An investigation to identify tumor microenvironment-related genes of prognostic value in lung squamous cell carcinoma based on The Cancer Genome Atlas
Introduction
Lung cancer accounts for over 25% of cancer-related deaths worldwide. Lung cancers mainly fall into 2 histologic categories: small cell lung carcinoma (SCLC) and non-small cell lung cancer (NSCLC). NSCLC accounts for the largest proportion (80%) of lung cancers and mainly consists of lung adenocarcinoma and lung squamous cell carcinoma (LUSC). Despite LUSC being the dominant form of NSCLC (nearly 40% of cases), it significantly lacks biomarkers and targeted agents compared to lung adenocarcinoma. The precise targets and pathogenesis of LUSC remain unclear (1-3).
Accumulative evidences suggest that non-tumor cells of the tumor microenvironment (TME) play a pivotal role in cancer development and progression (4-7). The TME refers to a complicated environment where tumor cell locates and proliferates. Immune, endothelial, and epithelial cells, together with extracellular matrix, fibroblasts, chemokines, and cytokines, also populate the TME (8,9). Infiltrating immune cells and stromal cells are 2 primary components of the TME, and investigations of their interactions have been valuable in the exploration of novel therapeutic approaches for LUSC (10). However, to date, the majority of research on the TME has been directed toward the immune microenvironment; as such, stromal cells in the TME have yet to be investigated in any depth.
Estimation of STromal and Immune cells in MAlignant Tumor tissues using Expression data (ESTIMATE) is an algorithm designed for the quantitation of immune and stromal cells in tumors. Through the calculation of gene expression data, ESTIMATE can facilitate a better understanding of the landscape of non-tumor cells in the TME (11). Studies on hepatocellular carcinoma, acute myeloid leukemia, and glioblastoma have shown the accuracy and robustness of ESTIMATE. However, a comprehensive investigation to explore the influences of TME immune and stromal scores on LUSC has yet to be performed (12-14). A publicly accessible gene expression database The Cancer Genome Atlas (TCGA) allows for the discovery and categorization of genomic abnormalities in large-scale datasets from around the globe. By using this approach, the potential associations exist between genes and prognostic outcomes in LUSC, can be explored (15-17). In this work, we downloaded the TCGA LUSC dataset and determined the immune and stromal score of patients using the ESTIMATE algorithm. Subsequently, TME-associated genes were screened to identify those that could potentially be predictive of a poor outcome of LUSC, and the underlying interactions of relevant differentially expressed genes (DEGs) were uncovered.
We present the following article in accordance with the REMARK reporting checklist (available at http://dx.doi.org/10.21037/tcr-21-401).
Methods
Data source
The publicly accessible LUSC dataset was downloaded from TCGA (http://portal.gdc.cancer.gov/). The dataset included patients’ gene expression profiling data (level 3), as well as clinical details such as sex, age, histological type, and survival time. The ESTIMATE algorithm was applied for the calculation of stromal and immune scores based on the downloaded RNA expression data. Cases were classified as having a high or low score according to the top and bottom quartiles of the immune/stromal scores.
The study was conducted in accordance with the Declaration of Helsinki (as revised in 2013).
Calculation of stromal and immune scores
ESTIMATE outputs stromal and immune scores by performing single-sample gene set-enrichment analysis (ssGSEA). In our analysis of LUSC samples from TCGA, gene expression values were subjected to rank normalization and rank ordering. Following that, the calculation of empirical cumulative distribution functions was performed for the signature genes and the remaining genes. Finally, a statistic was obtained through integrating the difference between the empirical cumulative distribution functions. Furthermore, a prognostic risk score model based on the immune and stromal scores was constructed by multivariate Cox regression, with the coefficient (β) used for weighting. We calculated the risk score using the following formula: risk score = immune score × β1 + stromal score × β2 (18). According to the median risk score, the model divided LUSC cases into high- and low-risk score groups. The performance of the risk score model in predicting prognosis was assessed by drawing time-dependent receiver operating characteristic (ROC) curves using the “survivalROC” package (version 1.0.3) in R software (19).
Screening of DEGs
Data were analyzed with “DESeq2” (version 1.28.1) in R software (version 3.6.0) (20). The criteria for screening DEGs were as follows: mean value of gene expression >5; absolute value of log2 fold change (log2FC) ≥1; and false discovery rate (FDR) <0.05. Subsequently, the common upregulated DEGs between the immune and stromal groups were used for further analyses.
