Use of 6 m6A-relevant lncRNA genes as prognostic markers of primary liver hepatocellular carcinoma based on TCGA database
Introduction
Hepatocellular carcinoma (HCC) is the most common primary liver cancer. It has the sixth highest incidence rate and is the fourth leading cause of cancer death worldwide (1). The initiation and progression of liver cancer are complicated processes that involve genetic, epigenetic, and transcriptional changes (2). Liver resection is the most effective treatment method for liver cancer, as most patients are already at the advanced stage when diagnosed. However, only approximately 15% of patients are suitable for surgery. In addition, the 5-year survival of patients following surgery is only 33–50% (3). Knowledge of the molecular mechanism of liver cancer would inform discoveries that lead to better survival outcomes. In the present study, we screened the prognostic biomarkers of HCC that could serve as potential therapeutic targets.
N6-methyladenosine (m6A) is an epigenetic modification of RNA molecules. During tumorigenesis and development, the formation of m6A can affect tumor progression by regulating the expression of oncogenes and tumor suppressor genes through molecular epigenetics involving regulatory methyltransferases (“writers”), signal transducers (“readers”), and demethylases (“erasers”) (4). m6A has been correlated with the initiation and progression of breast cancer, cervical cancer (5-7). Some M6A genes such as FTO and IGF2BP2 have been found to promotes hepatocellular carcinoma tumorigenesis through different mechanisms (8,9).
Long non-coding RNAs (lncRNAs) are linear RNAs that are >200 nucleic acids in length and lack protein coding potential. LncRNAs regulate gene expression at the transcriptional or the post-transcriptional level. The abnormal expression of lncRNAs is closely related with the degree of tumor malignancy (10). The roles of lncRNAs in the progression of HCC are important (11). For example, MCm3AP antisense RNA 1 can promote the growth of HCC and is associated with its poor prognosis (12), while 3-phosphoinositide-dependent protein kinase 2 is considered a biomarker of HCC due to its function in stimulating the initiation, proliferation, and metastasis of HCC (13).
Recent studies have suggested that lncRNAs are involved in the regulation of m6A modification, and consequently the development of cancers, such as glioma and liver cancer (14,15). However, the general mechanism of m6A in the dysregulation of lncRNAs in tumor cells remains unclear. Furthermore, no research has sought to determine how m6A-relevant lncRNAs promote the initiation and progression of HCC. Therefore, by understanding how m6A-relevant lncRNAs are involved in the initiation and progression of HCC, new biomarkers that can serve as useful therapeutic targets can be identified.
Li et al. constructed a prognostic model of three m6a-related lncRNA as a prognostic marker for HCC (16). Different from their research, in this study, patients with HCC were divided into two groups by consensus clustering based on prognostic m6A-relevant lncRNAs screened from (TCGA) database, and we explored the relationship between the grouping and their clinicopathological characteristics. Subsequently, a novel and more accurate risk score model that could predict the prognosis of HCC was established using 6 key prognostic m6A-relevant lncRNAs identified from least absolute shrinkage and selection operator (LASSO) Cox regression analysis. We present the following article in accordance with the TRIPOD reporting checklist (available at https://dx.doi.org/10.21037/tcr-21-2440).
Methods
Case data
The gene expression data of 374 HCC patients and 50 healthy controls were retrieved from TCGA (accessed in April 2021). Clinical information was available for 370 patients. Based on the literature, the expression data of 23 m6A-relevant genes were obtained from TCGA. These included 8 writers methyltransferase-like 3 (METTL3), methyltransferase-like 14 (METTL14), methyltransferase-like 16 (METTL16), Wilms tumor 1-associated protein (WTAP), Vir-like m6A methyltransferase associated protein (VIRMA), Zinc finger CCCH-Type containing 13 (ZC3H13), RNA-binding protein 15 (RBM15), and RNA-binding protein 15 B (RBM15B), 13 readers YT521-B homology domain containing 1 (YTHDC1), YT521-B homology domain containing 2 (YTHDC2), YT521-B homology domain family 1 (YTHDF1), YT521-B homology domain family 2 (YTHDF2), YT521-B homology domain family 3 (YTHDF3), heterogeneous nuclear ribonucleoprotein C (HNRNPC), fragile X mental retardation 1 (FMR1), Leucine-rich PPR-motif-containing protein (LRPRRC), heterogeneous nuclear ribonucleoprotein A2B1 (HNRNPA2B1), insulin-like growth factor 2 mRNA binding protein 1 (IGF2BP1), insulin-like growth factor 2 mRNA binding protein 2 (IGF2BP2), insulin-like growth factor 2 mRNA binding protein 3 (IGF2BP3), and RNA binding protein X (RBMX), and 2 erasers obesity-associated protein (FTO) and alkB homolog 5 (ALKBH5). The study was conducted in accordance with the Declaration of Helsinki (as revised in 2013).
