A gene-set approach to analyze copy number alterations in breast cancer
Introduction
Genomic instability is one of the major driving forces of the accumulation of mutations during tumorigenesis. It leads to the complex mutational landscapes of cancer genomes, which include subtle sequence changes, large-scale chromosome translocations, gene amplifications, and alterations in chromosome number (1). These chromosomal aberrations may result in dysregulation of oncogenes and tumor suppressor genes and affect essential cellular functions, such as proliferation, apoptosis, and cell cycle regulation, leading to the transformation of normal cells into tumor cells (2). Distinct copy number alteration (CNA) patterns were reported to affect cancer-related genes in various types of cancers, suggesting the significance of CNAs in the diversified oncogenic mechanisms underlying cancers (3-6). Moreover, recent studies also showed the association between CNAs and patient survival (7-9). These findings have illuminated the potential role of CNAs as prognostic biomarkers in cancers.
Breast cancer is one of the most common malignancies and ranks as the second cause of cancer death in women (10). It is a heterogeneous disease and can be classified into different subtypes based on the proteomic presence of estrogen receptor (ER), progesterone receptor (PR), and erb-b2 receptor tyrosine kinase 2 (Her2/neu) (11). CNA profiles in breast cancer have been investigated through genome-wide array comparative genomic hybridization (aCGH) and single-nucleotide polymorphism (SNP) microarrays in previous studies (3,12,13), revealing hot spots of CNAs in cancer genomes. For example, chromosomes 1q, 6p, 8q, 11q, 16p, 17q, 19, and 20q were reported to have highly frequent copy number gains, while chromosomes 6q, 16q, 17p, and 22q had copy number losses in breast cancer (14). Some CNAs are associated with specific cancer subtypes. For instance, copy number gains in chromosomes 8q and 12p and losses in 5q and 9p are often detected in basal-like tumors, while gains in 1q and 19q and losses in 10q are frequently present in luminal tumors (15). In addition, a handful of oncogenes and tumor suppressor genes such as Her2, c-Myc, CCND1, and TP53 have been reported to be altered by CNA and proved to be associated with both progression and prognosis of breast cancer (14). These reports strongly suggest the significant involvement of CNAs in breast cancer.
In addition to short DNA mutations in chromosomes (typically affecting single genes), complex alteration events including multiple focal and long-length copy number changes were found in cancer genomes (16-18). These combinations of alteration events can disturb the expression patterns of a large number of genes simultaneously. Although single genes with disturbed copy numbers have been proven to affect cancer progression, inter-gene CNA interaction and the functional landscape of CNAs in breast cancer remain to be explored. Therefore, a systematic analysis to comprehensively investigate the functional effects of CNAs is highly needed.
Several bioinformatics methods (19-21) have been developed to explore the molecular pathways and biological functions underlying different diseases from a gene-set point of view, based on the concept of “Gene Set Enrichment Analysis” (22). These methods typically test the significance of overlap between a set of dysregulated (i.e., differentially expressed) genes and the set of genes sharing a common biological function. The gene-set level methods are a systematic way of identifying the activated functions among groups of samples. Based on gene-set level analysis, here we describe the Gene Set analysis for Copy number Alteration (GSCA) algorithm, which utilizes the gene-set approach to explore the biological functions affected by CNAs and investigate their prognostic effects in breast cancer. By applying GSCA to the breast cancer datasets of The Cancer Genome Atlas (TCGA) (23), we identified functional gene sets that were significantly disturbed by CNAs. The sample-matched gene expression profiles were incorporated to evaluate the influence of CNAs on gene expression. We also performed survival analysis to pinpoint the prognostic CNA-enriched gene sets. The results have demonstrated the potency of our novel method for delineating the complexity of CNA-affected functions in cancers.
