A visual model for prognostic estimation in patients with primary diffuse large B-cell lymphoma of small intestine and colon: analysis of 1,613 cases from the SEER database
Introduction
Diffuse large B-cell lymphoma (DLBCL) is a malignant disease and the most common subtype of non-Hodgkin’s lymphoma (NHL), with an incidence rate of 4.92–7.00/100,000, and nearly one-third of NHL are DLBCL that can spread around the body (1). About 40% of DLBCL patients’ primary site are gastrointestinal, and their symptoms are nonspecific. Patients often go to hospital for complications such as intestinal bleeding, intestinal obstruction (2). As we known, DLBCL is a heterogeneous disease with a wide range of prognoses among individuals. Prognosis estimation are particularly important for health care providers and the families of the patients to make decisions about treatment, so it has long been a hotbed of research. Much attention is currently focused on the routine types of DLBCL and gastric involvement, with few studies of the primary DLBCL of intestinal and colon (PIC-DLBCL).
Currently, PIC-DLBCL prognosis estimation is based mainly on the International Prognostic Index scoring model (IPI) constructed in 1977 and related improved versions such as R-IPI, NCCN-IPI, etc. They all use low or high-risk groups as a predictive outcome and only focus on medical data ignoring the influence of families and social support factors. With increasing concern about prognosis, individualized prognostic models based on big data seem to be more appropriate. The anti-CD20 Rituximab combined with CHOP has emerged as the mainstay of treatment for PIC-DLBCL in the past two decades (3), which has led to a significant improvement in 5-year Overall Survival (OS) (54–88%) (4). Due to survive longer than before, family, social support and medical resources had more weight on the prognosis (5).
To provide a better individualized prognosis estimation of PIC-DLBCL, we performed a retrospective analysis and constructed the predictive model, including marital status, type of insurance and clinical medical data. The data were based on patients diagnosed with PIC-DLBCL between 2002–2016 in the SEER database from the National Cancer Institute of the United States, which collects and publishes cancer incidence, treatment, and survival data from population based cancer registries, representing approximately 28% of the US population (6). We present the following article in accordance with the TRIPOD reporting checklist (available at: http://dx.doi.org/10.21037/tcr-20-3086).
Methods
Excluded the incomplete-data cases, all patients with PIC-DLBCL between 2002 and 2016 from the SEER database were included in this study. The endpoint of this study was patient death from blood-related diseases. The survival-related covariates included Sex, Race, Marital status, Medical insurance, Age at diagnosis, Primary site (Colon, Cecum and Appendix, Small Intestinal), Type of histology, Ann Arbo stage, First primary malignancy (No previous history of malignant disease), Systemic B symptoms and Surgical treatment. Patients whose data contained IPI scores were included in the validation cohort for testing the performance of the prognostic model constructed by this study, and others were assigned to the training cohort in order to analyse the survival and build up the model. Since the data from the SEER registry were deidentified and publicly available, no IRB approval was necessary.
The above data were extracted using SEER*Stat software (version 8.3.6) from the newest available SEER database (1975–2016), patients with PIC-DLBCL in this study were identified using the WHO-2008 Lymphoma Subtype Classification, and anatomically located in the small intestine (ICD-O-3 code: C17) and colon (ICD-O-3 code: C18). The study was conducted in accordance with the Declaration of Helsinki (as revised in 2013). Since the data from the SEER registry were de-identified and publicly available, no institutional review board approval was necessary and no informed consent was signed for this study.
Statistical analysis
Statistical analysis was performed using R 3.6.2 software. Overall survival was identified as time from diagnosis until death. Cause specific survival was defined as time from diagnosis until death due to PIC-DLBCL. In the initial, Kaplan-Meier (K-M) survival curves were plotted and the Log-rank test was used to find the statistical significance by Survival package (version 3.1-8). For the training cohort, Cox proportional-hazards univariate regression was used to find out the significant covariates in the survival analysis, and checked by Proportional-Hazards (PH) assumption using Schoenfeld residuals (7). If the p-value of Schoenfeld residuals is greater than 0.05, it means that the variable conforms to PH assumption and can be included in the Cox regression analysis. Then, these significant prognostic covariates were considered for the final Cox proportional-hazards multivariable regression models. The prediction model was constructed and converted to the visual form. The nomogram was built by RMS Package (version 5.1-4) (8) and the graphical web page was by shiny Package (version 1.4.0.2). Predicted survival rates at different times could be obtained from the model by cross-referencing or filling in the relevant information.