Identification of prognostic m6A-relevant lncRNAs and consensus clustering
m6A-relevant lncRNAs were first identified by co-expression analysis using the R software package “limma” based on Pearson R>0.4 and P<0.001. The co-expression network was established using the R package “igraph”. Prognostic m6A-relevant lncRNAs were recognized by univariate Cox regression analysis (P<0.001). HCC patients were allocated into different clusters using consensus clustering based on the expression levels of these lncRNAs. Kaplan-Meier (KM) analysis was used to investigate the difference in the overall survival (OS) of the different clusters. The R plugins “pheatmap” and “limma” were used to analyze differences in the clinical characteristics, expression level of programmed death-ligand 1 (PD-L1), and the expression levels of m6A-relevant lncRNAs between the different clusters.
Gene set enrichment analysis (GSEA)
Pathway analysis was completed using GSEA software (version 4.0.3). Nominal P<0.05 and false discovery rate q values <0.25 were considered statistically significant.
Establishment and evaluation of the risk score model
Patients were first randomly divided into training and test groups. LASSO Cox analysis was used to identify key m6A-relevant lncRNAs associated with the prognosis of patients in the training group. Coefficients were determined through the minimum standard method (minimum 10 cross-validations to evaluate penalty parameters). Once the m6A-relevant lncRNAs were identified, a risk score model was calculated as:
where Coefi is the coefficient and xi is the fragments per kilobase of transcript per million (FPKM) value of the key prognostic m6A-relevant lncRNAs.
After patients were divided into low-risk and high-risk groups according to the average risk score, the efficacy of the risk score model was assessed by survival analysis, risk plots, and the receiver-operating characteristic (ROC) curve. In addition, the value of the risk score model was verified by the test group. Univariate and multivariate Cox analyses were used to determine the effect of the risk score and other clinical characteristics on OS. The prognostic value of the risk score in populations with different clinicopathological characteristics was evaluated using the R package “survminer”.
Relationships between risk score, patient grouping, and clinicopathological characteristics
R packages “pheatmap” and “limma” were used to investigate the correlation between the risk score, patient grouping determined by consensus clustering, and the clinicopathological characteristics of HCC patients.
Statistical analyses
Data analyses were performed using R software (version 4.0.5). Unless otherwise specified, P<0.05 was considered statistically significant; χ2-test was used to analyze the relationship between different groups and their clinicopathological characteristics. Univariate and multivariate Cox analyses were introduced to explore the relationship between individual factors and angiogenesis. Data visualization was performed using the R package “ggplot2”.
Results
Patient characteristics
The clinical characteristics of 370 HCC patients are shown in Table 1. The average follow-up time was 585 days, with a range of 0–3,675 days.
Table 1
Clinical characteristic | Total (n=370) | Percentage, % |
---|---|---|
Age at diagnosis (years) | 61 (range, 16–90) | |
Sex | ||
Male | 249 | 67.30 |
Female | 121 | 22.70 |
Grade | ||
G1 | 55 | 14.86 |
G2 | 177 | 47.84 |
G3 | 121 | 32.70 |
G4 | 12 | 3.24 |
GX | 5 | 1.36 |
Stage | ||
I | 171 | 46.22 |
II | 85 | 22.97 |
III | 85 | 22.97 |
IV | 5 | 1.35 |
X | 24 | 6.49 |
T stage | ||
T1 | 181 | 48.92 |
T2 | 93 | 25.14 |
T3 | 80 | 21.62 |
T4 | 13 | 3.51 |
TX | 3 | 0.81 |
Lymph nodes | ||
N0 | 252 | 68.11 |
N1 | 4 | 1.08 |
NX | 114 | 30.81 |
Distant metastasis | ||
M0 | 266 | 71.89 |
M1 | 4 | 1.08 |
MX | 100 | 27.03 |
HCC, hepatocellular carcinoma; TCGA, The Cancer Genome Atlas.
