Identification of hub genes in key hallmarks of ovarian cancer via bioinformatics analysis
Introduction
Ovarian cancer is one of the most lethal malignant gynecologic tumors worldwide (1). Nearly all patients suffering from ovarian cancer inevitably undergfo multistep development, such as occurrence, progression, regression, recurrence and chemotherapy resistance. Like biological evolution, there must be a series of key steps involved in the occurrence and evolution of neoplastic diseases, such as sustaining proliferative signaling, resisting cell death, enabling replicative immortality, inducing angiogenesis, and activating invasion and metastasis (2). However, not all tumor cells in every ovarian cancer patient go through all the processes mentioned above. It would be significant to explore these essential steps as normal cells evolve progressively into a neoplastic state.
An increasing number of bioinformatics methods have been exploited to identify prognostic biomarkers in neoplastic diseases; however, the overall survival (OS) rate of patients with ovarian cancer remains poor. Inspired by the theory of biological evolution, we aimed to first identify critical hallmarks that could be found by comparing expression profiles of the surface epithelium between normal ovaries and serous ovarian carcinomas and then obtain the hub genes involved in these critical hallmarks.
In this study, we chose GSE36668, GSE54388 and GSE69428 as data sources from the Gene Expression Omnibus (GEO). Global gene expression was analyzed in the above datasets with the limma package in R statistical software (version 3.6.1). Gene set enrichment analysis (GSEA) was performed using clusterProfiler package in R to determine the significantly enriched hallmarks (3). The differentially expressed genes (DEGs) (P<0.05, log2 fold change >1) in each hallmark were screened separately. These DEGs were analyzed again through the Metascape website. These DEGs were imported into Kaplan-Meier plotter to obtain significant prognostic information (P<0.05). Finally, we obtained the hub genes through a protein-protein interaction (PPI) network by Molecular Complex Detection (MCODE) app in Cytoscape. Public databases [Gene Expression Profiling Interactive Analysis (GEPIA) and The Human Protein Atlas] were used to further define the differential expression of hub genes between ovarian cancer tissues and normal tissues at the mRNA and protein levels. Fourteen pairs of hub genes were selected for correlation analysis through R, and all of them showed a strong correlation. Not only the hallmarks enriched by GSEA but also the hub genes extracted by the PPI network indicate that the most fundamental trait of ovarian cancer cells involves their ability to sustain chronic proliferation.
We present the following article in accordance with the MDAR checklist (available at http://dx.doi.org/10.21037/tcr-20-2604).
Methods
Ethical statement
The study was conducted in accordance with the Declaration of Helsinki (as revised in 2013). The present study was reviewed and approved by the Institutional Review Board and the Research Ethics Committee of Shanghai General Hospital.
Datasets
The GEO database is a free public database of gene profiles, and the gene expression profiles of GSE36668, GSE54388 and GSE69428, which include a total of 20 normal ovarian tissues and 30 ovarian cancer tissues, were downloaded. Microarray data of GSE36668, GSE54388 and GSE69428 were based on the GPL570 Platform [(HG-U133_Plus_2) Affymetrix Human Genome U133 Plus 2.0 Array]. We excluded four serous ovarian borderline tumors in GSE36668 and only selected samples of normal ovaries and serous ovarian carcinomas in GSE69428.
GSEA
The raw data were subjected to significance analysis with the limma package in R statistical software (version 3.6.1). Global gene expression was analyzed by the limma package in R and is displayed as volcano maps. Next, GSEA, a computational method that determines whether an a priori defined set of genes shows statistically significant concordant differences between two biological states, was carried out to determine the significantly enriched pathways with clusterProfiler package in R. The hallmark gene sets “hallmark gene sets, gene symbols” were downloaded from the GSEA website (http://software.broadinstitute.org/gsea/index.jsp). The significant hallmarks shown in the Venn diagram (generated with the VennDiagram package in R) indicate that these hallmarks were found in all three datasets.
Identification of DEGs
The core significantly enriched genes or genes in the leading edge in each gene set were extracted by their Entrez Gene ID and then translated into their symbol name with the clusterProfiler package in R. Genes with P<0.05 and log2 fold change >1 were regarded as differentially expressed. However, only the genes that were expressed differently in all three datasets were identified as DEGs. The process was completed with the limma package in R.
Metascape gene list analysis
Metascape is a portal designed to provide a comprehensive gene list annotation and analysis resource (http://metascape.org). In terms of design features, Metascape combines functional enrichment, interactome analysis, gene annotation, and a membership search to leverage over 40 independent knowledge bases within one integrated portal (4). The DEGs identified in different gene sets were submitted to Metascape in the multiple gene list pattern. Heatmap summary, overlap between enrichment pathway, pathway and process enrichment analysis and PPI enrichment analysis were performed simultaneously. For each given gene list, pathway and process enrichment analyses were carried out with the following ontology sources: Kyoto Encyclopedia of Genes and Genomes (KEGG) Functional Sets, KEGG Pathway, Gene Ontology (GO) Molecular Functions, GO Cellular Components, GO Biological Processes, Immunologic Signatures, KEGG Structural Complexes, Oncogenic Signatures, Reactome Gene Sets, Canonical Pathways, Chemical and Genetic Perturbations and CORUM. All genes in the genome were used as the enrichment background. Terms with a P value <0.01, a minimum count of 3, and an enrichment factor >1.5 (the enrichment factor is the ratio between the observed counts and the counts expected by chance) were collected as representative terms and grouped into clusters based on their membership similarities. To capture the relationships between the terms, a subset of enriched terms was selected and rendered as a network plot, where terms with a similarity >0.3 are connected by edges. The terms with the best P values from each of the 20 clusters were selected, with the constraints that there were no more than 15 terms per cluster and no more than 250 terms in total. The network was visualized with Cytoscape.
