Diagnostic value of circulating cell-free DNA for renal cell carcinoma: a meta-analysis
Introduction
RCC is a global malignancy that represents the 6th most common diagnosed tumor in men and the 10th in women, accounting for approximately 5% and 3% of all malignancies, respectively (1-3). RCC ranks as the 13th most frequent reason for cancer death worldwide, with over 140,000 RCC-associated deaths annually (1). RCC primarily consists of three histological morphotypes, including clear cell RCC (80–90%), papillary RCC (10–15%) and chromophobe RCC (5%) (4). Currently, partial or total nephrectomy is considered as the gold standard to cure the localized and early-stage RCC (5). Though the majority of detected RCC lesions are small tumors, about 25–30% of patients present with metastatic disease at initial diagnosis and up to 20–30% of patients who have been performed by surgical intervention harbor distant metastasis (6,7). Radiological examinations and histopathological examinations are performed for the conventional diagnosis of RCC. Nevertheless, abdominal imaging detection is are insufficient to qualitatively diagnose RCC. Pathological examinations are invasive and unrepeatable as well as inappropriate to monitor disease (8-10). Therefore, novel and promising non-invasive blood biomarkers are urgently needed to more conveniently and accurately diagnose RCC (2).
Liquid biopsy, based on cfDNA, circulating tumor cell (CTC) and circulating tumor DNA (ctDNA) from blood, can confer a minimally-invasive potential for monitoring the tumor status in RCC patients (11). Circulating cell-free DNA is fragment of acellular nucleic acids with multifarious length (approximately 0.18 to 21 kB), which originates from apoptotic and necrotic tumor cells. The detection strategies for RCC by cfDNA can be classified as quantitative and qualitative analysis. The former one is recognized as a quantitative approach to measure the aberrant levels of cfDNA. While qualitative method encompasses the detection of cfDNA integrity, microsatellite alteration, mutation, or methylation and so on (12,13). Leon et al. revealed elevated concentrations of circulating cfDNA in plasma of patients with a variety of malignancies compared with healthy individuals in 1977 (14). A number of studies have demonstrated elevated circulating cfDNA concentrations in RCC patients. CfDNA levels can allow for malignant renal tissue to be distinguished from normal sample as well as benign renal hyperplasia (15-19). Furthermore, other tumor-specific signature, such as aberrant cfDNA methylation in blood, has been described a hallmark of RCC. The detection of cfDNA hypermethylation is also conducive to differentiating RCC patients from healthy individuals, which has been actively investigating for minimally invasive clinical screening and diagnosis. Especially, DNA methylation is relatively chemically stable and the techniques for detecting hypermethylated cfDNA in RCC are well-established. Therefore, in the qualitative analysis subgroup, we primarily concentrated on the detection of cfDNA methylation (20-22).
An increasing number of researches have been demonstrating that these complex modifications in circulating cfDNA exert a crucial effect on the pathogenetic events of RCC and potentially confer a reference to assist the diagnostic process. Nevertheless, the results concerning cfDNA indicators which are the most clinically relevant are still inconsistent, which is largely attributed to the heterogeneity of the study backgrounds, such as sample size and source, clinical stage, and assay methods (18). In our report, we included all relevant publications which described the diagnostic value of the concentration and methylation of blood cfDNA in RCC patients to conduct the first diagnostic meta-analysis. We primarily investigated two subgroups, including qualitative analysis of abnormal concentrations of cfDNA and qualitative analysis of single-gene methylation.
We present the following article in accordance with the PRISMA reporting checklist (available at http://dx.doi.org/10.21037/tcr-20-3448).
Methods
Search strategy
Two authors independently searched the following databases through October 30, 2020, including PubMed, Web of Science, CNKI and Cochrane Library as well as Embase databases. The retrieved terms included: (“cell-free DNA” OR “circulating DNA” OR “cfDNA” OR “serum DNA” OR “plasma DNA” OR “blood DNA”) AND (“renal cell carcinoma” OR “kidney cancer” OR “renal cell cancer” OR “kidney neoplasms”) AND (“diagnosis” OR “accuracy” OR “sensitivity” OR “specificity”). In case of disagreement, a third author was invited into the discussion.
