Identification of prognostic genes and tumor-infiltrating immune cells in the tumor microenvironment of esophageal squamous cell carcinoma and esophageal adenocarcinoma
Introduction
Esophageal cancer (EC) is extremely aggressive and is the eighth most common malignancy worldwide, with a mortality rate that ranks sixth but is increasing (1-3). According to data from the National Central Cancer Registry of China, Chinese EC patients account for up to 70% of all EC cases globally (4). Of the 2 major histopathologic subtypes of EC, esophageal squamous cell carcinoma (ESCC) is more prevalent among Asians whereas esophageal adenocarcinoma (EAC) occurs at a higher rate in Western countries (5,6). Diagnostic tools and multidisciplinary treatment options for ESCC and EAC are constantly evolving; notably, the emergence of molecular targeted therapy and immunotherapy has prolonged the survival of patients. However, the prognosis of patients without sensitive gene mutations remains poor with a 5-year survival rate <25%, which is primarily due to the fact that cases tend to be diagnosed at an advanced stage of disease (7,8). There is therefore an urgent need for more specific and functionally relevant biomarkers for monitoring disease progression and predicting the outcome of EC.
The focal or systemic immune response against tumor cells is an important determinant of tumor progression (9). Immune biomarkers in antitumor immune responses can predict the immune status and prognosis of ESCC and EAC patients (10-12). Tumor cells and tumor-related cell structures in the tumor microenvironment (TME) are potential biomarkers for predicting cancer prognosis (13,14). Besides inflammatory cytokines and other factors (15), the 2 most important components of the tumor immune microenvironment (TIME) are tumor-infiltrating immune cells (TIICs) and stromal cells, which also have diagnostic and prognostic significance (16-19). The Estimation of Stromal and Immune Cells in Malignant Tumor Tissues Using Expression Data (ESTIMATE) algorithm uses stromal and immune scores to predict the infiltration of stromal/immune cells into the TME, which is related to prognosis in various cancers (20) including acute myeloid leukemia (21), glioblastoma (22), colon cancer (23), prostate cancer (24), breast cancer (25), and lung cancer (26). Although some researchers have used this algorithm to study EC, they did not include ESCC and EAC at the same time to find more accurate prognostic genes (27,28).
To this end, in the present study, we calculated stromal and immune scores of patient-derived EC tissues from The Cancer Genome Atlas (TCGA) database using the ESTIMATE package in R software, and used these to screen immune-related differentially expressed genes (DEGs) as candidate prognostic biomarkers in the TIME of EC. We then evaluated their prognostic value of the genes using Gene Expression Omnibus (GEO) datasets, and confirmed the expression of TIIC components in ESCC and EAC tissues and assessed their correlations with EACC and EAC prognosis. We present the following article in accordance with the MDAR checklist (available at http://dx.doi.org/10.21037/tcr-20-3078).
Methods
Data acquisition
Demographic and clinical characteristics including sex and tumor–node–metastasis (TNM) stage, respectively, as well as RNA sequencing reads from a cohort of 95 ESCC and 87 EAC patients were obtained from the TCGA database (https://portal.gdc.cancer.gov). Stromal/immune scores of patient-derived EC tissues were calculated using ESTIMATE. We also selected gene expression profiles of patient-derived ESCC tissue (GSE53625, GPL18109, n=358) and EAC tissue (GSE19417, GPL4372, n=76) from the GEO database to validate the candidate prognostic genes identified from TCGA data. The proportions of TIICs in tumor tissues were analyzed using the CIBERSORT algorithm (http://cibersort.stanford.edu/). The study was conducted in accordance with the Declaration of Helsinki (as revised in 2013). All data in this study come from public data in the TCGA and GEO databases, and therefore ethics approval was waived ethics approval was waived.
Identification of DEGs based on ESTIMATE scores
EC patients were assigned to the high- or low-score group according to median stromal/immune (ESTIMATE) score. Genes that were differentially expressed between the 2 groups were screened using the Bioconductor “limma” package (https://bioconductor.org/packages/release/bioc/html/limma.html) in R v3.6.3 software, with a threshold of P<0.05 and |fold change|>1.
Functional enrichment analysis
The “Bioconductor clusterProfiler 3.8”, “enrichplot 3.8”, and “Rlease ggplot2 3.1.1” packages of R software were used to evaluate functional enrichment of identified DEGs. Kyoto Encyclopedia of Genes and Genomes (KEGG) pathway analysis was performed to identify relevant pathways; and Gene Ontology (GO) analysis encompassing biological process (BP), cell composition (CC), and molecular function (MF) was carried out to determine the functions of DEGs. An adjusted P value <0.05 was set as the cutoff.
Protein-protein interaction (PPI) network construction
PPI networks were constructed by mapping DEGs using Search Tool for the Retrieval of Interacting Genes/Proteins (STRING) database v11.0 (http://string-db.org/) (29). We imported the PPI networks into Cytoscape software (https://cytoscape.org/), and the top 3 significant modules for ESCC and EAC were identified using the MCODE algorithm in Cytoscape.