Functional enrichment analyses
Fisher’s exact test was applied in the Gene Ontology (GO) term and Kyoto Encyclopedia of Genes and Genomes (KEGG) pathway analyses (21,22). GO terms reflect the functions of candidate signatures with respect to biological processes (BPs), molecular functions (MFs), and cellular components (CCs). KEGG analysis uncovers the pathways in which DEGs are enriched. A P value of <0.05 was set as the cut-off for significance.
Protein-protein interaction (PPIs) network construction
An analysis of the PPIs of the TME-related DEGs was performed using the Search Tool for the Retrieval of Interacting Genes (STRING) database (http://string-db.org). Visualization of the interaction network was performed with Cytoscape software (23,24). We employed the Cytoscape plugin Molecular Complex Detection (MCODE) for the detection of the module with the greatest degree of significance in regard to the topology of the connected regions. The MCODE parameters used were: degree cut-off =2, node score cut-off =0.2, k-core =2, and maximum depth =100.
Statistical analyses
The prognostic significance of TME-related common upregulated DEGs, high and low immune/stromal scores, and high and low risk scores was examined with Kaplan-Meier estimation followed by the Log-rank test. A P value of <0.05 served as the criterion for statistical significance (25). The above analyses were performed using R software. Additionally, the PrognoScan online tool was utilized to verify the DEGs of significant prognostic value (26).
Results
Immune and Stromal Scores of Patients with LUSC
A total of 551 patients with LUSC were included from the TCGA database, and their clinical information is shown in Table 1. The ESTIMATE algorithm was utilized for the assessment of immune and stromal cells in the samples on the basis of RNA sequencing data. The immune and stromal scores of the samples ranged from −2,336 to 6,956 and from −190 to 8,913, respectively. To investigate possible correlations between overall survival (OS) and stromal and immune scores, we assigned the LUSC cases to the high- and low-score group according to their stromal/immune scores. The survival analysis results (Figure 1A,B) suggested that the immune/stromal scores showed significant associations with OS (log-rank P<0.05). Meanwhile, the overall survival analysis of patients with lung adenocarcinoma was also performed to assess the effect of immune/stromal score on prognosis, however, the result was not statistical significant, shown as Figures S1 and S2, respectively. Furthermore, the prognostic risk score model of LUSC based on immune and stromal scores also showed significant correlations with OS (Figure 1C). The risk score formula was: risk score = immune score × 0.4374 + stromal score × 0.2985, and the effectiveness of this prognostic model was evidenced by time dependent ROC curves (Figure 1D), the area under curve (AUC) is 0.728.
Table 1
Clinical Information | Statistics |
---|---|
Sex (male/female) | 373/131 |
Age (>60 years old, yes/no) | 404/91 |
Pathologic_M (M0/M1) | 7/414 |
Pathologic_N (N0/N1/N2/N3) | 320/133/40/5 |
Pathologic_T (T1/T2/T3/T4) | 114/295/71/24 |
Stage (I/II/III/IV) | 245/163/85/7 |
Survival status (alive/dead) | 284/220 |
Overall survival, days | 968.4±957.7 |
Smoking duration, years | 39.6±12.2 |
LUSC, lung squamous cell carcinoma; TCGA, The Cancer Genome Atlas.
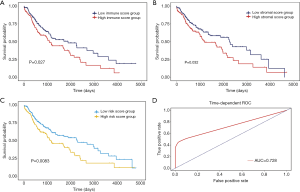
DEG screening
Statistically, 3,045 DEGs were screened out from the high and low immune score groups, of which 1,718 were upregulated and 1,327 were downregulated. In a similar way, 3,240 DEGs were identified from the high and low stromal score groups, including 1,813 that were upregulated and 1,427 that were downregulated. Volcano plots (Figure 2A,B) and heatmaps (Figure 2C,D) displayed the DEG expression profiles of the immune and stromal score groups, respectively. Venn diagram analysis showed that 824 upregulated DEGs and 548 downregulated DEGs overlapped between the immune and stromal score groups, as shown in Figure 2E and Figure 2F, respectively. These overlapping upregulated genes, all of which met the criteria of |log2FC| >1 and FDR <0.05, were selected for further analysis.