Survival analysis
Kaplan-Meier plotter (http://kmplot.com/analysis), a commonly used website tool, was used to assess the effect of DEGs on the survival of ovarian cancer patients based on the GEO, European Genome-phenome Archive (EGA), and The Cancer Genome Atlas (TCGA) databases (5). The log-rank P value and hazard ratio (HR) with the 95% confidence interval are shown on the plot. The DEGs that showed a significant association with poor survival were chosen for further analysis.
PPI network construction and module analysis
The PPI network was predicted using the Search Tool for the Retrieval of Interacting Genes (STRING; http://string-db.org) online database (6). In the present study, a PPI network of DEGs was constructed using the STRING database, and an interaction with a combined score greater than 0.4 was considered statistically significant. Cytoscape (version 3.7.2) is an open-source bioinformatics software platform that can be used to visualize gene interaction networks. The plug-in MCODE (version 1.5.1) of Cytoscape is an app for clustering a given network based on topology to find densely connected regions. The MCODE app in Cytoscape was used to check modules of the PPI network. The criteria for selection were as follows: degree cutoff =2, max. depth =100, k-core =2, and node score cutoff =0.2. The plug-in CentiScaPe 2.2 of Cytoscape was used to differentiate the size of the nodes by degree.
RNA sequencing expression of hub genes and immunohistochemistry (IHC)
GEPIA (http://gepia.cancer-pku.cn/) is an online tool based on data from the TCGA and Genotype Tissue Expression databases (7). In this study, we applied the GEPIA website to analyze the RNA sequencing expression of hub genes. The Human Protein Atlas (www.proteinatlas.org), which collects data on approximately 20 types of cancer, provides a map showing the distribution and relative abundance of proteins in the human body (8). All of the primary Human Pathology Atlas data are available without restrictions. We detected the protein IHC of hub genes in ovarian cancer tissues and normal ovarian tissues.
Correlations between hub genes
We used the cor.test and ggplot2 packages of R to analyze the correlations of the most significant hub genes. “TOIL RSEM tpm (n=10,535) UCSC Toil RNA-seq Recompute” was downloaded from the website (https://xena.ucsc.edu). Gene expression data of ovarian cancer were subjected to correlation analysis.
Statistical analysis
We used limma package for assessment of genes differential expression. The linear model and differential expression functions such as estimating the fold changes and standard errors in this R package apply to all gene expression technologies, including microarrays, RNA-seq and quantitative PCR. Empirical Bayesian methods are used to provide stable results. The method Pearson correlation was used to test for association between two genes. P<0.05 was considered to indicate a statistically significant difference.
Results
GSEA
Twenty normal ovarian tissues and 30 ovarian cancer samples were analyzed in the present study. Global gene expression is shown as volcano maps (Figure 1A,B,C). Via R statistical software (version 3.6.1), we extracted 20, 21 and 29 gene sets from GSE36668, GSE54388 and GSE69428, respectively. Then, we used the Venn diagram package in R to identify 11 significant gene sets among the three datasets (Figure 1D). The adjusted P values and ranks of these hallmarks in the three datasets are shown in Figure 1E. A brief description of the 11 hallmarks from GSEA is shown in Table 1. The actual results of GSEA are shown in Figure S1. We used a bubble chart to show the hallmarks enriched in the three datasets. In Figure S1, if the hallmark has a positive correlation with ovarian cancer, the color of the curve in GSEA is red; otherwise, the curve is green. In E2F_TARGETS, G2M_CHECKPOINT, GLYCOLYSIS, MYC_TARGETS_V1, MYC_TARGETS_V2, UV_RESPONSE_DN, MITOTIC_SPINDLE and MTORC1_SIGNALING, the color of the curve is the same among the three datasets, indicating that the gene sets mentioned above were consistent in all patients with ovarian cancer. However, ESTROGEN_RESPONSE_EARLY, EPITHELIAL_MESENCHYMAL_TRANSITION and RAS_SIGNALING_UP presented different correlations with ovarian cancer among the three datasets.
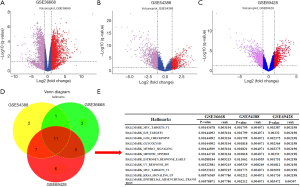
Table 1
Hallmark | Process category | Brief description |
---|---|---|
HALLMARK_E2F_TARGETS | Proliferation | Genes encoding cell cycle related targets of E2F transcription factors |
HALLMARK_EPITHELIAL_MESENCHYMAL_TRANSITION | Development | Genes defining epithelial-mesenchymal transition, as in wound healing, fibrosis and metastasis |
HALLMARK_ESTROGEN_RESPONSE_EARLY | Signaling | Genes defining early response to estrogen |
HALLMARK_G2M_CHECKPOINT | Proliferation | Genes involved in the G2/M checkpoint, as in progression through the cell division cycle |
HALLMARK_GLYCOLYSIS | Metabolic | Genes encoding proteins involved in glycolysis and gluconeogenesis |
HALLMARK_KRAS_SIGNALING_UP | Signaling | Genes up-regulated by KRAS activation |
HALLMARK_MITOTIC_SPINDLE | Proliferation | Genes important for mitotic spindle assembly |
HALLMARK_MTORC1_SIGNALING | Signaling | Genes up-regulated through activation of mTORC1 complex |
HALLMARK_MYC_TARGETS_V1 | Proliferation | A subgroup of genes regulated by MYC—version 1 (v1) |
HALLMARK_MYC_TARGETS_V2 | Proliferation | A subgroup of genes regulated by MYC—version 2 (v2) |
HALLMARK_UV_RESPONSE_DN | DNA damage | Genes down-regulated in response to ultraviolet (UV) radiation |
GSEA, gene set enrichment analysis.
DEGs
DEG (P<0.05, log2 fold change >1) were identified via the limma package of R. In this study, the DEGs identified were not only present in all three datasets but were also enriched in the hallmark leading-edge subset. Though 11 significant hallmarks were identified, only 100 significant DEGs (61 unique DEGs) were identified in 7 hallmarks; these are shown separately in Table 2.
