Reconstruction and analysis of the aberrant lncRNA-miRNA-mRNA network based on competitive endogenous RNA in adenoid cystic carcinoma of the salivary gland
Introduction
Adenoid cystic carcinoma (ACC) is a relatively rare cancer, accounting for 1% of head and neck tumors and 10% of salivary gland tumors (1-3). SACC is characterized by high rates of recurrence, perineural invasion, and distant metastasis (2,3). Current treatments are still mainly surgery and adjuvant radiation, and no systemic chemotherapy has been proven to be fully effective (4). However, over time, most patients develop recurrence and distant metastasis (5). One study had suggested that the overall 5-, 10-, and 15-year survival estimates for all stages among ACC were 68%, 52%, and 28%, respectively (6). Despite some remarkable achievements have been made recently, the etiology and pathogenesis of SACC remain largely unclear (7). Therefore, it is important to have a deeper understanding of the molecular mechanism underlying SACC tumorigenesis and to seek new therapeutic strategies for SACC.
Long noncoding RNAs (lncRNAs), a class of noncoding RNAs (ncRNAs) with no known protein-coding functions and a length of more than 200 nucleotides (8), are involved in numerous important cell biological processes, including cellular homeostasis and transcriptional and posttranscriptional regulation (8,9). MicroRNAs (miRNAs), a type of short ncRNA with approximately 22 nucleotides (10), can partially or entirely bind to the 3' untranslated regions (3' UTRs) of target genes to promote the degradation of targeted mRNAs and translation suppression, along with the negative regulation of gene expression at posttranscriptional levels (11,12). The competing endogenous RNA (ceRNA) hypothesis was proposed by Salmena et al. (13) as early as 2011, according to the ceRNA hypothesis, endogenous RNA molecules have miRNA action sites and can competitively bind to miRNAs, thus indirectly regulating the use of miRNA target factors. A series of studies have shown that miRNAs can silence genes by binding to mRNA (14), and lncRNA can be a “molecular sponge” of miRNA, binding miRNA to attenuate its silencing effects on target genes and to regulate them (15). Therefore, the interaction between three types of RNAs results in the formation of lncRNA-miRNA-mRNA networks, which gives rise to ceRNA.
To date, numerous experimental studies have supported the theory of ceRNA network regulation and it is reported that ceRNA network has been constructed in gastric cancer (16), hepatocellular carcinoma (17), cervical cancer (18), ovarian clear cell carcinoma (19) and other cancers. However, ceRNA networks have rarely been reported in the field of SACC. The rapid development of gene sequencing technology and the establishment of online public database provide a resource platform for data mining and research. In our work, the disciplinary advantages of molecular biology and bioinformatics were integrated to construct a molecular regulatory network in ACC, and we screened RNAs that may be associated with SACC. Through this analysis, we aim to better understand the pathogenesis of SACC and reveal some potential lncRNA biomarkers.
We present the following article in accordance with the MDAR reporting checklist (available at https://dx.doi.org/10.21037/tcr-21-1771).
Methods
Data downloading and preprocessing
We obtained RNA sequencing data from the Gene Expression Omnibus (GEO) database. The lncRNA/mRNA expression profile data were downloaded from GSE88804 (https://www.ncbi.nlm.nih.gov/geo/query/acc.cgi?acc=GSE88804) RNA sequencing datasets, which contained 13 SACC tissues and 7 normal salivary gland (NSG) tissues. The miRNA expression profile data were extracted from the GSE117275 (https://www.ncbi.nlm.nih.gov/geo/query/acc.cgi?acc=GSE117275) database, which contained 3 SACC tissues and 3 NSG tissues. The downloaded raw lncRNA and mRNA data were identified and annotated by BLAST comparison according to gene annotation files in the NCBI, Ensembl, and NONCODEv5 databases. All data from GEO are publicly available and they do not require the approval of a local ethics committee. The analysis process of this experiment is shown in Figure 1.
Tissue collection
Samples came from SACC patients who had finished the operation at our center from August 2020 to March 2021. These patients met the following inclusion and exclusion criteria: the tumor was primary, the pathological type was ACC, patients with preoperative radiotherapy or chemotherapy were excluded, patients with other malignant tumors were excluded. A total of 3 samples were used in our study. Immediately after tumor resection, tumors and normal salivary gland tissues were frozen in liquid nitrogen, and stored at −80 °C. Both preoperative biopsy and postoperative histopathology were diagnosed as ACC of the salivary gland. Three matched SACC tissue samples and corresponding normal salivary gland tissues were investigated by quantitative real-time polymerase chain reaction (qRT-PCR). The study was approved by the Ethics Committee and was conducted under the guidance of international ethical standards (IRB number: PKUSSIRB-202056098). The study was conducted in accordance with the Declaration of Helsinki (as revised in 2013). Written informed consent was obtained from all patients.
Screening of differentially expressed RNAs
The Edge R package in R (version 3.4.1) was used to identify the differentially expressed RNAs (DE-RNAs) (20). By setting |log2(fold change)| >1, false discovery rate (FDR) <0.05 and P<0.05 as the criteria (21), the differentially expressed lncRNAs (DE-lncRNAs), differentially expressed mRNAs (DE-mRNAs) and differentially expressed miRNAs (DE-miRNAs) were screened for subsequent analyses. Cluster analyses of DE-lncRNAs, DE-mRNAs, and DE-miRNAs were performed by clustergram.
Functional enrichment analysis of DE-mRNAs
Kyoto Encyclopedia of Genes and Genomes (KEGG) and Gene Ontology (GO) functional enrichment analyses were conducted on DE-mRNAs using the R clusterProfiler package (22). Fisher’s test was used to identify the significant GO terms and KEGG pathways, and P value <0.05 was considered statistically significant.
