Systemic immune microenvironment and regulatory network analysis in patients with lung adenocarcinoma
Introduction
Lung cancer is a relatively common malignancy, and global cancer statistics indicate that the incidence is increasing yearly (1,2). In the United States in 2019 there were approximately 116,000 newly diagnosed lung cancers in females and 112,000 in males (1). However, with advances of medical technology, delay-adjusted incidence rates in males decreased 2.9% from 2008 to 2015, and decreased 1.5% in females from 2006 to 2015 (3). Mortality rates also decreased for lung cancer patients: from 2012 to 2016 the mortality rate decreased 4.3% for males and 3.1% for females (1). In countries with more advanced healthcare and education systems, there has been a decline in the incidence of new lung cancer cases of about 3% annually from 2008 through 2016. In addition, data has suggested that lung cancer-specific survival has improved in large part due to advances in immune-targeted treatments (4).
The use of immunotherapies, including those targeted at anti-cytotoxic T-lymphocyte associated protein 4 (CTLA-4) and programmed death-1 (PD-1) and its ligands (PD-L1 and PD-L2), has become an important treatment for many solid tumors, including lung cancer. Nishio et al. demonstrated that treatment with pembrolizumab, a humanized monoclonal antibody against PD-1, was well-tolerated and improved progression-free survival (PFS) and overall survival (OS) in Japanese patients (5). Quoix et al. reported that treatment with TG4010, an immunosuppressive agent targeting CD16, CD56, and CD69 triple-positive activated lymphocytes (TrPAL), combined with chemotherapy, improved PFS of patients with advanced non-small cell lung cancer (NSCLC) (6). Hui et al. also reported that pembrolizumab improved long-term OS in patients with advanced NSCLC (7).
Emerging evidence has revealed a close relation between immune system dysfunction and NSCLC, and immune checkpoint inhibitors are considered as a second-line treatment option for patients who have failed chemotherapy (8). However, the significant heterogeneity had revealed between the lung adenocarcinoma (LUAD) and lung squamous cell carcinoma (LUSC) in term of their genomic and clinical attributes. For example, the subtype and prediction model constructed based on gene expression can accurately assess the response to the checkpoint of CD274 (programmed death ligand 1) in patients with LUSC and LUAD (9). Studies of the effectiveness of immunotherapies have shown the importance of interactions between the tumor microenvironment and cancer cell heterogeneity (10). Identifying the truly cancer-specific biomarkers or neoantigens can help to elucidate the immune adaptation, cancer cells escape, and thus is important to develop the individualized therapy. Especially, LUAD can be considered as a treatable cancer if targeting to the driver biomarkers (3).
Thus, this study applied a complex bioinformatics analysis to explore the hub regulators and immune network, and to further elucidate the molecular mechanisms of LUAD immune regulation.
Methods
Analysis of LUAD immunological microenvironment features
In order to determine tumor purity and the degree of immune infiltration, the ESTIMATE algorithm (https://bioinformatics.mdanderson.org/estimate/) and gene expression signatures was used (11). The algorithm calculates a stromal and immune score of each tissue sample which predicts tumor stromal and immune infiltration, and then produces the ESTIMATE score, a comprehensive score reflecting the tumor microenvironment, which reflects the tumor purity (11). The RNA-seq fragments per kilobase of exon per million fragments mapped reads (FPKM) from The Cancer Genome Atlas (TCGA)-LUAD project were downloaded using the R Package “TCGA biolinks” (12). Raw Affymetrix microarray data of GSE31210 was downloaded from Gene Expression Omnibus (GEO; http://www.ncbi.nlm.nih.gov/geo/) (13). The download included data of 246 tumor samples, including 11 ALK fusion tumor samples, 20 KRAS mutation samples, 127 epidermal growth factor receptor (EGFR) mutation samples, and 68 EGFR/KRAS/ALK- tumor samples. In addition, the GSE31210 dataset matching the GPL570-Affymetrix Human Genome U133 plus 2.0 Array platform (Affymetrix, Santa Clara, CA, USA) was also downloaded. For each sample, the stromal score, immune score, and TCGA-LUAD and GSE31210 estimated scores were calculated using the ESTIMATE algorithm in the “ESTIMATE” R package. Kaplan-Meier survival analysis using the stromal, immune, and ESTIMATE scores was performed using the “survminer” package, and an optimal cutoff point for each variable was calculated using the “maxstat” algorithm. The study was conducted in accordance with the Declaration of Helsinki (as revised in 2013).