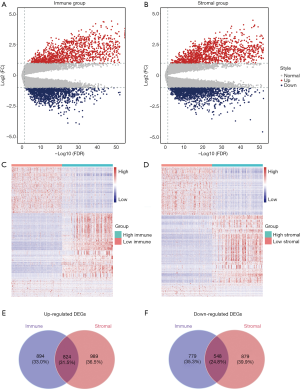
Functional enrichment analysis
Fisher’s exact test was applied in the functional enrichment analysis of the common upregulated DEGs. The results suggested that the DEGs were closely linked to immune response and cytokine-cytokine receptor interaction. As shown in Figure 3A, the results of GO term analysis revealed the DEGs to have significant enrichment in BPs including immune response, cell adhesion, and immune system process. In terms of CCs, the DEGs were predominantly enriched in the plasma membrane, extracellular region and nucleus. With respect to MFs, the DEGs were involved in calcium ion binding, DNA binding, and receptor activity. Additionally, as shown in Figure 3B, the DEG-related enriched KEGG pathways were obviously associated with cytokine-cytokine receptor interaction, cell adhesion molecules, and tumor relevant pathways including the ras, the transforming growth factor (TGF) beta, and the nuclear factor (NF) kappa B signaling pathways.
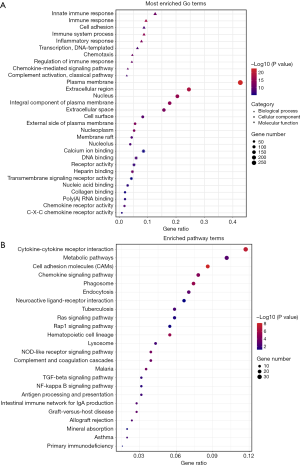
Survival analysis and verification with gene expression omnibus data
To determine the significance of the TME-related DEGs for predicting the prognosis of LUSC, Kaplan-Meier survival curves were drawn to examine the relationships of common upregulated DEGs with the OS of patients with LUSC from the TCGA database. Among the 824 co-upregulated DEGs, 94 were significantly associated with a poor prognosis of LUSC according to the log-rank test (data not shown). Finally, BHMT2, FES, HSPB7, NOVA2, LPAP2, and SEMA3B (BFHNLS) were identified as potential predictors of a poor prognosis (Figure 4), and were verified using the PrognoScan online tool and Gene Expression Omnibus datasets GSE4573 and GSE17710 (Figure 5).
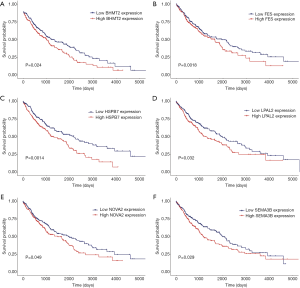
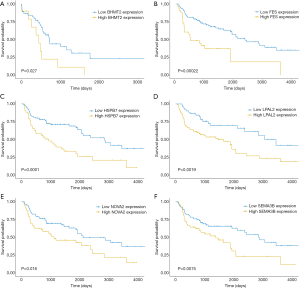
PPI network construction
To explore the potential interactions of the TME-related DEGs, a PPI analysis of the co-upregulated DEGs was performed using the STRING database. Visualization of the interaction network was performed with Cytoscape. The top 3 modules in the network, as assessed by MCODE, are shown in Figure 6. Pathway enrichment analysis indicated that genes involved in module 1 were enriched in the terms of signaling by GPCR and signaling transduction (Figure 6A,B). Module 2 genes were primarily clustered in pathways related to the immune system and interleukin signaling (Figure 6C,D). The most enriched pathway terms for the module 3 genes were similar to those mentioned above for the other 2 modules (Figure 6E,F). Moreover, the 94 DEGs with significant unfavorable prognostic value were used for PPI network construction (Figure 7A). Cytokine-cytokine receptor interaction, endocytosis, and PI3K-Akt signaling pathway were the top pathway terms (Figure 7B).