Survival analysis
Correlations between ESTIMATE scores and survival rates of ESCC and EAC patients were separately evaluated in the high- and low-score groups, and the correlation between overlapping DEGs and survival rates was analyzed. In addition, we used the ESCC and EAC datasets of the GEO database to further validate the prognostic value of these genes. P<0.05 is considered a significant difference in survival.
Correlation analysis between TIICs and prognosis in EC
We calculated the proportions of 22 subpopulations of TIICs in ESCC and EAC using the CIBERSORT algorithm (30). Then the correlation heatmap was produced to evaluate the associations between these proportions and the prognosis of ESCC and EAC.
Gene set enrichment analysis (GSEA)
GSEA was performed using TCGA cohort to identify pathways that were most relevant to prognostic DEGs in ESCC and EAC (31). The median expression levels of validated DEGs in the high- and low-infiltration groups were used as standards. Cancer hallmark-based gene sets (50 genes each for ESCC and EAC) were identified. A weighted enrichment statistic was used with 5,000 permutations. P<0.01 and a false discovery rate <0.25 were considered significant.
Statistical analysis
Nonparametric tests (Wilcox test for 2 groups and Kruskal–Wallis test for ≥3 groups) were used to assess correlations between stromal/immune scores and clinicopathologic parameters. DEG and functional pathway enrichment analyses were performed using the Bioconductor package in R. Kaplan–Meier survival analysis and the log-rank test were used to evaluate the relationships between ESTIMATE scores, levels of DEGs and TIICs, and prognosis. Correlations between gene expression levels were evaluated using Spearman’s rank correlation coefficient. An absolute R value >0.1 was considered as a significant correlation. In all other analyses, P<0.05 was considered statistically significant.
Results
Clinical information and immune score distribution and DEGs associated with the TIME in ESCC and EAC
After excluding cases with missing data, 182 EC patients with complete clinicopathologic records in the TCGA database were included in the study. Clinicopathologic parameters extracted for analysis were sex and TNM stage. The 95 ESCC cases (52.2%) comprised 80 (84.2%) males and 15 (15.8%) females, and among the 87 EAC cases there were 75 (86.2%) males and 12 (13.8%) females. The higher representation of males among ESCC and EAC patients is in line with the global sex distribution of EC. Most patients had advanced-stage ESCC or EAC and had missed the optimal time window for surgical treatment (Table 1). Stromal scores in ESCC ranged from −1,713.78 to 1,694.52 and immune scores ranged from −1,066.89 to 2,000.92. In EAC, stromal scores ranged from −2,350.48 to 1,372.93 and immune scores ranged from −875.76 to 3,377.79. Median values of both scores differed significantly between the 2 EC subtypes (Figure 1A).
Table 1
Characteristics Number of case (%) | ESCC | EAC | |||
---|---|---|---|---|---|
n | % | n | % | ||
Gender | |||||
Female | 15 | (15.8) | 12 | (86.2) | |
Male | 80 | (84.2) | 75 | (13.8) | |
T stage | |||||
T1 | 8 | (8.4) | 23 | (26.4) | |
T2 | 32 | (33.7) | 11 | (12.6) | |
T3 | 49 | (51.6) | 37 | (42.5) | |
T4 | 4 | (4.2) | 1 | (1.1) | |
TX | 2 | (2.1) | 15 | (17.2) | |
N stage | |||||
N0 | 55 | (57.8) | 21 | (24.1) | |
N1 | 28 | (29.4) | 40 | (45.9) | |
N2 | 6 | (6.4) | 6 | (6.9) | |
N3 | 3 | (3.2) | 5 | (5.7) | |
NX | 3 | (3.2) | 15 | (17.2) | |
M stage | |||||
M0 | 83 | (87.4) | 51 | (58.6) | |
M1 | 4 | (4.2) | 5 | (5.7) | |
MX | 8 | (8.4) | 31 | (35.6) | |
TNM stage | |||||
I | 7 | (7.4) | 11 | (12.6) | |
II | 56 | (58.9) | 22 | (25.2) | |
III | 26 | (27.4) | 29 | (33.3) | |
IV | 4 | (4.2) | 5 | (5.7) | |
NR | 2 | (2.1) | 20 | (22.9) |
NR, not reported; TX, T stage not recognized; NX, N stage not recognized; MX, M stage not recognized.