Table 2
Hallmark | Genes | DEGs with significant survival difference |
---|---|---|
E2F_TARGETS | BUB1B, CDC20, CDK1, CDKN3, CENPE, CKS1B, CTPS1, DLGAP5, EZH2, GINS1, HMGB3, HMMR, KIF18B, KIF4A, LMNB1, MCM2, MCM3, MCM7, MELK, MKI67, NCAPD2, NME1, PTTG1, RACGAP1, RAD51AP1, RNASEH2A, RRM2, SNRPB, SPAG5, SPC25, TACC3, TK1 | BUB1B, CDKN3, CENPE, CKS1B, HMMR, KIF18B, MCM2, MKI67, NCAPD2, RACGAP1, RAD51AP1, RNASEH2A, SPC25 |
G2M_CHECKPOINT | CDC20, CDC45, CDC7, CDK1, CDKN3, CENPE, CKS1B, E2F3, EZH2, HMGB3, HMMR, KIF11, KIF15, KIF4A, LMNB1, MCM2, MCM3, MKI67, NDC80, NUSAP1, PBK, PRC1, PTTG1, RACGAP1, STIL, TACC3, TPX2, TTK, UBE2C | CDKN3, CENPE, CKS1B, E2F3, HMMR, KIF11, KIF15, MCM2, MKI67, NDC80, PBK, RACGAP1, STIL, TPX2, UBE2C |
GLYCOLYSIS | CDK1, CLDN3, DSC2, HMMR, KIF20A, TSTA3 | CLDN3, DSC2, HMMR, KIF20A |
MITOTIC_SPINDLE | CDK1, CENPE, DLGAP5, KIF11, KIF15, KIF4A, LMNB1, NDC80, NUSAP1, PRC1, RACGAP1, SAC3D1, TPX2, TTK | CENPE, KIF11, KIF15, LMNB1, NDC80, RACGAP1, TPX2 |
MTORC1_SIGNALING | DHCR24, ELOVL6, MCM2, PIK3R3, RRM2, SLC2A1 | ELOVL6, MCM2 |
MYC_TARGETS_V1 | CCT3, CDC20, CDC45, CTPS1, CYC1, MCM2, MCM7, NME1, RFC4 | MCM2, RFC4 |
UV_RESPONSE_DN | AKT3, CELF2, SYNE1, TFPI | AKT3, TFPI |
DEGs, differentially expressed genes.
Metascape gene list analysis
All 100 DEGs (61 unique DEGs) in the gene sets were analyzed with Metascape in a multiple gene list pattern. The top 20 enriched clusters are shown as a heatmap (Figure 2A). The top 10 clusters with their representative enriched terms are shown in Figure 2B. The overlapping DEGs of the 7 hallmark gene lists are shown as a circos (9) plot (Figure 2C). Overlapping genes based on their functions or shared pathways was another useful representation. The overlaps can be significantly improved by considering overlaps between genes sharing the same enriched ontology terms (Figure 2D). For each given gene list of the 7 significant hallmarks, pathway and process enrichment analyses were carried out with the ontology sources mentioned above. The gene lists of the 7 significant hallmarks were provided, so the nodes were presented as pie charts. The size of the pie is proportional to the total number of hits that falls into that specific term. The pie charts are color coded based on the gene list identities, and the size of het slice represents the percentage of genes under the term that originated from the corresponding gene list. This plot is particularly useful for visualizing whether the terms are shared by multiple pathways, as well as for understanding how these terms are associated with each other within the biological context of the meta-study (Figure 2E). For the given enriched gene sets, PPI enrichment analysis was carried out with the following databases: BioGrid, InWeb_IM, and OmniPath. The resulting network (Figure 2F) contained the subset of proteins that physically interacted with at least one other member in the list. The MCODE algorithm 11 was applied to identify densely connected network components. The MCODE networks were gathered and are shown in Figure 2G.
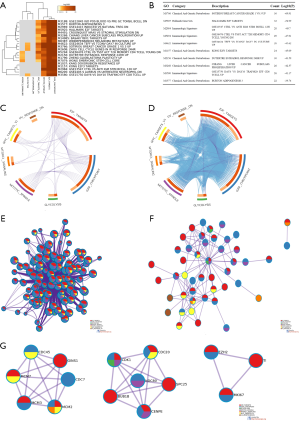
Survival analysis
We utilized Kaplan-Meier plotter (http://kmplot.com/analysis) to analyze the associations of 61 DEGs with patient survival. Twenty-nine genes showed a significant association with poor survival, while 23 showed no significant association. Survival data on the OS rate of patients with ovarian cancer are shown in Figure S2 (P<0.05). Figure S3 shows the progression-free survival (PFS) rate of patients with ovarian cancer (P<0.05). DEGs in the 7 hallmarks with significant survival differences are shown in Table 2.
PPI network construction and module analysis
We uploaded 29 DEGs to STRING; however, “ELOVL6”, “CLDN3” and “TFPI” had no connection with the other DEGs. The remaining 26 DEGs were imported into Cytoscape to build the DEG PPI network complex, which included 26 nodes and 226 edges. The PPI network of the DEGs with significant survival differences was constructed (Figure 3A), and the most significant module was obtained using Cytoscape (Figure 3B). Ultimately, we obtained 22 hub genes whose names and functions are shown in Table 3.