The consistency of the model was verified by internal resampling calibration plot. The receiver operating characteristic (ROC) curves were plotted using survival ROC Package (version 1.0.3) to clarify the high and low risk group cutoff values of the model, then Log-rank test served to verify the statistical difference between the groups, in order to further validate the discriminatory ability of the model.
Decision curve analysis (DCA) has been increasingly used to estimate the validity of models in clinical studies (9). The advantage of DCA over ROC is that it introduces the concept of net benefit, allowing the threshold probability to change to check whether one model is superior to another within a particular range of threshold probabilities, which is highly prized (10). In the validation cohort, the actual survival and 5-year survival probabilities predicted by IPI was known, so both DCA and ROC curves were utilized for comparing the predictive power of our prognostic model with IPI.
Results
Input data from SEER and patient characteristics
From 2002 to 2016, a total of 1,994 PIC-DLBCL patients were registered in the SEER database. Of those, 381 cases were excluded due to incomplete information and 1,613 cases were included followed for 1–150 months, with a median follow-up of 37 months. The patient characteristics were presented in Table 1. The included cases contained 1,031 (63.9%) males and 582 (36.1%) females. 1,155 (71.6%) cases underwent surgery during treatment. The great majority of patients (1,151, 71.4%) were non-Hispanic whites, 159 cases (9.9%) were non-Hispanic yellow, and 303 (18.7%) were other races. The principal primary site was Small intestine (923, 57.2%), followed by Colon (350, 21.7%), Cecum and Appendix (340, 21.0%). Among patients with Ann Arbor stage, 590 cases (36.6%) were stage I, and 553 (34.3%), 111 (6.9%), 359 (22.2%) for stages II, III, IV. In total, 164 cases with IPI score were included in the validation cohort and the other 1449 cases were included in the training cohort.
Table 1
Factor | Value | Group | Frequency | Proportion | Chisq | P valuef |
---|---|---|---|---|---|---|
Age | 1 | 0–20 | 38 | 2.4% | 349.835 | <0.001 |
2 | 21–40 | 108 | 6.7% | |||
3 | 41–60 | 437 | 27.1% | |||
4 | 61–80 | 738 | 45.8% | |||
5 | ≥81 | 292 | 18.1% | |||
Sex | 1 | Male | 1,031 | 63.9% | 0.811 | 0.368 |
2 | Female | 582 | 36.1% | |||
Maritala | 1 | Married (including common law) | 966 | 59.9% | 3.977 | 0.137 |
2 | Others | 513 | 31.8% | |||
3 | Divorced or Separated | 134 | 8.3% | |||
Insuranceb | 1 | Insured | 891 | 55.2% | 25.608 | <0.001 |
2 | Basic Medicaid | 321 | 19.9% | |||
3 | Others | 401 | 24.9% | |||
Race | 1 | Hispanic | 210 | 13.0% | 3.794 | 0.435 |
2 | Non-His Indian | 8 | 0.5% | |||
3 | Non-His Asian | 159 | 9.9% | |||
4 | Non-His Black | 85 | 5.3% | |||
5 | Non-His White | 1,151 | 71.4% | |||
Site | 1 | Cecum and Appendix | 340 | 21.1% | 14.88 | 0.001 |
2 | Small Intestinal | 923 | 57.2% | |||
3 | Colon | 350 | 21.7% | |||
Histologic | 1 | Diffuse large B-cell lymphoma | 1,592 | 98.7% | 2.822 | 0.420 |
2 | Large B-cell, diffuse, immunoblastic | 9 | 0.6% | |||
3 | T cell/histiocyte rich large B-cell | 1 | 0.1% | |||
4 | Plasmablastic | 11 | 0.7% | |||
Ann Arbor | 1 | Stage I | 590 | 36.6% | 76.418 | <0.001 |
2 | Stage II | 553 | 34.3% | |||
3 | Stage III | 111 | 6.9% | |||
4 | Stage IV | 359 | 22.3% | |||
First malignantc | 1 | No | 309 | 19.2% | 27.352 | <0.001 |
2 | Yes | 1,304 | 80.8% | |||
Body symptomd | 1 | No | 1,167 | 72.3% | 4.871 | 0.027 |
2 | Yes | 446 | 27.7% | |||