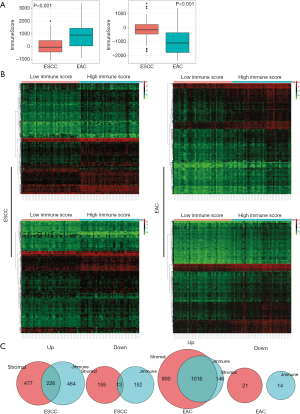
We evaluated the relationship between DEG expression levels and stromal/immune scores in ESCC and EAC using TCGA datasets. The heatmaps in Figure 1B show the distribution of gene expression profiles based on stromal/immune scores. Genes that were differentially expressed between high- and low-stromal/immune score groups were identified (Figure 1C). Of the immune-associated DEGs in ESCC, 690 genes were upregulated and 165 were downregulated. Of the stromal DEGs in ESCC, 703 genes were upregulated and 168 were downregulated (fold change >1.5, P<0.05). Among intersecting DEGs, 226 genes were upregulated and 13 were downregulated. Similar trends were observed in EAC: of the identified stromal DEGs, 1,916 genes were upregulated and 21 were downregulated; 1,162 immune-related genes were upregulated and 14 were downregulated (fold change >1.5, P<0.05); and among intersecting DEGs, 1016 genes were upregulated and no genes were downregulated.
Correlations between stromal/immune scores, clinicopathologic characteristics, and prognosis of ESCC and EAC
The cohort was divided into high- and low-score groups according to median stromal/immune scores, and the correlation between the scores and clinicopathologic characteristics was evaluated. The median stromal score in ESCC was correlated with sex (P=0.037) and T stage (P=0.022). In EAC, the immune score in females was higher than that in males (P=0.022), and stromal score was correlated with TNM stage (P=0.019). There were no significant correlations between the 2 scores and other characteristics (Figure 2A,B,C,D,E,F,G,H,I,J,K,L,M,N,O,P,Q,R,S,T), and the Kaplan–Meier survival analysis showed that neither score was correlated with overall survival (OS) in ESCC and EAC (Figure 2U,V,W,X).
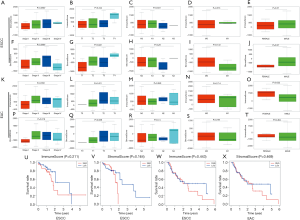
PPI network for DEGs in ESCC and EAC
The intersecting DEGs were used to construct a PPI network that revealed their interactions. The top 3 modules for ESCC were as follows (Figure 3A,B,C). Module 1 had 42 nodes connected via 676 edges; C-C motif chemokine receptor 1 (CCR1), CCR2, CCR5, Colony-stimulating factor 1 receptor (CSF1R), interleukin 10 receptor subunit α (IL10RA), Transmembrane immune signaling adaptor (TYROBP), and Toll-like receptor 8 (TLR8) were the most remarkable nodes as they had more connections with other members within the module. Module 2 consisted of 24 nodes connected via 78 edges, with Cluster of differentiation 53 (CD53), Immunoglobulin superfamily member 6 (IGSF6), Myeloid cell nuclear differentiation antigen (MNDA), Fc fragment of IgG receptor IIb (FCGR2B), and Membrane-spanning 4-domains A6A (MS4A6A) having the highest degree values. Module 3 was composed of 4 nodes [tumor necrosis factor receptor superfamily member 9 (TNFRSF9), TNFSF4, TNFSF8, and Inducible T cell costimulator (ICOS)] connected by 5 edges; these genes are known to play an important role in the TIME. The top 3 modules for EAC were as follows (Figure 3D,E,F). Module 1 had 13 nodes with 69 edges; all nodes were C-X-C and C-C motif chemokines, of which CCR5, CCR1, C-X-C motif chemokine ligand 10 (CXCL10), CXCL9, and CXCL12 were the most significant DEGs. Module 2 contained 8 nodes connected via 26 edges, and module 3 comprised 8 nodes with 26 edges. All nodes in modules 2 and 3 were related to immune responses.
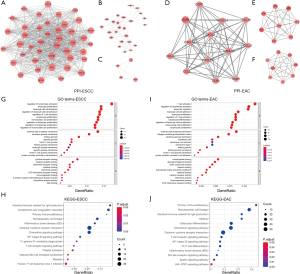
To clarify the functional significance of intersecting DEGs in ESCC, we carried out GO and KEGG pathway analyses. The most significant DEG-related BP, CC, and MF in ESCC were regulation of lymphocyte activation, external side of the plasma membrane, and cytokine receptor binding, respectively (Figure 3G). The KEGG pathway analysis showed that these DEGs in ESCC were enriched in the intestinal immune network for IgA production, complement and coagulation cascades, and primary immunodeficiency (Figure 3H). In EAC, the most significant DEG-related BP, CC, and MF were T cell activation, external side of the plasma membrane, and carbohydrate-binding activity, respectively (Figure 3I). These DEGs were enriched in the primary immunodeficiency, hematopoietic cell lineage, and intestinal immune network for IgA production KEGG pathways (Figure 3J).