Inclusion and exclusion criteria
Articles were eligible and incorporated when they conformed to all the following criteria: (1) sample circulating cfDNA were derived from plasma or serum or blood; (2) the SEN and SPE could be readily obtained from articles or were sufficient to be calculated based on true positives (TP), false positives (FP), false negatives (FN), and true negatives (TN). The excluded criteria were as followings: (I) reviews, letters, case reports, or conference reports; (II) sample was from urine; (III) sample size was less than 10.
Data extraction
Two researchers independently assessed qualified articles and further extracted data from these articles. The crucial information included: first author, publication year, research type, sample features (ethnicity of individuals, cases number and the type of controls), determination information (sample source, sampling time, assay methods, detective gene, cutoff values) and diagnostic data (SEN and SPE, PLR and NLR, TP, TN, FP and FN).
Quality assessment
Two authors independently evaluated the methodological qualities of the incorporated qualified publications through the revised Quality Assessment of Diagnostic Accuracy Studies-2 (QUADAS-2). Four pivotal domains, such as the patient selection index, index test, reference standard and flow and timing, were applied for evaluating risk of bias and applicability. The seven indicators (four indicators on bias risk and three indicators on applicability) were evaluated for all incorporated publications.
Statistical analysis
Data were analyzed using RevMan Manager 5.3 and Meta-Disc 1.4 software. The pooled SEN and SPE, PLR and NLR, DOR, AUC and their 95% CIs were calculated based on a bivariate random effects model, thus evaluating the overall diagnostic accuracy (2,23-26). Heterogeneity among all included publications was assessed through a χ2-based Cochran’s Q test. I2≥50% or P<0.10 displayed substantial heterogeneity. Meta-regression and subgroup analyses were conducted to dissect the sources of heterogeneity (27). We formulated Deeks’ funnel plot asymmetry test to detect the publication bias of all incorporated articles (2).
Results
Study characteristics and quality assessment
A Preferred Reporting Items for Systematic Reviews and Meta-Analyses (PRISMA) flow diagram of the literature search were revealed in Figure 1. The initial document retrieval identified 194 publications. Eight eligible articles (15-22) were ultimately incorporated into our report following the rigorous process of selection based on the inclusion and exclusion criteria. Table 1 revealed the specific characteristics of qualified articles published between 2008 and 2020. A total of 912 individuals (including 585 RCC patients, 43 individuals with benign renal diseases and 284 healthy controls) were included in our meta-analysis. A large proportion of individuals were from Europe (including Germany, Ukraine, and Italy), with the remaining subjects from Asia (Korea and Japan) and Austria. Among the five diagnostic articles with known design type, three trials were prospective studies (15,17,22) and other two focused on retrospective analysis (16,19). Of eight publications, five studies evaluated abnormal levels of circulating cfDNA in RCC cases, which were considered as the quantitative analysis subgroup (15-19). Two publications assessing single-gene methylation changes in RCC were regarded as qualitative analysis subgroup (20,21). The remaining one study described both quantitative and qualitative alterations in RCC (22). Healthy individuals were considered as controls in seven studies (15-20,22) and the other one publication (21) included benign renal diseases as control. On the basis of QUADAS-2, the quality assessment was performed through RevMan 5.3. As revealed in Figure 2 and Figure S1, these selected publications had relatively high quality.