Surgerye | 1 | No | 458 | 28.4% | 5.075 | 0.024 |
2 | Yes | 1,155 | 71.6% |
aMarital was categorized as (1) Married, presenting as having a legal spouse in law; (2) Other, presenting as single (never married), unmarried, domestic partner and widowed; (3) Divorced and separated, presenting as living apart from your spouse no matter whether getting the judgment of divorce in law. bInsurance was categorized as (1) Insured, presenting as private insurance, Medicare-administered through a Managed Care plan, Medicare with private supplement, Medicare with the supplement; (2) Basic Medicaid, presenting as Indian/Public Health Service, Medicaid-administered through a Managed Care plan, Medicare with Medicaid eligibility, and no specific insured; (3) Blank, presenting as uninsured, Insurance status unknown and blank. cFirst Malignant was categorized as (1) Yes, presenting as that PIC-DLBCL was the first malignant tumor; (2) No, presenting as that PIC-DLBCL was not the first malignant tumor. dBody symptom was categorized as (1) Yes, presenting as any B symptom, such as night sweats, unexplained fever (above 38 °C), unexplained weight loss (generally greater than 10% of body weight in the six months before admission), and pruritus (recurrent and unexplained); (2) No, presenting as no B symptoms (asymptomatic). eSurgery was categorized as (1) Yes, presenting as having surgical treatment during the PIC-DLBCL; (2) No, presenting as not having surgical treatment during the PIC-DLBCL. fP value was the result of Log-rank test between different subgroups.
Clinical prognosis outcomes
Analysis of the information in the SEER database revealed that the 5-year overall survival (OS) of all cases was 64.5% (Figure 1A). Age at diagnosis, medical insurance, primary site, Ann Arbo stage, first primary malignancy, systemic B symptoms and surgical treatment had statistical differences between subgroups by Log-rank test (Table 1). Prognosis got significantly worse with Age, Ann Arbor stage and Second malignancy (vs. first primary malignancy, used to describe that PIC-DLBCL occurs in a person who has had another cancer in the past). The 5-year OS was less than 50% if the patient’s age was older than 80, Ann Arbor stage was IV or was diagnosed with other malignant disease before PIC-DLBCL (Figure 1B,C,D). The K-M curve showed that married patients had the longer survival time compared with others (divorced, separated, single and so on) but no statistical difference (Figure 1E). Being insured could improve the prognosis statistically (Figure 1F). About Primary site, the prognosis of colon was the worst, followed by Small intestine, and Cecum and Appendix (Figure 1G). Systemic B symptoms and Non-surgical treatment might also make the prognosis worse with statistical differences, but survival was above 50% in all subgroups within 5 years (Figure 1H,I). Other covariates with no significant difference of survival was Sex, Race and Type of histology.
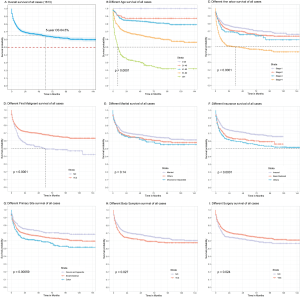
Univariate and multivariable analysis in the training cohort
Cox proportional-hazards univariate analysis identified Age at diagnosis, Ann Arbo, Marital status, Medical insurance, Primary site and First primary malignancy were associated with survival in the training cohort statistically. At the same time, although the overall trend of K-M survival curves stratification was obvious, there was still little crossover, for which Schoenfeld residuals was used to clarify the covariates met PH assumption, and the results show that all but First primary malignancy (P=0.002) met the assumption (Table 2).