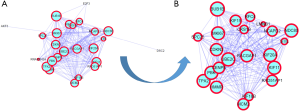
Table 3
ID | Gene name | Function |
---|---|---|
BUB1B | BUB1 mitotic checkpoint serine/threonine kinase B | Essential component of the mitotic checkpoint. Required for normal mitosis progression. The mitotic checkpoint delays anaphase until all chromosomes are properly attached to the mitotic spindle |
CKS1B | CDC28 protein kinase regulatory subunit 1B | Binds to the catalytic subunit of the cyclin dependent kinases and is essential for their biological function |
NDC80 | NDC80, kinetochore complex component | Acts as a component of the essential kinetochore-associated NDC80 complex, which is required for chromosome segregation and spindle checkpoint activity |
PBK | PDZ binding kinase | Phosphorylates MAP kinase p38. Seems to be active only in mitosis. May also play a role in the activation of lymphoid cells |
RAD51AP1 | RAD51 associated protein 1 | May participate in a common DNA damage response pathway associated with the activation of homologous recombination and double-strand break repair. Binds to single and double stranded DNA, and is capable of aggregating DNA. Also binds RNA |
RACGAP1 | Rac GTPase activating protein 1 | Essential for the early stages of embryogenesis and may play a role in the microtubule-dependent steps in cytokinesis. Plays key roles in controlling cell growth and differentiation of hematopoietic cells through mechanisms other than regulating Rac GTPase activity |
STIL | SCL/TAL1 interrupting locus | Plays an important role in embryonic development as well as in cellular growth and proliferation |
SPC25 | Spindle component 25 | Acts as a component of the essential kinetochore-associated NDC80 complex, which is required for chromosome segregation and spindle checkpoint activity |
TPX2 | Targeting protein for xenopus kinesin-like protein 2 | Exclusively expressed in proliferating cells from the transition G1/S until the end of cytokinesis |
CENPE | centromere protein E | Essential for the maintenance of chromosomal stability through efficient stabilization of microtubule capture at kinetochores |
CDKN3 | Cyclin dependent kinase inhibitor 3 | May play a role in cell cycle regulation. Dual specificity phosphatase active toward substrates containing either phosphotyrosine or phosphoserine residues |
HMMR | Hyaluronan mediated motility receptor | Involved in cell motility. When hyaluronan binds to HMMR, the phosphorylation of a number of proteins, including the focal adhesion kinase occurs |
KIF11 | Kinesin family member 11 | Motor protein required for establishing a bipolar spindle |
KIF15 | Kinesin family member 15 | Plus-end directed kinesin-like motor enzyme involved in mitotic spindle assembly |
KIF18B | Kinesin family member 18B | Belongs to the kinesin-like protein family |
KIF20A | Kinesin family member 20A | Interacts with guanosine triphosphate (GTP)-bound forms of RAB6A and RAB6B |
LMNB1 | Lamin B1 | Lamins are components of the nuclear lamina, a fibrous layer on the nucleoplasmic side of the inner nuclear membrane, which is thought to provide a framework for the nuclear envelope and may also interact with chromatin |
MKI67 | Marker of proliferation ki-67 | Thought to be required for maintaining cell proliferation |
MCM2 | Minichromosome maintenance complex component 2 | Acts as a factor that allows the DNA to undergo a single round of replication per cell cycle. Required for the entry in S phase and for cell division |
NCAPD2 | Non-SMC condensin I complex subunit D2 | Regulatory subunit of the condensin complex, a complex required for conversion of interphase chromatin into mitotic-like condense chromosomes. The condensin complex probably introduces positive supercoils into relaxed DNA in the presence of type I topoisomerases and converts nicked DNA into positive knotted forms in the presence of type II topoisomerases. May target the condensin complex to DNA via its C-terminal domain |
RFC4 | Replication factor C subunit 4 | The elongation of primed DNA templates by DNA polymerase delta and epsilon requires the action of the accessory proteins proliferating cell nuclear antigen (PCNA) and activator 1. This subunit may be involved in the elongation of the multiprimed DNA template |
UBE2C | Ubiquitin conjugating enzyme E2 C | Catalyzes the covalent attachment of ubiquitin to other proteins. Required for the destruction of mitotic cyclins |
RNA sequencing expression of hub genes and IHC
GEPIA (http://gepia.cancer-pku.cn) was used to analyze the differential expression of hub genes in ovarian cancer tissues (based on the TCGA database) and normal ovarian tissues (based on the Genotype Tissue Expression database). We found that the expression of all hub genes in ovarian cancer tissues was higher than that in normal tissues, as shown in Figure S4. To further research the distinction of the hub genes, protein expression was analyzed with the Human Protein Atlas (https://www.proteinatlas.org). As shown in Figure 4, the protein levels of 8 selected hub genes were significantly upregulated in ovarian cancer tissues compared to normal tissues. Furthermore, IHC staining suggested that the expression of these 8 genes was closely related. The protein expression levels of these 8 genes were consistently significantly higher in TCGA 2,347 than in TCGA 2,114.
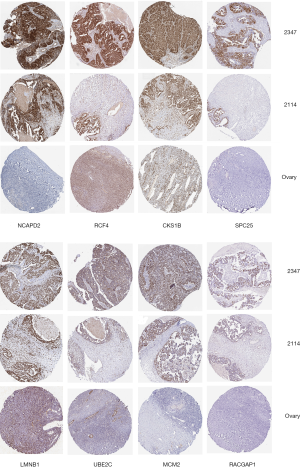
Correlations between hub genes
GEPIA was applied to determine the correlations between hub genes. Gene expression was analyzed in 422 ovarian cancer patients from the TCGA. BUB1B, KIF20A, CENPE, MCM2, NDC80 and UBE2C were subjected to correlation analysis. The results are showed in Figure 5. The correlation coefficient ranged from 0.61 to 0.83 (P<0.001), suggesting that these genes were strongly correlated.
