Identification of candidate genes associated with papillary thyroid carcinoma pathogenesis and progression by weighted gene co-expression network analysis
Introduction
The incidence of thyroid cancer has increased in recent years. According to data from the National Cancer Center, the 2018 incidence of thyroid cancer in China was 10.1 per 100,000 individuals, while the global incidence of thyroid cancer was 6.7 per 100,000 individuals; during the same period, the 5-year survival rate was 84.3% in China and was 98.1% in the United States (1). Papillary thyroid carcinoma (PTC) is the primary thyroid cancer type, accounting for approximately 80% of all thyroid cancers (2). Although the majority of patients with PTC have a good prognosis with surgery, adjuvant radio-iodine therapy, and thyroid-stimulating hormone therapy (3,4), the prognosis of advanced PTC is worse, with a significantly reduced 5-year survival rate of less than 60% and a markedly increased recurrence rate of more than 30% (5). It is therefore critical to explore the genes and potential mechanisms related to the occurrence and development of PTC, and to guide the molecular diagnosis and treatment of PTC patients.
In recent years, many studies have used bioinformatics analysis to analyze genes related to the incidence of PTC. Li’s research has indicated that the survivin gene mutation might be an excellent diagnostic criterion for PTC (6). Meanwhile, Han et al. (7) identified four differentially expressed genes (DEGs), including COMP, COL3A1, ZAP70, and CD247, to be related to PTC clinical phenotypes, that might be promising biomarkers for early-stage PTC. Furthermore, Ao et al. reported that 5 candidate genes (LRP4, KLK7, PRICKLE1, ETV4, and ETV5) could be used to predict the pathogenesis of PTC (8). These important findings have identified several biomarkers for early-stage PTC, primarily through the Gene Expression Omnibus (GEO) database; however, The Cancer Genome Atlas (TCGA), which includes a large number of PTC sequencing samples, has yet to be the subject of such an investigation to find genes related to the pathogenesis and progression of PTC. Therefore, exploring the molecular mechanisms and searching for novel biomarkers of PTC in the TCGA database may have considerable clinical value.
Weighted gene co-expression network analysis (WGCNA) is a novel method that has been used to effectively screen for new biomarkers in cancers (9). The WGCNA algorithm can group genes into modules based on the gene co-expression similarities across the samples, yielding a cluster of genes with similar functions that can be useful for relating modules and external sample traits. From these correlation networks, tissue-specific biomarkers and pathophysiological-related pathways can then be identified (10). This study used WGCNA to select core network genes and modules with high-expression oncogenes, to find candidate genes related to the pathogenesis and progression of PTC.
We present the following article in accordance with the MDAR reporting checklist (available at http://dx.doi.org/10.21037/tcr-20-2866).
Methods
Data acquisition
We downloaded the RNA sequencing (RNA-seq) expression data of 560 samples, including 58 normal and 502 PTC samples, and the corresponding clinical information of the 502 cases from the TCGA database (https://portal.gdc.cancer.gov/). The flowchart of the study design and the process of sample enrollment is illustrated in Figure 1. After using the random number method, 116 PTC samples were randomly selected from the dataset of the 502 PTC samples for pairing with normal tissues. We merged the count matrix of 116 PTC samples and 58 normal samples to form a test data matrix; we merged the count matrix of 386 PTC samples and 58 normal samples to form a validation data matrix; we merged the count matrix of 502 PTC samples and clinical-stage data to form a clinical-stage matrix.
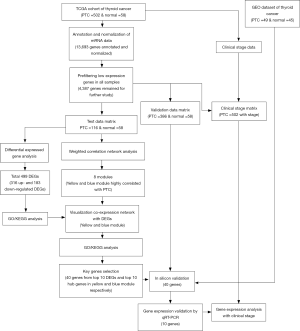
We also downloaded the gene expression dataset (accession number: GSE33630), which contained microarray data of 49 PTC samples and 45 adjacent normal thyroid samples from the NCBI GEO database (http://www.nibi.nih.gov/geo/) for further verification of the test data matrix.
The study was conducted in accordance with the Declaration of Helsinki (as revised in 2013).
DEG analysis
We used the test data matrix (RNA-seq data of 116 PTC and 58 normal samples) to analyze the DEGs between the PTC and normal samples using the “DESeq2” R package. We set the false discovery rate (FDR) to 0.05, and fold change was considered the differential expression ratio between PTC and normal samples. The criteria for DEGs were |log2(fold change)| >1 and FDR <0.05.