Methods
Microarray datasets
A total of 1,045 aCGH data sets of breast tumors were downloaded from TCGA (23), of which 975 were available with clinical information, including the status of ER, PR, and Her2 and survival data. We also collected 529 sample-matched gene expression profiles from TCGA. TCGA pre-normalized (level 3) data was used in this study. The array platforms for copy number and gene expression were Affymetrix Genome-Wide Human SNP Array 6.0 and Agilent 244K Custom Gene Expression G4502A-07, respectively.
Gene sets
We downloaded gene sets from the Molecular Signatures Database (MSigDB v4.0) (22). A total of 7,570 gene sets were used in the analysis, including chemical and genetic perturbations, transcription factor targets, gene ontology terms, oncogenic signatures, and immunologic signatures.
The GSCA algorithm
Model overview
The GSCA algorithm was developed to analyze biological functions affected by CNAs through a gene-set approach. Conceptually, GSCA analyzes the overlap between genes affected by CNAs and genes sharing a common biological function (i.e., a gene set from MSigDB). If there is a significant overlap between the two sets of genes, we define the gene set (or function) as a CNA-affected gene set (or function).
Definition of matrices
To identify the set of genes affected by CNA, we first mapped the CNA to chromosomal regions based on genome coordinates and calculated an estimated copy number (ECN) for each gene. For the i-th sample that harbors the k-th CNA, the estimated copy number (ECNij) of the j-th gene was computed as
where Sik is the measured copy number of the k-th CNA in the i-th sample on a log2 scale, Dijk is the length of the overlapped region between the j-th gene and the k-th CNA of the i-th sample, and Tj is the total length of the j-th gene. Conceptually, ECN measures the average copy number for each gene. Assuming there are in total J genes and P samples in the dataset and Q gene sets, we constructed two index matrices, G = (gj,i)J×P and L = (lj,i)J×P, based on the ECN values to present the gene-level status of copy number gains and losses. The parameter gj,i is set at 1 if ECNij > log2(1.2) (i.e., the gene has more than 2.4 copies), and otherwise set at 0. Similarly, lj,i is set at 1 if ECNij < log2(0.8) (i.e., the gene has less than 1.6 copies), and otherwise at 0. Another index matrix M was constructed to represent the genetic contents of gene sets, defined as M = (mq,j)Q×J, where mq,j is set at 1 if the j-th gene is included in the q-th gene set, otherwise 0.
Gene-set enrichment analysis of CNAs
Based on the three index matrices, G, L, and M, we performed Fisher’s exact test to evaluate the enrichment of genes with copy number gains/losses in each gene set. Let Ki denote the number of genes affected by a gain or decrease in copy number in the i-th sample, Nq be the total number of genes in the q-th gene set, and a be the overlapped genes between the two sets of genes. The significance of overrepresented overlap was assessed by Fisher’s exact test using Eq. [2]:
Benjamini-Hochberg adjustment was performed on the P values to address the issue of multiple comparisons.
Concurrent gene expression analysis
We compared the changes in gene expression with CNA levels to test whether functional (or expressional) changes can be attributed to the enrichment of CNAs in gene sets. For each gene set, samples were divided into three groups (no change in copy number, gain, and loss) based on the significance from Eq. [2]. A P value <0.05 from the Fisher’s exact test was used as the cutoff for significance. Samples with significant overlap between gene expression and genes with positive or negative ECN values were assigned to the gain or loss groups, respectively, and the others were categorized as normal. A Kolmogorov-Smirnov (K-S) test was then performed to compare the cumulative distributions of gene expression profiles between normal and gain/loss samples.
Survival analysis
To explore the prognostic effects of gene sets in terms of their enrichment of CNA, we conducted survival analysis to analyze the association between groups of samples (normal, gain, and loss) and patient survival. Each gene set was independently tested in each of the subtypes of breast cancer, i.e., ER+, Her2+, and triple negative (ER−, PR−, and Her2−). We employed a log-rank test to compare survival curves between groups of samples. Kaplan-Meier curves were used for visualization of survival data.