Data processing and identification of co-differentially expressed genes
Based on the cutoff point of ESTIMATE score, TCGA-LUAD and GSE31210 tumor samples were divided into a higher risk group and a lower risk group. In order to facilitate comparative analysis, FPKM data were transformed into transcripts per kilobase million (TPM) (14,15), using the formula:
where i is total exon fragments and j is mapped reads. Differential expression analysis of TPM normalized TCGA-LUAD data was performed using the “DESeq2” R package (16). Raw GSE31210 data were subjected to background correction, quantile normalization, and Log2 transformation of the expression level, then a linear model for microarray analyses (LIMMA) algorithm was used to identify differential expressed genes (DEGs) (17). Importantly, the raw data were background-adjusted, normalized, and log-transformed using the robust multi-array average (RMA) method, and missing values in the microarray dataset were calculated using the K-Nearest Neighbor (KNN) classifier method. For multiple probes mapped to the same gene symbol, the average value was considered the gene expression value (17,18). For statistical effectiveness, the P value of the false discovery rate (FDR) was adjusted using the Benjamini-Hochberg method, and subsequently used to detect the gene expression fold-change (FC). In this study, genes satisfying the standard criterion of |log2FC| >1.5 and adjusted P value <0.05 in both the TCGA-LUAD project and microarray dataset were consider DEGs (16-18). Venn diagrams were drawn, and intersections of DEGs from the TCGA-LUAD project and microarray dataset were considered co-DEGs.
Gene function enrichment analysis
Gene Ontology (GO) functional enrichment analysis was performed using “GO plot”, “Annotation Hub”, and “Cluster Profiler” of the R package (19,20). These components of R package allow extraction of biological process (BP), molecular function (MF), and cellular composition (CC) among DEGs from large lists of genomic studies and databases (19,20). GO terms associated with a value of P<0.05 were considered to be significantly enriched.
After the identification of co-DEGs, extensive functional enrichment analysis was performed using the Metascape online database tool (http://metascape.org/gp/index.html#/main/step1) and Kyoto Encyclopedia of Genes and Genomes (KEGG) pathway networks were constructed using cutoff criteria of P<0.05 and enrichment score (ES) >3.0 (21). Cytoscape software (V3.5.1; http://cytoscape.org/) was used to visualize and evaluate interactions of the KEGG pathway networks (22).
Identification of candidate biomarkers
After extracting enriched genes from significant KEGG pathways, an automatic Kaplan-Meier survival analysis based on the “maxstat” algorithm was performed to visualize and evaluate prognostic effects and identifying the hub gene for the subsequent analysis. Genes enriched in KEGG pathway networks with a survival analytical P<0.05 were considered candidate genes. Univariate and multivariate Cox regression analyses were used to examine the associations of candidate genes combined with clinical and pathological factors (stromal score, immune score, ESTIMATE score, pathological stage, smoking history, primary tumor, lymph node status, distant metastasis status) and OS of patients from the TCGA-LUAD database. Different from the Kaplan-Meier survival log-rank test, Cox regression analysis allows predicting the effect (hazard) of risk variables as follows: H (t) = H0 (t) × exp(b1X1 + b2X2 + … + bkXk). Here, X1 ... Xk are considered as predictor variables, and H0 (t) is the baseline effect at the time point t. Factor with a value of P<0.05 were defined as significant risk factors.
Immunocyte infiltration and regulatory network analysis
To characterize the prognosis associated with LUAD immune cell subsets, CIBERSORT estimate software was used to quantify the immune cell fractions of the gene expression matrices derived from LUAD samples. The advantage of the CIBERSORT algorithm is that is uses the ν-support vector regression (ν-SVR) method to reduce data noise from high-dimensional genomic matrices owing to multicollinearity, and thus accurately captures the infiltration of various immune cell subtypes (23). Subsequently, an automatic Kaplan-Meier survival analysis of immune cell subtypes was constructed to detect the cell populations significantly associated with survival and prognosis. To increase the credibility of the results, analyses were performed using immune cell subsets from TCGA-LUAD and GEO-LUAD tissue expression profiles, and common immune cell subtypes with a significant prognostic value were extracted. Spearman correlation analysis was used to examine correlations of immune cell subtypes, and P values were calculated. Based on the correlation values, immune cell association networks were constructed using a graph algorithm (Igraph; https://github.com/igraph).
Gene set enrichment analysis (GSEA) and detection of tumor mutation burden (TMD)
GSEA, based on Molecular Signature Database gene sets, was performed to identify hub gene correlated regulatory pathways involved in LUAD pathogenesis. Here, GSEA analysis stratified by the cut-point of each hub gene expression level calculated by the “maxstat” algorithm. Based on weighted Kolmogorov-Smirnov-like statistics, ES and normalized enrichment scores (NES) were calculated to reflect the correlation of gene sets with phenotype (24).
To gain further insight into the TMB involved in LUAD pathogenesis in response to hub genes expression, somatic mutation analysis was performed based on the Wilcoxon signed-rank test. The somatic variants of TCGA-LUAD 567 tumor samples were obtained from “TCGA biolinks” as the raw mutation count. For estimates, the files were aligned to the genome of hg38 GRCh38 (25).
Validation of hub gene expression and clinical-pathological variables
The expression profiling of TCGA-LUAD with the different levels of the immune score, stromal score, and ESTIMATE score were used to validate the candidate biomarkers; the KRAS/EGFR mutation, pathological stage, and tissue differential expression. In addition, hub gene expression levels and corresponding clinical features associated with ALK fusion and KRAS/EGFR mutations were extracted from the GSE31210 dataset and included in further differential analysis.