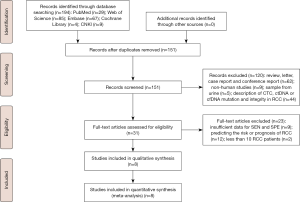
Table 1
First author, year | Country | Study type | Control type | No. of RCC/BD/HC | Timing sample | Sample source | Detection methods | Assay indicators | Cutoff value | SEN (%) | SPE (%) | PLR | NLR | TP | FP | FN | TN |
---|---|---|---|---|---|---|---|---|---|---|---|---|---|---|---|---|---|
Kim, 2019 | Korea | Retrospective | HC | 40/–/39 | Preoperative | Serum | ELISA | APEX1 | 0.202 ng/mL | 82.5 | 97.4 | 31.73 | 0.18 | 33 | 1 | 7 | 38 |
Yamamoto, 2018 | Japan | NA | HC | 92/–/41 | Pre-treatment | Plasma | RT-qPCR | cfDNA | 2,876 copies/mL | 63.0 | 78.1 | 2.88 | 0.47 | 58 | 9 | 34 | 32 |
Skrypkina, 2016 | Ukraine | NA | HC | 27/–/15 | Preoperative | Plasma | MSP | Methylation (LRRC3B) | NA | 74.1 | 66.7 | 2.23 | 0.39 | 20 | 5 | 7 | 10 |
Methylation (RASSF1) | NA | 62.9 | 93.3 | 9.39 | 0.40 | 17 | 1 | 10 | 14 | ||||||||
Methylation (FHIT1) | NA | 55.6 | 100.0 | Infinite | 0.44 | 15 | 0 | 12 | 15 | ||||||||
Methylation (APC) | NA | 51.9 | 93.3 | 7.75 | 0.52 | 14 | 1 | 13 | 14 | ||||||||
Lu, 2015 | Germany | Retrospective | HC | 145/–/40 | Preoperative | Plasma | RT-qPCR | cfDNA | 20.5 ng/mL | 70.0 | 88.0 | 5.83 | 0.34 | 102 | 5 | 43 | 35 |
Hauser, 2013 | Germany | Prospective | HC | 35/–/54 | Preoperative | Serum | MSP | Methylation (APC) | 0.37 | 54.3 | 90.7 | 5.84 | 0.50 | 19 | 5 | 16 | 49 |
Methylation (RASSF1A) | 0.09 | 22.9 | 98.2 | 12.72 | 0.79 | 8 | 1 | 27 | 53 | ||||||||
Methylation (PTGS2) | 0.47 | 22.9 | 96.3 | 6.19 | 0.80 | 8 | 2 | 27 | 52 | ||||||||
Methylation (RAR-B) | 0.19 | 40.0 | 85.2 | 2.70 | 0.70 | 14 | 8 | 21 | 46 | ||||||||
Martino, 2012 | Austria | Prospective | Benign renal disease | 157/43/– | Preoperative | Serum | RT-qPCR | Methylation (VHL) | NA | 50.3 | 90.7 | 5.41 | 0.55 | 79 | 4 | 78 | 39 |
Methylation (RASSF1A) | NA | 45.9 | 93.0 | 6.56 | 0.58 | 72 | 3 | 85 | 40 | ||||||||
Hauser, 2010 | Germany | Prospective | HC | 35/–/54 | Preoperative | Serum | RT-qPCR | cfDNA† | 1.03 ng/mL | 68.6 | 70.4 | 2.32 | 0.45 | 24 | 16 | 11 | 38 |
cfDNA¶ | 1.70 ng/mL | 57.0 | 81.0 | 3.00 | 0.53 | 20 | 10 | 15 | 44 | ||||||||
Perego, 2008 | Italy | NA | HC | 54/–/41 | Preoperative | Plasma | Real-time PCR | cfDNA | 6.2 ng/mL | 63.0 | 97.6 | 25.81 | 0.38 | 34 | 1 | 20 | 40 |
†, sample was derived from apoptotic cells; ¶, sample was derived from necrotic cells. No. of RCC/BD/HC, number of renal cell carcinoma /benign renal diseases/ healthy controls; SEN, sensitivity; SPE, specificity; TP, true positive; TN, true negative; FP, false positive; FN, false negative; PLR, positive likelihood ratio; NLR, negative likelihood ratio; NA, not applicable; ELISA, enzyme-linked immunosorbent assay; RT-qPCR, real-time quantitative polymerase chain reaction; MSP, methylation-specific polymerase chain reaction; APEX1, apurinic/apyrimidinic endonuclease 1/redox effector factor 1; APC, adenomatosis-polyposis-coli gene; RAR-B, Retinoid-acid receptor-beta gene; RASSF1A, Ras association domain family 1 isoform A; LRRC3B, leucine-rich repeats containing 3B; VHL, Von Hippel-Lindau; FHIT, fragile histidine triad; PTGS2, Prostaglandin-endoperoxide synthase 2.
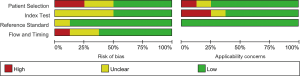
Diagnostic accuracy
Pooled SEN and SPE, PLR and NLR, and DOR as well as AUC in the SROC curve are applied for estimating the diagnostic performance in our diagnostic meta-analysis (2,26) (Table 2). The overall SEN and SPE of circulating cfDNA assays, to differentiate RCC cases from non-tumor controls, were 0.56 (95% CI, 0.53–0.59) and 0.89 (95% CI, 0.86–0.91) (Figure 3A,B). The overall DOR, PLR and NLR were 9.87 (95% CI, 6.59–14.77), 4.51 (95% CI, 3.15–6.47) and 0.50 (95% CI, 0.43–0.60). To further estimate how methodology influenced the diagnostic efficiency, we also explored two subgroups that focused on quantitative (assessing cfDNA levels) and qualitative (detecting single-gene methylation aberrations) methodologies.