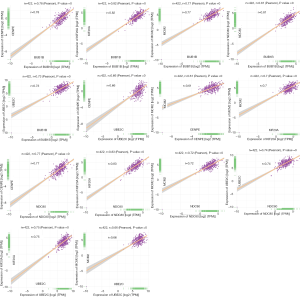
Discussion
The expression of genes is the basis of biological function, however, genes are located in large and complex networks. Alterations in gene expression undoubtedly cause a series of chain reactions, therefore, the effect may be amplified or inefficient. However, the hallmarks of cancer are characterized by functional capabilities that allow malignant tumor cells to proliferate and disseminate during tumorigenesis (10). Hence, hallmarks are simply a reflection of a series of chain reactions triggered by gene expression differences. In the clinic, BRCA1/2 mutation is the indication for olaparib as maintenance therapy in patients with platinum-sensitive, relapsed ovarian cancer (11); however, a more scientific description is that homologous recombination repair (HRR) deficiency, whether driven by defects in BRCA1, BRCA2 or other pathway components, and PARP inhibitors (PARPi) together cause synthetic lethality (12). In summary, hallmarks more accurately reflect biological function than DEGs. Similar to the evolution of organisms, tumorigenesis is a multistep process, as normal cells evolve progressively into a neoplastic state. In the process, cells acquire a succession of hallmark capabilities (2), such as sustained proliferative signaling and activation of invasion and metastasis. To identify common hallmarks of ovarian cancer, we used three GEO datasets and identified 11 significant hallmarks through GSEA using hallmark gene sets (13). It is absolutely clear that 5 of the 11 enriched hallmarks are associated with cell proliferation (hallmarks: E2F targets, G2M_checkpoint, MYC_TARGETS_V1, MYC_TARGETS_V2 and MITOTIC_SPINDLE). This perfectly explains why infinite proliferation is a common feature of all malignant tumors. Three hallmarks (ESTROGEN_RESPONSE_EARLY, KRAS_SIGNALING_UP and MTORC1_SIGNALING) of signaling were enriched. Changes in metabolism, such as glycolysis and gluconeogenesis, occur in ovarian cancer and are predictable (10) because malignant tumor cells need more energy than normal cells to proliferate and migrate. EPITHELIAL_MESENCHIMAL_TRANSITION is another essential hallmark (14). However, there were no common DEGs in 4 of the 11 enriched hallmarks (e.g., EPITHELIAL_MESENCHIMAL_TRANSITION). One possible reason for this finding may be that several key genes play important roles independently in these hallmarks that is reflected in another way (i.e., hallmarks more accurately explain biological function than DEGs).
According to Metascape gene list analysis on web, the DEGs in selected hallmarks have more functions than these hallmarks (e.g., chemical and genetic perturbations and immunologic signatures). Next, we found a close relationship among the hallmarks through a network of enriched terms in the multiple gene list pattern. However, all genes coexist in one cell, so we should build a closer network without repetitive genes.
Through survival analysis and PPI network construction, we identified 22 hub genes involved in the oncogenesis of ovarian cancer. The majority of these genes are related to proliferation (BUB1B, CDKN3, CENPE, CKS1B, HMMR, KIF11, KIF15, KIF18B, MCM2, MKI67, NCAPD2, RACGAP1, RAD51AP1, SPC25, NDC80, PBK, STIL, TPX2, and UBE2C).
In the PPI network, PBK (PDZ binding kinase) had the most connections (PBK interacted with other 21 genes). PBK is one of the key downstream molecules in the phosphoinositide 3-kinase signaling pathway. PBK knockdown results in G2/M cell cycle arrest that causes a decrease in CDC2 and cyclin B expression (15). However, high PBK expression may promote tumor cell growth by mediating p38 activation and helping cells resist DNA damage (16). Among the genes mentioned above, BUB1B, NDC80, CDC20, CDK1, CENPE and SPC25, as MCODE components, interact with each other. BUB1B (mitotic checkpoint serine/threonine kinase B), as an essential component of the mitotic checkpoint, has been demonstrated to promote tumor proliferation in breast cancer (17). It has also been proven that BUB1B knockdown inhibited tumor cell growth and triggered apoptosis in vivo (18). NDC80 (kinetochore complex component), as a component of the essential kinetochore-associated NDC80 complex, plays an important role in cell mitosis. NDC80 is a key component of the outer kinetochore that maintains chromosome stability. Cell apoptosis and cell cycle arrest at S phase may be caused by NDC80 knockdown (19). Though CDC20 (cell division cycle protein 20) showed no significant correlation with ovarian cancer in Kaplan-Meier plotter survival analysis, the important role of CDC20 in the regulation of the cell cycle and apoptosis cannot be denied. CDC20 not only regulates the cell cycle and apoptosis but also plays a role in the resistance of cancer cells to chemotherapy and radiotherapy (20). CDK1 plays a key role in the M phase of the cell cycle. Regardless of whether the cell enters or exits the proliferation cycle, it is directly related to the overexpression of CDK1, which can disrupt the cell cycle process so that the cell cannot grow and differentiate normally. Overexpression of CDK1 often leads to malignant cell proliferation and eventually to malignant tumor formation (21). CENPE (centromere-associated protein E) is a centromere binding protein and mitotic kinesin that accumulates in the G2 phase of the cell cycle. The elevated expression of CENPE is essential to prostate cancer progression (22). SPC25 (spindle component 25) is a protein that involves in spindle checkpoint activity and kinetochore-microtubule interactions. Tumorigenesis can arise from genetic instability in the cell cycle owing to inaccurate chromosomal segregation (23), a process in which SPC25 plays a key role. SPC25 is upregulated in lung cancer and prostate cancer and affects proliferation and cell cycle progression in prostate cancer cells (24,25).