Statistical analysis
To sum up the statistical methods being mentioned in previous analyses. No descriptive statistics were used. Kaplan-Meier survival analysis and log-rank test was used to compare the OS among subgroups stratified by a single variable. Univariate and multivariate Cox proportional hazards models were used to investigate factors associated with OS. All independent variables were entered into both univariate and multivariate models, and variables which were significant in both univariate and multivariate models were considered factors associated with OS, and associations were reported as hazard ratio (HR) and 95% confidence interval (CI). Pearson and Spearman correlation coefficients were calculated, and used to determine the degree of correlation between variables. For the GSEA analysis, ES, NES, FDR, and q-value were reported. The statistical significance level for all tests was set at a two-tailed value of P<0.05. All analyses were done using R version 3.5.2 statistical software.
Results
LUAD immunological microenvironment features
Kaplan-Meier survival analyses were performed using TCGA-LUAD and GEO-LUAD data for determination of LUAD microenvironment score (table available at: https://cdn.amegroups.cn/static/public/tcr-20-2275-1.pdf) (Figure S1). The results showed that immunological microenvironment features including the stromal score (TCGA: HR =0.65, P=0.003; GEO: HR =1.63, P=0.151), immune score (TCGA: HR =0.58, P<0.001; GEO: HR =1.96, P=0.048), and ESTIMATE score (TCGA: HR =0.65, P=0.003; GEO: HR =1.83, P=0.046) were positively correlated with a better prognosis of patients with LUAD (Figure 1A,B).
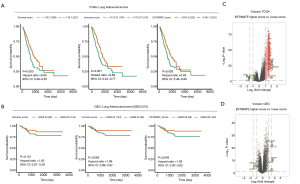
Differential microenvironment score-related differential gene detection
Based on the cutoff points using ESTIMATE score and prognostic information, and Linear Models for Microarray Data (LIMMA) powers differential expression analyses, 250 DEGs (7 down-regulated, 243 up-regulated) were identified in the comparison between higher ESTIMATE score and lower ESTIMATE score samples in TCGA-LUAD patients. In GEO-LUAD patients, 511 DEGs were identified (102 down-regulated, 409 up-regulated) (Figure 1C,D and table available online: https://cdn.amegroups.cn/static/public/tcr-20-2275-2.pdf).
DEG functional enrichment analysis
The GO enrichment analysis results of DEGs identified in the TCGA-LUAD group are as follows: (I) with respect to ESTIMATE score-related DEGs, the BP maps included T-cell activation (gene count =46; P=3.20e−27), leukocyte cell-cell adhesion (gene count =36; P=4.03e−25), and regulation of leukocyte cell-cell adhesion (gene count =33; P=2.20e−23). Based on the BP network, 3-block clusters of T-cell activation related to immune inflammatory response, leukocyte proliferation, and regulation of immune effectors processes were detected. (II) The MF maps of significantly enriched in immune microenvironment-related DEGs included chemokine activity (gene count =12; P=2.79e−13), chemokine receptor binding (gene count =13; P=5.76e−13), and signaling pattern recognition receptor activity (gene count =8; P=7.77e−08). (III) The CC maps of significantly enriched in immune microenvironment-related DEGs included the external side of the plasma membrane (gene count =44; P=3.78e−33), specific granule (gene count =14; P=3.10e−09), and collagen trimer (gene count = 8; P=5.54e−06) (Figure 2A,B,C and table available online: https://cdn.amegroups.cn/static/public/tcr-20-2275-3.pdf).
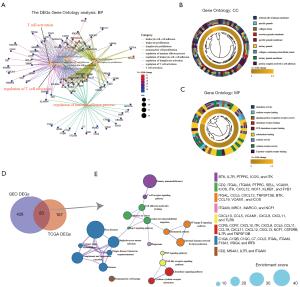
In summary, the DEGs analysis indicated that immune microenvironment-related DEGs are predominantly involved in the regulation of T-cell activation, chemokine activity, and immune effector dysfunction.
Identifying candidate biomarkers from immunological microenvironment feature
A total of 83 co-DEGs were identified in the TCGA-LUAD and GEO-LUAD groups (Figure 2D). Metascape analysis indicated that the primary areas of enrichment were immunodeficiency (gene count =4; P=8.0e−06), cell adhesion molecules (CAMs) (gene count =7; P=7.0e−07), NF-kappa B signaling pathway (gene count =6; P=2.0e−05), tumor necrosis factor (TNF) signaling pathway (gene count =3; P=0.002), and chemokine signaling pathway (gene count =12; P=1.0e−12) (Figure 2E). A visualization of the significant interactions among these pathways is shown in table available online: https://cdn.amegroups.cn/static/public/tcr-20-2275-4.pdf.