Construction of the lncRNA-miRNA-mRNA regulatory network
We searched for connections between significant DE-lncRNAs and DE-miRNAs using miRanda (http://www.microrna.org/) and PITA (https://genie.weizmann.ac.il/pubs/mir07/mir07_exe.html), and we predicted the target mRNAs of the DE-miRNAs using miRanda (http://www.microrna.org/), TargetScan (http://www.targetscan.org/), and miRWalk (http://129.206.7.150/). At the same time, only the connection pairs with opposite expression directions in the connection relationship were retained, thereby constructing the lncRNA-miRNA and miRNA-mRNA connection network. Then, the lncRNA-miRNA-mRNA regulatory network was obtained based on lncRNA-miRNA and miRNA-mRNA regulatory pairs. The ceRNA network was plotted with Cytoscape v3.6.0 (23).
Construction of the PPI network and screening for the hub genes
Protein-protein interaction (PPI) network prediction for target genes was carried out using the Search Tool for the Retrieval of Interacting Genes/Proteins (STRING) database (https://string-db.org/). Based on the PPI network, we chose the top several genes with the highest degree of connections to other genes as hub genes. In addition, mRNAs in the modules were selected as core RNAs to constitute a subnetwork.
RNA extraction and qRT-PCR analysis
Total RNA was extracted with TRIzol (15596026, Invitrogen, USA) reagent following the manufacturer's instructions and quantified the RNA using Nanodrop 2000 (Thermo Fisher Scientific, MA, USA). For lncRNA and mRNA quantifying, cDNA was synthesized using the M-MLV Reverse Transcriptase (m1705, Promega, USA) and dNTP Mix (u1511, Promega, USA) and M-MLV RT 5× Buffer (M531A, Promega, USA) and Random Primers (C1181, Promega). And then FastStart Universal SYBR Green Master (ROX) (04913914001, Germany) was used for qRT-PCR. The detection of miRNAs was used the miDETECT A Track miRNA qRT-PCR Starter Kit (C10712-1, RIBBIO). RPS18 and U6 were employed as endogenous controls for lncRNA/mRNA qRT-PCR and miRNA qRT-PCR, respectively. Primer sequences are listed in Table 1. The primer sequences of U6 were not available from the provider. The relative expression of lncRNAs, mRNAs, and miRNAs was calculated by the 2−△△Ct method. The qPCRs were all repeated three times for the three matched samples.
Table 1
Gene | Forward primer (5' to 3') | Reverse primer (5' to 3') |
---|---|---|
NONHSAT251752.1 | TCATGGCAGCTAAAGCACGA | CAACTGTGAACGCTGCTGAC |
NONHSAT232163.1 | AAGCGTCTCCCACAGAAAGA | AGCGTGAGGACAAAGCAGAT |
NONHSAT250051.1 | CGTGTCATTAGAGCAGCAGG | GCAGGAGGTCAAACCGTAGA |
NONHSAT192459.1 | GTAAGGACTGAGGACCGACAATGC | CGCTCTTGGTGTGGAACTAAGTGG |
hsa-miR-486-3p | TATACGGGGCAGCTCAGTACAGG | — |
hsa-miR-6817-5p | TATCTGCCATAGGAAGCTTGGAGTGG | — |
hsa-miR-20b-5p | CGCAAAGTGCTCATAGTGCAGGTAG | — |
hsa-miR-204-5p | CGCTTCCCTTTGTCATCCTATGCC | — |
hsa-miR-138-5p | AGCTGGTGTTGTGAATCAGGCC | — |
hsa-miR-338-5p | CCGAACAATATCCTGGTGCTGAGTG | — |
hsa-miR-20b-3p | CGACTGTAGTATGGGCACTTCCAG | — |
hsa-miR-625-5p | GCGAGGGGGAAAGTTCTATAGTCC | — |
hsa-miR-34c-5p | CCAGGCAGTGTAGTTAGCTGATTGC | — |
hsa-miR-135a-3p | TATAGGGATTGGAGCCGTGGC | — |
hsa-miR-512-3p | CGAAGTGCTGTCATAGCTGAGGTC | — |
hsa-miR-34b-5p | GCGTAGGCAGTGTCATTAGCTGATTG | — |
hsa-miR-183-5p | CCGCTATGGCACTGGTAGAATTCACT | — |
hsa-miR-455-3p | CCGCAGTCCATGGGCATATACA | — |
hsa-miR-181b-5p | AACATTCATTGCTGTCGGTGGGT | — |
NOTCH1 | CGCTGACGGAGTACAAGTG | GTAGGAGCCGACCTCGTTG |
CCND1 | CAATGACCCCGCACGATTTC | CATGGAGGGCGGATTGGAA |
BCL2L11 | TAAGTTCTGAGTGTGACCGAGA | GCTCTGTCTGTAGGGAGGTAGG |
GNB4 | TGCGAACAAGACGTACACTGA | TGAGAAGCACTGACTAGCAGC |
CDK1 | AAACTACAGGTCAAGTGGTAGCC | TCCTGCATAAGCACATCCTGA |
IGF1R | TCGACATCCGCAACGACTATC | GCCTCCTTAGATCACAGCTCC |
SOX4 | AGCGACAAGATCCCTTTCATTC | CGTTGCCGGACTTCACCTT |
CDK6 | TCTTCATTCACACCGAGTAGTGC | TGAGGTTAGAGCCATCTGGAAA |
IGF1 | GCTCTTCAGTTCGTGTGTGGA | GCCTCCTTAGATCACAGCTCC |
SLC2A4 | TGGGCGGCATGATTTCCTC | GCCAGGACATTGTTGACCAG |
ERBB4 | GTCCAGCCCAGCGATTCTC | AGAGCCACTAACACGTAGCCT |
GATA3 | GCCCCTCATTAAGCCCAAG | TTGTGGTGGTCTGACAGTTCG |
PRKCA | GTCCACAAGAGGTGCCATGAA | AAGGTGGGGCTTCCGTAAGT |
TGFBR2 | GTAGCTCTGATGAGTGCAATGAC | CAGATATGGCAACTCCCAGTG |
RPS18F | GCGGCGGAAAATAGCCTTTG | GATCACACGTTCCACCTCATC |
Statistical analysis
The Student’s t-test was used to evaluate the differences of mRNAs, miRNAs and lncRNAs expression between cancer and normal samples. Pathway enrichment analysis was estimated by Fisher’s test, and false discovery rate (FDR) was calculated to correct the P values. The results of RT-qPCR were repeated three times for the three matched samples. Data were presented as mean ± standard deviation. Statistical analysis was performed using GraphPad Prism 8.0.2 (GraphPad Software, USA). Differences between the two groups were determined by t-test. P<0.05 was considered statistically significant.