Notably, all the genes mentioned above participate in the regulation of the cell cycle and play a positive role in carcinogenesis. In addition, the following genes have similar effects. MCM2 (minichromosome maintenance protein 2) is regulated during the cell cycle and depends on oxygen concentrations (26). MCM2 mRNA is synthesized through the action of E2F during the G1 phase of the cell cycle. The protein levels of MCM2 are dramatically decreased in aged cells (27). Increasing evidence indicates that the MCM2 protein is a reliable diagnostic marker of several cancers. MCM2 is overexpressed in cervical carcinoma (28). MKI67 (marker of proliferation Ki-67), which is required for maintaining cell proliferation, is considered the gold standard biomarker for proliferation and prognosis. UBE2C (ubiquitin conjugating enzyme E2 C) is a key regulator of cell cycle progression. Cells overexpressing UBE2C fail to maintain spindle checkpoint activity after entering mitosis, resulting in chromosome missegregation and aneuploidy (29). UBE2C is overexpressed in malignant melanoma and is associated with poor survival in these patients (30). STIL (SCL/TAL1 interrupting locus) is important for controlling spindle orientation and gives rise to subventricular zone cells (31). STIL, as a cell cycle-regulated protein specifically recruited at the mitotic centrosome, promotes the duplication of centrioles in dividing cells (32). The high expression of STIL in lung cancer and pancreatic cancer is related to the invasion and prognosis of tumors (33,34). TPX2 (targeting protein for Xenopus kinesin-like protein 2) is a microtubule-associated protein that plays a crucial role in the formation of the mitotic spindle (35). Cells with a deficiency in TPX2 develop short spindles, causing mitotic failure and cell apoptosis (36). However, overexpressed TPX2 is closely correlated with chromosomal instability, resulting in the development of malignant tumors (35). CKS1B (CDC28 protein kinase regulatory subunit 1B) binds to the catalytic subunit of cyclin-dependent kinases and is essential for their biological function. CKS1B downregulation can inhibit the proliferation, invasion and angiogenesis of retinoblastoma cells by suppressing the MEK/ERK signaling pathway (37). RFC4 (replication factor C subunit 4) is highly overexpressed in cancer tissues, such as liver cancer, prostate cancer and cervical cancer (38-40). The expression level of RFC4 is correlated with the degree of lesion progression. Niu et al. found that RFC4 expression was higher in cervical squamous cell carcinoma than in high-grade squamous intraepithelial lesions (38). Genes in the kinesin family (KIF11, KIF15, KIF18B, and KIF20A) are derived from a superfamily of motor proteins. All can be induced by estrogen. It has been shown that knocking down KIF11 leads to cellular death because of permeabilization of the lysosomal membrane (41). KIF15 is increased in breast cancer and serves as an independent prognostic factor of poor patient outcomes (42). The expression of KIF18B is regulated in a cell cycle-dependent manner and may play a crucial role in cell division. KIF18B controls microtubule length to center the mitotic spindle at M phase and plays an essential role in positioning the spindle (43). KIF20A is upregulated in malignant tumors such as gastric cancer (44) and pancreatic cancer (45) but barely expressed in normal organs. Silencing KIF20A inhibits the migration, invasion and proliferation of non-small cell lung cancer (46). The abnormal expression of KIF20A mainly interferes with spindle formation and chromosome separation during mitosis, thus forming polyploidy, which eventually leads to the proliferation, invasion and distant metastasis of cancer cells and affects the prognosis of patients. It, as a glycolysis hallmark, can hydrolyze ATP to produce mechanical energy. RACGAP1 (Rac GTPase activating protein 1), as a member of the guanine triphosphatase (GTPase) activation protein (GAP) family, is essential for the early stages of embryogenesis and plays a role in the microtubule-dependent steps in cytokinesis. Because of its potential role in promoting tumor progression, RACGAP1 is treated as an oncogene in basal-like breast cancers (47). RACGAP1 could be a predictive biomarker for a poor prognosis in colorectal cancer and lymph node metastasis (48). CDKN3 (cyclin-dependent kinase inhibitor 3), also known as cyclin-dependent kinase-associated protein phosphatase (KAP), is essential for the maintenance of chromosomal stability and accumulates just before mitosis at the G2 phase of the cell cycle. CDKN3 acts as a tumor suppressor depending on its dephosphorylation function of CDK1 (49,50). HMMR (hyaluronan-mediated motility receptor), as a multifunctional oncogenic protein (51), is involved in cell proliferation and motility. Many studies have reported that it is overexpressed in human leukemias, ovarian cancer and breast cancer (52,53). HMMR overexpression indicates a poor clinical outcome (53). RAD51AP1 (RAD51-associated protein 1) participates in a common DNA damage response pathway associated with the activation of double-strand break repair and homologous recombination. The alternative lengthening of telomeres is a homology-directed repair mechanism of telomere elongation that controls proliferation in aggressive cancers. RAD51AP1 protein levels are elevated in tumor cells with alternative telomere lengths. This indicates that RAD51AP1 is an essential mediator of the alternative telomere lengthening (54).
Using public databases (GEPIA and The Human Protein Atlas), we further determined that the hub genes are more highly expressed at the mRNA and protein levels in ovarian cancer tissues than in normal tissues. The strong correlations of these genes were verified through correlation analysis. The results were consistent with those from the PPI network analysis. Therefore, these hub genes have a synergistic effect on ovarian tumorigenesis.
In summary, most of the hub genes described herein are directly involved in cell cycle regulation, and the remainder also play an indirect role in cell proliferation.
Conclusions
Not only the hallmarks enriched by GSEA but also the hub genes extracted by the PPI network indicate that the most fundamental trait of ovarian cancer cells involves their ability to sustain chronic proliferation.
Acknowledgments
Funding: The present study was supported by
Footnote
Reporting Checklist: The authors have completed the MDAR checklist. Available at http://dx.doi.org/10.21037/tcr-20-2604
Conflicts of Interest: All authors have completed the ICMJE uniform disclosure form (available at http://dx.doi.org/10.21037/tcr-20-2604). The authors have no conflicts of interest to declare.
Ethical Statement: The authors are accountable for all aspects of the work in ensuring that questions related to the accuracy or integrity of any part of the work are appropriately investigated and resolved. The study was conducted in accordance with the Declaration of Helsinki (as revised in 2013). The present study was reviewed and approved by the Institutional Review Board and the Research Ethics Committee of Shanghai General Hospital (No. 2018KY075).
Open Access Statement: This is an Open Access article distributed in accordance with the Creative Commons Attribution-NonCommercial-NoDerivs 4.0 International License (CC BY-NC-ND 4.0), which permits the non-commercial replication and distribution of the article with the strict proviso that no changes or edits are made and the original work is properly cited (including links to both the formal publication through the relevant DOI and the license). See: https://creativecommons.org/licenses/by-nc-nd/4.0/.