A total of 36 co-DEGs considered key regulatory genes were identified in the KEGG pathway enrichment network results (P<0.05 and ES >3.0). To access potential biomarkers targeting immunological microenvironment feature and their value for predicting survival LUAD, we performed Kaplan-Meier survival analysis using TCGA-LUAD data. Eight genes were found to be significantly correlated with prognosis: tyrosine-protein kinase (ITK; HR =1.34, P=0.046), neutrophil cytosol factor 1 (NCF1; HR =1.4, P=0.028), macrophage receptor (MARCO; HR =1.49, P=0.007), cytokine receptor common subunit beta (CSF2RB; HR =1.37, P=0.031), integrin alpha-L (ITGAL; HR =1.41, P=0.019), killer cell lectin-like receptor subfamily B member 1 (KLRB1; HR =1.39, P=0.026), chemokine ligands-11 (CCL11; HR =0.71, P=0.03), and v-set and immunoglobulin domain-containing protein-4 (VSIG4; HR =1.41, P=0.039) (Figure 3).
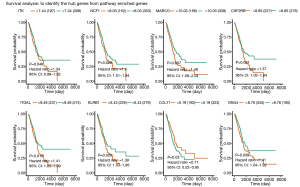
Univariate and multivariate Cox regression analysis was performed to identify variables associated with OS, the 8 candidate genes, and clinicopathological characteristics in the TCGA-LUAD group. Significant factors included pathologic stage (univariate HR =1.64, 95% CI: 1.40–1.92, P<0.01; multivariate HR =1.61, 95% CI: 1.04–2.49, P=0.03), pathological tumor stage (univariate HR =1.72, 95% CI: 1.39–2.13, P<0.01; multivariate HR =1.48, 95% CI: 1.10–1.89, P<0.01), ITGAL (univariate HR =0.80, 95% CI: 0.69–0.93, P<0.01; multivariate HR =0.59, 95% CI: 0.40–0.86, P=0.01), and KLRB1 (univariate HR =0.78, 95% CI: 0.69–0.89, P<0.01; multivariate HR =0.72, 95% CI: 0.58–0.90, P<0.01) (Table 1). As the hub genes, ITGAL higher expression was significantly correlated with poorer OS (HR =1.41; 95% CI: 1.05–1.89; P=0.019), as was KLRB1 higher expression (HR =1.39; 95% CI: 1.03–1.86; P=0.026) (Figure 3).
Table 1
Variable | Univariate regression | Multivariate regression | |||
---|---|---|---|---|---|
HR (95% CI) | P value | HR (95% CI) | P value | ||
Age | 0.95 (0.67–1.35) | 0.79 | 1.27 (0.87–1.84) | 0.22 | |
Pathological stage | 1.64 (1.40–1.92) | <0.01 | 1.61 (1.04–2.49) | 0.03 | |
Smoking history | 1.05 (0.90–1.23) | 0.50 | 1.09 (0.92–1.30) | 0.31 | |
Pathological metastasis | 2.00 (1.12–3.56) | 0.02 | 0.58 (0.20–1.72) | 0.32 | |
Pathological lymph node | 1.78 (1.46–2.18) | <0.01 | 1.15 (0.80–1.66) | 0.46 | |
Pathological stage | 1.72 (1.39–2.13) | <0.01 | 1.48 (1.10–1.89) | 0.01 | |
CCL11 | 1.00 (0.92–1.10) | 0.87 | 0.98 (0.86–1.11) | 0.76 | |
CSF2RB | 0.88 (0.77–1.01) | 0.07 | 0.78 (0.55–1.11) | 0.17 | |
ITGAL | 0.80 (0.69–0.93) | <0.01 | 0.59 (0.40–0.86) | 0.01 | |
ITK | 0.85 (0.75–0.96) | <0.01 | 1.35 (0.99–1.83) | 0.06 | |
KLRB1 | 0.78 (0.69–0.89) | <0.01 | 0.72 (0.58–0.90) | <0.01 | |
MARCO | 0.98 (0.90–1.06) | 0.65 | 1.14 (0.96–1.36) | 0.15 | |
NCF1 | 0.90 (0.80–1.01) | 0.10 | 1.22 (0.89–1.66) | 0.22 | |
VSIG4 | 0.97 (0.87–1.08) | 0.61 | 0.86 (0.65–1.13) | 0.28 |
LUAD, lung adenocarcinoma; HR, hazard ratio; CI, confidence interval.