Results
Identification of CNA-affected biological functions
We devised the GSCA algorithm to identify gene sets with enrichment of CNAs in breast tumors. Briefly, GSCA started by calculating the ECN values, which is simply the length-weighted average of copy number levels, for each gene in a sample; positive and negative ECN values indicate copy number gains and losses, respectively. CNA was determined based on the selection criteria of ECN values. GSCA then tested the gene-set level enrichment of CNAs for each sample by Fisher’s exact test. Figure 1 shows the flowchart of activities during GSCA. The mathematical details are described in the Methods section.
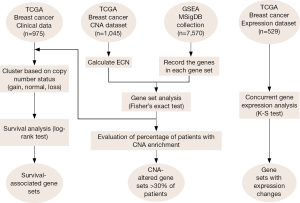
We applied GSCA to the 1,045-sample copy number dataset of breast cancer from TCGA. We found that CNAs occurred in a wide range of genes. The average numbers of genes with copy number gains and losses in a sample were 4,086 and 3,814, respectively (Figure 2A,B). About 32% of human genes exhibited copy number changes in an average of one sample. For gene-set analysis, a gene set was defined as a CNA-affected gene set if the P value of Fisher’s exact test after Benjamini-Hochberg adjustment was less than 0.05. On average, each sample carried ~57 CNA-affected gene sets, of which ~39 and ~18 gene sets were associated with copy number gains and losses, respectively (Figure 2C,D).
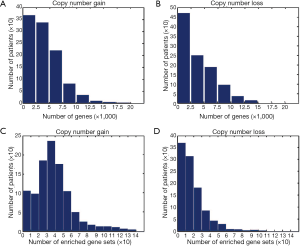
The gene sets affected by CNAs in more than 30% of samples are shown in Table 1. Thirty-five and ten gene sets showed significant associations with copy number gains and losses, respectively. Among the 35 gene sets with a gain in copy number, seven were transcription factor target genes. These results implied that CNAs could affect the ability of a handful of transcriptional factors to regulate gene expression. The gene sets “PATIL_LIVER_CANCER,” originally derived from the up-regulated genes in liver cancer (24), and “RUNNE_GENDER_EFFECT_UP” were found enriched in the largest numbers of samples [760 (72.7%) and 1,037 (99.2%) of all samples, respectively] among gene sets enriched in copy number gain and loss respectively. Interestingly, a large proportion of the identified gene sets was derived from previous studies of CNAs in various types of cancers (Table 1). This suggested the existence of CNA-sensitive regions in cancer genomes.
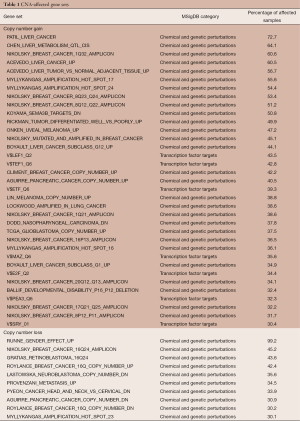
Full table
Realizing that genomic regions with high-level CNAs, including high-level amplification and homozygous deletion, generally indicate novel oncogenes or tumor suppressors, we further explored the functional changes caused by high-level amplification and homozygous deletion. We set the filtering criterion of genes as ECN > log2(2) (i.e., genes with more than four copies) and ECN < log2(0.5) (i.e., genes with less than one copy) to represent the two copy number statuses, respectively. Notably, none of the high-level amplified genes showed frequent enrichment (with a percentage of affected samples >30%) while the homozygously deleted genes were frequently enriched in “RUNNE_GENDER_EFFECT_UP” and “PYEON_CANCER_HEAD_AND_NECK_VS_CERVICAL_DN”. Our data imply that these high-level CNAs are not frequent events in terms of the affected biological functions in breast cancer.