Table 2
Group | SEN (95% CI) | SPE (95% CI) | PLR (95% CI) | NLR (95% CI) | DOR (95% CI) | AUC (95% CI) |
---|---|---|---|---|---|---|
Overall group | 0.56 (0.53–0.59) | 0.89 (0.86–0.91) | 4.51 (3.15–6.47) | 0.50 (0.43–0.60) | 9.87 (6.59–14.77) | 0.775 |
Quantitative analysis | 0.59 (0.55–0.63) | 0.88 (0.85–0.91) | 4.42 (2.81–6.96) | 0.50 (0.38–0.65) | 9.98 (5.64–17.69) | 0.7843 |
Qualitative analysis | 0.47 (0.43–0.52) | 0.92 (0.88–0.94) | 4.45 (3.03–6.54) | 0.60 (0.51–0.69) | 8.83 (5.64–13.84) | 0.774 |
SEN, sensitivity; SPE, specificity; RCC, Renal cell carcinoma; cfDNA, cell-free DNA; PLR, positive likelihood ratio; NLR, negative likelihood ratio; DOR, diagnostic odds ratio; AUC, the area under the curve; 95% CI, 95% confidence interval.
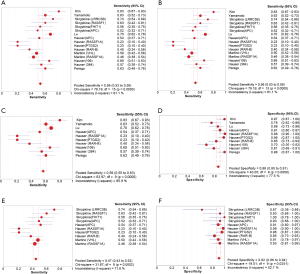
The pooled results from six publications associated with quantitative cfDNA to detect RCC revealed that the SEN and SPE were 0.59 (95% CI, 0.55–0.63) and 0.88 (95% CI, 0.85–0.91) (Figure 3C,D). PLR and NLR was 4.42 (95% CI, 2.81–6.96) and 0.50 (95% CI, 0.38–0.65). Additionally, the estimates of SEN and SPE of the three qualitative analysis of circulating cfDNA for RCC diagnosis were 0.47 (95% CI, 0.43–0.52) and 0.92 (95% CI, 0.88–0.94) (Figure 3E,F). The value for PLR and NLR was 4.45 (95% CI, 3.03–6.54) and 0.60 (95% CI, 0.51–0.69).
The AUC value in the overall group was 0.775 (Figure 4A), indicating a moderate overall accuracy. Specifically, DOR and AUC in the quantitative group was 9.98 (95% CI, 5.64–17.69) and 0.784 (Figure 4B), highlighting the moderately discriminatory power of quantitative cfDNA assays between non-RCC and renal malignancies. The DOR and AUC value in the qualitative group was 8.83 (95% CI, 5.64–13.84) and 0.774 (Figure 4C).
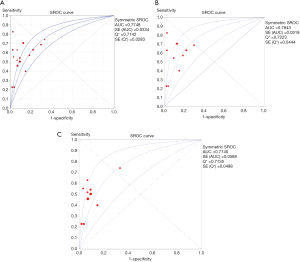
Heterogeneity and meta-regression analysis
A significant heterogeneity existed in all eight studies (SEN: I2=81.1%, P=0.000; SPE: I2=70.5%, P=0.000). The Spearman correlation coefficient was 0.343 with a P value of 0.194, revealing that the heterogeneity was potentially caused by non-threshold effect. A heterogeneity derived from non-threshold effects was also presented in the quantitative analysis subgroup (SEN: I2=85.9%, P=0.000; SPE: I2=77.5%, P=0.000; Spearman correlation coefficient=0.343, P=0.194). There was also significant heterogeneity resulted from non-threshold effects in the qualitative analysis subgroup (SEN: I2=71.6%, P=0.002; SPE: I2=52.7%, P=0.0251; Spearman correlation coefficient=0.329, P=0.353). Thus, regardless of the parameter applied, significant heterogeneity caused by non-threshold effects was revealed in above publications.