Prognostic landscape of TIICs in ESCC and EAC
We evaluated correlations between the proportions of 22 types of immune cell and ESCC and EAC prognosis. The percentages of activated and resting natural killer (NK) cells, mast cells, and dendritic cells (DCs); neutrophils; monocytes; plasma cells; eosinophils; and macrophages (M0–M2) were associated with innate immunity; and the percentages of naïve CD4+ T cells, activated memory CD4+ T cells, resting memory CD4+ T cells, CD8+ T cells, gamma delta T cells, regulatory T (Treg) cells, T follicular helper (Tfh) cells, naïve B cells, and memory B cells were associated with adaptive immunity.
The gene expression profiles of tumor tissues in TCGA (48 tumor samples and 1 paracancerous sample of ESCC, and 27 tumor samples and 3 paracancerous samples of EAC) were imported into CIBERSORT software and the proportions of the 22 TIICs in the 2 EC subtypes were calculated (Figure 4). The heatmaps revealed weak-to-moderate associations among the 22 TIICs in ESCC and EAC (Figure 5A,B). Specifically, immune cells in ESCC with strong intercorrelations included memory and naïve B cells (0.64), Treg and Tfh cells (0.53), and resting memory CD4+ T cells and CD8+ T cells (−0.56); and those with significant intercorrelations in the TIME of EAC were resting mast cells and monocytes (0.64), activated memory CD4+ T cells and CD8+ T cells (0.61), and M0 macrophages and monocytes (−0.55). The violin plot of the relative proportions of TIICs in all samples and differences in TIIC distribution between paracancerous and cancerous tissues confirmed these results (Figure 5C,D and Figure 4). Possibly because of the limited sample size, we observed a lower proportion of resting NK cells in cancerous tissues than in paracancerous tissues in ESCC patients (P=0.049), whereas in cancerous tissues of EAC patients there was a lower proportion of monocytes (P=0.005) and higher proportion of M0 macrophages (P=0.032).
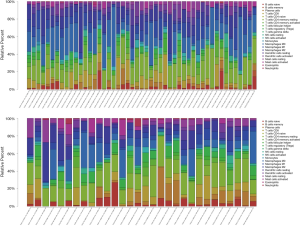
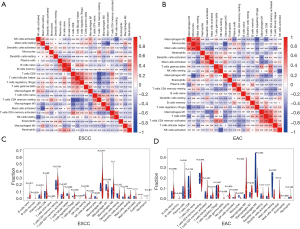
To determine the correlations between TIIC infiltration and prognosis in EC, ESCC and EAC patients were divided into the high- and low-infiltration groups according to the median proportion of TIICs. High levels of infiltrating resting DCs and CD8+ T cells predicted shorter OS in ESCC (P<0.05, Figure 6A,B), while high levels of infiltrating naïve B cells, activated mast cells, and resting memory CD4+ T cells predicted shorter OS in EAC (P<0.05, Figure 6C,D).
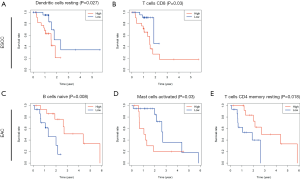
Intersecting DEGs predict the prognosis of ESCC and EAC
We assessed the relationships between intersecting DEGs and OS in ESCC and EAC patients by Kaplan–Meier survival analysis. In total, 37 in ESCC and 9 in EAC were correlated with OS (both P<0.05). Representative DEGs are shown in Figure 7, and prognostic DEGs in ESCC and EAC are summarized in Table 2.
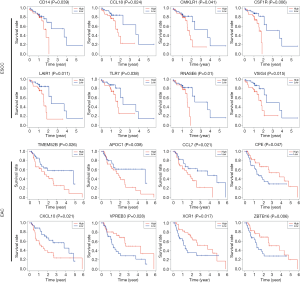
Table 2
Subtype of EC | Significant DEGs for overall survival |
---|---|
ESCC | ADAMDEC1, APBB1IP, APOC1, C1QA, C4B, CCL18, CD4, CD14, CD300A, CD300LF, CHST13, CMKLR1, CSF1R, CYBB, DNAJC5B, EVI2B, FBP1, FCGR3A, HLA-DPB1, IGSF6, LAIR1, LRRC25, MS4A4A, MS4A6, MYO1F, NCKAP1L, RNASE6, SLCO2B1, SOD3, STAB1, TLR7, TMIGD3, TNFAIP8L2, VSIG4, AXL, OTOP2, TMPRSS11E |
EAC | ANKRD1, APOC1, CCL7, CPE, CXCL10, TMEM52B (C12orf59), VPREB3, XCR1, ZBTB16 |
Abbreviations: EC, esophageal cancer; ESCC, esophageal squamous cell carcinoma; EAC, esophageal adenocarcinoma; DEGs, differentially expressed genes.