Concurrent gene expression analysis
The identified CNA-affected gene sets (as listed in Table 1) were further tested for concordant association with gene expression changes using the K-S test (Figure 1, right branch). The analysis was conducted in sample-matched CNA and gene expression datasets of 529 breast cancer samples. Forty-four (97.8%) out of the 45 CNA-enriched gene sets exhibited significant concordant changes in expression profiles (K-S test P value <0.05); i.e., genes of a gene set significantly associated with copy number gains (or losses) were generally highly (or lowly) expressed. The gene set “NIKOLSKY BREAST CANCER 8Q12 Q22 AMPLICON” showed the most significant increase in gene expression between samples with a copy number gain and those with normal status (K-S test P value <1×10−20). As depicted in Figure 3A, the cumulative distribution of gene expression was right-shifted in samples with copy number gains (red line) as compared with normal status (blue line). The gene set “NIKOLSKY BREAST CANCER 16Q24 AMPLICON” exhibited the most significantly down-regulated gene expression profiles in the samples with copy number losses (P value <1×10−20; Figure 3B). Overall, the data strongly indicated that CNAs can effectively lead to deregulation of gene expression levels, and in turn modulate the associated biological functions.
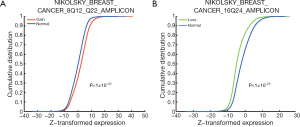
Survival analysis of CNA-affected gene sets
We conducted survival analysis to examine the influences of CNA-disturbed functions on patients’ clinical outcome (Figure 1, left branch). Due to the highly distinct clinical characteristics of breast cancer subtypes, we divided the 975 breast cancer samples into three groups according to the presence of ER, PR, and Her2 (see Methods). In each group, we further analyzed the association between the CNAs and patient survival as described in the Methods section. Her2+ samples were eliminated from our analysis due to a small sample size. A log-rank test was used to evaluate the statistical significance of survival differences. We identified a total of 31 prognostic gene sets (Table 2). In the ER+ cohort, 11 and nine gene sets were identified with survival association from copy number gain/normal and loss/normal comparisons, respectively. Four of the 11 gene sets identified from gain/normal comparison were originally derived from CNA studies in breast cancer, and four of the nine loss/normal gene sets were transcription factor target gene sets (MYC, SF1, USF2, and SP1). The gene sets of “CAMPS COLON CANCER COPY NUMBER UP” (P=0.006, Figure 4A) and “V$SF1_Q6” (P=0.002, Figure 4B) achieved the most significant log-rank P values in gain/normal and loss/normal comparisons, respectively. For the triple negative cohort, eight gene sets were identified with significant prognostic differences between samples with copy number gain versus normal status. Among them, the “ZHAN MULTIPLE MYELOMA HP DN” gene set carried the most significant P value (P=0.004, Figure 4C). Only one gene set, “NIKOLSKY BREAST CANCER 10Q22 AMPLICON,” exhibited a survival difference between samples with copy number loss and normal status (P=0.006, Figure 4D). In addition, in both the ER+ and triple negative groups, several survival associated gene sets were previously reported as associated with copy number or gene expression changes in other cancers such as colon cancer, seminoma, pancreatic cancer, lung cancer, and retinoblastoma (25-29).
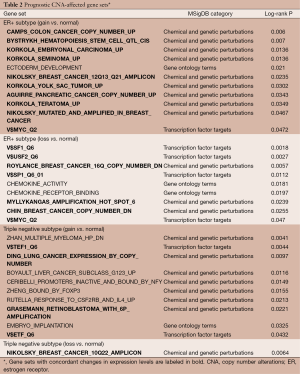
Full table
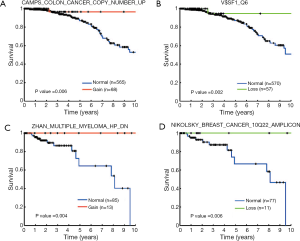
For the identified prognostic gene sets, we again used the K-S test to confirm that the CNAs can effectively lead to expression changes in genes belonging to these gene sets. Gene sets with concordant expression changes are labeled in bold in Table 2. Among the 31 prognostic gene sets, genes of 22 (71.0%) gene sets showed concordant expressional changes. Five and four gene sets of the chemical and genetic perturbations and gene ontology terms collections, respectively, did not show concordant changes in expression, which implies that the driving force of these biological functions, such as the development of the ectoderm, the activity of chemokines, transcriptional inactivation of genes with promoters bound by the NF-Y transcription factor, genes with promoters bound by FOXP3, and the implantation of the embryo, is less likely to be affected by CNAs.