Construction of weighted gene co-expression networks and module identification
We analyzed the weighted co-expression network by using the test data matrix. To identify biologically meaningful gene modules containing highly correlated genes, a gene co-expression network was constructed using the “WGCNA” package in R software. We detected modules by setting MEDissThres to 0.25 in order to merge similar modules. We used average linkage hierarchical clustering based on topology overlap measurement (TOM) to identify gene co-expression modules, consisting of a group of genes with similar expression patterns. The soft threshold power was set to four based on the criterion of approximate scale-free topology. We used gene significance (GS) to qualify the link of individual genes to the trait of interest (PTC). In each module, we used module membership (MM) to evaluate the gene expression profile correlation and the module eigengene (11).
Gene interaction network construction and key gene identification
When the correlations between modules and traits (PTC and normal samples, respectively) were >0.5 and the P value was <0.05, and we considered this to be a module of interest. Topological data of the modules of interest (yellow and blue modules) were imported from the R program to Cytoscape (version 3.80) to visualize gene interaction network construction. The hub genes and subnetworks were identified using CytoHubba in Cytoscape. The analysis of DEGs was matched to the gene interaction network: genes that had a lower expression in PTC samples [log2 (fold change) <–1 and FDR <0.05] were labeled in green, while genes that had a higher expression in PTC samples [log2 (fold change) >1, and adjusted P<0.05] were labeled in red. The top 10 DEGs and the top 10 hub genes in each module of interest were selected for validation.
Validation by the RNA-seq data and GEO data in the modules of interest
We exported gene interactive networks of the yellow and blue modules from the R program to Cytoscape for visualization with the thresholds set as 0.17 and 0.27, respectively, to screen highly centralized genes (hub genes) in these modules. The validation data matrix (RNA-seq data of 386 PTC and 58 normal samples) and the GEO data were used to validate selected genes’ expression.
Cell culture and quantitative real-time polymerase chain reaction (qRT-PCR)
We used the human PTC-derived cell line (B-CPAP) and normal thyroid epithelial cell line (Nthy Ori3-1) from the American Type Culture Collection (ATCC, Manassas, VA, USA), and cultured them in DMEM/F-12 (Gibco, Grand Island, NY, USA) with 10% fetal bovine serum and 1% penicillin G and streptomycin in a 37 °C environment containing 5% CO2.
Ten core genes (the top five DEGs: LCN2, PDZK1IP1, GDF15, SLPI, and KCNN4; the top five of hub genes: MVP, OCIAD2, MMP2, SH3BGRL3, and S100A11) of interest in the yellow module were further verified in the B-CPAP and Nthy Ori3-1 cell lines by qRT-PCR. The primers of the 10 genes are listed in Table 1. We set β-actin as a control and the Nthy Ori-3-1 cell line as a calibrator sample for the PTC-derived cell line, and then calculated the relative expressions using the 2–ΔΔCt method. The Student’s t-test was used to compare gene expression levels, PTC-derived cell lines, and normal thyroid epithelial cell lines.
Table 1
Primer name | Forward | Backward |
---|---|---|
H-actin | GTCCACCGCAAATGCTTCTA | TGCTGTCACCTTCACCGTTC |
H-MVP | GGGCTTGGTGCTGTTTGAT | CCTTTAGATGGAGGGCAGTGTT |
H-OCIAD2 | AAAACACAAAGGGCCGGAAG | CTGTGTGGTCTGGGTCGC |
H-MMP2 | CGAGTGGATGCCGCCTTTA | CACGCTCTTCAGACTTTGGTTC |
H-SH3BGRL3 | CCAATGCAGGCCACTTCTC | CATTTGGGGCTGTTGCTTAA |
H-S100A11 | GAGTCCCTGATTGCTGTCTTCC | AGGGTCCTTCTGGTTCTTTGTG |
H-LCN2 | AGACAAAGACCCGCAAAAGAT | GCTGGCAACCTGGAACAAA |
H-PDZK1IP1 | GCACCCCGATGTAACCTTCT | ACCTTGGCTGGCTATACTTCAA |
H-GDF15 | CCGGATACTCACGCCAGAAG | GTCACGTCCCACGACCTTG |
H-SLPI | TGACACCCCAAACCCAACA | GACTCCAGAGCCTCCTCCATA |
H-KCNN4 | GAGAGGCAGGCTGTTAATGC | ACGTGCTTCTCTGCCTTGTT |
qRT-PCR, quantitative real-time polymerase chain reaction.
The expression of key genes in TNM stage
We used the clinical-stage matrix to analyze the connection between gene expression and the clinical TNM stage. The five genes (GDF15, LCN2, KCNN4, MMP2, and SH3BGRL3) verified by qRT-PCR were stratified by pathologic T, N, M, and American Joint Committee on Cancer (AJCC) stage, respectively. We used the Kruskal-Wallis test to analyze the omnibus difference and used the Wilcoxon test to analyze post-hoc pairwise comparison.