References
- Siegel RL, Miller KD, Jemal A. Cancer statistics, 2019. CA Cancer J Clin 2019;69:7-34. [Crossref] [PubMed]
- Hanahan D, Weinberg RA. Hallmarks of cancer: the next generation. Cell 2011;144:646-74. [Crossref] [PubMed]
- Yu G, Wang LG, Han Y, et al. clusterProfiler: an R package for comparing biological themes among gene clusters. Omics 2012;16:284-7. [Crossref] [PubMed]
- Zhou Y, Zhou B, Pache L, et al. Metascape provides a biologist-oriented resource for the analysis of systems-level datasets. Nat Commun 2019;10:1523. [Crossref] [PubMed]
- Nagy A, Lanczky A, Menyhart O, et al. Validation of miRNA prognostic power in hepatocellular carcinoma using expression data of independent datasets. Sci Rep 2018;8:9227. [Crossref] [PubMed]
- Franceschini A, Szklarczyk D, Frankild S, et al. STRING v9.1: protein-protein interaction networks, with increased coverage and integration. Nucleic Acids Res 2013;41:D808-15. [Crossref] [PubMed]
- Tang Z, Li C, Kang B, et al. GEPIA: a web server for cancer and normal gene expression profiling and interactive analyses. Nucleic Acids Res 2017;45:W98-W102. [Crossref] [PubMed]
- Kampf C, Olsson I, Ryberg U, et al. Production of tissue microarrays, immunohistochemistry staining and digitalization within the human protein atlas. J Vis Exp 2012;3620. [Crossref] [PubMed]
- Krzywinski M, Schein J, Birol I, et al. Circos: an information aesthetic for comparative genomics. Genome Res 2009;19:1639-45. [Crossref] [PubMed]
- Vajaria BN, Patel PS. Glycosylation: a hallmark of cancer? Glycoconj J 2017;34:147-56. [Crossref] [PubMed]
- Pujade-Lauraine E, Ledermann JA, Selle F, et al. Olaparib tablets as maintenance therapy in patients with platinum-sensitive, relapsed ovarian cancer and a BRCA1/2 mutation (SOLO2/ENGOT-Ov21): a double-blind, randomised, placebo-controlled, phase 3 trial. Lancet Oncol 2017;18:1274-84. [Crossref] [PubMed]
- Lord CJ, Ashworth A. PARP inhibitors: Synthetic lethality in the clinic. Science 2017;355:1152-8. [Crossref] [PubMed]
- Liberzon A, Birger C, Thorvaldsdottir H, et al. The Molecular Signatures Database (MSigDB) hallmark gene set collection. Cell Syst 2015;1:417-25. [Crossref] [PubMed]
- Raja R, Pandey A, Kumar P. Epithelial to mesenchymal plasticity: role in cancer progression. Front Biosci (Landmark Ed) 2020;25:838-73. [Crossref] [PubMed]
- Liu Y, Liu H, Cao H, et al. PBK/TOPK mediates promyelocyte proliferation via Nrf2-regulated cell cycle progression and apoptosis. Oncol Rep 2015;34:3288-96. [Crossref] [PubMed]
- Ayllon V, O'Connor R. PBK/TOPK promotes tumour cell proliferation through p38 MAPK activity and regulation of the DNA damage response. Oncogene 2007;26:3451-61. [Crossref] [PubMed]
- Wang Z, Katsaros D, Shen Y, et al. Biological and Clinical Significance of MAD2L1 and BUB1, Genes Frequently Appearing in Expression Signatures for Breast Cancer Prognosis. PLoS One 2015;10:e0136246. [Crossref] [PubMed]
- Wan X, Yeung C, Kim SY, et al. Identification of FoxM1/Bub1b signaling pathway as a required component for growth and survival of rhabdomyosarcoma. Cancer Res 2012;72:5889-99. [Crossref] [PubMed]
- Ju LL, Chen L, Li JH, et al. Effect of NDC80 in human hepatocellular carcinoma. World J Gastroenterol 2017;23:3675-83. [Crossref] [PubMed]
- Wan L, Tan M, Yang J, et al. APC(Cdc20) suppresses apoptosis through targeting Bim for ubiquitination and destruction. Dev Cell 2014;29:377-91. [Crossref] [PubMed]
- Brown NR, Korolchuk S, Martin MP, et al. CDK1 structures reveal conserved and unique features of the essential cell cycle CDK. Nat Commun 2015;6:6769. [Crossref] [PubMed]
- Liang Y, Ahmed M, Guo H, et al. LSD1-Mediated Epigenetic Reprogramming Drives CENPE Expression and Prostate Cancer Progression. Cancer Res 2017;77:5479-90. [Crossref] [PubMed]
- Tooley J, Stukenberg PT. The Ndc80 complex: integrating the kinetochore's many movements. Chromosome Res 2011;19:377-91. [Crossref] [PubMed]
- Wang Q, Zhu Y, Li Z, et al. Up-regulation of SPC25 promotes breast cancer. Aging (Albany NY) 2019;11:5689-704. [Crossref] [PubMed]
- Cui F, Tang H, Tan J, et al. Spindle pole body component 25 regulates stemness of prostate cancer cells. Aging (Albany NY) 2018;10:3273-82. [Crossref] [PubMed]
- Ishimi Y. Regulation of MCM2-7 function. Genes Genet Syst 2018;93:125-33. [Crossref] [PubMed]
- Dumit VI, Kuttner V, Kappler J, et al. Altered MCM protein levels and autophagic flux in aged and systemic sclerosis dermal fibroblasts. J Invest Dermatol 2014;134:2321-30. [Crossref] [PubMed]
- Ishimi Y, Okayasu I, Kato C, et al. Enhanced expression of Mcm proteins in cancer cells derived from uterine cervix. Eur J Biochem 2003;270:1089-101. [Crossref] [PubMed]
- Reddy SK, Rape M, Margansky WA, et al. Ubiquitination by the anaphase-promoting complex drives spindle checkpoint inactivation. Nature 2007;446:921-5. [Crossref] [PubMed]