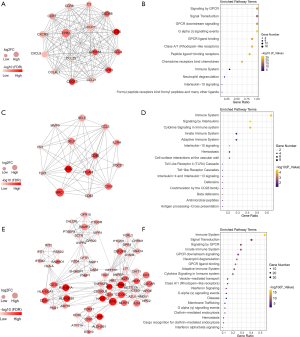
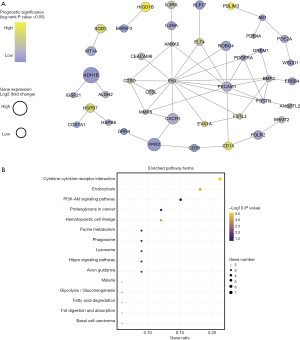
Discussion
Male patients with LUSC are significantly more than female patients, and smokers are more prone to develop the disease. The prevalence and mortality are higher in patients over 60 years old. The prevalence is higher in developed countries, and the disease is more susceptible for urban patients than for rural ones. LUSC originates from bronchial mucosal epithelium and is mostly found in lobar and segmental bronchi. LUSC belongs to central type lung cancer in the clinical classification, of which the cells are often accompanied with cornification, showing irregular spindle-shaped. The early LUSC is in situ or early invasive carcinoma, and deteriorates as bronchial lumen tumor in advanced stage.
Despite being a more prevalent subtype of lung cancer, LUSC lacks effective therapeutic targets as compared to lung adenocarcinoma (27). Large-scale mining of data from publicly available collections has been applied to identify potential biomarkers in various cancers, including LUSC. Previous research has revealed that the TME plays a pivotal role in the growth and progression of LUSC (28,29). Infiltrating immune and stromal cells are primary non-tumor constituents of the TME that can affect cell proliferation and therapeutic resistance. Several bioinformatics tools have been developed for the assessment of cell types in tumor tissues. Specifically, the ESTIMATE algorithm has proven effectiveness for the calculation of immune/stromal scores in lung cancer (30). In this study, the groups categorized according to immune/stromal scores showed a significant association with OS. Moreover, our prognostic model showed a close correlation of risk score with a poor prognosis (P value =0.0083), which was evidenced by a favorable result (AUC =0.728) in the ROC analysis. The survival analysis for immune/stromal scores indicated the important influence of non-tumor components on the pathogenesis of LUSC. In recent studies, mining a signature of prognostic value related to the TME has attracted much attention (12-14).
A total of 824 common upregulated DEGs were subjected to further analysis. The functional enrichment assessment of these genes was consistent with the findings of previous research that immune response and inflammatory response are the significantly enriched BP terms (31-33). Meanwhile, several typical carcinogenesis-related pathways were among the significantly enriched pathways, such as the TGF-beta and the NF-kappa B signaling pathways. The former plays a key role in cell proliferation, interstitial production, differentiation, apoptosis, embryonic development, organ formation, immune function, and inflammatory response (34). Meanwhile, the NF-kappa B signaling pathway is involved in pathological processes such as infection, inflammation, immune response, apoptosis, and tumor formation, as well as cell cycle regulation and cell differentiation through target gene expression products (35). In the PPI analysis of common upregulated DEGs, the genes of the module 1 network were mainly enriched in the pathway terms regarding GPCR, which is related to cancer progression. GPCRs are responsible for the activation of receptor tyrosine kinases, which are over-expressed in numerous carcinomas (36). In the functional assessment of both module 2 and module 3 networks, immune system-related pathways were involved in the top enriched pathway terms. Dysregulation of the immune system leads to abnormal cell proliferation, resulting in the formation of the TME (29,37). In particular, PPI analysis of the DEGs of prognostic value revealed that the PI3K-Akt signaling pathway and hippo signaling pathway were significantly enriched, which suggested these genes are probably associated with the growth and progression of LUSC. Furthermore, to investigate the potential relation of gene mutation to immunological characteristics in LUSC, a total of 22 LUSC gene mutations were identified after querying online tools. FireBrowse was performed using TCGA datasets (Figure S3), in which IRF6 was also found to be included in the DEGs of immune group. IRF6 is one of the enriched genes of nod-like receptor signaling pathway that co-upregulated DEGs clustered in, which suggested an underlying role of IRF6 in the tumorigenesis of LUSC with respect to immunological characteristics, yet need a further exploration.