Results
Identification of DE-mRNAs, DE-lncRNAs, and DE-miRNAs
In total, 13 SACC tissues and 7 NSG tissues were available in the GSE88804 dataset, and 3 SACC tissues and 3 NSG tissues were available in the GSE117275 dataset. Through representational difference analysis, we identified 1,611 DE-mRNAs (745 up-regulated and 866 down-regulated), 155 DE-lncRNAs (103 up-regulated and 52 down-regulated), and 82 DE-miRNAs (39 up-regulated and 43 down-regulated). Detailed results are shown in Table 2, and clustergrams of DE-lncRNAs, DE-miRNAs, and DE-mRNAs were generated (shown in Figure 2).
Table 2
Gene | Gene number | Up-regulation gene number | Down-regulation gene number |
---|---|---|---|
lncRNA | 155 | 103 | 52 |
miRNA | 82 | 39 | 43 |
mRNA | 1611 | 745 | 866 |

GO and KEGG analyses of significant DE-mRNAs
The significantly DE-mRNAs were utilized for GO and KEGG analyses. The results revealed that these mRNAs were mainly involved in 853 GO terms and 77 KEGG pathways. The up and down-regulated genes were mainly enriched for the following GO terms: regulation of transcription DNA-templated, transcription, cell division, negative regulation of transcription from the RNA polymerase II promoter, cell proliferation, oxidation-reduction process, signal transduction, positive regulation of transcription from RNA polymerase II promoter, neutrophil degranulation, negative regulation of cell proliferation. The top 25 GO functions according to the P value are shown in Figure 3. The most significant KEGG pathway terms for the DE-mRNAs are in cell cycle, pathways in cancer, PI3K-Akt signaling pathway, breast cancer, microRNAs in cancer, metabolic pathways, salivary secretion, PPAR signaling pathway, pathways in cancer, AMPK signaling pathway. By combining GO and KEGG pathway analyses, 539 significant mRNAs were obtained. The top 25 KEGG pathways according to the P value are shown in Figure 4.
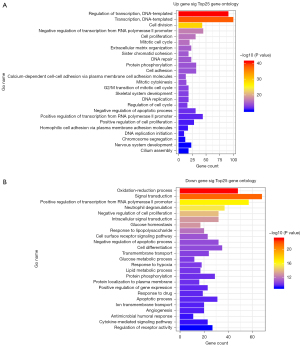
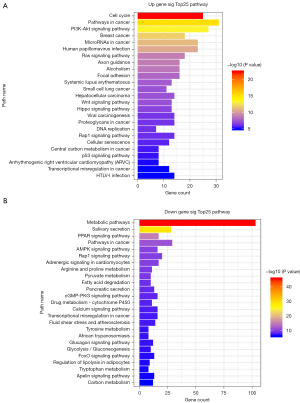
Construction of the lncRNA-miRNA-mRNA network
By predicting 155 DE-lncRNAs, 82 DE-miRNAs, and 539 mRNAs, we constructed 535 lncRNA-miRNA interaction pairs and 419 miRNA-mRNA interaction pairs. A total of 76 lncRNAs and 80 miRNAs were included in the lncRNA-miRNA pairs, and 73 miRNAs and 192 mRNAs were included in the miRNA-mRNA pairs. Depending on the interactions between lncRNA-miRNA and miRNA-mRNA, we screened the negative correlation of miRNA-mRNA and miRNA-lncRNA pairs and constructed two lncRNA-miRNA-mRNA ceRNA networks. The lncRNA (down-regulated)-miRNA (up-regulated)-mRNA (down-regulated) network consisted of 22 lncRNAs, 31 miRNAs, and 71 mRNAs with a total of 480 interactions. The lncRNA (up-regulated)-miRNA (down-regulated)-mRNA (up-regulated) network consisted of 38 lncRNAs, 18 miRNAs, and 32 mRNAs with a total of 344 interactions. Subsequently, we combined the files of these pairs and visualized them using Cytoscape, yielding a ceRNA topology network (Figure 5).
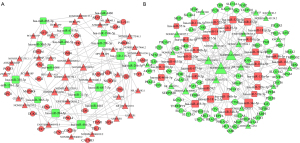
PPI network construction
Based on the STRING database, we used the ceRNA-related DE-mRNAs to build a PPI network for investigating the function of DE-mRNAs at the protein level and filtering for functional genes. The PPI network is composed of 59 up-regulated mRNAs and 55 down-regulated mRNAs. The PPI network is shown in Figure 6.
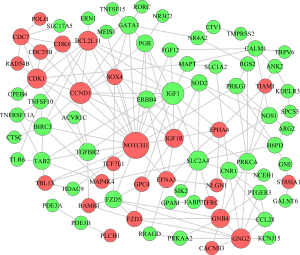
Validation of part of the DE-RNAs using qRT-PCR
To verify the results of bioinformation analysis, a total of 33 DE-RNA transcripts were validated, including 4 lncRNAs, 14 mRNAs, and 15 miRNAs, the selected DE-RNAs are shown in Table 3. The results of 29 (87.9%, 29/33) RNA transcriptions were consistent with the sequencing data (P<0.05) (Figure 7) by comparing the expression level in SACC tissues and NSG tissues, except for has-mi-34c-5p, has-mi-135a-3p, has-mi-34b-5p, has-mi-20b-3p.