Construction of the immune cell regulatory network
After filtering the relative values of immune cell infiltration, supervised clustering analysis was applied to all TCGA-LUAD and GEO-LUAD samples. The comprehensive landscape interactions of tumor-immune regulatory networks involved in OS and Pearson correlation analysis coefficient are calculated according to the HR and weighted coefficient index from the comprehensive evaluation. The distinct subtypes involved in ESTIMATE score and landscape interactions are illustrated in plots for the TCGA-LUAD group (Figure 4A,B) and GEO-LUAD group (Figure 4C,D). Based on the effects on the OS of TCGA-LUAD and GEO-LUAD patients, key regulators were found to be infiltration subtypes of mast T-cells resting (TCGA-LUAD HR =0.66, 95% CI: 0.42–1.01, P=0.038; GEO-LUAD HR =0.34, 95% CI: 0.17–0.68, P=0.008), and activated T-cells (CD4 memory) (TCGA-LUAD HR =1.55, 95% CI: 1.05–2.29, P=0.026; GEO-LUAD HR =2.3, 95% CI: 1.17–4.52, P=0.017) (Figure 4E,F; Figure S1). In addition, immune infiltration subtypes mast cells (masT-cells) resting (TCGA-LUAD HR =0.01, 95% CI: 0.01–3.04, log-rank P=0.01, weighted coefficient index =1.97; GEO-LUAD HR =1.88e−07, 95% CI: 5.07e−13 to 0.07, log-rank P=1.79e−05, weighted coefficient index =4.75), and activated T-cells (CD4 memory) (TCGA-LUAD HR =896.32, 95% CI: 0.59–1,372,396.37, log-rank P<0.01, weighted coefficient index =4.16; GEO-LUAD HR =0.40, 95% CI: 0.01–5945.07, log-rank P=8.52e−05, weighted coefficient index =4.07) were also found to have important effects on the LUAD-related immune cells interaction network (table available online: https://cdn.amegroups.cn/static/public/tcr-20-2275-5.pdf).
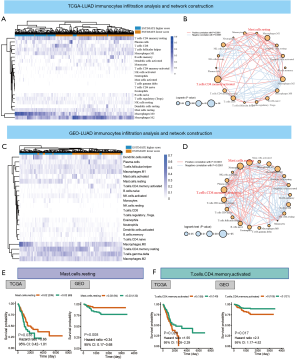
GSEA and detection of TMB
GSEA of low- and high-expression levels of ITGAL and KLRB1 revealed the following: (I) the maps of the T-cell receptor signaling pathway (ES =0.75, NES =2.19, FDR q-value =0.013) and B-cell receptor signaling pathway (ES =0.7, NES =2.1, FDR q-value =0.011) were correlated with higher ITGAL expression, while the citrate cycle TCA cycle (ES =−0.62, NES =−2.05, FDR q-value =0.023) and RNA polymerase (ES =−0.59, NES =−1.97, FDR q-value =0.024) were correlated with lower ITGAL expression. (II) The maps of T-cell the receptor signaling pathway (ES =0.73, NES =2.07, FDR q-value =0.018) and FC epsilon Ri signaling pathway (ES =0.67, NES =2.04, FDR q-value =0.027) correlated with higher KLRB1 expression, while the excision repair (ES =−0.65, NES =−1.96, FDR q-value =0.047) and splice some (ES =−0.48, NES =−1.89, FDR q-value =0.040) were correlated with lower KLRB1 expression (Figure 5A; table available online: https://cdn.amegroups.cn/static/public/tcr-20-2275-6.pdf). Thus, based on the overall results of GO enrichment, immune cell regulatory network, and GSEA analyses, the map of the T-cell receptor signaling pathway was selected as the most important regulatory pathway. The expression level of enriched genes (including ITGAL and KLRB1) in the T-cell receptor signaling pathway are shown in Figure 5B.
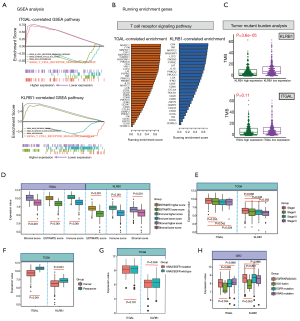
TMB has been reported as a clinically validated biomarker involved in anti-tumor immunity that drives T-cell responses. As shown in Figure 5C and table available online: https://cdn.amegroups.cn/static/public/tcr-20-2275-7.pdf, there was a significant difference between KLRB1 higher expression and lower expression (P=3.6e−05), while no statistical difference was detected in ITGAL expression level (P=0.11).
Correlation of hub gene expression levels and clinicopathological variables
As shown in Figure 5D,E, decreased expression of ITGAL and KLRB1 was significantly correlated with lower ESTIMATE, immune, and stromal scores (all, P<0.001), as well as correlated with clinical stage (ITGAL: stage I vs. stage III, P=0.042, stage I vs. stage IV, P=0.028; KLRB1: stage I vs. stage III, P=0.038, stage I vs. stage IV, P=0.005). There was a significant difference between cancer and para-cancer samples in both ITGAL and KLRB1 genes (all, P<0.001; Figure 5F). Additionally, reduced ITGAL and KLRB1 gene expression in LUAD samples was significantly associated with ALK fusion (ITGAL: P=0.034; KLRB1: P=0.050), but not with EGFR and KRAS mutations (Figure 5G,H).
