A bioinformatics analysis to evaluate the prognostic value of stemness-related genes in gastric cancer
Introduction
Gastric cancer (GC) is one of the deadliest tumors worldwide. In China, GC is ranked second among all malignancies for incidence and mortality (1). Although current comprehensive treatment protocols have shown improved outcomes in GC, locally advanced gastric cancer (LAGC) still demonstrates a high recurrence rate and metastatic drug resistance, and its 5-year survival rate is less than 25% (2,3).
In the past decade, researchers have found that in a variety of solid tumors, such as gastric, breast, and lung cancers, a small number of cancer cells exert the characteristics of stem cells; these are known as cancer stem cells (CSCs) (4-7). CSCs are characterized by self-renewal, drug resistance, and differentiation (8). In 2009, Takaishi et al. identified gastric cancer stem cells (GCSCs) by studying CD44+ cell surface markers (4). This small part of GCSCs is closely related to the drug resistance, recurrence, and metastasis of GC (9,10). Traditional chemotherapy or radiotherapy can eliminate ordinary cancer cells, but it cannot completely eliminate CSCs. Thus, this part of stem cells is likely to be the key factor in tumor recurrence, and could provide a promising therapeutic target for clinical treatment.
The present study aimed to establish a prognostic model incorporating stemness-related genes in the hope of facilitating a deeper understanding of GCSCs, which may provide potential treatment targets for GC.
We present the following article in accordance with the MDAR checklist (available at http://dx.doi.org/10.21037/tcr-20-2622).
Methods
Selection of stemness-related genes
A list of stemness-related genes involved in stemness-related signaling pathways was obtained from the Gene Set Enrichment Analysis (GSEA) database (http://software.broadinstitute.org/gsea/downloads.jsp). Using the edgeR package (v3.53) (http://bioconductor.org/packages/edgeR/), we analyzed the GSE112631 data set with stemness-characteristic cell groups and non-stemness cell groups, which allowed us to identify the stemness-related differentially expressed genes (DEGs) (|Log2 fold change [FC]| >1.0 and P<0.05) (11). Through the intersection of two gene lists, we acquired the final stemness-related gene list.
Data collection
Patient clinical information and messenger RNA (mRNA) sequencing data were obtained from The Cancer Genome Atlas (TCGA) and the GSE84437 dataset of the Genome Expression Omnibus (GEO). The TCGA dataset included 375 GC tissues and 32 adjacent cancer tissues, and the GSE84437 dataset comprised 433 GC tissues. Screening to identify suitable genes was performed as follows: (I) The stemness-related genes list was obtained as outlined above; (II) genes that were expressed in both the TCGA GC database and in the GSE84437 dataset were selected.
Identification of stemness-related DEGs in the TCGA database
Using the edgeR limma package, we identified stemness-related DEGs for GC in the TCGA database. DEGs were defined as genes with a |Log2 fold change (FC)| >1.0 and a false discovery rate (FDR) adjusted to P<0.05.
Establishment of a prognostic model and validation model
A prognostic risk score was obtained for all patients by lasso-penalized Cox regression and multivariate Cox regression analysis. The risk score calculation used as follows: . In this formula, n represents the number of genes, Ci represents the coefficient of each gene in multivariate Cox model, and Ei represents the expression level of each gene. Patients were classified into a high-risk score group and a low-risk score group according to the median risk score. To further verify the feasibility of the prognostic model, we also divided the GSE84437 patients into two groups according to the median risk score. The survival of the two groups of patients was analyzed with the Kaplan–Meier (KM) curve.
Construction of the prognostic nomogram and receiver operating characteristic (ROC) curves
A prognostic nomogram was established using the ‘rms’ package in R (12). To further verify the accuracy of the prognostic model, ROC curves were drawn using the ‘ROCR’ package. A bootstrapping method with 1,000 resamples was used to reduce over-fitting.
Functional enrichment analysis
To better understand the underlying biological mechanisms of these genes, Kyoto Encyclopedia of Genes and Genomes (KEGG) pathway analyses were performed (GSEA) (12). KEGG pathway analyses were based on the threshold of P<0.05.