Prognostic m6A-relevant lncRNAs in HCC
A total of 1852 m6A-relevant lncRNAs were identified in the co-expression analysis (Figure 1). Of these, 68 were related to the prognosis of HCC in the univariate Cox regression analysis. The expression levels of lncRNAs in tumor and normal tissues, and their hazard ratios, are shown in Figure 2.
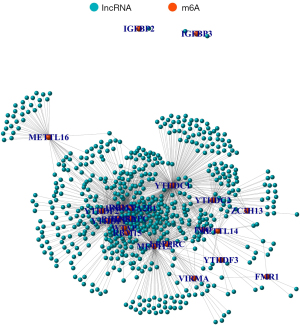
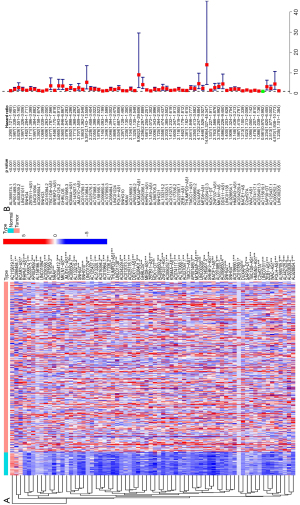
Patient clusters determined by consensus clustering
The consensus clustering distribution function (CDF) for k=2–9, the increment in the area under the CDF curve (AUC), and the tracking plot for k=2–9 are shown in Figure 3A-3C, respectively. Two HCC clusters (k=2, clusters 1 and 2) were subsequently determined according to the largest increment of AUC and the correlation of the expression of prognostic m6A-relevant lncRNAs in the clusters (Figure 3D).
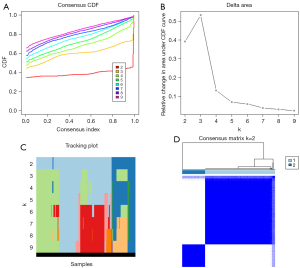
Clinical characteristics and pathway analysis of the different clusters
KM survival analysis indicated that the OS of cluster 2 was substantially longer than that of cluster 1 (P<0.001) (Figure 4A). The clinical characteristics and expression of the lncRNAs of the different clusters were compared. A heat map revealed that the pathological grade, American Joint Committee on Cancer (AJCC) stage, and T stage of patients in cluster 1 were significantly more advanced than those of patients in cluster 2 (Figure 4B). In addition, GSEA suggested that mitotic spindle, G2M_CHECKPOINT, glycolysis, PI3K AKT mTOR pathway, and DNA repair were more enriched in cluster 1 than in cluster 2 (Figure 4C, Table 2). PD-L1 expression was substantially higher in cluster 1 than in cluster 2 (Figure 4D).
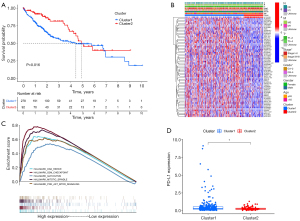
Table 2
Clusters | Enriched pathways | NES | NOM P value | FDR q value |
---|---|---|---|---|
Cluster 1 vs. cluster 2 | HALLMARK_MITOTIC_SPINDLE | 2.012 | 0.000 | 0.003 |
HALLMARK_G2M_CHECKPOINT | 2.012 | 0.000 | 0.002 | |
HALLMARK_GLYCOLYSIS | 1.935 | 0.000 | 0.005 | |
HALLMARK_PI3K_AKT_MTOR_SIGNALING | 1.882 | 0.000 | 0.007 | |
HALLMARK_DNA_REPAIR | 1.948 | 0.002 | 0.005 |
Pathways with NOM P<0.05 and FDR q value <0.05 are considered statistically significant. FDR, false discovery rate; NES, normalized enrichment score; NOM, nominal.
