to obtain a total score for comparison.
Western blot
Extract the protein in the tissue, determine the protein concentration by quantitative method of BCA (bicinchoninic acid), and adjust each group of protein to the same concentration. After mixing with the loading buffer, the protein is denatured and transferred to the membrane. After blocking the non-specific antigen, the primary antibody was added and incubated overnight. The secondary antibody was diluted at a ratio of 1:2,000, and GAPDH was used as an internal control. The image was exposed, and was taken with Bio-RAD gel imaging system.
qRT-PCR
The RNA kit was used to extract the total RNA from the tissue samples, and the operation was carried out according to the instructions. Measure the total RNA concentration, synthesize cDNA according to the instructions of the reverse transcription synthesis kit, and then use the cDNA as a template to amplify the extracted RNA in the PCR instrument for reverse transcription. Real-time quantitative PCR was performed using SYBR Green dye method.
Statistical methods
All statistical analyses were performed using SPSS 19.0 software. Statistical analysis was conducted using a t-test or Bonferroni multiple comparisons test. A P value of less than 0.05 was considered statistically significant.
Results
Identification of differential mRNA genes
EdgeR analysis was used to analyze the different mRNA expressions between the normal tissues and tumor tissues (P<0.01); 5,561 differentially expressed genes were found, 3,473 of which were differentially upregulated mRNAs and 2,088 of which were differentially downregulated mRNAs (Figure 1A,B).
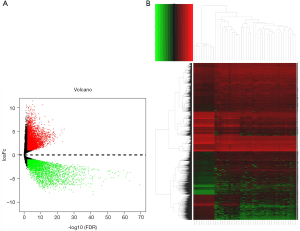
Gene set enrichment analysis
To further understand the effect of the screened differential genes on CCA, gene enrichment analysis was performed using the Database for Annotation, Visualization and Integrated Discovery (DAVID) which includes GO and KEGG pathway enrichment analyses. Enrichment analysis was performed on up- and down-regulated genes. Through GO enrichment analysis of the upregulated genes, we observed many enriched gene sets. In terms of biological processes, they were enriched in cell adhesion and cell division. In terms of cellular components, they were significantly enriched in concentrated chromosomal motility and kinesin complexes. In terms of molecular function, they were mainly enriched in microtubule motility (Figure 2A). Through GO enrichment analysis of downregulated genes, we found that downregulated genes were mainly enriched in heterologous metabolism, exosomes, and oxidoreductase activity (Figure 2B).
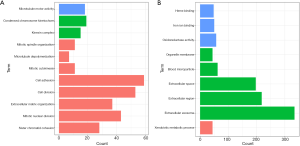
We further analyzed the functional significance of differential mRNAs in the development of CCA through KEGG pathway analysis and discovered that upregulated genes were significantly enriched in the cancer pathway and cell cycle (Figure 3A). Meanwhile, the downregulated genes were enriched in metabolic pathways and biosynthesis of antibiotics (Figure 3B).
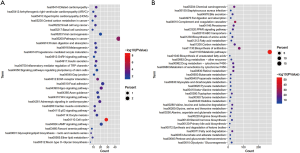
Protein interaction network
A protein interaction network diagram of differentially expressed genes was constructed. The top 10 genes were selected as central genes according to degree and included CDK1, CDC20, CCNB1, CCNB2, CCNA2, BUB1, KNG1, PLK1, AURKB, and CDCA8 (Table 1). Subsequently, the hub genes were submitted to STRING again to verify their interaction. The protein interaction network consisted of 10 nodes and showed the protein interactions between the closer hub genes (Figure 4). Then, the survival analysis was determined by a combination of score and clinical data, and the node genes that were closely related to survival prognosis were selected for verification.