Table 3
RNA Symbol | Log FC | P value | Style | Type of gene |
---|---|---|---|---|
NONHSAT251752.1 | 1.889353187 | 0.000632343 | Up | LncRNA |
NONHSAT232163.1 | −1.068788242 | 5.37617E-10 | Down | LncRNA |
NONHSAT250051.1 | −1.27976978 | 2.23249E-06 | Down | LncRNA |
NONHSAT192459.1 | −2.835246154 | 8.9695E-06 | Down | LncRNA |
NONHSAT114577.2 | −1.224763516 | 0.000396321 | Down | LncRNA |
hsa-miR-486-3p | −4.576017794 | 8.89977E-07 | Down | MiRNA |
hsa-miR-6817-5p | −4.000334253 | 0.000341183 | Down | MiRNA |
hsa-miR-20b-5p | −2.960689838 | 4.49293E-06 | Down | MiRNA |
hsa-miR-204-5p | −2.684075649 | 0.000367464 | Down | MiRNA |
hsa-miR-138-5p | −5.554665948 | 1.23631E-18 | Down | MiRNA |
hsa-miR-338-5p | −2.61678987 | 1.8934E-06 | Down | MiRNA |
hsa-miR-20b-3p | −2.93874657 | 0.000365336 | Down | MiRNA |
hsa-miR-625-5p | −4.491772088 | 6.76427E-11 | Down | MiRNA |
hsa-miR-31-5p | −3.067973863 | 4.03445E-05 | Down | MiRNA |
hsa-miR-34c-5p | 3.049043997 | 0.000138147 | Up | MiRNA |
hsa-miR-135a-3p | 4.724569925 | 2.81884E-06 | Up | MiRNA |
hsa-miR-512-3p | 4.558235486 | 5.66518E-05 | Up | MiRNA |
hsa-miR-34b-5p | 3.375134442 | 1.90128E-05 | Up | MiRNA |
hsa-miR-183-5p | 2.92955842 | 1.09418E-07 | Up | MiRNA |
hsa-miR-455-3p | 5.334890663 | 9.89814E-21 | Up | MiRNA |
hsa-miR-181b-5p | 3.066406127 | 2.91677E-09 | Up | MiRNA |
hsa-miR-93-3p | 2.814756293 | 9.99313E-08 | Up | MiRNA |
hsa-miR-512-5p | 4.620712911 | 0.000162247 | Up | MiRNA |
NOTCH1 | 1.853868791 | 2.01423E-09 | Up | Protein-coding |
CCND1 | 1.538207033 | 1.56042E-09 | Up | Protein-coding |
BCL2L11 | 1.425769231 | 4.19707E-08 | Up | Protein-coding |
GNB4 | 1.480336923 | 2.53083E-06 | Up | Protein-coding |
CDK1 | 2.069204615 | 1.27211E-08 | Up | Protein-coding |
IGF1R | 1.17767011 | 2.80746E-10 | Up | Protein-coding |
SOX4 | 2.011386813 | 1.8408E-14 | Up | Protein-coding |
CDK6 | 2.635606593 | 3.19174E-13 | Up | Protein-coding |
IGF1 | −1.983501209 | 2.11522E-07 | Down | Protein-coding |
SLC2A4 | −1.098253187 | 3.83538E-11 | Down | Protein-coding |
SLC2A4 | −1.098253187 | 3.83538E-11 | Down | Protein-coding |
ERBB4 | −2.921263077 | 2.09929E-07 | Down | Protein-coding |
GATA3 | −1.860703407 | 2.2411E-07 | Down | Protein-coding |
GATA3 | −1.860703407 | 2.2411E-07 | Down | Protein-coding |
PRKCA | −1.517311868 | 5.94524E-07 | Down | Protein-coding |
TGFBR2 | −1.078917912 | 1.82159E-05 | Down | Protein-coding |
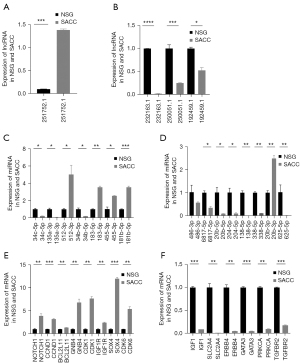
The selection of lncRNA-miRNA-mRNA regulatory axis
According to the node degree, the regulation and control abilities in the PPI network, P values, and combined with ceRNA network, we screened NONHSAT251752.1-hsa-miR-6817-5p-NOTCH1, NONHSAT251752.1-hsa-miR-204-5p/hsa-miR-138-5p-CDK6, NONHSAT232163.1/NONHSAT250051.1/NONHSAT192459.1-hsa-miR-34c-5p/hsa-miR-135a-3p-IGF1. In bioinformation analysis, NONHSAT251752.1, hsa-miR-34c-5p, hsa-miR-135a-3p, NOTCH1 were up-regulated in SACC patients, while NONHSAT232163.1, NONHSAT250051.1, NONHSAT192459.1, hsa-miR-6817-5p, hsa-miR-204-5p, hsa-miR-138-5p, IGF1 were down-regulated in SACC patients. In the experiment of qRT-PCR, hsa-miR-34c-5p, hsa-miR-135a-3p were inconsistent with bioinformation analysis (P<0.05) as shown in Figure 8. Therefore, we determined NONHSAT251752.1-hsa-miR-6817-5p-NOTCH1, NONHSAT251752.1-hsa-miR-204-5p/hsa-miR-138-5p-CDK6 as the key regulatory axise.