We further conducted a meta-regression analysis to reveal the sources of the heterogeneity in our meta-analysis. We evaluated primary parameters of all publications, including “publication year (recent 5 years or >5 years)”, “region (Europe or others)”, “individuals number (≥100 or <100 cases)”, control type (HC or BD; only for the qualitative analysis subgroup), “sampling source (plasma or serum)”, and “assay methods (RT-qPCR or the others for the quantitative analysis subgroup; MSP or the others for the qualitative analysis subgroup)”. As revealed in Table 3, the “publication year” parameter was a potential source of heterogeneity in the quantitative analysis subgroup (P=0.048). The “control type” covariate might generate primary heterogeneity for the qualitative subgroup (P=0.0352).
Table 3
Analysis | Covariates | Coefficient | SE | P value | RDOR (95% CI) |
---|---|---|---|---|---|
Quantitative analysis | Year | 4.306 | 1.3303 | 0.048 | 74.13 (1.07–5,112.12) |
Region | 0.945 | 0.6774 | 0.2572 | 2.57 (0.30–22.23) | |
Sample size | −5.485 | 1.7506 | 0.0519 | 0 (0.00–1.09) | |
Sample source | 2.202 | 1.1062 | 0.1406 | 9.04 (0.27–305.59) | |
Assay method | 0.137 | 0.7427 | 0.8652 | 1.15 (0.11–12.19) | |
Qualitative analysis | Year | 1.38 | 0.8796 | 0.1553 | 3.97 (0.52–30.21) |
Region | 0.483 | 0.6365 | 0.4698 | 1.62 (0.37–7.03) | |
Sample size | 0.259 | 0.9246 | 0.7864 | 1.3 (0.15–10.93) | |
Sample source | −0.11 | 0.6695 | 0.8739 | 0.9 (0.19–4.20) | |
Assay method | −0.226 | 0.8523 | 0.7979 | 0.8 (0.11–5.70) | |
Control type | −1.37 | 0.5411 | 0.0352 | 0.25 (0.07–0.89) |
RCC, renal cell carcinoma; cfDNA, cell-free DNA; RDOR, relatively diagnostic odds ratio; 95% CI, 95% confidence interval.
Publication bias
The Deek’s funnel plot was applied for evaluating the publication biases in this meta-analysis. No significant publication bias was revealed among all eight publications to distinguish RCC patients from control individuals (Figure 5A, P=0.596). There was no obvious publication bias in the quantitative analysis group (Figure 5B, P=0.774) and qualitative analysis group (Figure 5C, P=0.351).
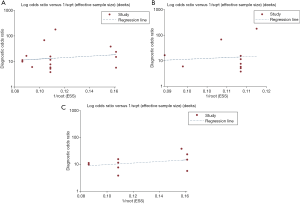
Discussion
RCC is a usual and fatal disease in America where it occupies 4% of all adult malignancies (4). Currently, there were no dependable biomarkers to screen and diagnosis RCC in a minimally invasive manner (5,28). Recently, liquid biopsy-based assays are developing into potential supplementary tools to assist radiological examinations and tissue biopsies (29). Circulating cfDNA can be a universal signature of malignancy status and is advantageous to diagnose and monitor several tumors (2,30,31). For example, enhanced cfDNA levels were revealed in individuals with mammary cancer, gastric cancer, ovary cancer, colon cancer and prostate cancer (32-37). Compared with conventional tumor biomarker carcinoembryonic antigen or carbohydrate antigen 19-9 to screen colon cancer, the diagnostic efficiency of circulating cfDNA level was more precise (38). Additionally, certain tumor-specific alterations, including methylation, were revealed in circulating cfDNA (32,39). Accordingly, we established the first diagnostic meta-analysis for estimating the diagnostic efficiency of cfDNA tests for RCC detection and diagnosis.