Enrichment analysis of DEGs and modules of interest
The Database for Annotation, Visualization, and Integrated Discovery (DAVID, http://david.abcc.ncifcrf.gov) was used for Gene Ontology (GO) enrichment analysis and Kyoto Encyclopedia of Genes and Genomes (KEGG) pathway enrichment. We uploaded the genes from the critical co-expression modules to identify the potential functions. The Benjamini correction was performed to calibrate the confidence level, with a q value <0.05 being considered the cutoff criterion. The upregulated and downregulated DEGs, and the modules of interest were enriched in GO and KEGG functions by DAVID. GO analysis results indicated enrichment in biological process (BP), cellular component (CC), and molecular function (MF).
Statistical analysis
Data from validation data matrix, clinical stage matrix and GEO dataset were displayed by median (also from minimum to maximum) due to that gene expression from matrix and dataset did not conform to a normal distribution. For validation in silicon, we used Wilcox test to verify the gene difference between normal and PTC groups. For examining gene expressions relating to TNM stage, we used the Kruskal-Wallis test to analyze the omnibus difference and used the Wilcoxon test to analyze post-hoc pairwise comparison. We set α as 0.05 and used Bonferroni correction to calibrate the confidence level, with a q value <0.05 being considered the cutoff criterion (12). Data from validation in vitro were displayed by mean ± standard deviation and Student t-test was used to analyze the difference between normal and PTC groups; P<0.05 was regarded as being statistically significant.
Results
Sample characteristics and DEG screening
Among the 502 samples, the mean age was 47.35 (range, 15 to 89) years. Male were 135, and female were 367. The main PTC clinical data are shown in Table 2. The RNA-seq count matrix contained 13,693 genes. The filtering criteria required that the row sums of gene counts be bigger than the upper quantile of the row sums, and 4,387 highly expressed genes in 560 samples were thus selected for test, validation, and clinical-stage analysis. We finally identified 499 DEGs, 316 of which were upregulated and 183 were downregulated in the PTC samples compared with normal samples (Figure 2A). The expression of DEGs was highly clustered in both PTC and normal samples (Figure 2B).
Table 2
Characteristics | PTC | Quantity |
---|---|---|
Gender | Male | 135 |
Female | 367 | |
T stage | T1 | 142 |
T2 | 164 | |
T3 | 171 | |
T4 | 23 | |
Tx* | 2 | |
N stage | N0 | 228 |
N1 | 224 | |
Nx* | 50 | |
M stage | M0 | 283 |
M1 | 9 | |
Mx* | 210 | |
AJCC TNM stage | I | 281 |
II | 52 | |
III | 113 | |
IV | 55 | |
NA* | 1 |
*, as we could not evaluate pathologic Tx representing papillary tumor, pathologic Nx representing regional (nearby) lymph nodes, or pathologic Mx representing distant metastasis, we treated Tx, Nx and Mx, as not applicable. PTC, papillary thyroid carcinoma.
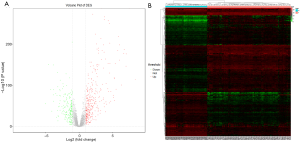
The yellow module was positively correlated with PTC, while the blue module was negatively correlated with PTC
Eight modules were selected: red (76 genes), turquoise (1,135 genes), yellow (94 genes), green (86 genes), black (73 genes), blue (896 genes), brown (468 genes), and grey (244 genes). The TOM of all genes is shown in Figure 3A. As shown in Figure 3B, seven modules (grey module excluded) were categorized into two main clusters: one cluster covered three modules (red, turquoise, and yellow module), while the other cluster involved four modules (green, black, blue, and brown module). Three pairs of modules had higher adjacencies: yellow and turquoise, blue and black, and brown and blue modules (Figure 3C). We selected 2 modules of interest: the yellow module (R2=0.61, P=2E–19) and the blue module (R2=–0.94, P=3E–85) (Figure 3D). The yellow module's correlation analysis revealed a positive interrelationship between gene expression and PTC, while that of the blue module showed a negative correspondence between gene expression and PTC. In the yellow module, GS and MM’s association was 0.84 (P=3.7E–26; Figure 3E), while that in the blue module was 0.96 (P<1E–200; Figure 3F).
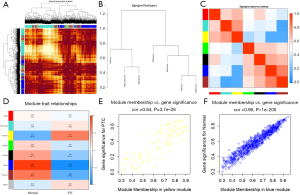
Network construction and key gene identification in PTC of the yellow and blue modules
After screening with a 0.17 threshold in the yellow module, 64 genes were visualized, with 31 of these being matched to DEGs (Figure 4A). After screening with a 0.27 threshold in the blue module, 111 genes were visualized, with 84 of these genes being matched to DEGs (Figure 4B). The top 10 DEGs in the yellow and blue modules are shown in Tables 3,4, respectively, and the top 10 hub genes in the core network in the yellow and blue modules are shown in Figure 4C,D.