Table 1
Rank | Name | Score |
---|---|---|
1 | CDK1 | 87 |
2 | CDC20 | 76 |
3 | CCNB1 | 75 |
4 | CCNB2 | 72 |
5 | CCNA2 | 68 |
6 | BUB1 | 65 |
7 | KNG1 | 65 |
8 | PLK1 | 63 |
9 | AURKB | 63 |
10 | CDCA8 | 62 |
CDK1, cyclin-dependent kinases 1; CDC20, Cell division cyclin 20; CCNB1, CyclinB1; CCNB2, CyclinB2; CCNA2, CyclinA2; BUB1, budding uninhibited by benzimidazoles 1; KNG1, kininogen 1; PLK1, Polo-like kinase 1; AURKB, aurora kinase B; CDCA8, Cell division cycle associated 8.
Survival analysis
We made a matrix of differential mRNA expression and clinical survival time for the survival analysis. Then, we took the differential mRNAs with a P value <0.05 and obtained two differentially expressed genes: AURKB and PLK1 (Figure 5A,B,C,D,E,F,G,H,I,J).
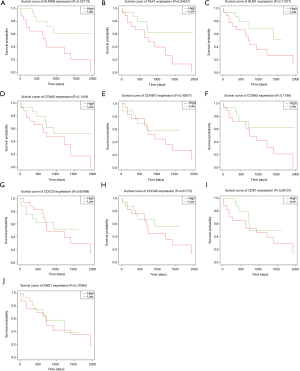
Expression of AURKB and PLK1 in CCA tissues and adjacent tissues
Gene expression showed that the expression of AURKB (Figure 6A) in cancer tissues (staining scores, 3.67±1.27) was higher than that in adjacent tissues (staining scores, 2.56±1.13). The expression of PLK1 (Figure 6B) in cancer tissues (staining scores, 4.96±1.16) was higher than that in adjacent tissues (staining scores, 3.67±1.41). The difference was statistically significant (P<0.05). The immunohistochemical staining is shown in Figure 7. Also, we selected three pairs of cancer and adjacent tissue samples for Western blot experiments, the results suggested that the overall expression of PLK1 and AURKB in cancer tissues is higher than that in adjacent tissues (Figure 8). The qRT-PCR results showed that the expression of PLK1 and AURKB in cancer tissues at the gene level was also higher than that in adjacent tissues (Figure 9).
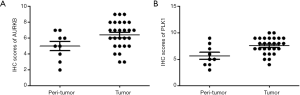
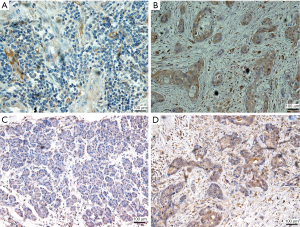
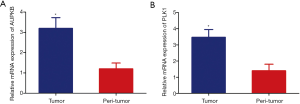
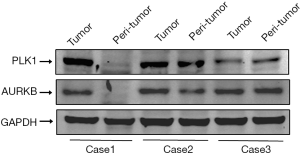
Discussion
Although surgical resection is the primary treatment for CCA, most patients are found to already be in an advanced stage and are therefore unable to undergo an operation. Thus, the early diagnosis of CCA is critical. Due to its occult clinical features and limited treatment measures, the prognosis of this malignant tumor is also poor. Therefore, it is critical to identify one or more biomarkers that could clarify the disease stage and prognosis. Carbohydrate antigen 19-9 (CA19-9) and cancer antigen 125 (CA125) have been widely used in most routine early detection tests for CCA. However, they have a broad range of sensitivity (50–90%) and specificity (54–98%) (17,18). Meanwhile, the increase in CA19-9 levels is also related to other metastatic diseases, and this is one of the reasons for its poor specificity as a serum biomarker for early diagnosis of CCA.