In our findings, the SEN and AUC of quantitative analysis were slightly greater than those of the overall group (0.59 vs. 0.56 for SEN; 0.7843 vs. 0.775 for AUC). Nevertheless, the qualitative analysis subgroup yielded a higher SPE compared with did quantitative analysis subgroup (0.92 vs. 0.88). This was potentially because certain methylated genetic loci which was detected were specifically expressed in RCC cases. The DOR of overall group to discriminate RCC cases from control individuals was 9.87, while the DOR of the qualitative analysis subgroup was slightly lower at 8.83. The DOR was further increased to 9.98 with the quantitative cfDNA detection. Three groups exhibited a similar PLR value (4.51 for the overall group; 4.42 for the quantitative analysis subgroup; 4.45 for the qualitative analysis subgroup), suggesting that RCC patients had an approximately 4.5-fold greater probability of being cfDNA detection-positive in contrast to healthy volunteers or individuals with benign renal diseases. The qualitative analysis subgroup had an NLR value of 0.60, which was higher than an NLR value of 0.50 in the overall group and the quantitative analysis subgroup. Thus, the possibility for individuals with negative qualitative cfDNA assay results to have RCC was approximately 60%. Above findings indicate that circulating cfDNA assessments should not be applied independently for a biomarker to diagnosis RCC, which can potentially develop into an auxiliary means in a combination with cytological or histological detection of kidney tissue to make precise diagnosis.
We also took the impact of publication bias into consideration. The results would be biased on the condition that positive results were prone to be published (2). Nevertheless, the publication bias was not revealed through Deek’s funnel plot asymmetry test. Additionally, we conducted meta-regression analysis and demonstrated that the parameters of “publication year” and “control type” potentially generated primary heterogeneity in the quantitative cfDNA detection and the qualitative cfDNA detection, respectively.
The conclusions concerning the effectiveness of circulating cfDNA assays on RCC screen and diagnosis were usually inconsistent, potentially caused by different assay methods, non-uniform source of sample and variable analytical factors. Though the SEN values based on eight publications in our report showed imperfect robustness, newly-emerging cfDNA assays are strongly recommended as an auxiliary implement combined with traditional cytological and histological tests for RCC diagnosis. Certain limitations should be discussed in our present study. Firstly, we failed to estimate all sources of heterogeneity. Additional crucial parameters were unavailable from the included publications, such as tumor size, histological subtype and TNM stages (2,40,41). Secondly, as only eight publications were incorporated into our meta-analysis and methylation genes are various in each study, we cannot conduct the qualitative subgroup analyses of a particular single-gene methylation alteration (such as APC, APEX1 or RASSF1A) to evaluate the diagnostic value of such gene for RCC. Furthermore, a few publications were incorporated into the qualitative subgroup following a thorough literature search, which made the findings less robust. Therefore, further standardization and longitudinal studies with larger sample size are required to favor the conclusions of our meta-analysis.
Conclusions
In conclusion, the first diagnostic meta-analysis was established for evaluating the overall accuracy of circulating cfDNA assays in RCC screening and diagnosis. Circulating cfDNA assay may be a promising biomarker to distinguish RCC patients from healthy individuals, with a moderate diagnostic accuracy. However, the quantitative and qualitative cfDNA assays should not be utilized independently for RCC diagnosis on account of lack of robustness, which can be considered as supplementary means combined with cytological or histological examinations to improve the diagnostic efficiency for the RCC detection.
Acknowledgments
We would like to thank Weiping Xia for his help in polishing the language of our paper.
Funding: This work was supported by
Footnote
Reporting Checklist: The authors have completed the PRISMA reporting checklist. Available at http://dx.doi.org/10.21037/tcr-20-3448
Conflicts of Interest: All authors have completed the ICMJE uniform disclosure form (available at http://dx.doi.org/10.21037/tcr-20-3448). The authors have no conflicts of interest to declare.
Ethical Statement: The authors are accountable for all aspects of the work in ensuring that questions related to the accuracy or integrity of any part of the work are appropriately investigated and resolved.
Open Access Statement: This is an Open Access article distributed in accordance with the Creative Commons Attribution-NonCommercial-NoDerivs 4.0 International License (CC BY-NC-ND 4.0), which permits the non-commercial replication and distribution of the article with the strict proviso that no changes or edits are made and the original work is properly cited (including links to both the formal publication through the relevant DOI and the license). See: https://creativecommons.org/licenses/by-nc-nd/4.0/.