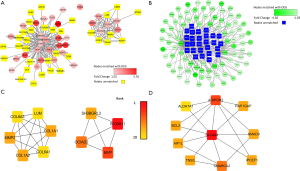
Table 3
Gene ID | Gene name | Log2 fold change | Adjusted P value |
---|---|---|---|
ENSG00000148346 | LCN2 | 5.553841607 | 2.95E–91 |
ENSG00000162366 | PDZK1IP1 | 4.530363669 | 2.63E–58 |
ENSG00000130513 | GDF15 | 4.319358493 | 1.57E–92 |
ENSG00000124107 | SLPI | 4.15074815 | 3.09E–55 |
ENSG00000104783 | KCNN4 | 4.029339699 | 1.14E–60 |
ENSG00000131435 | PDLIM4 | 3.990102564 | 1.68E–164 |
ENSG00000171345 | KRT19 | 3.194147299 | 2.67E–92 |
ENSG00000133110 | POSTN | 3.161981456 | 1.91E–33 |
ENSG00000108821 | COL1A1 | 2.845246535 | 4.43E–29 |
ENSG00000132470 | ITGB4 | 2.35982993 | 1.11E–59 |
DEG, differentially expressed gene.
Table 4
Gene | Gene name | Log2 fold change | Adjusted P value |
---|---|---|---|
ENSG00000074706 | IPCEF1 | –4.55752 | 1.33E–151 |
ENSG00000115112 | TFCP2L1 | –4.24682 | 8.54E–91 |
ENSG00000153246 | PLA2R1 | –3.85242 | 3.68E–150 |
ENSG00000080493 | SLC4A4 | –3.85126 | 6.26E–78 |
ENSG00000157680 | DGKI | –3.80398 | 3.17E–90 |
ENSG00000147606 | SLC26A7 | –3.80141 | 3.00E–62 |
ENSG00000157404 | KIT | –3.48921 | 6.25E–81 |
ENSG00000112562 | SMOC2 | –3.29676 | 2.92E–76 |
ENSG00000132561 | MATN2 | –3.23942 | 4.00E–132 |
ENSG00000091137 | SLC26A4 | –3.23634 | 4.50E–40 |
DEG, differentially expressed gene.
Among the 36 genes selected, 31 genes were consistent with the test data matrix, while five genes (COL1A2, COL6A3, MMP2, LUM and ABI3BP) were not consistent with the test data matrix
All the top 10 DEGs and the top 10 hub genes in the yellow and blue modules were selected for validation. COL1A1 was repeated both in the DEGs and hub genes in the yellow module, and thus, we finally selected 19 genes in the yellow module for validation. In all, 16 of 19 selected genes were highly expressed in the PTC group in the yellow module (q<0.05), while the expression of 3 genes (COL1A2, COL6A3, and MMP2) showed no significant difference between the PTC and normal group (q>0.05) in the validation data matrix (Figure 5). DGKI, IPCEF1, and SLC4A4 were the repeat genes in the blue module’s DEGs and hub genes. Thus, 17 genes were finally chosen to be validated in the blue module. All of the selected genes in the blue module had a low expression in the PTC group in the validation data matrix (q<0.05) (Figure 6).
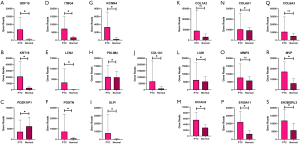
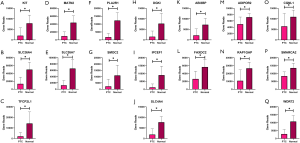
Because the array data from GEO did not contain ITBG4, we selected 35 genes from the test data matrix to verify in the GEO data. Among the 35 genes selected for validation, the expression of 32 genes was consistent with the test matrix (q<0.05), while the expression of three genes (MMP2 and LUM in the yellow module, and ABI3BP in the blue module) were inconsistent with the test matrix (q>0.05) (Figures 7,8).
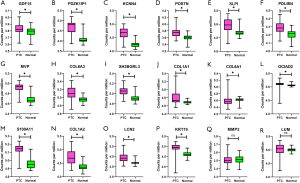
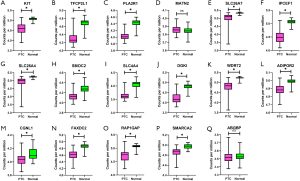
The expression of GDF15, MMP2, SH3BGRL3, KCNN4, and LCN2 were higher in the BCPAP cell line compared with the Nthy Ori3-1 cell line
Subsequently, we selected the 10 PTC-related genes in the yellow module and then validated the mRNA levels using qRT-PCR. We discovered that the expression levels of GDF15 (P=0.0004), MMP2 (P=0.0003), SH3BGRL3 (P=0.0003), KCNN4 (P=0.0228), and LCN2 (P=0.0389) were higher in the BCPAP cell line compared with the Nthy Ori3-1 cell line. In contrast, the expression of SLPI (P<0.0001), S100A11 (P<0.0001), OCIAD2 (P<0.0001), MVP (P=0.0004), and PDZK1IP1 (P=0.0013) were downregulated in the BCPAP cell line (Figure 9).