Validation of prognostic DEGs in GEO and GSEA
We assessed the prognostic value of the identified DEGs with regard to the OS of EAC patients using a GEO validation cohort (GSE19417). Two of the 9 genes—i.e., CXCL10 and TMEM52B (C12orf59)—were positively correlated with poor prognosis in EAC. Similarly, 5 of the 37 genes (CD14, CMKLR1, LAIR1, VSIG4, RNASE6) in ESCC dataset (GSE53625) also have the same prognostic value in the GEO database (Figure 8). GSEA analysis showed that the prognostic DEGs were enriched in allograft rejection, epithelial–mesenchymal transition, the interleukin 6 (IL-6)/Janus kinase (JAK)/signal transducer and activator of transcription (STAT3) signaling pathway, interferon α/γ response, myogenesis, angiogenesis, inflammatory response, and pancreas β cells (Figure 9).
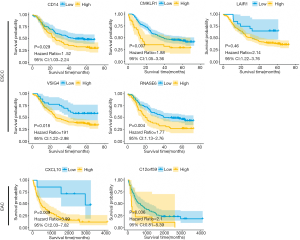
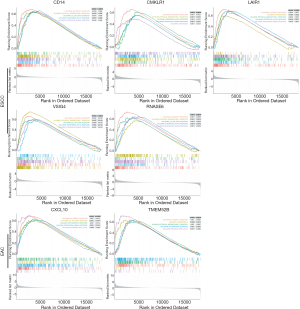
Discussion
ESCC and EAC differ in terms of genotype, phenotype, and therapeutic priorities. The effective management of EC requires a full understanding of the TIME of the 2 subtypes (32) and the interactions between TIICs and cancer cells in antitumor responses. In order to identify more specific and relevant prognostic biomarkers, we investigated the prognostic landscape of TIICs and prognostic DEGs in the TIME of ESCC and EAC by bioinformatics analysis.
We identified candidate prognostic DEGs in the TIME based on stromal/immune scores calculated from TCGA datasets. However, there was no significant correlation between ESTIMATE scores and OS in ESCC or EAC. This may be explained by the small sample sizes of the cohorts. The median immune score for ESCC was significantly lower whereas the stromal score was higher than the scores for EAC; this indicates that the TIME differs between the 2 pathologic subtypes of EC. In ESCC, the median stromal score was lower in females than in males and was correlated with T stage. In EAC, the median immune score was higher in females than in males and the stromal score was correlated with TNM stage. These findings are consistent with the functional implications of intersecting DEGs: GO analysis of the 239 and 1016 intersecting DEGs in ESCC and EAC, respectively, that were identified in this study revealed functions related to the activation of lymphocytes—especially T lymphocytes—and carbohydrate binding. The results of the KEGG pathway enrichment analysis indicated that it was possible to identify immune-related DEGs with precision based on ESTIMATE scores. Moreover, we defined multiple immune-related modules by cluster analysis of the PPI network. Within the top 3 modules in the network, CCR5 (33), TLR8 (34), and CXCL12 (35) were the most significant DEGs related to the survival, migration, and invasion of ESCC or EAC cells. It is worth noting that ICOS in the PPI network of ESCC has been shown to predict the prognosis of EAC patients (36).
We found 37 genes in ESCC and 9 in EAC with prognostic significance. Among the latter, 8 genes [Ankyrin repeat domain 1 (ANKRD1), APOC1, C-C motif chemokine ligand 7 (CCL7), Carboxypeptidase E (CPE), Transmembrane protein 52B (TMEM52B), V-set pre-B cell surrogate light chain 3 (VPREB3), X-C motif chemokine receptor 1 (XCR1), and Zinc finger and BTB domain-containing 16 (ZBTB16)] are potential biomarkers in EAC, although this has not yet been demonstrated. Validation of the 9 genes using a GEO cohort revealed that CXCL10 and TMEM52B (C12orf59) could predict OS of EAC patients. C12orf59 down-regulation is associated with poor prognosis in renal cell carcinoma, but C12orf59 was significantly up-regulated in gastric cancer cells and can promote cancer cell invasion and metastasis (37,38). On the other hand, downregulation of CXCL10 in EAC tissues has been linked to a more favorable outcome (39). In addition, overexpression of CCL18, a significant DEG in ESCC, was shown to predict a lower survival rate and contribute to the malignant progression of ESCC by increasing the expression of the long noncoding RNA HOX transcript antisense intergenic RNA (HOTAIR) (40). Transmembrane serine protease 11E (TMPRSS11E) reduces the chemoresistance of ESCC cells by activating the epidermal growth factor receptor/AKT signaling pathway (41). Importantly, 5 genes [CD14, chemerin chemokine-like receptor 1 (CMKLR1), leukocyte-associated immunoglobulin-like receptor 1 (LAIR1), V-set and immunoglobulin domain containing 4 (VSIG4), and ribonuclease A family member k6 (RNASE6)] have prognostic value in both TCGA and GEO databases, and none of these 5 genes have been reported before. In this study, we additionally observed enrichment of several pathways for the validated DEGs by GSEA including allograft rejection, epithelial–mesenchymal transition, IL-6/JAK/STAT3 signaling pathway, interferon alpha/gamma response, myogenesis, angiogenesis, inflammatory response, and pancreas beta cells. These pathways determined by GSEA need to be further validated in vitro and in vivo.