This study processed large amounts of data through bioinformatics methods to obtain more information about differentially expressed genes. In all, 5,561 differentially expressed genes were identified, 3,473 of which were differentially upregulated mRNAs, and 2,088 of which were differentially down-regulated mRNAs. GO enrichment analysis and KEGG enrichment analysis showed upregulated differential genes were significantly enriched in cell cycle and mitosis-related biological processes. We combined the survival data of 44 samples with the expression of the top ten key genes screened by CytoHubba, and performed a Kaplan–Meier survival analysis. Results from comparing the survival time between gene high and low expression groups, the results showed that the expression levels of Aurora kinase B (AURKB) and Polo-like kinase 1 (PLK1) were significantly correlated with OS. Results of the IHC also showed the expression levels of AURKB and PLK1 were significantly negatively correlated with OS. Gene expression also showed that the expression of AURKB and PLK1 in cancer tissues was higher than that in adjacent tissues. These findings suggest that the bioinformatics analysis method can provide a reliable basis for early clinical diagnosis and screening of prognostic biomarkers. Furthermore, AURKB and PLK1, as two key nodes of differentially expressed genes, can be used as potential early diagnostic tools and prognostic biomarkers.
Aurora kinases and Polo-like kinases are two crucial regulatory proteins in mitosis (19). Aurora kinases are a class of evolutionarily highly conserved serine/threonine kinases, in which the AURKB gene is the catalytic subunit of the chromosomal passenger complex (20). In recent years, research on AURKB in tumor-related fields has increased. Studies have shown that AURKB participates in the development of breast cancer, and its expression is related to the prognosis of breast cancer (21). In non-small cell lung cancer (NSCLC), overexpression of AURKB is associated with poor patient prognosis. It has been linked to the inhibition of p53-related pathways, which thereby promotes the proliferation of NSCLC cells, making it a possible mechanism of action (22). In colorectal cancer (CRC), AURKB has also been proven to be an essential oncogenic factor, which promotes drug resistance and progression of CRC (23,24). These studies collectively indicate that AURKB promotes canceration and is related to drug resistance, and that patients with high AURKB expression are more likely to have a poor prognosis (25). Polo-like kinases are a family of serine/threonine kinases that participate in multiple biological processes, including mitosis, meiosis, and cytokinesis (26). Among them, PLK1 is a vital regulator of the eukaryotic cell cycle (27-29). PLK1 activity gradually increases during mitosis, reaches a peak at the G2/M phase, and enters the mitotic phase (30). PLK1 has different localization in different stages of mitosis, which determines its corresponding functions. PLK1 also acts as a mitotic switch to mediate repair after DNA damage, reducing genetic mutations caused by DNA damage (19). Similarly, PLK1 is highly expressed in most human cancers, and its overexpression correlates with poor prognosis in cancer patients (31,32). The above findings are consistent with the results of functional enrichment analysis in this study, suggesting that AURKB and PLK1 molecules play key roles in regulating the proliferation of bile duct cancer cells.
Conclusions
Through bioinformatics analysis based on the TCGA database and verification of clinical tissue samples, we found that AURKB and PLK1 genes are differentially expressed in patients with CCA. These genes are closely related to the patients’ prognosis and could potentially be used as biomarkers for early diagnosis and prognosis. Furthermore, the bioinformatics analysis method can provide a reliable basis for early clinical diagnosis and the screening of prognostic biomarkers.
Acknowledgments
We thank our anonymous reviewers for their valuable comments on the manuscript, which contributed greatly to the improvement of the article.
Funding: This work was supported by grants from
Footnote
Data Sharing Statement: Available at http://dx.doi.org/10.21037/tcr-20-812
Conflicts of Interest: All authors have completed the ICMJE uniform disclosure form (available at http://dx.doi.org/10.21037/tcr-20-812). The authors have no conflicts of interest to declare.
Ethical Statement: The authors are accountable for all aspects of the work in ensuring that questions related to the accuracy or integrity of any part of the work are appropriately investigated and resolved. The study was conducted in accordance with the Declaration of Helsinki (as revised in 2013). The study was approved by the Ethics Committee of the General Hospital of Northern Theater Command [No.: k(2017)12] and informed consent was taken from all the patients.
Open Access Statement: This is an Open Access article distributed in accordance with the Creative Commons Attribution-NonCommercial-NoDerivs 4.0 International License (CC BY-NC-ND 4.0), which permits the non-commercial replication and distribution of the article with the strict proviso that no changes or edits are made and the original work is properly cited (including links to both the formal publication through the relevant DOI and the license). See: https://creativecommons.org/licenses/by-nc-nd/4.0/.