Discussion
We have demonstrated the capability and robustness of GSCA in a large breast cancer dataset. For the gene sets affected by copy number gains, most of them were originally derived from studies of CNAs (30-32). Most of these genomic regions were previously reported in breast cancer (33-38). Notably, four of the gene sets enriched in copy number losses were located in chromosome 16q. Our findings are consistent with previous reports showing that gain in 1q and/or loss in 16q were observed in epithelial tumors such as hepatocellular, ovarian, nasopharyngeal, prostate, and breast cancers (39,40). Our results confirmed the conclusion of previous studies that cancer genomes share highly similar patterns of CNAs. Moreover, we identified seven target gene sets of transcription factors (LEF1, TEF1, ETF, MAZ, E2F, PEA3, and SRY), all of which are reported to be associated with breast cancer tumorigenesis (41-47). Our results indicate that the expression of abundant genes is associated with both transcription factors and CNAs.
To explore the potentially prognostic roles of CNA-affected gene sets for clinical applications, we conducted survival analysis on the copy number-enriched gene sets. The subtypes of breast cancer were taken into consideration. The gene set “CAMPS COLON CANCER COPY NUMBER UP,” which was derived from a colon cancer study (25), was the most statistically significant gene set in the gain/normal comparison in the ER+ group. The result indicated the CNAs in the genomic regions where genes in the gene sets located could affect tumor malignancy. Further exploration into the underlying mechanism is warranted. Target gene sets of several transcription factors were also identified with correlations to patient survival. All of the transcription factors have been reported to contribute to disease progression of breast cancer. It is noteworthy that the gene set “V$MYC_Q2” was found to be significantly associated with patient survival in both gain/normal and loss/normal comparisons. MYC has been reported to be highly involved in cell growth, proliferation, transformation, angiogenesis, cell-cycle control, and apoptosis, with dysregulation in various cancers, including breast cancer (48-51). Our results suggest that CNAs could dysregulate target genes of MYC and result in improved survival of patients. In summary, our findings shed light on the effects of these transcription factor-related gene sets from the prognosis point of view in breast cancer.
There are some limitations of our proposed method. First, we utilized the ECN to evaluate the copy number status of each gene. However, this scoring method may underestimate the potential effects of short CNAs and have limitations in analyzing subtle genomic changes. However, since our approach focuses on the overall effects of “copy number patterns” on “gene set functions”, missing information of a few genes from a gene set would be unlikely to bias the analysis. Second, our analysis is based on the pre-defined gene sets of MSigDB. Realizing that not all gene sets related to known biological functions have been discovered or defined, the resolution of our method may be limited due to insufficient gene set information. Therefore, future research that identifies gene sets with unified biological themes will be helpful to improve the efficacy of our method. Also, large datasets with sample-paired CNA and expression data are rare. Without another suitable dataset, we were not able to validate the findings in our study.
Conclusions
In this paper, we proposed a systematic method, GSCA, to analyze the involvement of CNAs in biological functions and tumor progression in breast cancer on the basis of gene set enrichment analysis. To evaluate the efficacy of our analysis, a dataset of breast cancer samples from TCGA was analyzed. The results showed that our analysis is capable of exploring the chromosomal distribution of genomic aberrations, as well as the potential mechanisms underlying the pathogenesis of breast cancer.
Acknowledgments
The authors thank Melissa Stauffer, PhD, of Scientific Editing Solutions, for editing the manuscript.