Survival analysis of the co-upregulated TME-related DEGs demonstrated that 94 genes were significantly associated with poor outcomes of patients with LUSC. Besides canonical cancer pathways, pathway enrichment analysis of these genes illustrated their close connection with metabolic processes. Previous studies have reported that glycolysis supplies cancer cells with energy, thus supporting unlimited proliferative activity (38,39). In addition, fat acid metabolism is, to some degree, involved in tumor formation. With regard to cell assembly, fat acid participates in phospholipid synthesis and vital signal transduction in the cell membrane, such as PI3K-Akt signaling. For cellular metabolism, cancer cells mainly utilize fat acid β-oxidation to produce ATP in order to meet the energy requirement, and also use nicotinamide adenine dinucleotide phosphate (NADPH) to maintain the balance of intracellular redox reactions; however, the mechanism needs further investigation (40,41). ALDH3B1 and ADH1B are major genes linked to the pathways of gluconeogenesis and fatty acid degradation (42,43). In patients with LUSC, tobacco exposure for a certain duration may lead to chemical carcinogenesis; however, unfortunately, this conclusion could not summarized with a statistically non-significant result in the pathway enrichment analysis. KEAP1 and NFE2L2 can encode interacting proteins that mediate the cytoprotective response to oxidative stress and exogenous stimuli, the Kaplan-Meier survival curves of KEAP1 and NFE2L2 suggested their expression level were significantly associated with prognosis of patients with LUSC (Figures S4 and S5). However, the mutation of KEAP1 and NFE2L2 could not predict the prognosis of LUSC patients (Figures S6 and S7).
With respect to the 6 verified TME-related DEGs with prognostic value, the identifier FES is the only known member of a specific subfamily of non-receptor protein-tyrosine kinases. Previous reports indicated the involvement of these kinases in the regulation of cytoskeletal rearrangement and inside-out signaling accompanying receptor–ligand, cell-matrix, and cell-cell interactions (44,45). NOVA2 is possibly a regulator of RNA splicing or metabolism in a defined subset of developing neurons, as well as a binder of single-strand RNA. Diseases associated with NOVA2 include paraneoplastic polyneuropathy (46,47). LPAL2 is the distinguishing protein moiety of lipoprotein(a). Each transcript of LPAL2 displays open reading frame truncation and is a candidate for nonsense-mediated decay (44). HSPB7 encodes a small heat shock family B member which is able to heterodimerize with similar heat shock proteins. As part of the p53 pathway, HSPB7 performs a possible tumor-suppressive function, and its association with renal cell carcinoma has also been uncovered (48). The membrane function of SEMA3B is involved in the growth cone guidance during neuronal development, and SEMA3B expression is suggestive of carcinogenic progression in endometrial cancer (49). Finally, BHMT2 has been reported to be related to the progression of hepatocellular carcinoma (50). The genes described above might serve as prognostic biomarkers for patients with LUSC, and this finding needs to be the subject of further clinical study.
Facing the poor situation that targeted therapy lacks sufficient efficacy in the treatment of LUSC, there is an urgent need for novel high-throughput sequencing technology and analysis strategy to identify more effective molecular signature in LUSC. Patients with LUSC may benefit from a combination treatment of chemotherapy and targeted therapy. Besides, the CIK treatment can also provide supportive options. Moreover, the immune checkpoint strategy appears to be a promising way for the treatment of LUSC in the coming days.
In contrast with previous studies that paid attention to the activation of intrinsic gene in oncogenesis, this work focused on the gene signature of the TME in LUSC, which supports the progression of LUSC, thus influencing the prognostic outcomes of patients. The current work may offer a novel perspective on the intricate interactions of LUSC with its TME. However, we were unable to comprehensively analyze survival with regard to other prognosis-associated factors, due to the lack of detailed treatment information. Further, biases may have been introduced by the fact that all cases in this study were acquired from a single cohort. Finally, except for the 6 confirmed TME-related prognostic genes, the remaining candidate biomarkers for LUSC still need to be validated through investigations involving reverse transcription-polymerase chain reaction and western blot.
Conclusions
In conclusion, the immune and stromal scores of LUSC tissue samples were calculated using the ESTIMATE algorithm. A total of 94 TME-associated genes were found to be related to an unfavorable prognostic outcome in a LUSC cohort from the TCGA database. Among them, 6 potential biomarkers (BHMT2, FES, HSPB7, NOVA2, LPAP2, and SEMA3B) were verified using Gene Expression Omnibus datasets, and may be considered to be a candidate gene signature for predicting the prognosis of patients with LUSC. This study is the first to demonstrate the significance of these genes in the prognosis of LUSC, and they should be studied more extensively to shed more light on and to gain a deeper understanding of the correlation that potentially exists between the TME and prognosis in LUSC. Additionally, strategically, this study evidenced that mining TME-related genes from big-scale data may offer new perspectives in efforts to discover more biomarkers which have prognostic value for LUSC and other cancers.