References
- Brandi G, Venturi M, Pantaleo MA, et al. Cholangiocarcinoma: Current opinion on clinical practice diagnostic and therapeutic algorithms: A review of the literature and a long-standing experience of a referral center. Dig Liver Dis 2016;48:231-41. [Crossref] [PubMed]
- Squadroni M, Tondulli L, Gatta G, et al. Cholangiocarcinoma. Crit Rev Oncol Hematol 2017;116:11-31. [Crossref] [PubMed]
- Doherty B, Nambudiri VE, Palmer WC. Update on the Diagnosis and Treatment of Cholangiocarcinoma. Curr Gastroenterol Rep 2017;19:2. [Crossref] [PubMed]
- Patel T. Worldwide trends in mortality from biliary tract malignancies. BMC Cancer 2002;2:10. [Crossref] [PubMed]
- Liang B, Zhong L, He Q, et al. Diagnostic accuracy of serum CA19-9 in patients with cholangiocarcinoma: a systematic review and meta-analysis. Med Sci Monit 2015;21:3555-63. [Crossref] [PubMed]
- Li Y, Li DJ, Chen J, et al. Application of joint detection of AFP, CA19-9, CA125 and CEA in identification and diagnosis of cholangiocarcinoma. Asian Pac J Cancer Prev 2015;16:3451-5. [Crossref] [PubMed]
- Ince AT, Yildiz K, Baysal B, et al. Roles of serum and biliary CEA, CA19-9, VEGFR3, and TAC in differentiating between malignant and benign biliary obstructions. Turk J Gastroenterol 2014;25:162-9. [Crossref] [PubMed]
- Loosen SH, Roderburg C, Kauertz KL, et al. CEA but not CA19-9 is an independent prognostic factor in patients undergoing resection of cholangiocarcinoma. Sci Rep 2017;7:16975. [Crossref] [PubMed]
- Liu CH, Huang Q, Jin ZY, et al. Circulating microRNA-21 as a prognostic, biological marker in cholangiocarcinoma. J Cancer Res Ther 2018;14:220-5. [Crossref] [PubMed]
- Cheng Q, Feng F, Zhu L, et al. Circulating miR-106a is a novel prognostic and lymph node metastasis indicator for cholangiocarcinoma. Sci Rep 2015;5:16103. [Crossref] [PubMed]
- Wu X, Xia M, Chen D, et al. Profiling of downregulated blood-circulating miR-150-5p as a novel tumor marker for cholangiocarcinoma. Tumour Biol 2016;37:15019-29. [Crossref] [PubMed]
- Huang L, Chen W, Liang P, et al. Serum CYFRA 21–1 in biliary tract cancers: a reliable biomarker for gallbladder carcinoma and intrahepatic cholangiocarcinoma. Dig Dis Sci 2015;60:1273-83. [Crossref] [PubMed]
- Uenishi T, Yamazaki O, Tanaka H, et al. Serum cytokeratin 19 fragment (CYFRA21-1) as a prognostic factor in intrahepatic cholangiocarcinoma. Ann Surg Oncol 2008;15:583-9. [Crossref] [PubMed]
- Leelawat K, Narong S, Wannaprasert J, et al. Prospective study of MMP7 serum levels in the diagnosis of cholangiocarcinoma. World J Gastroenterol 2010;16:4697-703. [Crossref] [PubMed]
- Leelawat K, Sakchinabut S, Narong S, et al. Detection of serum MMP-7 and MMP-9 in cholangiocarcinoma patients: evaluation of diagnostic accuracy. BMC Gastroenterol 2009;9:30. [Crossref] [PubMed]
- Loosen SH, Roderburg C, Kauertz KL, et al. Elevated levels of circulating osteopontin are associated with a poor survival after resection of cholangiocarcinoma. J Hepatol 2017;67:749-57. [Crossref] [PubMed]