Statistical analysis
Statistical analyses were performed with GraphPad Prism (version 8.0, San Diego, USA). Independent prognostic factors were determined through multivariate Cox regression. Patient survival time was analyzed using the KM curve, and the log-rank test was used for statistical analysis. A P value <0.05 was considered to be statistically significant. The study was conducted in accordance with the Declaration of Helsinki (as revised in 2013). All information from TCGA, GSEA and GEO is available and free for public, so the agreement of the medical ethics committee board was not necessary.
Results
Selected stemness-related DEGs
A total of 3,639 DEGs were identified from the GSE112631 dataset. Of these DEGs, 1,842 were upregulated and 1,797 were downregulated, respectively, with the thresholds of |log2 FC| >1.0 and P<0.05 (Figure S1A).
The stemness-related genes list was obtained from the stemness pathways in the GSEA database (http://software.broadinstitute.org/gsea/downloads.jsp).
Through the intersection of two gene lists, we finally obtained 715 stemness-related genes (Figure S1B).
Identification of stemness-related DEGs in the TCGA GC database
Co-expressed stemness-related genes were obtained by intersecting the TCGA GC database with the GSE84437 dataset. Using edgeR, we identified 127 DEGs among GC patients; of these DEGs, 42 were downregulated and 85 were upregulated, respectively, with the thresholds of |log2 FC| >1.0 and adjusted P<0.05 (Figure 1A,B).
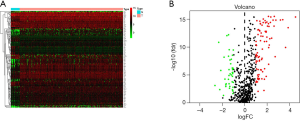
Construction of the stemness-related gene prognostic model
By using univariate Cox regression analysis, we obtained the survival-associated genes shown in Table 1. Lasso-penalized Cox regression and multivariate Cox regression analyses were performed to identify the genes in the prognostic model, and the risk score calculation formula used was as follows: we constructed a prognostic model (Figure 2A,B,C) and used the GSE84437 dataset to build a validation model (Figure 2D,E,F).
Table 1
Gene | Univariate analysis | |
---|---|---|
HR (95% CI) | P value | |
SAMD1 | 0.920 (0.971–0.898) | 0.011 |
DUSP1 | 1.827 (1.507–2.247) | <0.001 |
TXNIP | 1.609 (1.351–1.896) | 0.010 |
PIM1 | 1.714 (1.504–1.924) | 0.008 |
VCAN | 1.740 (1.439–1.996) | 0.005 |
ADAM8 | 1.816 (1.601–2.132) | 0.008 |
ERCC6L | 0.860 (0.781–0.992) | 0.018 |
DNASE1L3 | 1.805 (1.530–2.010) | 0.007 |
COL4A5 | 1.730 (1.532–1.959) | 0.038 |
HR, hazard ratio; CI, confidence interval.
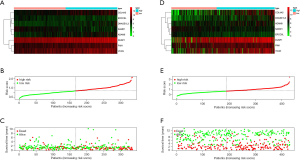
(Expression level of SAMD1×-0.00170) + (Expression level of DUSP1×-0.00005) + (Expression level of PIM1× 0.00046) + (Expression level of VCAN×0.00024) + (Expression level of ADAM8×0.00141) + (Expression level of ERCC6L×-0.00104) + (Expression level of DNASE1L3× 0.00206) + (Expression level of COL4A5×0.00078)
Patients were classified into low- and high-risk score groups with the median-risk score used as the cut-off, and the survival of the groups was analyzed by the KM curve. The low-risk score group had a better overall survival (OS) than the high-risk score group (P<0.001; Figure 3A). In the validation model, the OS in the low-risk score group was longer than that in the high-risk score group (P=0.047; Figure 3B).