Acknowledgments
Funding: This work was supported by grants from
Footnote
Reporting Checklist: The authors have completed the REMARK reporting checklist. Available at http://dx.doi.org/10.21037/tcr-21-401
Conflicts of Interest: All authors have completed the ICMJE uniform disclosure form (available at http://dx.doi.org/10.21037/tcr-21-401). The authors have no conflicts of interest to declare.
Ethical Statement: The authors are accountable for all aspects of the work in ensuring that questions related to the accuracy or integrity of any part of the work are appropriately investigated and resolved. Ethical approval was not sought for the present study, because it did not involve any experiments. The study was conducted in accordance with the Declaration of Helsinki (as revised in 2013).
Open Access Statement: This is an Open Access article distributed in accordance with the Creative Commons Attribution-NonCommercial-NoDerivs 4.0 International License (CC BY-NC-ND 4.0), which permits the non-commercial replication and distribution of the article with the strict proviso that no changes or edits are made and the original work is properly cited (including links to both the formal publication through the relevant DOI and the license). See: https://creativecommons.org/licenses/by-nc-nd/4.0/.
References
- Siegel R, Naishadham D, Jemal A. Cancer statistics, 2012. CA Cancer J Clin 2012;62:10-29. [Crossref] [PubMed]
- Ferlay J, Soerjomataram I, Dikshit R, et al. Cancer incidence and mortality worldwide: Sources, methods and major patterns in GLOBOCAN 2012. Int J Cancer 2015;136:E359-86. [Crossref] [PubMed]
- Wei Y, Chen L, Zhou W, et al. Tissue spray ionization mass spectrometry for rapid recognition of human lung squamous cell carcinoma. Sci Rep 2015;5:10077. [Crossref] [PubMed]
- Khamisipour G, Jadidi-Niaragh F, Jahromi AS, et al. Mechanisms of tumor cell resistance to the current targeted-therapy agents. Tumour Biol 2016;37:10021-39. [Crossref] [PubMed]
- Kürten C, Chen X, Kulkarni A, et al. Comprehensive investigation of the tumor microenvironment (TME) in Head and Neck Squamous cell carcinoma (HNSCC) using single cell RNA sequencing. Laryngo-Rhino-Otologie 2019;98:11148.
- Turley SJ, Cremasco V, Astarita JL. Immunological hallmarks of stromal cells in the tumour microenvironment. Nat Rev Immunol 2015;15:669-82. [Crossref] [PubMed]
- Zhang L, MacIsaac K, Zhou T, et al. Genomic Analysis of Nasopharyngeal Carcinoma Reveals TME-Based Subtypes. Mol Cancer Res 2017;15:1722-32. [Crossref] [PubMed]
- Bolouri H. Network dynamics in the tumor microenvironment. Semin Cancer Biol 2015;30:52-9. [Crossref] [PubMed]
- Ayala F, Dewar R, Kieran M, et al. Contribution of bone microenvironment to leukemogenesis and leukemia progression. Leukemia 2009;23:2233-41. [Crossref] [PubMed]
- Kulasingam V, Diamandis E. Strategies for discovering novel cancer biomarkers through utilization of emerging technologies. Nat Clin Pract Oncol 2008;5:588-99. [Crossref] [PubMed]
- Yoshihara K, Shahmoradgoli M, Martinez E, et al. Inferring tumour purity and stromal and immune cell admixture from expression data. Nat Commun 2013;4:2612. [Crossref] [PubMed]
- Yan H, Qu J, Cao W, et al. Identification of prognostic genes in the acute myeloid leukemia immune microenvironment based on TCGA data analysis. Cancer Immunol Immunother 2019;68:1971-8. [Crossref] [PubMed]
- Deng Z, Wang J, Xu B, et al. Mining TCGA Database for Tumor Microenvironment-Related Genes of Prognostic Value in Hepatocellular Carcinoma. Biomed Res Int 2019;2019:2408348. [Crossref] [PubMed]
- Jia D, Li S, Li D, et al. Mining TCGA database for genes of prognostic value in glioblastoma microenvironment. Aging (Albany NY) 2018;10:592-605. [Crossref] [PubMed]
- Tomczak K. CzerwiDska P, Wiznerowicz M. The Cancer Genome Atlas (TCGA): an immeasurable source of knowledge. Contemp Oncol (Pozn) 2015;19:A68-77. [Crossref] [PubMed]
- Koleczko S, Schäpers C, Scheffler M, et al. A comprehensive analysis of potentially targetable genetic aberrations and clinical findings in 821 patients with squamous-cell NSCLC - a comparison of NGM and TCGA LUSC data. Onkologie 2016;27:V1420.