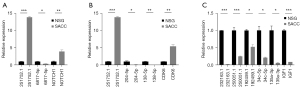
Discussion
SACC has a high degree of malignancy with extensive invasive and distant metastasis, especially neurotropic invasion and distant lung metastasis (2,3,24). Clinically, SACC has the characteristics of easy recurrence, high metastasis rate, poor long-term efficacy, low cure rate, and poor prognosis (4,25). However, the molecular mechanisms underlying SACC progression and metastasis are still elusive. Recent studies have suggested that ncRNAs, including miRNAs and lncRNAs, play important roles in cancer initiation and progression (26-28). In SACC, Xie et al. found that upregulation of the lncRNA ADAMTS9-AS2 promotes SACC metastasis (29). Chen et al. reported that the lncRNA MRPL23-AS1 promoted cystic carcinoma lung metastasis (30). In addition, miR-21 (31), miRNA-140-5p (32), miR-24-3p (33), miR-93-5p (34), miR-125a-5p (35), and miR-103a-3p (36) have been reported in cancer in recent years.
In 2011, Salmena et al. first proposed the ceRNA hypothesis (13). In ceRNA theory, endogenous RNAs, including lncRNAs, competitively bind to miRNAs to prevent miRNAs from regulating corresponding target genes. Therefore, the lncRNA-miRNA-mRNA network was constructed. To date, the ceRNA hypothesis has been used to build lncRNA-miRNA-mRNA networks in many cancers, such as MALAT1-miR-106b-5p-SLAIN2 in colorectal cancer (37) and lncRNA H19-miR-194-PFTK1 in pancreatic cancer (38). Moreover, a ceRNA axis has been discovered and reported in squamous cell carcinoma of the tongue (39), breast cancer (40), and a variety of other tumors. However, a ceRNA network has not been reported in SACC. To the best of our knowledge, this is the first study to investigate a specific ceRNA network in SACC.
In our study, we present the different expression profiles of lncRNA, miRNA, mRNA in SACC by bioinformation analysis, attempting to further understand the molecular mechanism of SACC. Based on the filtration by the absolute of log2 fold change >1, FDR <0.05, and P<0.05, We identified a total of 1,611 DE-mRNAs (745 up-regulated and 866 down-regulated), 155 DE-lncRNAs (103 up-regulated and 52 down-regulated), and 82 DE-miRNAs (39 up-regulated and 43 down-regulated). For the 1,611 DE-mRNAs, 853 GO terms and 77 KEGG pathways were enriched. By GO and KEGG analyses of the DE-mRNAs, 539 intersecting mRNAs were obtained. The most significant KEGG pathway terms for the DE-mRNAs are in cell cycle and metabolic pathways. Moreover, the most significant KEGG pathway terms of the downregulation DE-mRNAs is metabolic pathways. Metabolism is closely related to epithelial mesenchyme in ACC (41), which indicate that metabolism plays an important role. In addition, we tested the reliability of bioinformatics analysis by qRT-PCR, 29 (87.9%, 29/33) DE-RNAs were consistent with bioinformatics analysis, only 4 DE-miRNAs had the opposite results by comparing with NSG tissues. In the process of verifying the reliability of bioinformatics analysis, we also found a significantly high-expressed gene SOX4, and knockdown of SOX4 expression induces apoptosis in ACC3 cells in the literature (42). In the end, according to the node degree, the regulation and control abilities in the PPI network, P values, and combined with ceRNA network, NONHSAT251752.1-hsa-miR-6817-5p-NOTCH1, NONHSAT251752.1-hsa-miR-204-5p/hsa-miR-138-5p-CDK6 regulatory axises were identified.
NOTCH family of receptors including NOTCH1-4 regulate essential cellular functions linked with cell fate specification (43). Clinical studies have highlighted that Notch signaling impacts survival in human cancers (44). NOTCH1 is the most extensively studied and characterized NOTCH family member because its mutation prevalence among human cancers is higher than that for other members (45,46). Its deregulation can lead to changes in cancer-relevant functions such as proliferation, stemness, epithelial-mesenchymal transition, and angiogenesis (47). Aberrant NOTCH1 signaling is implicated in the progression of various cancer types including breast cancer (48), leukemia (49), HNSCC (50), and other cancers. Whole exome sequencing (WES) of ACC samples has shed light on 12.5% mutation rate of primary disease (51), and in recurrent and metastatic ACCs, the mutation rate even reached 33% (52). Additionally, a study showed that NOTCH1 mutation has been defined as a distinct group characterized by solid histology, with a propensity to bone and liver metastasis, and poor prognosis (53). Notch-1 knockdown suppresses the growth and migration of SACC cells in vitro and the metastasis of SACC cells in vivo (54). In conclusion, Notch-1 plays an important role in SACC and it is a vital candidate target in SACC. In our study, NOTCH1 has the most complex interaction with other mRNAs in PPI interactions and it was confirmed to be elevated in SACC tissues, which was consistent with the result of the previous study (55).
The family of cyclin-dependent kinases (CDKs) covers 13 different serine/threonine kinases. CDK6 is known as classic cell cycle kinases that facilitate the progression of cells through the early G1 phase of the cell cycle by forming complexes with D-type cyclins (D1, D2, and D3). CDK6 plays an important role in promoting cancer initiation, especially in hematologic malignancies, breast cancer, melanoma. At the same time, CDK6 is also inextricably linked to immune functions (56). Overexpression of CDK6 has been reported in T-cell lymphoblastic lymphoma (57) and leukemia (T-LBL or T-ALL) and in B-lymphoid malignancies (58), which is consistent with our result. According to studies, the regulatory axis of ceRNA targeting CDK6 has been reported many times. LncRNA AWPPH accelerates the progression of non-small cell lung cancer by sponging miRNA-204 to upregulate CDK6 (59). RP11-480I12.5-004 Promotes Growth and Tumorigenesis of Breast Cancer by Relieving miR-29c-3p-Mediated AKT3 and CDK6 degradation (60).