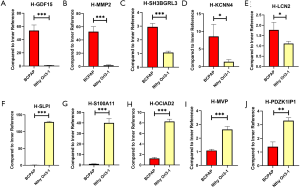
The expression of GDF15, LCN2, KCNN4, MMP2, and SH3BGRL3 was highly associated with TNM stage
As shown in Figure 10A,B,C,D,E, four genes (GDF15, LCN2, KCNN4, and SH3BGRL3) showed a much higher expression in the T3–T4 stages than in the T1–T2 stages (q<0.05). In contrast, the expression of MMP2 showed no difference across the T1–T4 stages (q>0.05), which indicated that the high expression of GDF15, LCN2, KCNN4, and SH3BGRL3 was associated with large tumor size and extensive growth beyond the thyroid gland. In pathological N analysis, the five genes were all more highly expressed in N1 than in N0 (q<0.05), indicating that the high expression of the five genes was associated with spread to nearby lymph nodes (Figure 10F,G,H,I,J). However, these five genes did not demonstrate a difference between M0 and M1 (q>0.05) (Figure 10K,L,M,N,O). Finally, we discovered that these five genes had a higher expression in stage IV than in other stages (q<0.05), which suggested that these genes might be correlated with a higher stage (Figure 10P,Q,R,S,T).
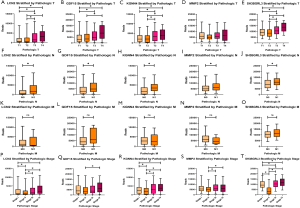
Functional enrichment in DEGs and modules of interest
The upregulated DEGs were significantly enriched in BP, CC, and MF showed in Figure 11A. As shown in Figure 11B, the downregulated DEGs that were significantly enriched in BP, CC, and MF. In the KEGG analysis, the upregulated DEGs were significantly enriched in the PI3K-Akt signaling pathway, focal adhesion, extracellular matrix (ECM)-receptor interaction, and proteoglycans in cancer. The downregulated DEGs were not significantly enriched in any pathways (q>0.05).
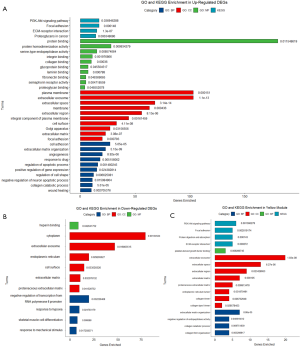
Genes in the yellow module were mainly enriched in 13 GO terms closely related to cancer, shown in Figure 11C. Moreover, in the yellow module, KEGG pathway enrichment analysis showed notable enrichment in the PI3K-Akt signaling pathway, focal adhesion, protein digestion and absorption, and ECM-receptor interaction. No GO or KEGG terms were significant (q>0.05) in the blue module, and no GO or KEGG pathways were enriched.
Discussion
In this study, we used WGCNA to identify two modules of interest: the yellow module, which was positively associated with PTC, and the blue module, which was negatively correlated with PTC. Four genes (GDF15, LCN2, KCNN4, and SH3BGRL3) that were identified from the yellow module, were highly correlated with the PTC occurrence. We also found that the four genes had significantly higher expression in cases of larger tumor size, lymph node metastasis, and higher AJCC stage, meaning that they were correlated with PTC progression.