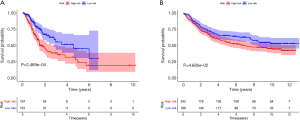
The clinical outcome of the prognostic model
In the TCGA prognostic model, univariate Cox regression analyses showed that age [hazard ratio (HR) =1.022; 95% confidence interval (CI), 1.003–1.042; P=0.024), high American Joint Committee on Cancer (AJCC) stage (HR =1.478; 95% CI, 1.172–1.863; P<0.001), high T stage (HR =1.289; 95% CI, 1.013–1.641; P=0.039), high N stage (HR =1.252; 95% CI, 1.053–1.490; P=0.011), and high-risk score (HR =2.766; 95% CI, 1.806–4.236; P<0.001) were significant risk factors for poor prognosis in GC patients. In the multivariate Cox regression analysis, age (HR =1.036; 95% CI, 1.015–1.058; P<0.001) and high-risk score (HR =2.941; 95% CI, 1.845–4.603; P<0.001) were found to be independently associated with worse OS (Table 2). The risk scores were significantly higher for patients with grade (Figure 4).
Table 2
Variables | Univariate analysis | Multivariate analysis | |||
---|---|---|---|---|---|
HR (95% CI) | P value | HR (95% CI) | P value | ||
Age | 1.022 (1.003–1.042) | 0.024 | 1.036 (1.015–1.058) | <0.001 | |
Gender | 1.473 (0.966–2.247) | 0.072 | 1.410 (0.912–2.180) | 0.123 | |
Grade | 1.357 (0.934–1.972) | 0.110 | 1.334 (0.906–1.965) | 0.144 | |
Stage | 1.478 (1.172–1.863) | <0.001 | 1.274 (0.817–1.987) | 0.285 | |
T | 1.289 (1.013–1.641) | 0.039 | 1.118 (0.799–1.565) | 0.516 | |
N | 1.728 (0.871–3.429) | 0.118 | 1.683 (0.705–4.016) | 0.241 | |
M | 1.252 (1.053–1.490) | 0.011 | 1.077 (0.834–1.390) | 0.571 | |
Risk score | 2.766 (1.806–4.236) | <0.001 | 2.914 (1.845–4.603) | <0.001 |
HR, hazard ratio; CI, confidence interval.
In the GSE84437 validation model, univariate Cox regression analyses revealed age (HR =1.019; 95% CI, 1.006–1.032; P=0.003), high T stage (HR =1.729; 95% CI, 1.369–2.184; P<0.001), high N stage (HR =1.269; 95% CI, 1.012–1.659; P=0.036), and high-risk score (HR =1.669; 95% CI, 1.421–1.959; P=0.040) to be significant risk factors for poor prognosis in GC patients. In the multivariate Cox regression analysis, age (HR =1.024; 95% CI, 1.012–1.037; P<0.001), high T stage (HR =1.598; 95% CI, 1.252–2.038; P<0.001), high N stage (HR =1.373; 95% CI, 1.055–1.787; P=0.025), and high risk score (HR =1.525; 95% CI, 1.296–1.794; P=0.018) were found to be independently associated with worse OS (Table 3).
Table 3
Variables | Univariate analysis | Multivariate analysis | |||
---|---|---|---|---|---|
HR (95% CI) | P value | HR (95% CI) | P value | ||
Age | 1.019 (1.006–1.032) | 0.003 | 1.024 (1.012–1.037) | <0.001 | |
Gender | 1.239 (0.915–1.679) | 0.166 | 1.174 (0.865–1.594) | 0.304 | |
T | 1.729 (1.369–2.184) | <0.001 | 1.598 (1.252–2.038) | <0.001 | |
N | 1.296 (1.012–1.659) | 0.036 | 1.373 (1.055–1.787) | 0.025 | |
Risk score | 1.669 (1.421–1.959) | 0.040 | 1.525 (1.296–1.794) | 0.018 |
HR, hazard ratio; CI, confidence interval.
Verification of the accuracy of the prognostic model
In order to visualize the predictive model, a nomogram was established based on the results of the Cox regression analyses (Figure 5A). The ROC curve analysis of the TCGA prognostic model is shown in Figure 5B. The area under the curve (AUC) was 0.700, which was higher than those of other prognostic variables.