In the regulatory axises of NONHSAT251752.1-hsa-miR-6817-5p-NOTCH1, and NONHSAT251752.1-hsa-miR-204-5p/hsa-miR-138-5p-CDK6, hsa-miR-6817-5p is reported combined with circRFWD2 and circINO80 inducing osteogenic differentiation of hASCs (61). has-miR-204-5p was competitively bound to PBB12 affecting the proliferation and invasion of osteosarcoma cells (62). It is worth mentioning that through six matched SACC tissue samples and corresponding para-carcinoma tissues were examined by secondary sequencing, miR-138-5p was down-regulation and then the verification by qRT-PCR also proved the down-regulation of miR-138-5p expression in SACC tissues (63). These aforementioned literatures further imply the accuracy of our current analytic results.
Conclusions
Through bioinformatics analyses, the significantly upregulated and downregulated RNA and pathways in SACC were found, constructing the lncRNA-miRNA-mRNA interaction network and PPI network in SACC. Moreover, we build NONHSAT251752.1-hsa-miR-6817-5p-NOTCH1, NONHSAT251752.1-hsa-miR-204-5p/hsa-miR-138-5p-CDK6 regulatory networks. However, the detailed regulation effect and underlying mechanism of the regulatory axis still need further investigation and it would be our focus in future work. This study provides some key clues for molecular mechanistic investigations of SACC in the future.
Acknowledgments
Funding: This work was supported by the National Key Research and Development Program of China (No. 2016YFC1102800 and 2016YFC1102805).
Footnote
Reporting Checklist: The authors have completed the MDAR reporting checklist. Available at https://dx.doi.org/10.21037/tcr-21-1771
Data Sharing Statement: Available at https://dx.doi.org/10.21037/tcr-21-1771
Peer Review File: Available at https://dx.doi.org/10.21037/tcr-21-1771
Conflicts of Interest: All authors have completed the ICMJE uniform disclosure form (available at https://dx.doi.org/10.21037/tcr-21-1771). The authors have no conflicts of interest to declare.
Ethical Statement: The authors are accountable for all aspects of the work in ensuring that questions related to the accuracy or integrity of any part of the work are appropriately investigated and resolved. The study was conducted in accordance with the Declaration of Helsinki (as revised in 2013). The study was approved by the Ethics Committee and was conducted under the guidance of international ethical standards (IRB number: PKUSSIRB-202056098). Written informed consent was obtained from all patients.
Open Access Statement: This is an Open Access article distributed in accordance with the Creative Commons Attribution-NonCommercial-NoDerivs 4.0 International License (CC BY-NC-ND 4.0), which permits the non-commercial replication and distribution of the article with the strict proviso that no changes or edits are made and the original work is properly cited (including links to both the formal publication through the relevant DOI and the license). See: https://creativecommons.org/licenses/by-nc-nd/4.0/.
References
- Spiro RH, Huvos AG, Strong EW. Adenoid cystic carcinoma of salivary origin. A clinicopathologic study of 242 cases. Am J Surg 1974;128:512-20. [Crossref] [PubMed]
- Szanto PA, Luna MA, Tortoledo ME, et al. Histologic grading of adenoid cystic carcinoma of the salivary glands. Cancer 1984;54:1062-9. [Crossref] [PubMed]
- Tang Y, Liang X, Zheng M, et al. Expression of c-kit and Slug correlates with invasion and metastasis of salivary adenoid cystic carcinoma. Oral Oncol 2010;46:311-6. [Crossref] [PubMed]
- Xu B, Drill E, Ho A, et al. Predictors of Outcome in Adenoid Cystic Carcinoma of Salivary Glands: A Clinicopathologic Study With Correlation Between MYB Fusion and Protein Expression. Am J Surg Pathol 2017;41:1422-32. [Crossref] [PubMed]
- Terhaard CH, Lubsen H, Van der Tweel I, et al. Salivary gland carcinoma: independent prognostic factors for locoregional control, distant metastases, and overall survival: results of the Dutch head and neck oncology cooperative group. Head Neck 2004;26:681-92; discussion 692-3. [Crossref] [PubMed]
- van Weert S, Bloemena E, van der Waal I, et al. Adenoid cystic carcinoma of the head and neck: a single-center analysis of 105 consecutive cases over a 30-year period. Oral Oncol 2013;49:824-9. [Crossref] [PubMed]
- Wysocki PT, Izumchenko E, Meir J, et al. Adenoid cystic carcinoma: emerging role of translocations and gene fusions. Oncotarget 2016;7:66239-54. [Crossref] [PubMed]
- Mercer TR, Dinger ME, Mattick JS. Long non-coding RNAs: insights into functions. Nat Rev Genet 2009;10:155-9. [Crossref] [PubMed]
- Fatica A, Bozzoni I. Long non-coding RNAs: new players in cell differentiation and development. Nat Rev Genet 2014;15:7-21. [Crossref] [PubMed]
- Kim VN, Nam JW. Genomics of microRNA. Trends Genet 2006;22:165-73. [Crossref] [PubMed]