Funding: The study was supported partly by the National Health Research Institutes of Taiwan (NHRI-EX104-10419BI) and the Ministry of Science and Technology of Taiwan (103-2917-I-002-166). The authors thank the Center of Genomic Medicine, National Taiwan University, for providing financial support and computing facilities.
Footnote
Provenance and Peer Review: This article was commissioned by the Guest Editor (Jian-Bing Fan) for the series “Application of Genomic Technologies in Cancer Research” published in Translational Cancer Research. The article has undergone external peer review.
Conflicts of Interest: All authors have completed the ICMJE uniform disclosure form (available at http://dx.doi.org/10.3978/j.issn.2218-676X.2015.05.03). The series “Application of Genomic Technologies in Cancer Research” was commissioned by the editorial office without any funding or sponsorship. EYC serves as the Editor-in-Chief of Translational Cancer Research. YC serves as an unpaid editorial board member of Translational Cancer Research. The authors have no other conflicts of interest to declare.
Ethical Statement: The authors are accountable for all aspects of the work in ensuring that questions related to the accuracy or integrity of any part of the work are appropriately investigated and resolved. The study was conducted in accordance with the Declaration of Helsinki (as revised in 2013). The study used the public microarray data download from GEO database (http://www.ncbi.nlm.nih.gov/geo/). Informed consent isn’t needed and institutional ethical approval was waived.
Open Access Statement: This is an Open Access article distributed in accordance with the Creative Commons Attribution-NonCommercial-NoDerivs 4.0 International License (CC BY-NC-ND 4.0), which permits the non-commercial replication and distribution of the article with the strict proviso that no changes or edits are made and the original work is properly cited (including links to both the formal publication through the relevant DOI and the license). See: https://creativecommons.org/licenses/by-nc-nd/4.0/.
References
- Lengauer C, Kinzler KW, Vogelstein B. Genetic instabilities in human cancers. Nature 1998;396:643-9. [PubMed]
- Albertson DG, Collins C, McCormick F, et al. Chromosome aberrations in solid tumors. Nat Genet 2003;34:369-76. [PubMed]
- Russnes HG, Vollan HK, Lingjaerde OC, et al. Genomic architecture characterizes tumor progression paths and fate in breast cancer patients. Sci Transl Med 2010;2:38ra47 [PubMed]
- Diep CB, Kleivi K, Ribeiro FR, et al. The order of genetic events associated with colorectal cancer progression inferred from meta-analysis of copy number changes. Genes Chromosomes Cancer 2006;45:31-41. [PubMed]
- Massion PP, Kuo WL, Stokoe D, et al. Genomic copy number analysis of non-small cell lung cancer using array comparative genomic hybridization: implications of the phosphatidylinositol 3-kinase pathway. Cancer Res 2002;62:3636-40. [PubMed]
- Tanner MM, Grenman S, Koul A, et al. Frequent amplification of chromosomal region 20q12-q13 in ovarian cancer. Clin Cancer Res 2000;6:1833-9. [PubMed]
- Chin K, DeVries S, Fridlyand J, et al. Genomic and transcriptional aberrations linked to breast cancer pathophysiologies. Cancer Cell 2006;10:529-41. [PubMed]
- Hirsch D, Kemmerling R, Davis S, et al. Chromothripsis and focal copy number alterations determine poor outcome in malignant melanoma. Cancer Res 2013;73:1454-60. [PubMed]