- Xie ZC, Li T, Gan B, et al. Investigation of miR-136-5p key target genes and pathways in lung squamous cell cancer based on TCGA database and bioinformatics analysis. Pathol Res Pract 2018;214:644-54. [Crossref] [PubMed]
- Liao X, Wang X, Huang K, et al. Genome-scale analysis to identify prognostic microRNA biomarkers in patients with early stage pancreatic ductal adenocarcinoma after pancreaticoduodenectomy. Cancer Manag Res 2018;10:2537-51. [Crossref] [PubMed]
- Combescure C, Perneger T, Weber D, et al. Prognostic ROC curves: a method for representing the overall discriminative capacity of binary markers with right-censored time-to-event endpoints. Epidemiology 2014;25:103-9. [Crossref] [PubMed]
- Love MI, Huber W, Anders S. Moderated estimation of fold change and dispersion for RNA-seq data with DESeq2. Genome Biol 2014;15:550. [Crossref] [PubMed]
- Mehta C, Patel N. A Network Algorithm for Performing Fisher's Exact Test in r × c Contingency Tables. J Am Stat Assoc 1983;78:427-34. [Crossref]
- Glass K, Girvan M. Annotation Enrichment Analysis: An Alternative Method for Evaluating the Functional Properties of Gene Sets. Sci Rep 2014;4:4191. [Crossref] [PubMed]
- Szklarczyk D, Morris J, Cook H, et al. The STRING database in 2017: quality-controlled protein-protein association networks, made broadly accessible. Nucleic Acids Res 2017;45:D362-8. [Crossref] [PubMed]
- Demchak B, Hull T, Reich M, et al. Cytoscape: the network visualization tool for GenomeSpace workflows. F1000Res 2014;3:151. [Crossref] [PubMed]
- Guyot P, Ades A, Ouwens M, et al. Enhanced secondary analysis of survival data: reconstructing the data from published Kaplan-Meier survival curves. BMC Med Res Methodol 2012;12:9. [Crossref] [PubMed]
- Mizuno H, Kitada K, Nakai K, et al. PrognoScan: a new database for meta-analysis of the prognostic value of genes. BMC Med Genomics 2009;2:18. [Crossref] [PubMed]
- Osmani L, Askin F, Gabrielson E, et al. Current WHO guidelines and the critical role of immunohistochemical markers in the subclassification of non-small cell lung carcinoma(NSCLC): Moving from targeted therapy to immunotherapy. Semin Cancer Biol 2018;52:103-9. [Crossref] [PubMed]
- Li Y, Gu J, Xu F, et al. Transcriptomic and functional network features of lung squamous cell carcinoma through integrative analysis of GEO and TCGA data. Sci Rep 2018;8:15834. [Crossref] [PubMed]
- Chen S, Giannakou A, Golas J, et al. Multidimensional Coculture System to Model Lung Squamous Carcinoma Progression. J Vis Exp 2020; [Crossref] [PubMed]
- Seo JS, Kim A, Shin JY, et al. Comprehensive analysis of the tumor immune icro-environment in non-small cell lung cancer for efficacy of checkpoint inhibitor. Sci Rep 2018;8:14576. [Crossref] [PubMed]
- Ning P, Wu Z, Hu A, et al. Integrated genomic analyses of lung squamous cell carcinoma for identification of a possible competitive endogenous RNA network by means of TCGA datasets. PeerJ 2018;6:e4254. [Crossref] [PubMed]
- Yuan C, Xiang L, Bai R, et al. MiR-195 restrains lung adenocarcinoma by regulating CD4+ T cell activation via the CCDC88C/Wnt signaling pathway: a study based on the Cancer Genome Atlas (TCGA), Gene Expression Omnibus (GEO) and bioinformatic analysis. Ann Transl Med 2019;7:263. [Crossref] [PubMed]
- Das RK, Yan RT, Davis C, et al. Causal modeling of TCGA, NSCLC, and HNSCC data to identify network drivers of tumor immune subtypes. J Clin Oncol 2020;38:abstr 68.