Table 2
Factor | Coef | Hazard Ratio | 95% CI: | P value | P value of PH-test | ||
---|---|---|---|---|---|---|---|
Univariate analysis | Age | 0.924 | 2.52 | 2.23–2.84 | <0.001 | 0.915 | |
Sex | 0.09 | 1.09 | 0.92–1.31 | 0.318 | 0.227 | ||
Marital | 0.143 | 1.15 | 1.01–1.31 | 0.030 | 0.925 | ||
Insurance | 0.220 | 1.25 | 1.13–1.38 | <0.001 | 0.361 | ||
Race | 0.017 | 1.02 | 0.96–1.08 | 0.59 | 0.146 | ||
Site | 0.219 | 1.24 | 1.09–1.42 | 0.001 | 0.856 | ||
Histologic | 0.120 | 1.13 | 0.83–1.53 | 0.443 | 0.066 | ||
Ann Arbor | 0.294 | 1.34 | 1.25–1.44 | <0.001 | 0.092 | ||
First malignant | −0.494 | 0.61 | 0.50–0.74 | <0.001 | 0.002 | ||
Body symptom | 0.155 | 1.17 | 0.97–1.41 | 0.106 | 0.165 | ||
Surgery | −0.164 | 0.85 | 0.70–1.02 | 0.086 | 0.807 | ||
Multivariable analysisa | Age | 0.948 | 2.58 | 2.29–2.91 | <0.001 | 0.850 | |
Marital | 0.190 | 1.21 | 1.06–1.38 | 0.004 | 0.738 | ||
Insurance | 0.275 | 1.32 | 1.19–1.45 | <0.001 | 0.528 | ||
Site | 0.205 | 1.23 | 1.08–1.40 | 0.002 | 0.584 | ||
Ann Arbor | 0.290 | 1.34 | 1.24–1.44 | <0.001 | 0.170 |
aGlobal P value of Schoenfeld residuals was 0.750, including the whole covariates of Cox proportional-hazards multivariate regression. It was showed that the covariates were met Proportional-Hazards (PH) assumption when combined. In forest plots, covariates were labeled as purple lozenges if they were statistically different in the analysis, and green squares if they were not.
On Cox proportional-hazards multivariate regression, the results were consistent with that of univariate analysis and were same of forward, backward, and stepwise selections. The covariates still were significantly correlated with prognosis when combined and were all met PH assumption using Schoenfeld residuals (Global P value=0.750). Interestingly, the Age at diagnosis (HR=2.49, 95% CI: 2.21–2.81) had a higher risk of death compared with others (Table 2).
Prognostic model building, visualization and validation
Through the results of multivariate analysis, these associated risk covariates were used to build the nomogram of the model (Figure 2). To further facilitate the use of the model, this study made the model into a graphical web page where patients' survival could be predicted by entering relevant information (https://doctorwangyang.shinyapps.io/PIC-DLBCL/).
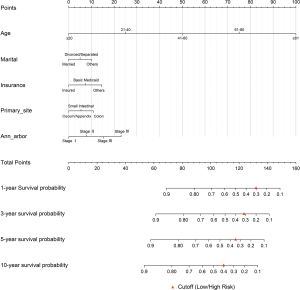
The model was internally validated using the 1000-times bootstrap validation method. The nomogram, which was set to predict OS for 1, 3, 5, 10 years, demonstrated good accuracy in estimating the survival rate. In addition, calibration plots graphically showed a good agreement on the survival rate of PIC-DLBCL between the estimation by the nomogram and actual survival (Figure 3).
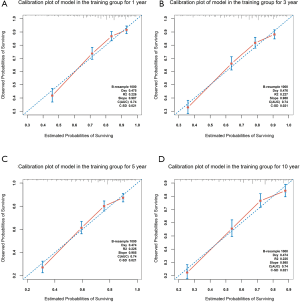
Through the actual survival of the training cohort, the C-indexes of the model were 0.776 (1-year), 0.775 (3-year), 0.787 (5-year), and 0.785 (10-year), and the high and low risk Cutoff values of the model were 0.300 (1-year), 0.313 (3-year), 0.355 (5-year), and 0.407 (10-year), which were calculated by ROC (Figure 4A). For ease of use, red triangles were labeled as Cutoff in the nomogram (Figure 2). To test the model's ability to distinguish between high and low risk patients, the model estimates for the training cohort was calculated, and divided the high and low risk groups by the Cutoffs. Then the K-M curve was plotted and did Log-rank test. The results showed significant differences (P<0.001) between the groups in the actual survival (Figure 4B,C,D,E). In the case of 5-year, the 5-year OS was only 39.1% for the high-risk group and 82.0% for the low risk group (Figure 4D).