Discussion
Calculation of the microenvironment features of LUAD found that the stromal, immune, and ESTIMATE scores were correlated with patient OS. Subsequently, based on the cutoff value of the ESTIMATE score, an indirect index of the immune microenvironment, we identified 250 DEGs in the TCGA-LUAD group based on higher or lower ESTIMATE score, and 511 DEGs in the GEO-LUAD group. In addition, 83 co-DEGs were identified. GO enrichment analysis showed the processes primarily enriched in immune microenvironment-related DEGs were T-cell activation, leukocyte cell-cell adhesion, and regulation of leukocyte cell-cell adhesion. Additionally, primary immunodeficiency, CAMs, NF-kappa B signaling pathway, TNF signaling pathway, and chemokine signaling pathway were primarily enriched in co-DEGs.
After filtering the results by KEGG pathway network criterion, Kaplan-Meier survival analysis and univariate/multivariate Cox regression analysis identified ITK, NCF1, MARCO, CSF2RB, ITGAL, KLRB1, CCL11, and VSIG4 genes as candidate biomarkers, and subsequently ITGAL and KLRB1 were considered predictor genes for LUAD OS. A LUAD-related immune cell regulatory network based on the OS of TCGA-LUAD and GEO-LUAD patients was constructed, and the immune infiltration subtypes of masT-cells resting and activated T-cells (CD4 memory) was identified. Correlation with GSEA enrichment results, the T-cell receptor signaling pathway was found to be primarily correlated with ITGAL and KLRB1 higher expression, and KLRB1 expression level was correlated with LUAD patient TMB. In addition, the expression level of both ITGAL and KLRB1 was correlated with clinicopathological features and ALK fusion.
After many relevant studies, immune-cancer dysfunction has become an accepted concept and immune checkpoint therapy (ICT) has become a common treatment for NSLSC (26). Due to tumor heterogeneity, LUAD patients can detect tumor markers for ICT (27).The anti-tumor function of peripheral T-cells, which shuttle back and forth between the tumor and systemic circulation, has received attention recently (28). Profiling of peripheral blood T-cell receptors has been found to have predictive value with respect to the prognosis of patients with advanced lung cancer (29). Immunotherapies, which are at the forefront of lung cancer therapeutics, are directed towards boosting host anti-tumor immunity, and T-cells play an important role (30). Zhang et al. showed that the high infiltration of T-cell presence in the tumor microenvironment is correlated to chemo-resistance in mesenchymal lung cancer cells (31). Furthermore, a recent study showed that targeting the DNA damage response promotes anti-tumor immunity through STING-mediated T-cell activation in small cell lung cancer (32).
ITGAL encodes the LFA-1 (aLb2) subunit of integrin, which is highly expressed in microglia, the spleen, bone marrow, and most immune cell populations (33). The results of the current study suggests that inherited variations of ITGAL can affect the expression level or function of encoded proteins, and thus may be potentially associated with cancer prognosis (34). Similarly, Boguslawska et al. showed that the expression signature of ITGAL is correlated with poor survival (35). Other study showed that ITGAL is involved in regulating triple-negative breast cancer cell migration through regulation of the actin cytoskeleton (36). Vendrell et al. also reported that under expression of ITGAL was correlated with survival of R0 Dukes B and C colorectal carcinomas patients (37).
The KLRB1 gene which encodes the CD161 receptor is located on chromosome 12 and is part of the natural killer gene complex (NKC) (38). Abnormal up-regulation of KLRB1 has been related to inflammatory syndromes, such as cryptococcosis-associated immune reconstitution inflammatory syndrome (39). A meta-analysis revealed a positive association of KLRB1 gene expression with favorable outcomes of NSCLC (40). In patients with myocardial infarction KLRB1 mRNA expression was found to be significantly down-regulated as compared to patients with angina patients and healthy controls (41). Gentles et al. found that KLRB1 mainly reflects the level of leukocytes infiltration in tumor tissues and is a good prognostic gene (42). Another study reported that expression of KLRB1 on human specific T-cells in the blood and cerebrospinal fluid (CSF) might be related to the compartmentalized inflammatory process in the central nervous system of patients with multiple sclerosis (43).
In summary, we found that the T-cell activation and activated T-cell (CD4 memory) pathways were predominantly involved in LUAD immune microenvironment regulation. The expression levels of ITGAL and KLRB1 were significantly correlated with the T-cell receptor signaling pathway and LUAD TMB, and were independent risk factors for OS.
Acknowledgments
The language of our revised manuscript has been checked by Richard Sandroe, who is a native English expert.
Funding: This work was supported by
Footnote
Conflicts of Interest: All authors have completed the ICMJE uniform disclosure form (available at https://dx.doi.org/10.21037/tcr-20-2275). The authors have no conflicts of interest to declare.
Ethical Statement: The authors are accountable for all aspects of the work in ensuring that questions related to the accuracy or integrity of any part of the work are appropriately investigated and resolved. The study was conducted in accordance with the Declaration of Helsinki (as revised in 2013).