- Toonkel RL, Borczuk AC, Powell CA. Tgf-beta signaling pathway in lung adenocarcinoma invasion. J Thorac Oncol 2010;5:153-7. [Crossref] [PubMed]
- Qin X, Yan M, Wang X, et al. Cancer-associated Fibroblast-derived IL-6 Promotes Head and Neck Cancer Progression via the Osteopontin-NF-kappa B Signaling Pathway. Theranostics 2018;8:921-40. [Crossref] [PubMed]
- Mendelsohn J. The epidermal growth factor receptor as a target for cancer therapy. Endocr Relat Cancer 2001;8:3-9. [Crossref] [PubMed]
- Najafi M, Goradel NH, Farhood B, et al. Tumor microenvironment: Interactions and therapy. J Cell Physiol 2019;234:5700-21. [Crossref] [PubMed]
- Vander Heiden MG, Cantley LC, Thompson CB. Understanding the Warburg effect: the metabolic requirements of cell proliferation. Science 2009;324:1029-33. [Crossref] [PubMed]
- Vaupel P, Schmidberger H, Mayer A. The Warburg effect: essential part of metabolic reprogramming and central contributor to cancer progression. Int J Radiat Biol 2019;95:912-9. [Crossref] [PubMed]
- Turner N, Cooney G, Kraegen E, et al. Fatty acid metabolism, energy expenditure and insulin resistance in muscle. J Endocrinol 2014;220:T61-79. [Crossref] [PubMed]
- Che L, Pilo MG, Cigliano A, et al. Oncogene dependent requirement of fatty acid synthase in hepatocellular carcinoma. Cell Cycle 2017;16:499-507. [Crossref] [PubMed]
- Marchitti SA, Orlicky D, Vasiliou V. Expression and initial characterization of human ALDH3B1. Biochem Biophys Res Commun 2007;356:792-8. [Crossref] [PubMed]
- Polimanti R, Gelernter J. ADH1B: From alcoholism, natural selection, and cancer to the human phenome. Am J Med Genet B Neuropsychiatr Genet 2018;177:113-25. [Crossref] [PubMed]
- O'Leary NA, Wright MW, Brister JR, et al. Reference sequence (RefSeq) database at NCBI: current status, taxonomic expansion, and functional annotation. Nucleic Acids Res 2016;44:D733-D745. [Crossref] [PubMed]
- Hanazono Y, Chiba S, Sasaki K, et al. Erythropoietin induces tyrosine phosphorylation and kinase activity of the c-fps/fes proto-oncogene product in human erythropoietin-responsive cells. Blood 1993;81:3193-6. [Crossref] [PubMed]
- Xiao H. MiR-7-5p suppresses tumor metastasis of non-small cell lung cancer by targeting NOVA2. Cell Mol Biol Lett 2019;24:60. [Crossref] [PubMed]
- Baek S, Oh TG, Secker G, et al. The Alternative Splicing Regulator Nova2 Constrains Vascular Erk Signaling to Limit Specification of the Lymphatic Lineage. Dev Cell 2019;49:279-92.e5. [Crossref] [PubMed]
- Lin J, Deng Z, Tanikawa C, et al. Downregulation of the tumor suppressor HSPB7, involved in the p53 pathway, in renal cell carcinoma by hypermethylation. Int J Oncol 2014;44:1490-8. [Crossref] [PubMed]
- Dziobek K, Opławski M, Grabarek B, et al. Expression of Semaphorin 3B (SEMA3B) in Various Grades of Endometrial Cancer. Med Sci Monit 2019;25:4569-74. [Crossref] [PubMed]
- Pellanda H, Namour F, Fofou-Caillierez M, et al. A splicing variant leads to complete loss of function of betaine-homocysteine methyltransferase (BHMT) gene in hepatocellular carcinoma. Int J Biochem Cell Biol 2012;44:385-92. [Crossref] [PubMed]
(English Language Editor: J. Reynolds)