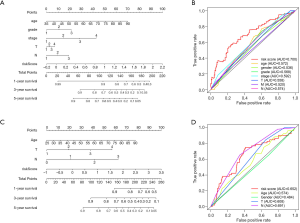
The nomogram based on the GSE84437 database is shown in Figure 5C, and the related AUC was 0.652 (Figure 5D).
The functional enrichment analysis of stemness related genes
Through GESA enrichment analysis, we found that the high-risk score group was enriched in the following KEGG pathway (Figure 6): hedgehog signaling pathway, TGF-β signaling pathway, cytokine-cytokine receptor interaction pathway, ECM-receptor interaction pathway and JAK-STAT signaling pathway. The low-risk score group was enriched in the following pathways (Figure 6): Huntington’s disease pathway, pyrimidine metabolism pathway, oxidative phosphorylation pathway, spliceosome pathway and proteasome pathway.
Discussion
In the present study, we constructed a prognostic model on the basis of eight stemness-related genes. Patients with low-risk scores were found to have better OS than those with high-risk scores. Furthermore, we verified the feasibility of the prognostic model using the GSE84437 dataset obtained from the GEO database.
In GC, GCSCs are a subpopulation of cancer cells with stemness characteristics. They can be identified using cell surface markers such as CD44, CD24, and CD133. Zhang et al. found that CD44+CD24+ GC cells have stemness characteristics, including self-renewal, differentiation, and tumorigenesis (13).
Previous studies have shown that certain genes and proteins are important for maintaining the characteristics of GCSCs. CD44 and Oct-4 can maintain the tumorigenesis, metastasis, and drug resistance of GCSCs (14). Tian et al. indicated that Sox2 can improve the colony formation of GCSCs and induce resistance to docetaxel (15). In our study, eight stemness-related genes were screened out to build our prognostic model. Some of these genes, such as SAMD1, DUSP1, PIM1, and VCAN, are known to be associated with various types of CSCs, and some are associated with other stem cells. Zhang et al. found that Uev1A-mediated SAMD1 ubiquitination induced osteosarcoma CSC differentiation and drug resistance (16). Boulding et al. pointed out that DUSP1 could promote breast cancer epithelial–mesenchymal transition (EMT) and maintain breast cancer stem cells (BCSCs) (17). Additionally, Mills et al. found that DUSP1 plays an important role in maintaining glioma stem cells (GSCs) (18). PIM1, a member of the PIM family, has crucial involvement in the maintenance of bladder CSCs and other stem cells (19). The expression of VCAN in bladder cancer CD24+CD44+ stem cells was found to be 46 times higher than that of CD24-CD44- cells. Among the genes mentioned above, DUSP1 (20,21) and PIM1 (22) are oncogenes in GC, and VCAN (23) and ADAM8 (24) are related to the clinical features of GC prognosis. These genes may be used as targets for the treatment of GCSCs. However, except for DUSP1, which is related to the drug resistance of GC, the mechanisms of these genes have rarely been studied.
In GC, researchers have found that the Wnt/β-catenin, sonic hedgehog (SHH), TGF-β, Notch, and other signaling pathways could help GCSCs to survive and self-renew (25,26). Through GSEA analysis, we found that most of the genes involved in the high-risk score patients are enriched in stemness-related pathways, such as the hedgehog, TGF-β, and JAK-STAT signaling pathways. In the low-risk score group, genes were enriched in the non-stemness pathways. A previous study has indicated that the expression of SHH and glioma-associated oncogene homolog 1 (GLI1) are increased in CD44+/Musashi-1+ GCSCs, and SHH contributes to the drug resistance of GCSCs (27). Xu et al. indicated that therapies targeting stem cells can achieve better results in high risk score patients via the BMX-ARHGAP JAK/STAT3 pathway.
An important reason for the recurrence and metastasis of GC is that radiotherapy and chemotherapy cannot completely eliminate these stem cells. In recent years, drugs targeting stem cells have been developed in clinical practice (28); however, the therapeutic effects have been unsatisfactory. Therefore, the development of more effective treatments targeting GCSCs is critical to improving the survival of patients with GC.