Open Access Statement: This is an Open Access article distributed in accordance with the Creative Commons Attribution-NonCommercial-NoDerivs 4.0 International License (CC BY-NC-ND 4.0), which permits the non-commercial replication and distribution of the article with the strict proviso that no changes or edits are made and the original work is properly cited (including links to both the formal publication through the relevant DOI and the license). See: https://creativecommons.org/licenses/by-nc-nd/4.0/.
References
- Siegel RL, Miller KD, Jemal A. Cancer statistics, 2019. CA Cancer J Clin 2019;69:7-34. [Crossref] [PubMed]
- Siegel RL, Miller KD, Jemal A. Cancer statistics, 2018. CA Cancer J Clin 2018;68:7-30. [Crossref] [PubMed]
- Visconti R, Morra F, Guggino G, et al. The between Now and Then of Lung Cancer Chemotherapy and Immunotherapy. Int J Mol Sci 2017;18:1374. [Crossref] [PubMed]
- Wood DE, Kazerooni EA, Baum SL, et al. Lung Cancer Screening, Version 3.2018, NCCN Clinical Practice Guidelines in Oncology. J Natl Compr Canc Netw 2018;16:412-41. [Crossref] [PubMed]
- Nishio M, Takahashi T, Yoshioka H, et al. KEYNOTE-025: Phase 1b study of pembrolizumab in Japanese patients with previously treated programmed death ligand 1-positive advanced non-small-cell lung cancer. Cancer Sci 2019;110:1012-20. [Crossref] [PubMed]
- Quoix E, Lena H, Losonczy G, et al. TG4010 immunotherapy and first-line chemotherapy for advanced non-small-cell lung cancer (TIME): results from the phase 2b part of a randomised, double-blind, placebo-controlled, phase 2b/3 trial. Lancet Oncol 2016;17:212-23. [Crossref] [PubMed]
- Hui R, Garon EB, Goldman JW, et al. Pembrolizumab as first-line therapy for patients with PD-L1-positive advanced non-small cell lung cancer: a phase 1 trial. Ann Oncol 2017;28:874-81. [Crossref] [PubMed]
- Giroux Leprieur E, Dumenil C, Julie C, et al. Immunotherapy revolutionises non-small-cell lung cancer therapy: Results, perspectives and new challenges. Eur J Cancer 2017;78:16-23. [Crossref] [PubMed]
- Faruki H, Mayhew GM, Serody JS, et al. Lung Adenocarcinoma and Squamous Cell Carcinoma Gene Expression Subtypes Demonstrate Significant Differences in Tumor Immune Landscape. J Thorac Oncol 2017;12:943-53. [Crossref] [PubMed]
- Hirsch FR, Scagliotti GV, Mulshine JL, et al. Lung cancer: current therapies and new targeted treatments. Lancet 2017;389:299-311. [Crossref] [PubMed]
- Yoshihara K, Shahmoradgoli M, Martinez E, et al. Inferring tumour purity and stromal and immune cell admixture from expression data. Nat Commun 2013;4:2612. [Crossref] [PubMed]
- Mounir M, Lucchetta M, Silva TC, et al. New functionalities in the TCGAbiolinks package for the study and integration of cancer data from GDC and GTEx. PLoS Comput Biol 2019;15:e1006701. [Crossref] [PubMed]
- Clough E, Barrett T. The Gene Expression Omnibus Database. Methods Mol Biol 2016;1418:93-110. [Crossref] [PubMed]
- Bray NL, Pimentel H, Melsted P, et al. Near-optimal probabilistic RNA-seq quantification. Nat Biotechnol 2016;34:525-7. [Crossref] [PubMed]
- Zhang W, Chang JW, Lin L, et al. Network-Based Isoform Quantification with RNA-Seq Data for Cancer Transcriptome Analysis. PLoS Comput Biol 2015;11:e1004465. [Crossref] [PubMed]
- Love MI, Huber W, Anders S. Moderated estimation of fold change and dispersion for RNA-seq data with DESeq2. Genome Biol 2014;15:550. [Crossref] [PubMed]
- Ritchie ME, Phipson B, Wu D, et al. limma powers differential expression analyses for RNA-sequencing and microarray studies. Nucleic Acids Res 2015;43:e47. [Crossref] [PubMed]
- Law CW, Alhamdoosh M, Su S, et al. RNA-seq analysis is easy as 1-2-3 with limma, Glimma and edgeR. F1000Res 2016;5:ISCB Comm J-1408.