Use of the risk score model based on 6 key prognostic m6A-relevant lncRNAs to predict the prognosis of HCC
When 6 key prognostic m6A-relevant lncRNAs were identified from the training group, a risk score model was established based on the FPKM value and the coefficient of these lncRNAs (Figure 5, Table 3). The survival analysis suggested that the Overall Survival (OS) of high-risk patients was shorter in both the training group and the test group (Figure 6A). The AUC of the 3-year OS curve of the training group and the test group was 0.86 and 0.65, respectively (Figure 6B). The risk plots and survival state also indicated that the risk score could accurately distinguish between high-risk and low-risk patients (Figure 6C,6D). Univariate and multivariate analyses revealed that the risk score was an independent factor for survival in the 2 groups (Figures 7,8). The prognostic value of the risk score in populations with different clinicopathological characteristics was evaluated. The findings suggested that the risk score could accurately distinguish the prognosis of almost all populations, except for female patients. Patients with distant metastasis and lymph node metastasis were excluded from the analysis, as there were only 4 patients with each of these metastases (Figure 9).
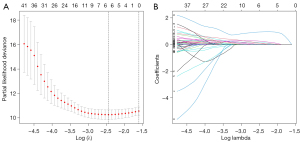
Table 3
Name | Coefficients |
---|---|
AC099850.4 | 0.022 |
AC098484.1 | 0.146 |
LINC01224 | 0.241 |
NRAV | 0.095 |
TMCC1-AS1 | 0.944 |
MKLN1-AS | 0.083 |
LINC, long non-coding; lncRNAs, long non-coding RNAs; NRAV, negative regulator of antiviral response; TMCC1-AS1, transmembrane and coiled-coil domain family 1 antisense RNA 1; MKLN1-AS, muskelin 1 antisense RNA 1.
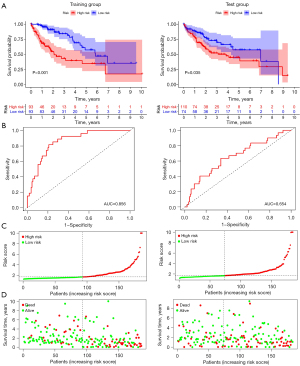
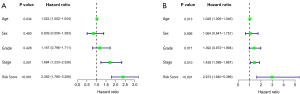
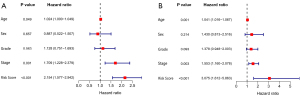
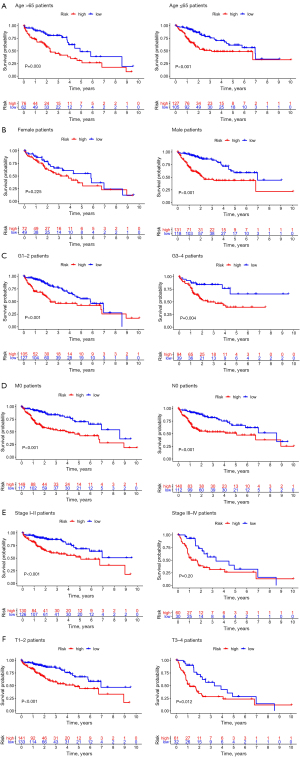
Relationship between risk score, clusters, and clinicopathological characteristics
The risk score of patients in cluster 1, whose prognosis was poor, was higher than that of patients in cluster 2, whose prognosis was good. In addition, the pathological grade, AJCC stage, and T stage of patients with a higher risk score was substantially more advanced than those with a lower risk score (Figure 10).
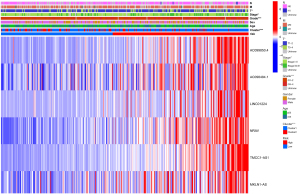
Discussion
HCC is usually asymptomatic in the early stage, and patients diagnosed with the disease are often already in the middle and advanced stages; this can rule out radical treatment (17). Therefore, it is important to identify specific and effective biomarkers that can predict the prognosis of HCC, and on this basis, discover potential therapeutic targets. Some recent studies have already explored effective biomarkers of HCC. One study established a prognostic model for HCC based on 3 m6A-relevant genes identified by the authors (18).