We compared the prognostic landscapes of TIICs between ESCC or EAC and normal tissues using EC gene expression profiles in the TCGA database. Our results indicate that selected TIICs can predict the prognosis of ESCC and EAC patients. Previous studies have reported that NK cell-based immunotherapy had a nonsignificant therapeutic effect in acute myeloid leukemia but was well tolerated in solid cancers including ESCC (42). M1 macrophages are involved in proinflammatory responses and antitumor immunity, while M2 macrophages promote cancer progression (43); the M2/M1 ratio has been used as an indicator of EAC lymph node metastasis and poor prognosis in patients who do not receive neoadjuvant therapy (44). Monocytes are known to accelerate tumor growth and enhance antitumor immunity (45); however, there are few studies on their role in EC, and further research on TIICs in EC will help provide a more effective treatment strategy. We identified several prognostic TIICs in ESCC and EAC in the present study including resting DCs, CD8+ T cells, naïve B cells, activated mast cells, and resting memory CD4+ T cells. DCs present tumor cell antigens to CD8+ T cells, thereby stimulating the antitumor function of cytotoxic T lymphocytes (46). We found that high infiltrating CD8+ T cell levels predicted poor prognosis in ESCC, which is in disagreement with previous findings in other tumors. High mast cell density has been linked to ESCC progression and lower postoperative survival rate (47). Our results showed that the proportion of mast cells was negatively correlated with OS of EAC patients, indicating that mast cell infiltration predicts an unfavorable prognosis in EAC. Cancer cells promote tumor growth, invasiveness, and treatment resistance by regulating the expression and production of IL-22 in memory CD4+ T cells in an IL-1–dependent manner (48). Thus, TIICs have diverse roles in the development of EC and other cancers, although the therapeutic effect achieved by targeting components of the TIME in EC remains to be determined.
There were some limitations to the present study. Firstly, the results may have been influenced by the small sample size. Additionally, our conclusions are based on in silico analyses of datasets comprising gene and other molecular information from patient-derived tissues, and require validation in clinical studies. Nonetheless, our study identified 5 DEGs in ESCC and 9 in EAC, which showed consistent prognostic value in multiple database. The functional relevance of these genes was demonstrated by the enrichment of specific pathways and prognostic landscape of selected TIICs. These findings provide new insight into the importance of the TIME in EC as well as a set of potential biomarkers that can be used for diagnosis and treatment response monitoring.
Acknowledgments
We sincerely thank the data provided by TCGA and GEO databases, and thank all the staff of thoracic surgery for their support during the study.
Funding: This work was supported by
Footnote
Reporting Checklist: The authors have completed the MDAR checklist. Available at http://dx.doi.org/10.21037/tcr-20-3078
Peer Review File: Available at http://dx.doi.org/10.21037/tcr-20-3078
Conflicts of Interest: All authors have completed the ICMJE uniform disclosure form (available at http://dx.doi.org/10.21037/tcr-20-3078). The authors have no conflicts of interest to declare.
Ethical Statement: The authors are accountable for all aspects of the work in ensuring that questions related to the accuracy or integrity of any part of the work are appropriately investigated and resolved. The study was conducted in accordance with the Declaration of Helsinki (as revised in 2013). All data in this study come from public data in the TCGA and GEO databases, and therefore ethics approval was waived.
Open Access Statement: This is an Open Access article distributed in accordance with the Creative Commons Attribution-NonCommercial-NoDerivs 4.0 International License (CC BY-NC-ND 4.0), which permits the non-commercial replication and distribution of the article with the strict proviso that no changes or edits are made and the original work is properly cited (including links to both the formal publication through the relevant DOI and the license). See: https://creativecommons.org/licenses/by-nc-nd/4.0/.