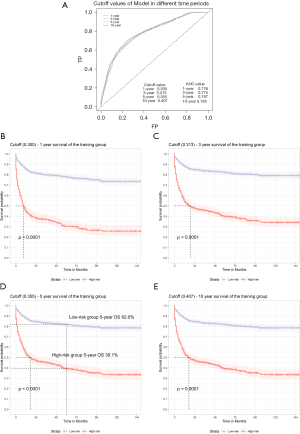
Comparison of the model and IPI in the validation cohort
The prognostic model and IPI survival estimate for the validation cohort were calculated, and ROC curves were plotted combined with actual survival. As a result, the C-index of the prediction model is better than IPI with a difference of 0.16 (AUC 0.820 vs. 0.714) in 5-year (Figure 5A). The DCA curve also showed a higher net benefit to the prognostic model near the Cutoff value, suggesting that its prediction accuracy and combined prediction benefit are better than IPI (Figure 5B).
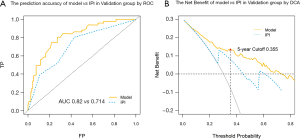
Discussion
With the development of medicine. There is an increase in individual prognosis estimation for both doctors and patients. The accuracy and reliability of the prognostic model directly affect the decisions on treatment. Sehn et al. (11) used information from over 10,000 patients to revise and refine the IPI and named the model R-IPI. It was a relatively more accurate and appropriate model for prognosis estimation under the new chemotherapy regimens. In 2014, National Comprehensive Cancer Network Center analyzed data from 1,650 DLBCL patients treated with R-CHOP, improved IPI and published NCCN-IPI. This model could better distinguish low/high risk patients (4). Despite the theoretical advantages of these models, IPI is recognized for its accuracy and still widely used, and there was no huge differences between the three models (4). Another study showed that nowadays, the clinical predictive power of the IPI scoring model remained high (12). Therefore, it was reasonable to compare our model with IPI.
It should be noted that the prognostic estimates of the IPI-related models are in the form of high/low risk groups and cannot accurately predict. This may have depleted the predictive power to some extent. With the development and updating of software packages related to nomogram and graphical web page, more and more scholars had been choosing to transform complex mathematical models into the visual form that could output accurate individual predictions. It had also been shown in many studies that the nomogram significantly improved the accuracy of prognostic models compared with group prognosis predictions (13,14). Due to heterogeneity and individual prognostic variation, prognostic estimation of PIC-DLBCL is important for patients. Therefore, this study translated the model into the visual form. At the same time, the results indicated that the model had better prognostic estimation capabilities than IPI, it meant that anyone could use the model for prognostic estimation about PIC-DLBCL, quickly, simply and correctly.
One important finding in this study was that marital and medical insurance status were related to prognosis. PIC-DLBCL prognostic studies (15,16) had focused on medical indicators in recent years. They treated the patient's prognosis as a disease process, ignoring the influence of families and social support, but it did play an important role in the prognosis of PIC-DLBCL. Therefore, marital status and medical insurance, were included in this study and the results showed that the prognostic model could outperform IPI without laboratory-related indicators. This illustrated the importance of family and social support in the prognosis of PIC-DLBCL, on the other hand, this study provided a new way to explore the better prognostic models.
Marital status could directly affect the prognosis of patients with PIC-DLBCL, which was consistent with current research. Quast et al. (5) study indicated that the prognosis for patients with tumors was directly related to families, with the effect increasing with time. Malpert et al. (17) also found that family support was essential for long-term survival of hematologic malignancy. The most important families are patient's mate, and it was important to emphasize that patients with tumors faced a higher risk of marital breakdown after diagnosis, particularly in male NHL patients up to 20% (18). It well knows that PIC-DLBCL is prevalent in middle-aged men. Changes in marital status during the treatment of PIC-DLBCL would inevitably have a major impact on the psychological state and social support, then this might affect survival time. So, there was a theoretical correlation between marital status and survival. In this study, married patients had a better prognosis than divorced patients. Log-rank test P-value of all cases was 0.137 about marital status, but this was statistically significant in regression analysis. This might be related to the inability of the data to reflect whether the patient's divorce occurred after the diagnosis of PIC-DLBCL.