Abundant research has found these four genes to be associated with the occurrence and progression of cancer. Mimeault et al. reported that growth differentiation factor 15 (GDF15) was significantly increased in injury, inflammation, cancer, and other diseases compared with normal physiological conditions (13), and higher expression of GDF15 was related to tumor tumorigenesis and progression. Studies by Yang et al. and Urakawa et al. showed that the high expression of GDF15 promoted tumorigenesis and progression in oral squamous cell carcinoma (14) and esophageal squamous cell carcinoma, respectively (15). Moreover, overexpression of GDF15 was shown to stimulate MEK/ERK and PI3K/Akt/mTOR signaling (16-18) and to assist in epithelial-mesenchymal transition (EMT) in ovarian and colorectal carcinomas cells (19,20). Many studies reported that lipocalin 2 (LCN2) was overexpressed in various cancers and associated with vascular invasion, TNM stage, and tumor recurrence (21-25). Wu et al. identified SH3 domain-binding glutamate-rich protein-like 3 (SH3BGRL3) as a highly relevant potential PTC candidate biomarker, as it was more highly expressed in PTC than in normal tissues (26); it was shown to promote the EMT, proliferation, and cell migration of urothelial carcinoma, and was also found to be highly connected with the epidermal growth factor receptor (EGFR) in bladder cancer (27). Potassium calcium-activated channel subfamily N member 4 (KCNN4) also demonstrated elevated expression in various types of cancer and was shown to be involved in promoting cell invasion and cell proliferation. Furthermore, the KCNN4-induced calcium signaling process also reported to be associated with the malignant phenotypes of numerous tumors, such as glioblastoma, pancreatic cancer, melanoma, and prostate cancer (28-31).
Our data indicated that a higher expression of these four genes was significantly related to the PTC’s pathogenesis and correlated with a higher stage. Consistent with our findings, recent studies have reported that the mRNA levels of GDF15 (32), LCN2 (24), and H3BGRL3 (26) display a notable increase in thyroid cancer, especially in PTC. KCNN4 may regulate cancer proliferation and invasion, and increased expression of KCNN4 has been found to be an indicator of poor clinical outcomes in various tumors (27,33-37). Collectively, these findings highlight this gene’s potentially significant role in cancer development. In this study, KCNN4 was screened out in PTC, implying that it might play an essential role in this malignancy.
By analyzing gene function and signaling pathways, we confirmed that genes in the yellow module and the upregulated DEGs were significantly enriched in the three vital signaling pathways of focal adhesion, ECM-receptor interaction, and PI3K-Akt signaling, which play critical roles in PTC. In line with our results, several studies have also reported focal adhesion (38,39), ECM-receptor interaction (39-41), and the PI3K-AKT signaling pathway (42,43) to be associated with the proliferation, metastasis, and development of PTC.
Several limitations of our study should also be mentioned. First, the test and validation data matrix used the same normal samples, which would lead to data independence. Thus, we used data from the GEO database for independent verification in the article and got the same result that four genes (GDF15, LCN2, KCNN4, and SH3BGRL3) from the yellow module that were highly correlated with the occurrence and progression of PTC. Second, we only used a single PTC cell line for verification, and more PTC cell lines would provide more substantial verification. Finally, future studies with in vivo animal models are required to further validate the present study’s conclusions.
Conclusions
We systematically identified four genes (GDF15, LCN2, KCNN4, and SH3BGRL3) from the yellow module that were highly correlated with the occurrence and progression of PTC. These findings provide insights into the mechanisms underlying the pathogenesis and progression of PTC and may serve as potential targets for the diagnosis and treatment of patients with this disease.
Acknowledgments
Funding: This work was supported by
Footnote
Reporting Checklist: The authors have completed the MDAR reporting checklist. Available at http://dx.doi.org/10.21037/tcr-20-2866
Peer Review File: Available at http://dx.doi.org/10.21037/tcr-20-2866
Conflicts of Interest: All authors have completed the ICMJE uniform disclosure form (available at http://dx.doi.org/10.21037/tcr-20-2866). The authors have no conflicts of interest to declare.
Ethical Statement: The authors are accountable for all aspects of the work in ensuring that questions related to the accuracy or integrity of any part of the work are appropriately investigated and resolved. The study was conducted in accordance with the Declaration of Helsinki (as revised in 2013).
Open Access Statement: This is an Open Access article distributed in accordance with the Creative Commons Attribution-NonCommercial-NoDerivs 4.0 International License (CC BY-NC-ND 4.0), which permits the non-commercial replication and distribution of the article with the strict proviso that no changes or edits are made and the original work is properly cited (including links to both the formal publication through the relevant DOI and the license). See: https://creativecommons.org/licenses/by-nc-nd/4.0/.