References
- Gupta B, Kumar N. Worldwide incidence, mortality and time trends for cancer of the oesophagus. Eur J Cancer Prev 2017;26:107-18. [Crossref] [PubMed]
- Rustgi AK, El-Serag HB. Esophageal carcinoma. N Engl J Med 2014;371:2499-509. [Crossref] [PubMed]
- Siegel RL, Miller KD, Jemal A. Cancer statistics, 2018. CA Cancer J Clin 2018;68:7-30. [Crossref] [PubMed]
- Chen W, Zheng R, Baade PD, et al. Cancer statistics in China, 2015. CA Cancer J Clin 2016;66:115-32. [Crossref] [PubMed]
- Pennathur A, Gibson MK, Jobe BA, et al. Oesophageal carcinoma. Lancet 2013;381:400-12. [Crossref] [PubMed]
- Siewert JR, Ott K. Are squamous and adenocarcinomas of the esophagus the same disease? Semin Radiat Oncol 2007;17:38-44. [Crossref] [PubMed]
- D'Journo XB, Thomas PA. Current management of esophageal cancer. J Thorac Dis 2014;6:S253-64. [PubMed]
- Malhotra U, Zaidi AH, Kosovec JE, et al. Prognostic value and targeted inhibition of survivin expression in esophageal adenocarcinoma and cancer-adjacent squamous epithelium. PLoS One 2013;8:e78343. [Crossref] [PubMed]
- Grivennikov SI, Greten FR, Karin M. Immunity, inflammation, and cancer. Cell 2010;140:883-99. [Crossref] [PubMed]
- Babar L, Kosovec JE, Jahangiri V, et al. Prognostic immune markers for recurrence and survival in locally advanced esophageal adenocarcinoma. Oncotarget 2019;10:4546-55. [Crossref] [PubMed]
- Li Y, Lu Z, Che Y, et al. Immune signature profiling identified predictive and prognostic factors for esophageal squamous cell carcinoma. Oncoimmunology 2017;6:e1356147. [Crossref] [PubMed]
- Noble F, Mellows T, McCormick Matthews LH, et al. Tumour infiltrating lymphocytes correlate with improved survival in patients with oesophageal adenocarcinoma. Cancer Immunol Immunother 2016;65:651-62. [Crossref] [PubMed]
- Coussens LM, Werb Z. Inflammation and cancer. Nature 2002;420:860-7. [Crossref] [PubMed]
- Maccalli C, Giannarelli D, Capocefalo F, et al. Immunological markers and clinical outcome of advanced melanoma patients receiving ipilimumab plus fotemustine in the NIBIT-M1 study. Oncoimmunology 2015;5:e1071007. [Crossref] [PubMed]
- Hanahan D, Coussens LM. Accessories to the crime: functions of cells recruited to the tumor microenvironment. Cancer Cell 2012;21:309-22. [Crossref] [PubMed]
- Gregorio AC, Lacerda M, Figueiredo P, et al. Therapeutic Implications of the Molecular and Immune Landscape of Triple-Negative Breast Cancer. Pathol Oncol Res 2018;24:701-16. [Crossref] [PubMed]
- Kang HJ, Oh JH, Chun SM, et al. Immunogenomic landscape of hepatocellular carcinoma with immune cell stroma and EBV-positive tumor-infiltrating lymphocytes. J Hepatol 2019;71:91-103. [Crossref] [PubMed]
- Mlecnik B, Van den Eynde M, Bindea G, et al. Comprehensive Intrametastatic Immune Quantification and Major Impact of Immunoscore on Survival. J Natl Cancer Inst 2018;110. [Crossref] [PubMed]
- Zhou R, Zhang J, Zeng D, et al. Immune cell infiltration as a biomarker for the diagnosis and prognosis of stage I-III colon cancer. Cancer Immunol Immunother 2019;68:433-42. [Crossref] [PubMed]
- Yoshihara K, Shahmoradgoli M, Martinez E, et al. Inferring tumour purity and stromal and immune cell admixture from expression data. Nat Commun 2013;4:2612. [Crossref] [PubMed]
- Yan H, Qu J, Cao W, et al. Identification of prognostic genes in the acute myeloid leukemia immune microenvironment based on TCGA data analysis. Cancer Immunol Immunother 2019;68:1971-8. [Crossref] [PubMed]
- Jia D, Li S, Li D, et al. Mining TCGA database for genes of prognostic value in glioblastoma microenvironment. Aging (Albany NY) 2018;10:592-605. [Crossref] [PubMed]
- Alonso MH, Ausso S, Lopez-Doriga A, et al. Comprehensive analysis of copy number aberrations in microsatellite stable colon cancer in view of stromal component. Br J Cancer 2017;117:421-31. [Crossref] [PubMed]
- Shah N, Wang P, Wongvipat J, et al. Regulation of the glucocorticoid receptor via a BET-dependent enhancer drives antiandrogen resistance in prostate cancer. Elife 2017;6:e27861. [Crossref] [PubMed]
- Priedigkeit N, Watters RJ, Lucas PC, et al. Exome-capture RNA sequencing of decade-old breast cancers and matched decalcified bone metastases. JCI Insight 2017;2:e95703. [Crossref] [PubMed]
- Qu Y, Cheng B, Shao N, et al. Prognostic value of immune-related genes in the tumor microenvironment of lung adenocarcinoma and lung squamous cell carcinoma. Aging (Albany NY) 2020;12:4757-77. [Crossref] [PubMed]