To identify new biomarkers of HCC, in the present study we investigated the prognostic value of m6A-relevant lncRNAs using TCGA data of 370 patients with HCC. A total of 1852 m6A-relevant lncRNAs were identified via co-expression analysis. Of these, 68 were prognostic related according to univariate Cox regression analysis. Subsequently, based on differences in the expression of these prognostic m6A-relevant lncRNAs, patients were divided into2 clusters via consensus clustering. Patients in cluster 1 had more advanced pathological grade, AJCC stage, and T stage, as well as poorer OS. The findings indicated that the proposed method could accurately distinguish the prognosis of HCC patients, and m6A-relevant lncRNAs could be important in the pathogenesis of HCC. In addition, the expression of PD-L1 in cluster 1 was higher than that in cluster 2, suggesting the existence of immune tolerance in cluster 1, which was consistent with the worse prognosis of this cluster. It also suggested that the effect of immunotherapy might be better in this cluster (19). GSEA also suggested that
mitotic spindle, G2M_CHECKPOINT, glycolysis, the PI3K AKT mTOR pathway, and DNA repair were more enriched in cluster 1 than cluster 2. Among them, mitotic spindle, G2M_CHECKPOINT, and DNA repair have been recognized to be related to cell-cycle and DNA damage repair. In addition, the abnormal expression of these pathways is often involved in the initiation and progression of cancer (20,21). Glycolysis pathways allow tumor cells to bypass oxidative phosphorylation at the cellular level, and in turn, accelerate the hydrolysis of glucose, thereby enabling tumor cells to compete with normal cells for glucose uptake and consequently maintain uninterrupted growth. Glycolysis is involved in the occurrence and development of different tumors, including prostate cancer, breast cancer, and liver cancer (22-25). Alternatively, the PI3K AKT mTOR pathway is one of the important pathways of liver cancer (26,27). LncRNAs associated with the dysregulation of the PI3K AKT mTOR pathway were related to the proliferation, metastasis, and drug resistance of liver cancer (28). However, the relationship between these dysregulated pathways, m6A-relevant lncRNAs, and the progression and prognosis of HCC requires further clarification.
Six key prognostic m6A-relevant lncRNAs based on the LASSO Cox regression analysis results of the training group were identified. Of these lncRNAs, AC098484.1 has not been studied. AC099850.4 was previously associated with the potentially poor prognosis of high-grade serous ovarian cancer (29). Long intergenic non-protein coding RNA 1224 (LINC01224) is upregulated in ovarian cancer and is related to tumor size and lymph node metastasis (30). In addition, the upregulation of LINC01224 is involved in the invasion and metastasis of tumor cells in gastric and bowel cancers (31,32). In HCC, silencing LINC01224 can downregulate the expression of Checkpoint Kinase 1 (CHEK1) by competitively binding to miR-330-5p, thereby inhibiting the proliferation, migration, and invasion of HCC cells (33). Alternatively, negative regulator of antiviral response (NRAV) predicts the efficacy and prognosis of HCC immunotherapy (34), while transmembrane and coiled-coil domain family 1 antisense RNA 1 (TMCC1-AS1) has been introduced in a number of liver cancer prognostic models (35-38), although its specific mechanism in HCC is unclear. muskelin 1 antisense RNA (MKLN1-AS) can promote the proliferation, migration, and invasion of HCC cells through miR-654-3p (39). It is also considered a biomarker for the diagnosis and prognosis of HCC, and consequently, a potential therapeutic target (40). Similar to TMCC1-AS1, MKLN1-AS has been implemented in multiple prognostic models for liver cancer (38,41). According to the FPKM values and the coefficients of these key prognostic m6A-relevant lncRNAs, a risk score model was established in the present study to predict the prognosis of HCC. The resulting risk scores of the training and test groups were subsequently compared. The results of the KM analysis, ROC curves, risk map analysis, and univariate and multivariate Cox regression analyses implicated the risk score as a reliable independent prognostic factor of HCC. In addition, with the exception of female patients, the risk score could predict the prognosis of almost all populations with different clinicopathological characteristics. Patients in the high-risk group had more advanced pathological grade, AJCC stage, and T stage, as well as shorter OS, than those in the low-risk group. Furthermore, the risk score of the high-risk cluster 1 was also greater than that of the low-risk cluster 2. These results indicated that key prognostic m6A-relevant lncRNAs identified in the present study could be important in the initiation and progression of HCC. The significance and accuracy of the risk score model in predicting the prognosis of HCC were successfully verified in the present study.