- Sapkota Y, Ghosh S, Lai R, et al. Germline DNA copy number aberrations identified as potential prognostic factors for breast cancer recurrence. PLoS One 2013;8:e53850 [PubMed]
- Jemal A, Bray F, Center MM, et al. Global cancer statistics. CA Cancer J Clin 2011;61:69-90. [PubMed]
- Sorlie T, Tibshirani R, Parker J, et al. Repeated observation of breast tumor subtypes in independent gene expression data sets. Proc Natl Acad Sci U S A 2003;100:8418-23. [PubMed]
- Endesfelder D, Burrell RA, Kanu N, et al. Chromosomal instability selects gene copy-number variants encoding core regulators of proliferation in ER+ breast cancer. Cancer Res 2014;74:4853-63. [PubMed]
- Huang CC, Tu SH, Lien HH, et al. Concurrent gene signatures for han chinese breast cancers. PLoS One 2013;8:e76421 [PubMed]
- Richard F, Pacyna-Gengelbach M, Schlüns K, et al. Patterns of chromosomal imbalances in invasive breast cancer. Int J Cancer 2000;89:305-10. [PubMed]
- Waddell N, Arnold J, Cocciardi S, et al. Subtypes of familial breast tumours revealed by expression and copy number profiling. Breast Cancer Res Treat 2010;123:661-77. [PubMed]
- Beroukhim R, Mermel CH, Porter D, et al. The landscape of somatic copy-number alteration across human cancers. Nature 2010;463:899-905. [PubMed]
- Knuutila S, Aalto Y, Autio K, et al. DNA copy number losses in human neoplasms. Am J Pathol 1999;155:683-94. [PubMed]
- Mitelman F. Recurrent chromosome aberrations in cancer. Mutat Res 2000;462:247-53. [PubMed]
- Hsiao TH, Chen HI, Roessler S, et al. Identification of genomic functional hotspots with copy number alteration in liver cancer. EURASIP J Bioinform Syst Biol 2013;2013:14.
- Huang da W. Sherman BT, Lempicki RA. Bioinformatics enrichment tools: paths toward the comprehensive functional analysis of large gene lists. Nucleic Acids Res 2009;37:1-13. [PubMed]
- Tomlins SA, Mehra R, Rhodes DR, et al. Integrative molecular concept modeling of prostate cancer progression. Nat Genet 2007;39:41-51. [PubMed]
- Subramanian A, Tamayo P, Mootha VK, et al. Gene set enrichment analysis: a knowledge-based approach for interpreting genome-wide expression profiles. Proc Natl Acad Sci U S A 2005;102:15545-50. [PubMed]
- Cancer Genome Atlas Network. Comprehensive molecular portraits of human breast tumours. Nature 2012;490:61-70. [PubMed]
- Patil MA, Chua MS, Pan KH, et al. An integrated data analysis approach to characterize genes highly expressed in hepatocellular carcinoma. Oncogene 2005;24:3737-47. [PubMed]
- Camps J, Grade M, Nguyen QT, et al. Chromosomal breakpoints in primary colon cancer cluster at sites of structural variants in the genome. Cancer Res 2008;68:1284-95. [PubMed]
- Korkola JE, Houldsworth J, Chadalavada RS, et al. Down-regulation of stem cell genes, including those in a 200-kb gene cluster at 12p13.31, is associated with in vivo differentiation of human male germ cell tumors. Cancer Res 2006;66:820-7. [PubMed]
- Aguirre AJ, Brennan C, Bailey G, et al. High-resolution characterization of the pancreatic adenocarcinoma genome. Proc Natl Acad Sci U S A 2004;101:9067-72. [PubMed]
- Ding L, Getz G, Wheeler DA, et al. Somatic mutations affect key pathways in lung adenocarcinoma. Nature 2008;455:1069-75. [PubMed]
- Grasemann C, Gratias S, Stephan H, et al. Gains and overexpression identify DEK and E2F3 as targets of chromosome 6p gains in retinoblastoma. Oncogene 2005;24:6441-9. [PubMed]
- Nikolsky Y, Sviridov E, Yao J, et al. Genome-wide functional synergy between amplified and mutated genes in human breast cancer. Cancer Res 2008;68:9532-40. [PubMed]