References
- Siegel RL, Miller KD, Jemal A. Cancer statistics, 2018. CA Cancer J Clin 2018;68:7-30. [Crossref] [PubMed]
- Zhang Z, Chen P, Xie H, et al. Using circulating tumor DNA as a novel biomarker to screen and diagnose hepatocellular carcinoma: A systematic review and meta-analysis. Cancer Med 2020;9:1349-64. [Crossref] [PubMed]
- Zhang Z, Tang H, Chen P, et al. Demystifying the manipulation of host immunity, metabolism, and extraintestinal tumors by the gut microbiome. Signal Transduct Target Ther 2019;4:41. [Crossref] [PubMed]
- Posadas EM, Limvorasak S, Figlin RA. Targeted therapies for renal cell carcinoma. Nat Rev Nephrol 2017;13:496-511. [Crossref] [PubMed]
- Wang Z, Zhang B, Chen Z, et al. The long noncoding RNA myocardial infarction-associated transcript modulates the epithelial-mesenchymal transition in renal interstitial fibrosis. Life Sci 2020;241:117187. [Crossref] [PubMed]
- Capitanio U, Bensalah K, Bex A, et al. Epidemiology of Renal Cell Carcinoma. Eur Urol 2019;75:74-84. [Crossref] [PubMed]
- Gupta K, Miller JD, Li JZ, et al. Epidemiologic and socioeconomic burden of metastatic renal cell carcinoma (mRCC): a literature review. Cancer Treat Rev 2008;34:193-205. [Crossref] [PubMed]
- Rossi SH, Prezzi D, Kelly-Morland C, et al. Imaging for the diagnosis and response assessment of renal tumours. World J Urol 2018;36:1927-42. [Crossref] [PubMed]
- Maher ER. Hereditary renal cell carcinoma syndromes: diagnosis, surveillance and management. World J Urol 2018;36:1891-8. [Crossref] [PubMed]
- Rossi SH, Klatte T, Usher-Smith J, et al. Epidemiology and screening for renal cancer. World J Urol 2018;36:1341-53. [Crossref] [PubMed]
- Cimadamore A, Gasparrini S, Massari F, et al. Emerging Molecular Technologies in Renal Cell Carcinoma: Liquid Biopsy. Cancers (Basel) 2019;11:196. [Crossref] [PubMed]
- Giacona MB, Ruben GC, Iczkowski KA, et al. Cell-free DNA in human blood plasma: length measurements in patients with pancreatic cancer and healthy controls. Pancreas 1998;17:89-97. [Crossref] [PubMed]
- Jahr S, Hentze H, Englisch S, et al. DNA fragments in the blood plasma of cancer patients: quantitations and evidence for their origin from apoptotic and necrotic cells. Cancer Res 2001;61:1659-65. [PubMed]
- Leon SA, Shapiro B, Sklaroff DM, et al. Free DNA in the serum of cancer patients and the effect of therapy. Cancer Res 1977;37:646-50. [PubMed]
- Hauser S, Zahalka T, Ellinger J, et al. Cell-free circulating DNA: Diagnostic value in patients with renal cell cancer. Anticancer Res 2010;30:2785-9. [PubMed]
- Lu H, Busch J, Jung M, et al. Diagnostic and prognostic potential of circulating cell-free genomic and mitochondrial DNA fragments in clear cell renal cell carcinoma patients. Clin Chim Acta 2016;452:109-19. [Crossref] [PubMed]
- Perego RA, Corizzato M, Brambilla P, et al. Concentration and microsatellite status of plasma DNA for monitoring patients with renal carcinoma. European Journal of Cancer 2008;44:1039-47. [Crossref] [PubMed]
- Yamamoto Y, Uemura M, Nakano K, et al. Increased level and fragmentation of plasma circulating cell-free DNA are diagnostic and prognostic markers for renal cell carcinoma. Oncotarget 2018;9:20467-75. [Crossref] [PubMed]
- Kim JM, Yeo MK, Lim JS, et al. APEX1 Expression as a Potential Diagnostic Biomarker of Clear Cell Renal Cell Carcinoma and Hepatobiliary Carcinomas. J Clin Med 2019;8:1151. [Crossref] [PubMed]
- Skrypkina I, Tsyba L, Onyshchenko K, et al. Concentration and Methylation of Cell-Free DNA from Blood Plasma as Diagnostic Markers of Renal Cancer. Dis Markers 2016;2016:3693096. [Crossref] [PubMed]
- de Martino M, Klatte T, Haitel A, et al. Serum Cell-Free DNA in Renal Cell Carcinoma: A Diagnostic and Prognostic Marker. Cancer 2012;118:82-90. [Crossref] [PubMed]