Medical insurance can also affect patient prognosis. The poorest counties had a 19% higher mortality rate than the wealthiest counties (192.2 vs. 161.9/100,000) by surveying counties with large economic disparities in Ohio, USA (19). Poor people without health insurance were less likely to receive timely screening, reasonable cancer treatment and care, which led to delayed diagnosis and a lower chance of longer survival. Ward et al. (20) study also supported these findings, with a 13% increase in cancer mortality among male residents of poor counties compared with affluent counties. Therefore, it was necessary to include medical insurance in the prognostic model.
Our study showed that age was the greatest prognostic factor in PIC-DLBCL patients. Klapper et al. (21) found that the prognostic effect of genetic molecular marker would lose statistical significance after including age in multivariate analysis by analyzing 364 patients.
Ann Arbor staging and Primary site were also significantly associated with DLBCL prognosis (22). As a long-term widely used clinical staging method for NHL, Ann Arbor staging was easily got and accessible, and its predictive efficacy was comparable to that of the Lugano staging in prognostic estimation (23), so it was reasonable to use Ann Arbor staging as a predictor in this study. Patients whose Primary site was the colon have the poorest prognosis, which was the same as the study by Sharma et al. (24). When the colon was involved, clinical symptoms appeared later and were more non-specific, these could lead to delayed diagnosis and a poorer prognosis. Due to the relatively independent anatomy and the relatively high proportion of patients undergoing surgery in the ileocecal region, cecum and appendix involvement had a significantly better prognosis (Overall survival time of 96 vs. 26 months, P=0.038) (25), with the 5-year OS (72%) (26).
As a common second malignancy, many patients with DLBCL had a history of other malignancies before diagnosis, and their prognosis was significantly worse. The second malignancy can increase risk of death 2.6-fold with SEER database study (27), and could have a significant impact on survival with huge differences between younger and older patients (28). As the impact on prognosis increased disproportionately with age, that was why the second malignancy was not consistent with PH assumption. Although second malignancy could not be included in the Cox regression prognostic model of this study, it served as a reminder that the second malignancy played an important role in prognosis of PIC-DLBCL.
Surgical treatment might have some effect on prognosis. A retrospective analysis indicated that patients receiving surgery for small bowel NHL had a better prognosis than the non-surgical group (29). Radman et al. (30) also showed a 10-year event-free survival of 58% and 52% of the surgically treated and untreated groups, suggesting that surgical treatment improved prognosis, which was the same as the findings of this study.
In this study, there were no significant prognostic differences in sex, race, and type of histology. Among them, Race and Sex issues had many confounding factors in the real world (31,32), and a more rational design was needed for further research in the follow-up. Regarding histology-related data, SEER database provided only abbreviated data and could not reflect the relationship with prognosis. It is hoped that more histology-related data will be made available to facilitate prognostic studies of lymphoma.
Finally, as we know, this study is the first PIC-DLBCL prognostic study, including marital status and medical insurance. Although this study focuses on PIC-DLBCL, we believe that this finding may be applicable to all prognostic analyses of malignancy with a longer survival time. At the same time, there were some limitations to this study. First, information of treatment was lacking. This made it difficult to determine what proportions of PB‐DLBCL patients actually received rituximab and anthracycline‐containing chemotherapy. However, it was reasonable to assume that most patients received appropriate and regular therapy. Another limitation was that the Lugano staging data and molecular markers for those patients were unavailable in SEER database. Nowadays, Germany has been spearheading a 15-year CAYA (the long-term outcomes of child, adolescent and young adult cancer survivors) program in 2018 (33). It focuses on observing the prognosis of patients with tumors under psychological and social intervention. Although the research data is not yet publicly available, more research will emerge as medicine evolves and survival time increases, and the family, social support and medical resources will become more relevant to disease prognosis.
Acknowledgments
Funding: None.
Footnote
Reporting Checklist: The authors have completed the TRIPOD reporting checklist. Available at http://dx.doi.org/10.21037/tcr-20-3086
Peer Review File: Available at http://dx.doi.org/10.21037/tcr-20-3086
Conflicts of Interest: All authors have completed the ICMJE uniform disclosure form (available at http://dx.doi.org/10.21037/tcr-20-3086). The authors have no conflicts of interest to declare.