- Liu G, Zhao J, Pan B, et al. UBE2C overexpression in melanoma and its essential role in G2/M transition. J Cancer 2019;10:2176-84. [Crossref] [PubMed]
- LaMonica BE, Lui JH, Hansen DV, et al. Mitotic spindle orientation predicts outer radial glial cell generation in human neocortex. Nat Commun 2013;4:1665. [Crossref] [PubMed]
- Patwardhan D, Mani S, Passemard S, et al. STIL balancing primary microcephaly and cancer. Cell Death Dis 2018;9:65. [Crossref] [PubMed]
- Erez A, Perelman M, Hewitt SM, et al. Sil overexpression in lung cancer characterizes tumors with increased mitotic activity. Oncogene 2004;23:5371-7. [Crossref] [PubMed]
- Kasai K, Inaguma S, Yoneyama A, et al. SCL/TAL1 interrupting locus derepresses GLI1 from the negative control of suppressor-of-fused in pancreatic cancer cell. Cancer Res 2008;68:7723-9. [Crossref] [PubMed]
- Aguirre-Portoles C, Bird AW, Hyman A, et al. Tpx2 controls spindle integrity, genome stability, and tumor de-velopment. Cancer Res 2012;72:1518-28. [Crossref] [PubMed]
- Ozlu N, Srayko M, Kinoshita K, et al. An essential function of the C. elegans ortholog of TPX2 is to localize activated aurora A kinase to mitotic spindles. Dev Cell 2005;9:237-48. [Crossref] [PubMed]
- Zeng Z, Gao ZL, Zhang ZP, et al. Downregulation of CKS1B restrains the proliferation, migration, invasion and angiogenesis of retinoblastoma cells through the MEK/ERK signaling pathway. Int J Mol Med 2019;44:103-14. [Crossref] [PubMed]
- Niu G, Wang D, Pei Y, et al. Systematic identification of key genes and pathways in the development of invasive cervical cancer. Gene 2017;618:28-41. [Crossref] [PubMed]
- LaTulippe E, Satagopan J, Smith A, et al. Comprehensive gene expression analysis of prostate cancer reveals distinct transcriptional programs associated with metastatic disease. Cancer Res 2002;62:4499-506. [PubMed]
- Arai M, Kondoh N, Imazeki N, et al. The knockdown of endogenous replication factor C4 decreases the growth and enhances the chemosensitivity of hepatocellular carcinoma cells. Liver Int 2009;29:55-62. [Crossref] [PubMed]
- Groth-Pedersen L, Aits S, Corcelle-Termeau E, et al. Identification of cytoskeleton-associated proteins essential for lysosomal stability and survival of human cancer cells. PLoS One 2012;7:e45381. [Crossref] [PubMed]
- Zou JX, Duan Z, Wang J, et al. Kinesin family deregulation coordinated by bromodomain protein ANCCA and histone methyltransferase MLL for breast cancer cell growth, survival, and tamoxifen resistance. Mol Cancer Res 2014;12:539-49. [Crossref] [PubMed]
- McHugh T, Gluszek AA, Welburn JPI. Microtubule end tethering of a processive kinesin-8 motor Kif18b is required for spindle positioning. J Cell Biol 2018;217:2403-16. [Crossref] [PubMed]
- Yan GR, Zou FY, Dang BL, et al. Genistein-induced mitotic arrest of gastric cancer cells by downregulating KIF20A, a proteomics study. Proteomics 2012;12:2391-9. [Crossref] [PubMed]
- Stangel D, Erkan M, Buchholz M, et al. Kif20a inhibition reduces migration and invasion of pancreatic cancer cells. J Surg Res 2015;197:91-100. [Crossref] [PubMed]
- Xie F, He C, Gao S, et al. KIF20A silence inhibits the migration, invasion and proliferation of non-small cell lung cancer and regulates the JNK pathway. Clin Exp Pharmacol Physiol 2020;47:135-142. [Crossref] [PubMed]
- Lawson CD, Der CJ. Filling GAPs in our knowledge: ARHGAP11A and RACGAP1 act as oncogenes in basal-like breast cancers. Small GTPases 2018;9:290-6. [Crossref] [PubMed]
- Imaoka H, Toiyama Y, Saigusa S, et al. RacGAP1 expression, increasing tumor malignant potential, as a predictive biomarker for lymph node metastasis and poor prognosis in colorectal cancer. Carcinogenesis 2015;36:346-54. [Crossref] [PubMed]
- Dai W, Miao H, Fang S, et al. CDKN3 expression is negatively associated with pathological tumor stage and CDKN3 inhibition promotes cell survival in hepatocellular carcinoma. Mol Med Rep 2016;14:1509-14. [Crossref] [PubMed]
- Li H, Jiang X, Yu Y, et al. KAP regulates ROCK2 and Cdk2 in an RNA-activated glioblastoma invasion pathway. Oncogene 2015;34:1432-41. [Crossref] [PubMed]
- Fagerberg L, Hallstrom BM, Oksvold P, et al. Analysis of the human tissue-specific expression by genome-wide integration of transcriptomics and antibody-based proteomics. Mol Cell Proteomics 2014;13:397-406. [Crossref]
- Buttermore ST, Hoffman MS, Kumar A, et al. Increased RHAMM expression relates to ovarian cancer progression. J Ovarian Res 2017;10:66. [Crossref] [PubMed]
- Wang C, Thor AD, Moore DH 2nd, et al. The overexpression of RHAMM, a hyaluronan-binding protein that regulates ras signaling, correlates with overexpression of mitogen-activated protein kinase and is a significant parameter in breast cancer progression. Clin Cancer Res 1998;4:567-76. [PubMed]
- Barroso-Gonzalez J, Garcia-Exposito L, Hoang SM, et al. RAD51AP1 Is an Essential Mediator of Alternative Lengthening of Telomeres. Mol Cell 2019;76:217. [Crossref] [PubMed]