However, there were certain limitations in our research. Among the eight genes in our model, the mechanism of some genes in relation to GC and GCSCs is unknown, and some of these genes are mainly involved in normal stem cells. These genes need future research to elaborate their specific mechanisms. Moreover, it is possible that due to the different methods of gene expression measurement in different databases, the indicator values obtained from the GSE84437 dataset were lower than those from TCGA, which led to differences in median risk scores in different cohorts. Also, when applying our predictive model in a clinical setting, clinicians would have to use the same method of genetic measurement as that used in the TCGA database, which is another limitation of our model. Confirming the results of this study in a more suitable validation cohort will be the aim of our future investigations.
Conclusions
In summary, we analyzed the prognostic value of stemness-related genes in GC using the TCGA and GEO databases. Our study may provide potential targets for GCSCs, in order to eliminate GCSCs and improve the treatment sensitivity and outcomes for patients with GC.
Acknowledgments
Funding: This work was supported by
Footnote
Reporting Checklist: The authors have completed the MDAR checklist. Available at http://dx.doi.org/10.21037/tcr-20-2622
Conflicts of Interest: All authors have completed the ICMJE uniform disclosure form (available at http://dx.doi.org/10.21037/tcr-20-2622). The authors have no conflicts of interest to declare.
Ethical Statement: The authors are accountable for all aspects of the work in ensuring that questions related to the accuracy or integrity of any part of the work are appropriately investigated and resolved. The study was conducted in accordance with the Declaration of Helsinki (as revised in 2013). All information from TCGA, GSEA and GEO is available and free for public, so the agreement of the medical ethics committee board was not necessary.
Open Access Statement: This is an Open Access article distributed in accordance with the Creative Commons Attribution-NonCommercial-NoDerivs 4.0 International License (CC BY-NC-ND 4.0), which permits the non-commercial replication and distribution of the article with the strict proviso that no changes or edits are made and the original work is properly cited (including links to both the formal publication through the relevant DOI and the license). See: https://creativecommons.org/licenses/by-nc-nd/4.0/.
References
- Chen W, Zheng R, Baade PD, et al. Cancer statistics in China, 2015. CA Cancer J Clin 2016;66:115-32. [Crossref] [PubMed]
- Takahashi T, Saikawa Y, Kitagawa Y. Gastric cancer: current status of diagnosis and treatment. Cancers (Basel) 2013;5:48-63. [Crossref] [PubMed]
- Nachshen DS. The Symptoms of High Blood Pressure. J Coll Gen Pract Res Newsl 1959;2:264-7. [PubMed]
- Takaishi S, Okumura T, Tu S, et al. Identification of gastric cancer stem cells using the cell surface marker CD44. Stem Cells 2009;27:1006-20. [Crossref] [PubMed]
- Fan CW, Chen T, Shang YN, et al. Cancer-initiating cells derived from human rectal adenocarcinoma tissues carry mesenchymal phenotypes and resist drug therapies. Cell Death Dis 2013;4:e828. [Crossref] [PubMed]
- Singh SK, Clarke ID, Terasaki M, et al. Identification of a cancer stem cell in human brain tumors. Cancer Res 2003;63:5821-8. [PubMed]
- Al-Hajj M, Wicha MS, Benito-Hernandez A, et al. Prospective identification of tumorigenic breast cancer cells. Proc Natl Acad Sci U S A 2003;100:3983-8. [Crossref] [PubMed]
- Bandhavkar S. Cancer stem cells: a metastasizing menace! Cancer Med 2016;5:649-55. [Crossref] [PubMed]