References
- Ferlay J, Colombet M, Soerjomataram I, et al. Estimating the global cancer incidence and mortality in 2018: GLO-BOCAN sources and methods. Int J Cancer 2019;144:1941-53. [Crossref] [PubMed]
- Liu J, Wang Y, Zhang B. Advances in diagnosis and treatment of thyroid cancer in children and adolescents. Zhongguo Yi Xue Ke Xue Yuan Xue Bao 2018;40:838-42. [PubMed]
- Morris LG, Shaha AR, Tuttle RM, et al. Tall-cell variant of papillary thyroid carcinoma: a matched-pair analysis of survival. Thyroid 2010;20:153-8. [Crossref] [PubMed]
- Wartofsky L. Increasing world incidence of thyroid cancer: increased detection or higher radiation exposure? Hormones (Athens) 2010;9:103-8. [Crossref] [PubMed]
- Stephen JK, Chitale D, Narra V, et al. DNA methylation in thyroid tumorigenesis. Cancers (Basel) 2011;3:1732-43. [Crossref] [PubMed]
- Li JY, Shi J, Sang JF, et al. Role of survivin in the pathogenesis of papillary thyroid carcinoma. Genet Mol Res 2015;14:15102-11. [Crossref] [PubMed]
- Han J, Chen M, Wang Y, et al. Identification of biomarkers based on differentially expressed genes in papillary thyroid carcinoma. Sci Rep 2018;8:9912. [Crossref] [PubMed]
- Ao ZX, Chen YC, Lu JM, et al. Identification of potential functional genes in papillary thyroid cancer by co-expression network analysis. Oncol Lett 2018;16:4871-8. [Crossref] [PubMed]
- Zhang B, Horvath S. A general framework for weighted gene co-expression network analysis. Stat Appl Genet Mol Biol 2005;4:Article17.
- Zhang G, Xu S, Yuan Z, et al. Weighted gene coexpression network analysis identifies specific modules and hub genes related to major depression. Neuropsychiatr Dis Treat 2020;16:703-13. [Crossref] [PubMed]
- Zhao W, Langfelder P, Fuller T, et al. Weighted gene coexpression network analysis: state of the art. J Biopharm Stat 2010;20:281-300. [Crossref] [PubMed]
- Storey JD, Taylor JE, Siegmund D. Strong control, conservative point estimation and simultaneous conservative consistency of false discovery rates: a unified approach. J R Stat Soc Series B Stat Methodol 2004;66:187-205. [Crossref]
- Mimeault M, Batra SK. Divergent molecular mechanisms underlying the pleiotropic functions of macrophage inhibitory cytokine-1 in cancer. J Cell Physiol 2010;224:626-35. [Crossref] [PubMed]
- Yang CZ, Ma J, Zhu DW, et al. GDF15 is a potential predictive biomarker for TPF induction chemotherapy and promotes tumorigenesis and progression in oral squamous cell carcinoma. Ann Oncol 2014;25:1215-22. [Crossref] [PubMed]
- Urakawa N, Utsunomiya S, Nishio M, et al. GDF15 derived from both tumor-associated macrophages and esophageal squamous cell carcinomas contributes to tumor progression via Akt and Erk pathways. Lab Invest 2015;95:491-503. [Crossref] [PubMed]
- Li S, Ma YM, Zheng PS, et al. GDF15 promotes the proliferation of cervical cancer cells by phosphorylating AKT1 and Erk1/2 through the receptor ErbB2. J Exp Clin Cancer Res 2018;37:80. [Crossref] [PubMed]
- Li YL, Chang JT, Lee LY, et al. GDF15 contributes to radioresistance and cancer stemness of head and neck cancer by regulating cellular reactive oxygen species via a SMAD-associated signaling pathway. Oncotarget 2017;8:1508-28. [Crossref] [PubMed]
- Sasahara A, Tominaga K, Nishimura T, et al. An autocrine/paracrine circuit of growth differentiation factor (GDF) 15 has a role for maintenance of breast cancer stem-like cells. Oncotarget 2017;8:24869-81. [Crossref] [PubMed]
- Li C, Wang J, Kong J, et al. GDF15 promotes EMT and metastasis in colorectal cancer. Oncotarget 2016;7:860-72. [Crossref] [PubMed]
- Griner SE, Joshi JP, Nahta R. Growth differentiation factor 15 stimulates rapamycin-sensitive ovarian cancer cell growth and invasion. Biochem Pharmacol 2013;85:46-58. [Crossref] [PubMed]
- Wang HJ, He XJ, Ma YY, et al. Expressions of neutrophil gelatinase-associated lipocalin in gastric cancer: a potential biomarker for prognosis and an ancillary diagnostic test. Anat Rec (Hoboken) 2010;293:1855-63. [Crossref] [PubMed]
- Zhang Y, Fan Y, Mei Z. NGAL and NGALR overexpression in human hepatocellular carcinoma toward a molecular prognostic classification. Cancer Epidemiol 2012;36:e294-9. [Crossref] [PubMed]