- Zhang Y, Wang Z, Gemeinhart RA. Progress in microRNA delivery. J Control Release 2013;172:962-74. [Crossref] [PubMed]
- Li J, Tan S, Kooger R, et al. MicroRNAs as novel biological targets for detection and regulation. Chem Soc Rev 2014;43:506-17. [Crossref] [PubMed]
- Salmena L, Poliseno L, Tay Y, et al. A ceRNA hypothesis: the Rosetta Stone of a hidden RNA language? Cell 2011;146:353-8. [Crossref] [PubMed]
- Shi X, Xu Y, Zhang C, et al. Subpathway-LNCE: Identify dysfunctional subpathways competitively regulated by lncRNAs through integrating lncRNA-mRNA expression profile and pathway topologies. Oncotarget 2016;7:69857-70. [Crossref] [PubMed]
- Li Z, Yang L, Liu X, et al. Long noncoding RNA MEG3 inhibits proliferation of chronic myeloid leukemia cells by sponging microRNA21. Biomed Pharmacother 2018;104:181-92. [Crossref] [PubMed]
- Qi M, Yu B, Yu H, et al. Integrated analysis of a ceRNA network reveals potential prognostic lncRNAs in gastric cancer. Cancer Med 2020;9:1798-817. [Crossref] [PubMed]
- Liu J, Li W, Zhang J, et al. Identification of key genes and long non-coding RNA associated ceRNA networks in hepatocellular carcinoma. PeerJ 2019;7:e8021. [Crossref] [PubMed]
- Bai X, Wang W, Zhao P, et al. LncRNA CRNDE acts as an oncogene in cervical cancer through sponging miR-183 to regulate CCNB1 expression. Carcinogenesis 2020;41:111-21. [Crossref] [PubMed]
- Wu Y, Deng Y, Guo Q, et al. Long non-coding RNA SNHG6 promotes cell proliferation and migration through sponging miR-4465 in ovarian clear cell carcinoma. J Cell Mol Med 2019;23:5025-36. [Crossref] [PubMed]
- Robinson MD, McCarthy DJ, Smyth GK. edgeR: a Bioconductor package for differential expression analysis of digital gene expression data. Bioinformatics 2010;26:139-40. [Crossref] [PubMed]
- Wright GW, Simon RM. A random variance model for detection of differential gene expression in small microarray experiments. Bioinformatics 2003;19:2448-55. [Crossref] [PubMed]
- Yu G, Wang LG, Han Y, et al. clusterProfiler: an R package for comparing biological themes among gene clusters. OMICS 2012;16:284-7. [Crossref] [PubMed]
- Smoot ME, Ono K, Ruscheinski J, et al. Cytoscape 2.8: new features for data integration and network visualization. Bioinformatics 2011;27:431-2. [Crossref] [PubMed]
- Kokemueller H, Eckardt A, Brachvogel P, et al. Adenoid cystic carcinoma of the head and neck--a 20 years experience. Int J Oral Maxillofac Surg 2004;33:25-31. [Crossref] [PubMed]
- Ho AS, Kannan K, Roy DM, et al. The mutational landscape of adenoid cystic carcinoma. Nat Genet 2013;45:791-8. [Crossref] [PubMed]
- Chan JJ, Tay Y. Noncoding RNA:RNA Regulatory Networks in Cancer. Int J Mol Sci 2018;19:1310. [Crossref] [PubMed]
- Bhan A, Soleimani M, Mandal SS. Long Noncoding RNA and Cancer: A New Paradigm. Cancer Res 2017;77:3965-81. [Crossref] [PubMed]
- Rupaimoole R, Slack FJ. MicroRNA therapeutics: towards a new era for the management of cancer and other diseases. Nat Rev Drug Discov 2017;16:203-22. [Crossref] [PubMed]
- Xie S, Yu X, Li Y, et al. Upregulation of lncRNA ADAMTS9-AS2 Promotes Salivary Adenoid Cystic Carcinoma Metastasis via PI3K/Akt and MEK/Erk Signaling. Mol Ther 2018;26:2766-78. [Crossref] [PubMed]
- Chen CW, Fu M, Du ZH, et al. Long Noncoding RNA MRPL23-AS1 Promotes Adenoid Cystic Carcinoma Lung Metastasis. Cancer Res 2020;80:2273-85. [Crossref] [PubMed]
- Jiang LH, Ge MH, Hou XX, et al. miR-21 regulates tumor progression through the miR-21-PDCD4-Stat3 pathway in human salivary adenoid cystic carcinoma. Lab Invest 2015;95:1398-408. [Crossref] [PubMed]
- Qiao Z, Zou Y, Zhao H. MicroRNA-140-5p inhibits salivary adenoid cystic carcinoma progression and metastasis via targeting survivin. Cancer Cell Int 2019;19:301. [Crossref] [PubMed]
- Zhang MX, Zhang J, Zhang H, et al. miR-24-3p Suppresses Malignant Behavior of Lacrimal Adenoid Cystic Carcinoma by Targeting PRKCH to Regulate p53/p21 Pathway. PLoS One 2016;11:e0158433. [Crossref] [PubMed]
- Hao J, Jin X, Shi Y, et al. miR-93-5p enhance lacrimal gland adenoid cystic carcinoma cell tumorigenesis by targeting BRMS1L. Cancer Cell Int 2018;18:72. [Crossref] [PubMed]
- Liang Y, Ye J, Jiao J, et al. Down-regulation of miR-125a-5p is associated with salivary adenoid cystic carcinoma progression via targeting p38/JNK/ERK signal pathway. Am J Transl Res 2017;9:1101-13. [PubMed]
- Fu M, Chen CW, Yang LQ, et al. MicroRNA-103a-3p promotes metastasis by targeting TPD52 in salivary adenoid cystic carcinoma. Int J Oncol 2020;57:574-86. [Crossref] [PubMed]
- Zhuang M, Zhao S, Jiang Z, et al. MALAT1 sponges miR-106b-5p to promote the invasion and metastasis of colorectal cancer via SLAIN2 enhanced microtubules mobility. EBioMedicine 2019;41:286-98. [Crossref] [PubMed]
- Sun Y, Zhu Q, Yang W, et al. LncRNA H19/miR-194/PFTK1 axis modulates the cell proliferation and migration of pancreatic cancer. J Cell Biochem 2019;120:3874-86. [Crossref] [PubMed]