- Takaishi S, Okumura T, Wang TC. Gastric cancer stem cells. J Clin Oncol 2008;26:2876-82. [Crossref] [PubMed]
- Li K, Dan Z, Nie YQ. Gastric cancer stem cells in gastric carcinogenesis, progression, prevention and treatment. World J Gastroenterol 2014;20:5420-6. [Crossref] [PubMed]
- Robinson MD, McCarthy DJ, Smyth GK. edgeR: a Bioconductor package for differential expression analysis of digital gene expression data. Bioinformatics 2010;26:139-40. [Crossref] [PubMed]
- Subramanian A, Tamayo P, Mootha VK, et al. Gene set enrichment analysis: a knowledge-based approach for interpreting genome-wide expression profiles. Proc Natl Acad Sci U S A 2005;102:15545-50. [Crossref] [PubMed]
- Zhang C, Li C, He F, et al. Identification of CD44+CD24+ gastric cancer stem cells. J Cancer Res Clin Oncol 2011;137:1679-86. [Crossref] [PubMed]
- Zhang X, Hua R, Wang X, et al. Identification of stem-like cells and clinical significance of candidate stem cell markers in gastric cancer. Oncotarget 2016;7:9815-31. [Crossref] [PubMed]
- Tian T, Zhang Y, Wang S, et al. Sox2 enhances the tumorigenicity and chemoresistance of cancer stem-like cells derived from gastric cancer. J Biomed Res 2012;26:336-45. [Crossref] [PubMed]
- Zhang W, Zhuang Y, Zhang Y, et al. Uev1A facilitates osteosarcoma differentiation by promoting Smurf1-mediated Smad1 ubiquitination and degradation. Cell Death Dis 2017;8:e2974. [Crossref] [PubMed]
- Boulding T, Wu F, McCuaig R, et al. Differential Roles for DUSP Family Members in Epithelial-to-Mesenchymal Transition and Cancer Stem Cell Regulation in Breast Cancer. PLoS One 2016;11:e0148065. [Crossref] [PubMed]
- Mills BN, Albert GP, Halterman MW. Expression Profiling of the MAP Kinase Phosphatase Family Reveals a Role for DUSP1 in the Glioblastoma Stem Cell Niche. Cancer Microenviron 2017;10:57-68. [Crossref] [PubMed]
- Xie Y, Bayakhmetov S. PIM1 kinase as a promise of targeted therapy in prostate cancer stem cells. Mol Clin Oncol 2016;4:13-7. [Crossref] [PubMed]
- Teng F, Xu Z, Chen J, et al. DUSP1 induces apatinib resistance by activating the MAPK pathway in gastric cancer. Oncol Rep 2018;40:1203-22. [Crossref] [PubMed]
- Wang Z, Zou F, Tian Y, et al. Paclitaxel reversed trastuzumab resistance via regulating JUN in human gastric cancer cells identified by FAN analysis. Future Oncol 2018;14:2701-12. [Crossref] [PubMed]
- Yan B, Yau EX, Samanta S, et al. Clinical and therapeutic relevance of PIM1 kinase in gastric cancer. Gastric Cancer 2012;15:188-97. [Crossref] [PubMed]
- Jiang K, Liu H, Xie D, et al. Differentially expressed genes ASPN, COL1A1, FN1, VCAN and MUC5AC are potential prognostic biomarkers for gastric cancer. Oncol Lett 2019;17:3191-202. [Crossref] [PubMed]
- Huang J, Bai Y, Huo L, et al. Upregulation of a disintegrin and metalloprotease 8 is associated with progression and prognosis of patients with gastric cancer. Transl Res 2015;166:602-13. [Crossref] [PubMed]
- Yong X, Tang B, Xiao YF, et al. Helicobacter pylori upregulates Nanog and Oct4 via Wnt/beta-catenin signaling pathway to promote cancer stem cell-like properties in human gastric cancer. Cancer Lett 2016;374:292-303. [Crossref] [PubMed]
- Song X, Xin N, Wang W, et al. Wnt/beta-catenin, an oncogenic pathway targeted by H. pylori in gastric carcinogenesis. Oncotarget 2015;6:35579-88. [Crossref] [PubMed]
- Xu M, Gong A, Yang H, et al. Sonic hedgehog-glioma associated oncogene homolog 1 signaling enhances drug resistance in CD44(+)/Musashi-1(+) gastric cancer stem cells. Cancer Lett 2015;369:124-33. [Crossref] [PubMed]
- Wen Z, Feng S, Wei L, et al. Evodiamine, a novel inhibitor of the Wnt pathway, inhibits the self-renewal of gastric cancer stem cells. Int J Mol Med 2015;36:1657-63. [Crossref] [PubMed]