Ethical Statement: The authors are accountable for all aspects of the work in ensuring that questions related to the accuracy or integrity of any part of the work are appropriately investigated and resolved. The study was conducted in accordance with the Declaration of Helsinki (as revised in 2013). Since the data from the SEER registry were de-identified and publicly available, no institutional review board approval was necessary and no informed consent was signed for this study.
Open Access Statement: This is an Open Access article distributed in accordance with the Creative Commons Attribution-NonCommercial-NoDerivs 4.0 International License (CC BY-NC-ND 4.0), which permits the non-commercial replication and distribution of the article with the strict proviso that no changes or edits are made and the original work is properly cited (including links to both the formal publication through the relevant DOI and the license). See: https://creativecommons.org/licenses/by-nc-nd/4.0/.
References
- Sukswai N, Lyapichev K, Khoury JD, et al. Diffuse large B-cell lymphoma variants: an update. Pathology 2020;52:53-67. [Crossref] [PubMed]
- Smith A, Howell D, Patmore R, et al. Incidence of haematological malignancy by sub-type: a report from the Haematological Malignancy Research Network. Br J Cancer 2011;105:1684-92. [Crossref] [PubMed]
- Sehn LH, Donaldson J, Chhanabhai M, et al. Introduction of combined CHOP plus rituximab therapy dramatically improved outcome of diffuse large B-cell lymphoma in British Columbia. J Clin Oncol 2005;23:5027-33. [Crossref] [PubMed]
- Ruppert AS, Dixon JG, Salles G, et al. International prognostic indices in diffuse large B-cell lymphoma: a comparison of IPI, R-IPI, and NCCN-IPI. Blood 2020;135:2041-8. [Crossref] [PubMed]
- Quast LF, Phillips PC, Li Y, et al. A prospective study of family predictors of health-related quality of life in pediatric brain tumor survivors. Pediatr Blood Cancer 2018;65:e26976. [Crossref] [PubMed]
- Harlan LC, Hankey BF. The surveillance, epidemiology, and end-results program database as a resource for conducting descriptive epidemiologic and clinical studies. J Clin Oncol 2003;21:2232-3. [Crossref] [PubMed]
- Xue Y, Schifano ED. Diagnostics for the Cox model. Communications for Statistical Applications and Methods 2017;24:583-604. [Crossref]
- ANKERST DP. Regression Modeling Strategies: With Applications, to Linear Models, Logistic and Ordinal Regression, and Survival Analysis. Biometrics 2016;72:1006-9. [Crossref]
- Kerr KF, Brown MD, Zhu K, et al. Assessing the Clinical Impact of Risk Prediction Models With Decision Curves: Guidance for Correct Interpretation and Appropriate Use. J Clin Oncol 2016;34:2534-40. [Crossref] [PubMed]
- Zhang Z, Rousson V, Lee WC, et al. Decision curve analysis: a technical note. Ann Transl Med 2018;6:308. [Crossref] [PubMed]
- Sehn LH, Berry B, Chhanabhai M, et al. The revised International Prognostic Index (R-IPI) is a better predictor of outcome than the standard IPI for patients with diffuse large B-cell lymphoma treated with R-CHOP. Blood 2007;109:1857-61. [Crossref] [PubMed]
- Yang S, Yu Y, Jun-Min L, et al. Reassessment of the prognostic factors of international prognostic index (IPI) in the patients with diffuse large B-cell lymphoma in an era of R-CHOP in Chinese population. Ann Hematol 2009;88:863-9. [Crossref] [PubMed]
- Hong T, Cai D, Jin L, et al. Development and validation of a nomogram to predict survival after curative resection of nonmetastatic colorectal cancer. Cancer Med 2020;9:4126-36. [Crossref] [PubMed]
- Pu N, Li J, Xu Y, et al. Comparison of prognostic prediction between nomogram based on lymph node ratio and AJCC 8th staging system for patients with resected pancreatic head carcinoma: a SEER analysis. Cancer Manag Res 2018;10:227-38. [Crossref] [PubMed]