- Zhang M, Zhao X, Deng Y, et al. Neutrophil gelatinase associated lipocalin is an independent predictor of poor prognosis in cases of papillary renal cell carcinoma. J Urol 2015;194:647-52. [Crossref] [PubMed]
- Celestino R, Nome T, Pestana A, et al. CRABP1, C1QL1 and LCN2 are biomarkers of differentiated thyroid carci-noma, and predict extrathyroidal extension. BMC Cancer 2018;18:68. [Crossref] [PubMed]
- Rosignolo F, Sponziello M, Durante C, et al. Expression of PAX8 target genes in papillary thyroid carcinoma. PLoS One 2016;11:e0156658. [Crossref] [PubMed]
- Wu CC, Lin JD, Chen JT, et al. Integrated analysis of fine-needle-aspiration cystic fluid proteome, cancer cell se-cretome, and public transcriptome datasets for papillary thyroid cancer biomarker discovery. Oncotarget 2018;9:12079-100. [Crossref] [PubMed]
- Chiang CY, Pan CC, Chang HY, et al. SH3BGRL3 protein as a potential prognostic biomarker for urothelial carci-noma: a novel binding partner of epidermal growth factor receptor. Clin Cancer Res 2015;21:5601-11. [Crossref] [PubMed]
- Lallet-Daher H, Roudbaraki M, Bavencoffe A, et al. Intermediate-conductance Ca2+-activated K+ channels (IKCa1) regulate human prostate cancer cell proliferation through a close control of calcium entry. Oncogene 2009;28:1792-806. [Crossref] [PubMed]
- Bonito B, Sauter DR, Schwab A, et al. K(Ca)3.1 (IK) modulates pancreatic cancer cell migration, invasion and proliferation: anomalous effects on TRAM-34. Pflugers Arch 2016;468:1865-75. [Crossref] [PubMed]
- Ruggieri P, Mangino G, Fioretti B, et al. The inhibition of KCa3.1 channels activity reduces cell motility in glioblastoma derived cancer stem cells. PLoS One 2012;7:e47825. [Crossref] [PubMed]
- Schmidt J, Friebel K, Schönherr R, et al. Migration-associated secretion of melanoma inhibitory activity at the cell rear is supported by KCa3.1 potassium channels. Cell Res 2010;20:1224-38. [Crossref] [PubMed]
- Reyes I, Reyes N, Suriano R, et al. Gene expression profiling identifies potential molecular markers of papillary thyroid carcinoma. Cancer Biomark 2019;24:71-83. [Crossref] [PubMed]
- Li QT, Feng YM, Ke ZH, et al. KCNN4 promotes invasion and metastasis through the MAPK/ERK pathway in hepatocellular carcinoma. J Investig Med 2020;68:68-74. [Crossref] [PubMed]
- Jiang S, Zhu L, Yang J, et al. Integrated expression profiling of potassium channels identifys KCNN4 as a prognostic biomarker of pancreatic cancer. Biochem Biophys Res Commun 2017;494:113-9. [Crossref] [PubMed]
- Sakashita M, Sakashita S, Murata Y, et al. High expression of ovarian cancer immunoreactive antigen domain containing 2 (OCIAD2) is associated with poor prognosis in lung adenocarcinoma. Pathol Int 2018;68:596-604. [Crossref] [PubMed]
- Zhao YN, He DN, Wang YD, et al. Association of single nucleotide polymorphisms in the MVP gene with platinum resistance and survival in patients with epithelial ovarian cancer. Oncol Lett 2016;11:2925-33. [Crossref] [PubMed]
- Ferrer I, Quintanal-Villalonga A, Molina-Pinelo S, et al. MAP17 predicts sensitivity to platinum-based therapy, EGFR inhibitors and the proteasome inhibitor bortezomib in lung adenocarcinoma. J Exp Clin Cancer Res 2018;37:195. [Crossref] [PubMed]
- Elango R, Vishnubalaji R, Manikandan M, et al. Concurrent targeting of BMI1 and CDK4/6 abrogates tumor growth in vitro and in vivo. Sci Rep 2019;9:13696. [Crossref] [PubMed]
- Zhao H, Li H. Network-based meta-analysis in the identification of biomarkers for papillary thyroid cancer. Gene 2018;661:160-8. [Crossref] [PubMed]
- Li S, Yin Y, Yu H. Genetic expression profile-based screening of genes and pathways associated with papillary thyroid carcinoma. Oncol Lett 2018;16:5723-32. [Crossref] [PubMed]
- Zhang K, Liu J, Li C, et al. Identification and validation of potential target genes in papillary thyroid cancer. Eur J Pharmacol 2019;843:217-25. [Crossref] [PubMed]
- Wen J, Wang H, Dong T, et al. STAT3-induced upregulation of lncRNA ABHD11-AS1 promotes tumour progression in papillary thyroid carcinoma by regulating miR-1301-3p/STAT3 axis and PI3K/AKT signalling pathway. Cell Prolif 2019;52:e12569. [Crossref] [PubMed]
- Zang C, Sun J, Liu W, et al. miRNA-21 promotes cell proliferation and invasion via VHL/PI3K/AKT in papillary thyroid carcinoma. Hum Cell 2019;32:428-36. [Crossref] [PubMed]