- Hauser S, Zahalka T, Fechner G, et al. Serum DNA hypermethylation in patients with kidney cancer: results of a prospective study. Anticancer Res 2013;33:4651-6. [PubMed]
- Glas AS, Lijmer JG, Prins MH, et al. The diagnostic odds ratio: a single indicator of test performance. J Clin Epidemiol 2003;56:1129-35. [Crossref] [PubMed]
- Jaeschke R, Guyatt GH, Sackett DL. Users’ guides to the medical literature. III. How to use an article about a diagnostic test. B. What are the results and will they help me in caring for my patients? The Evidence-Based Medicine Working Group. JAMA 1994;271:703-7. [Crossref] [PubMed]
- Reitsma JB, Glas AS, Rutjes AW, et al. Bivariate analysis of sensitivity and specificity produces informative summary measures in diagnostic reviews. J Clin Epidemiol 2005;58:982-90. [Crossref] [PubMed]
- Moses LE, Shapiro D, Littenberg B. Combining independent studies of a diagnostic test into a summary ROC curve: data-analytic approaches and some additional considerations. Stat Med 1993;12:1293-316. [Crossref] [PubMed]
- Thompson SG, Higgins JP. How should meta-regression analyses be undertaken and interpreted? Stat Med 2002;21:1559-73. [Crossref] [PubMed]
- Wang Z, Chen Z, Li B, et al. Curcumin attenuates renal interstitial fibrosis of obstructive nephropathy by suppressing epithelial-mesenchymal transition through inhibition of the TLR4/NF-κB and PI3K/AKT signalling pathways. Pharm Biol 2020;58:828-37. [Crossref] [PubMed]
- Heitzer E, Ulz P, Geigl JB. Circulating tumor DNA as a liquid biopsy for cancer. Clin Chem 2015;61:112-23. [Crossref] [PubMed]
- Sozzi G, Conte D, Mariani L, et al. Analysis of circulating tumor DNA in plasma at diagnosis and during follow-up of lung cancer patients. Cancer Res 2001;61:4675-8. [PubMed]
- Aarthy R, Mani S, Velusami S, et al. Role of Circulating Cell-Free DNA in Cancers. Mol Diagn Ther 2015;19:339-50. [Crossref] [PubMed]
- Schwarzenbach H, Hoon DS, Pantel K. Cell-free nucleic acids as biomarkers in cancer patients. Nat Rev Cancer 2011;11:426-37. [Crossref] [PubMed]
- Roth C, Pantel K, Muller V, et al. Apoptosis-related deregulation of proteolytic activities and high serum levels of circulating nucleosomes and DNA in blood correlate with breast cancer progression. BMC Cancer 2011;11:4. [Crossref] [PubMed]
- Sai S, Ichikawa D, Tomita H, et al. Quantification of plasma cell-free DNA in patients with gastric cancer. Anticancer Res 2007;27:2747-51. [PubMed]
- Wu TL, Zhang D, Chia JH, et al. Cell-free DNA: measurement in various carcinomas and establishment of normal reference range. Clin Chim Acta 2002;321:77-87. [Crossref] [PubMed]
- Bettegowda C, Sausen M, Leary RJ, et al. Detection of circulating tumor DNA in early- and late-stage human malignancies. Sci Transl Med 2014;6:224ra24. [Crossref] [PubMed]
- Kienel A, Porres D, Heidenreich A, et al. cfDNA as a Prognostic Marker of Response to Taxane Based Chemotherapy in Patients with Prostate Cancer. J Urol 2015;194:966-71. [Crossref] [PubMed]
- Qi J, Qian C, Shi W, et al. Alu-based cell-free DNA: a potential complementary biomarker for diagnosis of colorectal cancer. Clin Biochem 2013;46:64-9. [Crossref] [PubMed]
- Jung K, Fleischhacker M, Rabien A. Cell-free DNA in the blood as a solid tumor biomarker--a critical appraisal of the literature. Clin Chim Acta 2010;411:1611-24. [Crossref] [PubMed]
- Chen P, Zhang Z, Chen X. Overexpression of PKMYT1 Facilitates Tumor Development and Is Correlated with Poor Prognosis in Clear Cell Renal Cell Carcinoma. Med Sci Monit 2020;26:e926755. [Crossref] [PubMed]
- Liu S, Lai W, Shi Y, et al. Annotation and cluster analysis of long noncoding RNA linked to male sex and estrogen in cancers. NPJ Precis Oncol 2020;4:5. [Crossref]