- Miyagi S, Ishikawa E, Nakamura M, et al. Reappraisal of Primary Epstein-Barr Virus (EBV)-positive Diffuse Large B-Cell Lymphoma of the Gastrointestinal Tract: Comparative Analysis Among Immunosuppressed and Nonimmunosuppressed Stage I and II-IV Patients. Am J Surg Pathol 2020;44:1173-83. [Crossref] [PubMed]
- Jiang S, Zhen H, Jiang H. Second primary malignancy in diffuse large B-cell lymphoma patients: A SEER database analysis. Curr Probl Cancer 2020;44:100502. [Crossref] [PubMed]
- Malpert AV, Kimberg C, Luxton J, et al. Emotional distress in parents of long-term survivors of childhood acute lymphoblastic leukemia. Psychooncology 2015;24:1116-23. [Crossref] [PubMed]
- Kirchhoff AC, Yi J, Wright J, et al. Marriage and divorce among young adult cancer survivors. J Cancer Surviv 2012;6:441-50. [Crossref] [PubMed]
- Kollman J, Sobotka HL. Poverty and Cancer Disparities in Ohio. Prev Chronic Dis 2018;15:E152. [Crossref] [PubMed]
- Ward E, Jemal A, Cokkinides V, et al. Cancer disparities by race/ethnicity and socioeconomic status. CA Cancer J Clin 2004;54:78-93. [Crossref] [PubMed]
- Klapper W, Kreuz M, Kohler CW, et al. Patient age at diagnosis is associated with the molecular characteristics of diffuse large B-cell lymphoma. Blood 2012;119:1882-7. [Crossref] [PubMed]
- Xiao M, Lin J, Xiao T, et al. The incidence and survival outcomes of patients with primary cardiac lymphoma: A SEER-based analysis. Hematol Oncol 2020;38:334-43. [Crossref] [PubMed]
- Makita S, Maruyama D, Maeshima AM, et al. A comparison of clinical staging using the Lugano versus Ann Arbor classifications in Japanese patients with Hodgkin lymphoma. Asia Pac J Clin Oncol 2020;16:108-14. [Crossref] [PubMed]
- Sharma B, Pavelock N, Antoine M, et al. Primary Diffuse Large B-Cell Lymphoma of the Descending Colon. Am J Med Sci 2019;358:164-7. [Crossref] [PubMed]
- Skube SJ, Arsoniadis EG, Sulciner ML, et al. Colorectal Lymphoma: A Contemporary Case Series. Dis Colon Rectum 2019;62:694-702. [Crossref] [PubMed]
- Kim SJ, Choi CW, Mun YC, et al. Multicenter retrospective analysis of 581 patients with primary intestinal non-hodgkin lymphoma from the Consortium for Improving Survival of Lymphoma (CISL). BMC Cancer 2011;11:321. [Crossref] [PubMed]
- Goldfarb M, Rosenberg AS, Li Q, et al. Impact of latency time on survival for adolescents and young adults with a second primary malignancy. Cancer 2018;124:1260-8. [Crossref] [PubMed]
- Keegan THM, Bleyer A, Rosenberg AS, et al. Second Primary Malignant Neoplasms and Survival in Adolescent and Young Adult Cancer Survivors. JAMA Oncol 2017;3:1554-7. [Crossref] [PubMed]
- Iida T, Nozawa H, Sonoda H, et al. Upfront Surgery for Small Intestinal Non-Hodgkin's Lymphoma. Anticancer Res 2020;40:2373-7. [Crossref] [PubMed]
- Radman I, Kovacevic-Metelko J, Aurer I, et al. Surgical resection in the treatment of primary gastrointestinal non-Hodgkin's lymphoma: retrospective study. Croat Med J 2002;43:555-60. [PubMed]
- Guadamuz JS, Ozenberger K, Qato DM, et al. Mediation analyses of socioeconomic factors determining racial differences in the treatment of diffuse large B-cell lymphoma in a cohort of older adults. Medicine (Baltimore) 2019;98:e17960. [Crossref] [PubMed]
- Horesh N, Horowitz NA. Does gender matter in non-Hodgkin lymphoma? Differences in epidemiology, clinical behavior, and therapy. Rambam Maimonides Med J 2014;5:e0038. [Crossref] [PubMed]
- Salchow J, Mann J, Koch B, et al. Comprehensive assessments and related interventions to enhance the long-term outcomes of child, adolescent and young adult cancer survivors - presentation of the CARE for CAYA-Program study protocol and associated literature review. BMC Cancer 2020;20:16. [Crossref] [PubMed]