- Chinchilla-López P, Aguilar-Olivos NE, García-Gómez J, et al. Prevalence, Risk Factors, and Survival of Patients with Intrahepatic Cholangiocarcinoma. Ann Hepatol 2017;16:565-8. [Crossref] [PubMed]
- Patel AH, Harnois DM, Klee GG, et al. The utility of CA 19-9 in the diagnoses of cholangiocarcinoma in patients without primary sclerosing cholangitis. Am J Gastroenterol 2000;95:204-7. [Crossref] [PubMed]
- Lens SM, Voest EE, Medema RH. Shared and separate functions of polo-like kinases and aurora kinases in cancer. Nat Rev Cancer 2010;10:825-41. [Crossref] [PubMed]
- Liao Y, Liao Y, Li J, et al. Polymorphisms in AURKA and AURKB are associated with the survival of triple-negative breast cancer patients treated with taxane-based adjuvant chemotherapy. Cancer Manag Res 2018;10:3801-8. [Crossref] [PubMed]
- Naorem LD, Muthaiyan M, Venkatesan A. Integrated network analysis and machine learning approach for the identification of key genes of triple-negative breast cancer. J Cell Biochem 2019;120:6154-67. [Crossref] [PubMed]
- Yu J, Zhou J, Xu F, et al. High expression of Aurora-B is correlated with poor prognosis and drug resistance in non-small cell lung cancer. Int J Biol Markers 2018;33:215-21. [Crossref] [PubMed]
- Subramaniyan B, Kumar V, Mathan G. Effect of sodium salt of Butrin, a novel compound isolated from Butea monosperma flowers on suppressing the expression of SIRT1 and Aurora B kinase-mediated apoptosis in colorectal cancer cells. Biomed Pharmacother 2017;90:402-13. [Crossref] [PubMed]
- Tumino N, Martini S, Munari E, et al. Presence of innate lymphoid cells in pleural effusions of primary and metastatic tumors: Functional analysis and expression of PD-1 receptor. Int J Cancer 2019;145:1660-8. [Crossref] [PubMed]
- Zhu Q, Ding L, Zi Z, et al. Viral-Mediated AURKB Cleavage Promotes Cell Segregation and Tumorigenesis. Cell Rep 2019;26:3657-71.e5. [Crossref] [PubMed]
- Barr FA, Silljé HH, Nigg EA. Polo-like kinases and the orchestration of cell division. Nat Rev Mol Cell Biol 2004;5:429-40. [Crossref] [PubMed]
- Schöffski P. Polo-like kinase (PLK) inhibitors in preclinical and early clinical development in oncology. Oncologist 2009;14:559-70. [Crossref] [PubMed]
- Steegmaier M, Hoffmann M, Baum A, et al. BI 2536, a potent and selective inhibitor of polo-like kinase 1, inhibits tumor growth in vivo. Curr Biol 2007;17:316-22. [Crossref] [PubMed]
- van de Weerdt BC, Medema RH. Polo-like kinases: a team in control of the division. Cell Cycle 2006;5:853-64. [Crossref] [PubMed]
- Tut TG, Lim SH, Dissanayake IU, et al. Upregulated Polo-Like Kinase 1 Expression Correlates with Inferior Survival Outcomes in Rectal Cancer. PLoS One 2015;10:e0129313. [Crossref] [PubMed]
- Ramani P, Nash R, Sowa-Avugrah E, et al. High levels of polo-like kinase 1 and phosphorylated translationally controlled tumor protein indicate poor prognosis in neuroblastomas. J Neurooncol 2015;125:103-11. [Crossref] [PubMed]
- Zhang R, Shi H, Ren F, et al. Misregulation of polo-like protein kinase 1, P53 and P21WAF1 in epithelial ovarian cancer suggests poor prognosis. Oncol Rep 2015;33:1235-42. [Crossref] [PubMed]
(English Language Editors: J. Chapnick and J. Gray)