The present study had some limitations. Firstly, the sample sizes of the HCC RNA sequence FPKM data, specifically the adjacent normal tissues, were small, which can lead to potential statistical errors. Secondly, no experiment has been performed to confirm the interaction between prognostic factors lncRNAs and M6A modulators in HCC. Thirdly, further experiments are needed to explore the expression and functions of representative lncRNA, and the efficacy of the proposed prognostic model in the clinical environment to improve its reliability in predicting the prognosis of HCC patients.
In conclusion, our study verified the expression and prognostic value of m6A-relevant lncRNAs in HCC. The expression of m6A-relevant lncRNAs is closely related to the clinical characteristics and poor survival rate of HCC patients. The data will inform future research on the role of m6A-relevant lncRNAs in HCC.
Acknowledgments
We would like to thank Editage (www.editage.com) for English language editing.
Funding: This study was supported by the Science and Technology Development Plan of Taicang in Jiangsu Province, China (No. TC2020JCYL20).
Footnote
Reporting Checklist: The authors have completed the TRIPOD reporting checklist. Available at https://dx.doi.org/10.21037/tcr-21-2440
Conflicts of Interest: All authors have completed the ICMJE uniform disclosure form (available at https://dx.doi.org/10.21037/tcr-21-2440). The authors have no conflicts of interest to declare.
Ethical Statement: The authors are accountable for all aspects of the work in ensuring that questions related to the accuracy or integrity of any part of the work are appropriately investigated and resolved. The study was conducted in accordance with the Declaration of Helsinki (as revised in 2013).
Open Access Statement: This is an Open Access article distributed in accordance with the Creative Commons Attribution-NonCommercial-NoDerivs 4.0 International License (CC BY-NC-ND 4.0), which permits the non-commercial replication and distribution of the article with the strict proviso that no changes or edits are made and the original work is properly cited (including links to both the formal publication through the relevant DOI and the license). See: https://creativecommons.org/licenses/by-nc-nd/4.0/.
References
- Torre LA, Bray F, Siegel RL, et al. Global cancer statistics, 2012. CA Cancer J Clin 2015;65:87-108. [Crossref] [PubMed]
- Wong CM, Lee JM, Ching YP, et al. Genetic and epigenetic alterations of DLC-1 gene in hepatocellular carcinoma. Cancer Res 2003;63:7646-51. [PubMed]
- Kow AW. Transplantation versus liver resection in patients with hepatocellular carcinoma. Transl Gastroenterol Hepatol 2019;4:33. [Crossref] [PubMed]
- Lan Q, Liu PY, Haase J, et al. The Critical Role of RNA m6A Methylation in Cancer. Cancer Res 2019;79:1285-92. [Crossref] [PubMed]
- Zhang C, Samanta D, Lu H, et al. Hypoxia induces the breast cancer stem cell phenotype by HIF-dependent and ALKBH5-mediated m6A-demethylation of NANOG mRNA. Proc Natl Acad Sci U S A 2016;113:E2047-56. [Crossref] [PubMed]
- Ma X, Li Y, Wen J, et al. m6A RNA methylation regulators contribute to malignant development and have a clinical prognostic effect on cervical cancer. Am J Transl Res 2020;12:8137-46. [PubMed]