- Liu Y, Wang L, Liu H, et al. The Prognostic Significance of Metabolic Syndrome and a Related Six-lncRNA Signature in Esophageal Squamous Cell Carcinoma. Front Oncol 2020;10:61. [Crossref] [PubMed]
- Yuan C, Xiang L, Cao K, et al. The prognostic value of tumor mutational burden and immune cell infiltration in esophageal cancer patients with or without radiotherapy. Aging (Albany NY) 2020;12:4603-16. [Crossref] [PubMed]
- Szklarczyk D, Gable AL, Lyon D, et al. STRING v11: protein-protein association networks with increased coverage, supporting functional discovery in genome-wide experimental datasets. Nucleic Acids Res 2019;47:D607-13. [Crossref] [PubMed]
- Newman AM, Liu CL, Green MR, et al. Robust enumeration of cell subsets from tissue expression profiles. Nat Methods 2015;12:453-7. [Crossref] [PubMed]
- Subramanian A, Tamayo P, Mootha VK, et al. Gene set enrichment analysis: a knowledge-based approach for interpreting genome-wide expression profiles. Proc Natl Acad Sci U S A 2005;102:15545-50. [Crossref] [PubMed]
- Lin EW, Karakasheva TA, Hicks PD, et al. The tumor microenvironment in esophageal cancer. Oncogene 2016;35:5337-49. [Crossref] [PubMed]
- Kodama T, Koma YI, Arai N, et al. CCL3-CCR5 axis contributes to progression of esophageal squamous cell carcinoma by promoting cell migration and invasion via Akt and ERK pathways. Lab Invest 2020;100:1140-57. [Crossref] [PubMed]
- Helminen O, Huhta H, Lehenkari PP, et al. Nucleic acid-sensing toll-like receptors 3, 7 and 8 in esophageal epithelium, barrett's esophagus, dysplasia and adenocarcinoma. Oncoimmunology 2016;5:e1127495. [Crossref] [PubMed]
- Wang X, Cao Y, Zhang S, et al. Stem cell autocrine CXCL12/CXCR4 stimulates invasion and metastasis of esophageal cancer. Oncotarget 2017;8:36149-60. [Crossref] [PubMed]
- Humphries MP, Craig SG, Kacprzyk R, et al. The adaptive immune and immune checkpoint landscape of neoadjuvant treated esophageal adenocarcinoma using digital pathology quantitation. BMC Cancer 2020;20:500. [Crossref] [PubMed]
- Xie J, Zhu C, Wu J, et al. Down-regulation of C12orf59 is associated with a poor prognosis and VHL mutations in renal cell carcinoma. Oncotarget 2016;7:6824-34. [Crossref] [PubMed]
- Zhang JX, He WL, Feng ZH, et al. A positive feedback loop consisting of C12orf59/NF-kappaB/CDH11 promotes gastric cancer invasion and metastasis. J Exp Clin Cancer Res 2019;38:164. [Crossref] [PubMed]
- Blank S, Nienhüser H, Dreikhausen L, et al. Inflammatory cytokines are associated with response and prognosis in patients with esophageal cancer. Oncotarget 2017;8:47518-32. [Crossref] [PubMed]
- Wang W, Wu D, He X, et al. CCL18-induced HOTAIR upregulation promotes malignant progression in esophageal squamous cell carcinoma through the miR-130a-5p-ZEB1 axis. Cancer Lett 2019;460:18-28. [Crossref] [PubMed]
- Chang ZW, Jia YX, Zhang WJ, et al. LncRNA-TUSC7/miR-224 affected chemotherapy resistance of esophageal squamous cell carcinoma by competitively regulating DESC1. J Exp Clin Cancer Res 2018;37:56. [Crossref] [PubMed]
- Lim KS, Mimura K, Kua LF, et al. Implication of Highly Cytotoxic Natural Killer Cells for Esophageal Squamous Cell Carcinoma Treatment. J Immunother 2018;41:261-73. [Crossref] [PubMed]
- Chanmee T, Ontong P, Konno K, et al. Tumor-associated macrophages as major players in the tumor microenvironment. Cancers (Basel) 2014;6:1670-90. [Crossref] [PubMed]
- Cao W, Peters JH, Nieman D, et al. Macrophage subtype predicts lymph node metastasis in oesophageal adenocarcinoma and promotes cancer cell invasion in vitro. Br J Cancer 2015;113:738-46. [Crossref] [PubMed]
- Olingy CE, Dinh HQ, Hedrick CC. Monocyte heterogeneity and functions in cancer. J Leukoc Biol 2019;106:309-22. [Crossref] [PubMed]
- Gardner A, Ruffell B. Dendritic Cells and Cancer Immunity. Trends Immunol 2016;37:855-65. [Crossref] [PubMed]
- Fakhrjou A, Niroumand-Oscoei SM, Somi MH, et al. Prognostic value of tumor-infiltrating mast cells in outcome of patients with esophagus squamous cell carcinoma. J Gastrointest Cancer 2014;45:48-53. [Crossref] [PubMed]
- Voigt C, May P, Gottschlich A, et al. Cancer cells induce interleukin-22 production from memory CD4(+) T cells via interleukin-1 to promote tumor growth. Proc Natl Acad Sci U S A 2017;114:12994-9. [Crossref] [PubMed]