- Zhou RS, Zhang EX, Sun QF, et al. Integrated analysis of lncRNA-miRNA-mRNA ceRNA network in squamous cell carcinoma of tongue. BMC Cancer 2019;19:779. [Crossref] [PubMed]
- Fan CN, Ma L, Liu N. Systematic analysis of lncRNA-miRNA-mRNA competing endogenous RNA network identifies four-lncRNA signature as a prognostic biomarker for breast cancer. J Transl Med 2018;16:264. [Crossref] [PubMed]
- Jiang YP, Tang YL, Wang SS, et al. PRRX1-induced epithelial-to-mesenchymal transition in salivary adenoid cystic carcinoma activates the metabolic reprogramming of free fatty acids to promote invasion and metastasis. Cell Prolif 2020;53:e12705. [Crossref] [PubMed]
- Pramoonjago P, Baras AS, Moskaluk CA. Knockdown of Sox4 expression by RNAi induces apoptosis in ACC3 cells. Oncogene 2006;25:5626-39. [Crossref] [PubMed]
- Artavanis-Tsakonas S. The molecular biology of the Notch locus and the fine tuning of differentiation in Drosophila. Trends Genet 1988;4:95-100. [Crossref] [PubMed]
- Donnem T, Andersen S, Al-Shibli K, et al. Prognostic impact of Notch ligands and receptors in nonsmall cell lung cancer: coexpression of Notch-1 and vascular endothelial growth factor-A predicts poor survival. Cancer 2010;116:5676-85. [Crossref] [PubMed]
- Sanchez-Vega F, Mina M, Armenia J, et al. Oncogenic Signaling Pathways in The Cancer Genome Atlas. Cell 2018;173:321-337.e10. [Crossref] [PubMed]
- Campbell JD, Yau C, Bowlby R, et al. Genomic, Pathway Network, and Immunologic Features Distinguishing Squamous Carcinomas. Cell Rep 2018;23:194-212.e6. [Crossref] [PubMed]
- Aster JC, Pear WS, Blacklow SC. The Varied Roles of Notch in Cancer. Annu Rev Pathol 2017;12:245-75. [Crossref] [PubMed]
- Jiao X, Wood LD, Lindman M, et al. Somatic mutations in the Notch, NF-KB, PIK3CA, and Hedgehog pathways in human breast cancers. Genes Chromosomes Cancer 2012;51:480-9. [Crossref] [PubMed]
- Puente XS, Pinyol M, Quesada V, et al. Whole-genome sequencing identifies recurrent mutations in chronic lymphocytic leukaemia. Nature 2011;475:101-5. [Crossref] [PubMed]
- Agrawal N, Frederick MJ, Pickering CR, et al. Exome sequencing of head and neck squamous cell carcinoma reveals inactivating mutations in NOTCH1. Science 2011;333:1154-7. [Crossref] [PubMed]
- Wang S, Yu Y, Fang Y, et al. Whole-exome sequencing reveals genetic underpinnings of salivary adenoid cystic carcinoma in the Chinese population. J Genet Genomics 2020;47:397-401. [Crossref] [PubMed]
- Morris LGT, Chandramohan R, West L, et al. The Molecular Landscape of Recurrent and Metastatic Head and Neck Cancers: Insights From a Precision Oncology Sequencing Platform. JAMA Oncol 2017;3:244-55. [Crossref] [PubMed]
- Ferrarotto R, Mitani Y, Diao L, et al. Activating NOTCH1 Mutations Define a Distinct Subgroup of Patients With Adenoid Cystic Carcinoma Who Have Poor Prognosis, Propensity to Bone and Liver Metastasis, and Potential Responsiveness to Notch1 Inhibitors. J Clin Oncol 2017;35:352-60. [Crossref] [PubMed]
- Chen W, Cao G, Yuan X, et al. Notch-1 knockdown suppresses proliferation, migration and metastasis of salivary adenoid cystic carcinoma cells. J Transl Med 2015;13:167. [Crossref] [PubMed]
- Bell D, Hanna EY, Miele L, et al. Expression and significance of notch signaling pathway in salivary adenoid cystic carcinoma. Ann Diagn Pathol 2014;18:10-3. [Crossref] [PubMed]
- Nebenfuehr S, Kollmann K, Sexl V. The role of CDK6 in cancer. Int J Cancer 2020;147:2988-95. [Crossref] [PubMed]
- Chilosi M, Doglioni C, Yan Z, et al. Differential expression of cyclin-dependent kinase 6 in cortical thymocytes and T-cell lymphoblastic lymphoma/leukemia. Am J Pathol 1998;152:209-17. [PubMed]
- Hayette S, Tigaud I, Callet-Bauchu E, et al. In B-cell chronic lymphocytic leukemias, 7q21 translocations lead to overexpression of the CDK6 gene. Blood 2003;102:1549-50. [Crossref] [PubMed]
- Wu D, Qin BY, Qi XG, et al. LncRNA AWPPH accelerates the progression of non-small cell lung cancer by sponging miRNA-204 to upregulate CDK6. Eur Rev Med Pharmacol Sci 2020;24:4281-7. [PubMed]
- Lou W, Ding B, Zhong G, et al. RP11-480I12.5-004 Promotes Growth and Tumorigenesis of Breast Cancer by Relieving miR-29c-3p-Mediated AKT3 and CDK6 Degradation. Mol Ther Nucleic Acids 2020;21:916-31. [Crossref] [PubMed]
- Huang X, Cen X, Zhang B, et al. The roles of circRFWD2 and circINO80 during NELL-1-induced osteogenesis. J Cell Mol Med 2019;23:8432-41. [Crossref] [PubMed]
- Ma T, Liu A, Xu D, et al. Mechanisms underlying the promotion of osteosarcoma cell proliferation and invasion by lncRNA PBB12. Oncol Rep 2020;43:736-46. [PubMed]
- Han J, Han N, Xu Z, et al. Expression profile of circular RNA and construction of circular RNA-Micro RNA network in salivary adenoid cystic carcinoma. Cancer Cell Int 2021;21:28. [Crossref] [PubMed]