- Huang GZ, Wu QQ, Zheng ZN, et al. M6A-related bioinformatics analysis reveals that HNRNPC facilitates progression of OSCC via EMT. Aging (Albany NY) 2020;12:11667-84. [Crossref] [PubMed]
- Li J, Zhu L, Shi Y, et al. m6A demethylase FTO promotes hepatocellular carcinoma tumorigenesis via mediating PKM2 demethylation. Am J Transl Res 2019;11:6084-92. [PubMed]
- Pu J, Wang J, Qin Z, et al. IGF2BP2 Promotes Liver Cancer Growth Through an m6A-FEN1-Dependent Mechanism. Front Oncol 2020;10:578816. [Crossref] [PubMed]
- Zhan Y, Guan XY, Li Y. MAFA-AS1, a long non-coding RNA, predicts for poor survival of hepatocellular carcinoma. Transl Cancer Res 2020;9:2449-59. [Crossref]
- Huang Z, Zhou JK, Peng Y, et al. The role of long noncoding RNAs in hepatocellular carcinoma. Mol Cancer 2020;19:77. [Crossref] [PubMed]
- Wang Y, Yang L, Chen T, et al. A novel lncRNA MCM3AP-AS1 promotes the growth of hepatocellular carcinoma by targeting miR-194-5p/FOXA1 axis. Mol Cancer 2019;18:28. [Crossref] [PubMed]
- Pan W, Li W, Zhao J, et al. lncRNA-PDPK2P promotes hepatocellular carcinoma progression through the PDK1/AKT/Caspase 3 pathway. Mol Oncol 2019;13:2246-58. [Crossref] [PubMed]
- Tu Z, Wu L, Wang P, et al. N6-Methylandenosine-Related lncRNAs Are Potential Biomarkers for Predicting the Overall Survival of Lower-Grade Glioma Patients. Front Cell Dev Biol 2020;8:642. [Crossref] [PubMed]
- Zuo X, Chen Z, Gao W, et al. M6A-mediated upregulation of LINC00958 increases lipogenesis and acts as a nanotherapeutic target in hepatocellular carcinoma. J Hematol Oncol 2020;13:5. [Crossref] [PubMed]
- Li L, Xie R, Lu G. Identification of m6A methyltransferase-related lncRNA signature for predicting immunotherapy and prognosis in patients with hepatocellular carcinoma. Biosci Rep 2021;41:BSR20210760. [Crossref] [PubMed]
- Hou Z, Zhu K, Yang X, et al. Resection of “down-staged” advanced hepatocellular carcinoma after treatment with the VEGFR2 inhibitor apatinib: five cases report. Transl Cancer Res 2020;9:4999-5007. [Crossref]
- Li Z, Li F, Peng Y, et al. Identification of three m6A-related mRNAs signature and risk score for the prognostication of hepatocellular carcinoma. Cancer Med 2020;9:1877-89. [Crossref] [PubMed]
- Wu X, Gu Z, Chen Y, et al. Application of PD-1 Blockade in Cancer Immunotherapy. Comput Struct Biotechnol J 2019;17:661-74. [Crossref] [PubMed]
- Nicholson JM, Cimini D. How mitotic errors contribute to karyotypic diversity in cancer. Adv Cancer Res 2011;112:43-75. [Crossref] [PubMed]
- Bucher N, Britten CD. G2 checkpoint abrogation and checkpoint kinase-1 targeting in the treatment of cancer. Br J Cancer 2008;98:523-8. [Crossref] [PubMed]
- Ganapathy-Kanniappan S. Molecular intricacies of aerobic glycolysis in cancer: current insights into the classic metabolic phenotype. Crit Rev Biochem Mol Biol 2018;53:667-82. [Crossref] [PubMed]
- Yang J, Ren B, Yang G, et al. The enhancement of glycolysis regulates pancreatic cancer metastasis. Cell Mol Life Sci 2020;77:305-21. [Crossref] [PubMed]
- Wu Z, Wu J, Zhao Q, et al. Emerging roles of aerobic glycolysis in breast cancer. Clin Transl Oncol 2020;22:631-46. [Crossref] [PubMed]
- Feng J, Li J, Wu L, et al. Emerging roles and the regulation of aerobic glycolysis in hepatocellular carcinoma. J Exp Clin Cancer Res 2020;39:126. [Crossref] [PubMed]