- Climent J, Dimitrow P, Fridlyand J, et al. Deletion of chromosome 11q predicts response to anthracycline-based chemotherapy in early breast cancer. Cancer Res 2007;67:818-26. [PubMed]
- Myllykangas S, Himberg J, Böhling T, et al. DNA copy number amplification profiling of human neoplasms. Oncogene 2006;25:7324-32. [PubMed]
- Clark J, Edwards S, John M, et al. Identification of amplified and expressed genes in breast cancer by comparative hybridization onto microarrays of randomly selected cDNA clones. Genes Chromosomes Cancer 2002;34:104-14. [PubMed]
- Ethier SP. Identifying and validating causal genetic alterations in human breast cancer. Breast Cancer Res Treat 2003;78:285-7. [PubMed]
- Greenman C, Stephens P, Smith R, et al. Patterns of somatic mutation in human cancer genomes. Nature 2007;446:153-8. [PubMed]
- Latham C, Zhang A, Nalbanti A, et al. Frequent co-amplification of two different regions on 17q in aneuploid breast carcinomas. Cancer Genet Cytogenet 2001;127:16-23. [PubMed]
- Sinclair CS, Rowley M, Naderi A, et al. The 17q23 amplicon and breast cancer. Breast Cancer Res Treat 2003;78:313-22. [PubMed]
- Yao J, Weremowicz S, Feng B, et al. Combined cDNA array comparative genomic hybridization and serial analysis of gene expression analysis of breast tumor progression. Cancer Res 2006;66:4065-78. [PubMed]
- Baudis M. Genomic imbalances in 5918 malignant epithelial tumors: an explorative meta-analysis of chromosomal CGH data. BMC Cancer 2007;7:226. [PubMed]
- Tsarouha H, Pandis N, Bardi G, et al. Karyotypic evolution in breast carcinomas with i(1)(q10) and der(1;16)(q10;p10) as the primary chromosome abnormality. Cancer Genet Cytogenet 1999;113:156-61. [PubMed]
- Clementz AG, Rogowski A, Pandya K, et al. NOTCH-1 and NOTCH-4 are novel gene targets of PEA3 in breast cancer: novel therapeutic implications. Breast Cancer Res 2011;13:R63. [PubMed]
- Gong T, Xuan J, Chen L, et al. Motif-guided sparse decomposition of gene expression data for regulatory module identification. BMC Bioinformatics 2011;12:82. [PubMed]
- He Q, Liang CH, Lippard SJ. Steroid hormones induce HMG1 overexpression and sensitize breast cancer cells to cisplatin and carboplatin. Proc Natl Acad Sci U S A 2000;97:5768-72. [PubMed]
- Maeda T, Maeda M, Stewart AF. TEF-1 transcription factors regulate activity of the mouse mammary tumor virus LTR. Biochem Biophys Res Commun 2002;296:1279-85. [PubMed]
- Nguyen A, Rosner A, Milovanovic T, et al. Wnt pathway component LEF1 mediates tumor cell invasion and is expressed in human and murine breast cancers lacking ErbB2 (her-2/neu) overexpression. Int J Oncol 2005;27:949-56. [PubMed]
- Nguyen-Vu T, Vedin LL, Liu K, et al. Liver × receptor ligands disrupt breast cancer cell proliferation through an E2F-mediated mechanism. Breast Cancer Res 2013;15:R51. [PubMed]
- Wang X, Southard RC, Allred CD, et al. MAZ drives tumor-specific expression of PPAR gamma 1 in breast cancer cells. Breast Cancer Res Treat 2008;111:103-11. [PubMed]
- Xu J, Chen Y, Olopade OI. MYC and Breast Cancer. Genes Cancer 2010;1:629-40. [PubMed]
- Hynes NE, Stoelzle T. Key signalling nodes in mammary gland development and cancer: Myc. Breast Cancer Res 2009;11:210. [PubMed]
- Chen Y, Olopade OI. MYC in breast tumor progression. Expert Rev Anticancer Ther 2008;8:1689-98. [PubMed]
- Efstratiadis A, Szabolcs M, Klinakis A. Notch, Myc and breast cancer. Cell Cycle 2007;6:418-29. [PubMed]