- Walter W, Sanchez-Cabo F, Ricote M. GOplot: an R package for visually combining expression data with functional analysis. Bioinformatics 2015;31:2912-4. [Crossref] [PubMed]
- Yu G, Wang LG, Han Y, et al. clusterProfiler: an R package for comparing biological themes among gene clusters. Omics 2012;16:284-7. [Crossref] [PubMed]
- Zhou Y, Zhou B, Pache L, et al. Metascape provides a biologist-oriented resource for the analysis of systems-level datasets. Nat Commun 2019;10:1523. [Crossref] [PubMed]
- Shannon P, Markiel A, Ozier O, et al. Cytoscape: a software environment for integrated models of biomolecular interaction networks. Genome Res 2003;13:2498-504. [Crossref] [PubMed]
- Chen B, Khodadoust MS, Liu CL, et al. Profiling Tumor Infiltrating Immune Cells with CIBERSORT. Methods Mol Biol 2018;1711:243-59. [Crossref] [PubMed]
- Subramanian A, Kuehn H, Gould J, et al. GSEA-P: a desktop application for Gene Set Enrichment Analysis. Bioinformatics 2007;23:3251-3. [Crossref] [PubMed]
- Chalmers ZR, Connelly CF, Fabrizio D, et al. Analysis of 100,000 human cancer genomes reveals the landscape of tumor mutational burden. Genome Med 2017;9:34. [Crossref] [PubMed]
- Steven A, Fisher SA, Robinson BW. Immunotherapy for lung cancer. Respirology 2016;21:821-33. [Crossref] [PubMed]
- Anagnostou VK, Brahmer JR. Cancer immunotherapy: a future paradigm shift in the treatment of non-small cell lung cancer. Clin Cancer Res 2015;21:976-84. [Crossref] [PubMed]
- Gros A, Parkhurst MR, Tran E, et al. Prospective identification of neoantigen-specific lymphocytes in the peripheral blood of melanoma patients. Nat Med 2016;22:433-8. [Crossref] [PubMed]
- Liu YY, Yang QF, Yang JS, et al. Characteristics and prognostic significance of profiling the peripheral blood T-cell receptor repertoire in patients with advanced lung cancer. Int J Cancer 2019;145:1423-31. [Crossref] [PubMed]
- Singhal S, Stadanlick J, Annunziata MJ, et al. Human tumor-associated monocytes/macrophages and their regulation of T cell responses in early-stage lung cancer. Sci Transl Med 2019;11:eaat1500. [Crossref] [PubMed]
- Zhang LN, Xin T, Chen M, et al. Chemoresistance in mesenchymal lung cancer cells is correlated to high regulatory T cell presence in the tumor microenvironment. IUBMB Life 2019;71:986-91. [Crossref] [PubMed]
- Sen T, Rodriguez BL, Chen L, et al. Targeting DNA Damage Response Promotes Antitumor Immunity through STING-Mediated T-cell Activation in Small Cell Lung Cancer. Cancer Discov 2019;9:646-61. [Crossref] [PubMed]
- Keum S, Lee HK, Chu PL, et al. Natural genetic variation of integrin alpha L (Itgal) modulates ischemic brain injury in stroke. PLoS Genet 2013;9:e1003807. [Crossref] [PubMed]
- Xie W, Stopsack KH, Drouin SJ, et al. Association of genetic variation of the six gene prognostic model for castration-resistant prostate cancer with survival. Prostate 2019;79:73-80. [Crossref] [PubMed]
- Boguslawska J, Kedzierska H, Poplawski P, et al. Expression of Genes Involved in Cellular Adhesion and Extracellular Matrix Remodeling Correlates with Poor Survival of Patients with Renal Cancer. J Urol 2016;195:1892-902. [Crossref] [PubMed]
- Klahan S, Wu MS, Hsi E, et al. Computational analysis of mRNA expression profiles identifies the ITG family and PIK3R3 as crucial genes for regulating triple negative breast cancer cell migration. Biomed Res Int 2014;2014:536591. [Crossref] [PubMed]
- Vendrell E, Ribas M, Valls J, et al. Genomic and transcriptomic prognostic factors in R0 Dukes B and C colorectal cancer patients. Int J Oncol 2007;30:1099-107. [Crossref] [PubMed]
- Bartel Y, Bauer B, Steinle A. Modulation of NK cell function by genetically coupled C-type lectin-like receptor/ligand pairs encoded in the human natural killer gene complex. Front Immunol 2013;4:362. [Crossref] [PubMed]
- Vlasova-St Louis I, Chang CC, Shahid S, et al. Transcriptomic Predictors of Paradoxical Cryptococcosis-Associated Immune Reconstitution Inflammatory Syndrome. Open Forum Infect Dis 2018;5:ofy157. [Crossref] [PubMed]
- Braud VM, Biton J, Becht E, et al. Expression of LLT1 and its receptor CD161 in lung cancer is associated with better clinical outcome. Oncoimmunology 2018;7:e1423184. [Crossref] [PubMed]
- Yan W, Zhou L, Wen S, et al. Differential loss of natural killer cell activity in patients with acute myocardial infarction and stable angina pectoris. Int J Clin Exp Pathol 2015;8:14667-75. [PubMed]
- Gentles AJ, Newman AM, Liu CL, et al. The prognostic landscape of genes and infiltrating immune cells across human cancers. Nat Med 2015;21:938-45. [Crossref] [PubMed]
- Schirmer L, Rothhammer V, Hemmer B, et al. Enriched CD161high CCR6+ gammadelta T cells in the cerebrospinal fluid of patients with multiple sclerosis. JAMA Neurol 2013;70:345-51. [Crossref] [PubMed]