- Torresi J, Tran BM, Christiansen D, et al. HBV-related hepatocarcinogenesis: the role of signalling pathways and innovative ex vivo research models. BMC Cancer 2019;19:707. [Crossref] [PubMed]
- Jindal A, Thadi A, Shailubhai K. Hepatocellular Carcinoma: Etiology and Current and Future Drugs. J Clin Exp Hepatol 2019;9:221-32. [Crossref] [PubMed]
- Wu Y, Zhang Y, Qin X, et al. PI3K/AKT/mTOR pathway-related long non-coding RNAs: roles and mechanisms in hepatocellular carcinoma. Pharmacol Res 2020;160:105195. [Crossref] [PubMed]
- Zhao J, Song X, Xu T, et al. Identification of Potential Prognostic Competing Triplets in High-Grade Serous Ovarian Cancer. Front Genet 2021;11:607722. [Crossref] [PubMed]
- Xing S, Zhang Y, Zhang J. LINC01224 Exhibits Cancer-Promoting Activity in Epithelial Ovarian Cancer Through microRNA-485-5p-Mediated PAK4 Upregulation. Onco Targets Ther 2020;13:5643-55. [Crossref] [PubMed]
- Sun H, Yan J, Tian G, et al. LINC01224 accelerates malignant transformation via MiR-193a-5p/CDK8 axis in gastric cancer. Cancer Med 2021;10:1377-93. [Crossref] [PubMed]
- Chen L, Chen W, Zhao C, et al. LINC01224 Promotes Colorectal Cancer Progression by Sponging miR-2467. Cancer Manag Res 2021;13:733-42. [Crossref] [PubMed]
- Gong D, Feng PC, Ke XF, et al. Silencing Long Non-coding RNA LINC01224 Inhibits Hepatocellular Carcinoma Progression via MicroRNA-330-5p-Induced Inhibition of CHEK1. Mol Ther Nucleic Acids 2020;19:482-97. [Crossref] [PubMed]
- Xu Q, Wang Y, Huang W. Identification of immune-related lncRNA signature for predicting immune checkpoint blockade and prognosis in hepatocellular carcinoma. Int Immunopharmacol 2021;92:107333. [Crossref] [PubMed]
- Zhao QJ, Zhang J, Xu L, et al. Identification of a five-long non-coding RNA signature to improve the prognosis prediction for patients with hepatocellular carcinoma. World J Gastroenterol 2018;24:3426-39. [Crossref] [PubMed]
- Cui H, Zhang Y, Zhang Q, et al. A comprehensive genome-wide analysis of long noncoding RNA expression profile in hepatocellular carcinoma. Cancer Med 2017;6:2932-41. [Crossref] [PubMed]
- Deng B, Yang M, Wang M, et al. Development and validation of 9-long Non-coding RNA signature to predicting survival in hepatocellular carcinoma. Medicine (Baltimore) 2020;99:e20422. [Crossref] [PubMed]
- Deng X, Bi Q, Chen S, et al. Identification of a Five-Autophagy-Related-lncRNA Signature as a Novel Prognostic Biomarker for Hepatocellular Carcinoma. Front Mol Biosci 2021;7:611626. [Crossref] [PubMed]
- Gao W, Chen X, Chi W, et al. Long non-coding RNA MKLN1-AS aggravates hepatocellular carcinoma progression by functioning as a molecular sponge for miR-654-3p, thereby promoting hepatoma-derived growth factor expression. Int J Mol Med 2020;46:1743-54. [Crossref] [PubMed]
- Guo C, Zhou S, Yi W, et al. Long non-coding RNA muskelin 1 antisense RNA (MKLN1-AS) is a potential diagnostic and prognostic biomarker and therapeutic target for hepatocellular carcinoma. Exp Mol Pathol 2021;120:104638. [Crossref] [PubMed]
- Xiao JR, Wang K, Liu Y, et al. Exploring of a prognostic long non-coding RNA signature of hepatocellular carcinoma by using public database. Zhonghua Liu Xing Bing Xue Za Zhi 2019;40:805-9. [PubMed]
(English Language Editor: R. Scott)