An immune-associated ten-long noncoding RNA signature for predicting overall survival in cervical cancer
Introduction
According to the cancer statistics of China in 2015 (1), although the prevention, diagnosis, and treatment of cervical cancer has made great progress, the morbidity of cervical cancer has had an upward tendency in China in comparison with other developed countries. In recent years, there have been many studies on cervical cancer and numerous efforts have been made, but according to the global cancer statistics in 2018, compared with high-income countries, cancer-related deaths caused by cervical cancer are the main cause in 42 low-income countries and lower-middle-income countries (2). An optimal tool on prognosis prediction for cervical cancer is called for urgently to address this issue. Principal Component Analysis (PCA) is a multivariate statistical method to investigate the correlation between multiple variables. the internal structure of multiple variables were revealed through a few principal components and retain as much information as possible about the original variables.
Non-coding RNAs (ncRNAs) can be divided into short ncRNAs (length less than 200 nucleotides) and long ncRNAs (longer than 200 nucleotides). The long non-coding RNAs (lncRNAs) consist of long intergenic ncRNA (lincRNA), intronic lncRNA, antisense lncRNA, transcribed pseudogene lncRNA, and enhancer RNA (eRNA) (3). They have been shown to play important roles in regulating the innate immune response (4), and have been closely related with the cancer-immunity cycle, such as antigen release, antigen presentation, immune cells differentiation, immune cells migration, T cells infiltration, as well as the recognition and killing of cancer cells (5). LncRNA is abnormally expressed in a variety of malignant tumors and exerts a carcinogenic or anticancer role. Previous studies have found that lncRNA is closely related to cervical cancer and plays an important role in the occurrence, invasion, metastasis and drug resistance of cervical cancer.
Many researchers have already established relevant models for prognosis prediction based on clinical parameters (including α-fetoprotein, alanine aminotransferase, body mass index, tumor-node metastasis stage, tumor grade, tumor size, age, gender, vascular tumor cell, number of tumors, and so on) (6-8). Recently, several signature prognostic models were identified based on clinicopathological data, having displayed great advantages on cervical cancer prognosis prediction (9-13). Some immune-associated lncRNA signatures have been reported to be related with prognosis of cancer patients (14-17). Ye et al. finding demonstrated the value of lncRNAs in evaluating the immune infiltrate of the tumor. The immune-associated lncRNA signature could predict the prognosis of Cervical cancer and contribute to decisions regarding the immunotherapeutic strategy (18). These provide strong evidence for immunotherapy in the clinical setting. The occurrence and development of cervical cancer are closely related to inflammation and infection. Epidemiological results show that more than 90% of cervical cancer patients are infected with human papillomavirus (HPV). The combined action of many factors destroys cervical epithelial cells and induces imbalance of immune response. Currently, the immune-associated lncRNA signature for predicting overall survival (OS) in cervical cancer is unknown. This study is the first report on the immune-associated lncRNA signature for predicting OS in cervical cancer. We present the following article in accordance with the REMARK reporting checklist (available at https://dx.doi.org/10.21037/tcr-21-2390).
Methods
Databases
Recent studies have demonstrated that lncRNA plays a critical role in the immune system, and has become the focus of immunology. The occurrence, development and prognosis of various tumors were affected by lncRNA. In the study, the lncRNA expression profiles and clinical data of cervical cancer were acquired from The Cancer Genome Atlas (TCGA) database (https://portal.gdc.cancer.gov/). Immune-related genes were acquired from immune system process M13664 and immune response M19817 via the Molecular Signatures Database v 7.0 (MSigDB, http://www.broadinstitute.org/gsea/msigdb/index.jsp) (19,20). The study was conducted in accordance with the Declaration of Helsinki (as revised in 2013). If the survival time of patients was less than 1 month and clinical characteristics were deficient, the clinical datasets of these patients were excluded. The details are displayed in Table 1.
Table 1
Characteristics | Number of participants |
---|---|
Age | |
≤50 | 152 |
>50 | 89 |
Stage | |
I | 136 |
II | 53 |
III | 37 |
IV | 15 |
Status | |
Alive | 180 |
Dead | 61 |
Grade | |
G1 | 16 |
G2 | 124 |
G3 | 100 |
G4 | 1 |
Identification and Cox regression analyses of immune-associated lncRNAs
Immune-associated lncRNAs were identified from co-expression networks of lncRNAs and immune-associated genes. Univariate Cox regression analysis was used to identify the latent immune-associated lncRNAs with prognostic value in cervical cancer, filtered by the Pearson correlation coefficient ≥0.40 and the P value ≤0.001 via R version 3.6.1 (https://cran.r-project.org/bin/windows/base/old/3.6.1/). Then, the differentially expressed immune-associated lncRNAs were merged with survival time and status via R (survival) package in order to perform multivariate Cox regression analysis (21,22). Finally, the selected immune-associated lncRNAs (P≤0.01) were filtered by multivariate Cox regression analysis according to the Akaike Information Criterion (AIC) (23).
Construction of the signature
The least absolute shrinkage and selection operator (LASSO) Cox regression model was used to construct an immune-associated 10-lncRNA signature. The risk score method was assigned and assessed according to the mathematical formula: risk score = βgene [1] × Expression gene [1] +βgene [2] × Expression gene [2] + βgene [3] × Expression gene [3] + ... +βgene [n] × Expression gene [n]. The risk score of 10 immune-associated lncRNAs was calculated for each participant (19,24,25). The concordance index (C-index) was simultaneously used to evaluate the prediction accuracy of the signature in cervical cancer patients. Kaplan-Meier survival curves were performed by R version 3.6.1 (survival and survminer packages). The participants were divided into 2 groups (low-risk group and high-risk group). The Multi index receiver operating characteristic (ROC) curve was demonstrated utilizing the Survival ROC R package.
Statistical analysis
PCA was demonstrated utilizing R package. Gene Ontology (GO) and Kyoto Encyclopedia of Genes and Genomes (KEGG) associated with immunologic biological processes and pathways were extracted in the top 20 most significant terms via Gene Set Enrichment Analysis (GSEA) (version 4.0.3), with normalized enrichment score (NES) ≥1, nominal P value ≤0.05, false discovery rate (FDR), and q-value ≤0.25.
Results
Identification of immune-associated lncRNAs in cervical cancer
A total of 14,142 lncRNAs were identified from TCGA database among 306 cervical cancer samples and 3 non-cancer samples. A total of 331 immune-associated genes were extracted from MSigDB. A total of 685 immune-associated lncRNAs (Pearson correlation coefficient ≥0.40 and P≤0.001) were identified from co-expression networks of lncRNAs and immune-associated genes.
Acquisition of 10 immune-associated lncRNAs in cervical cancer patients
The differentially expressed immune-associated lncRNAs were merged with patient ID, survival time, and status via R (survival) package (P≤0.01). Univariate Cox regression analysis was used to identify the latent lncRNAs, and then 22 immune-associated lncRNAs were obtained to prepare for the multivariate Cox regression analysis (Figure 1). Finally, 10 immune-associated lncRNAs were identified for predicting the OS in cervical cancer patients, as presented in Table 2. The Kaplan-Meier survival curves of AL021807.1, AL109976.1, LINC02446, and MIR4458HG had statistical significance (P<0.05) (Figure 2). The Kaplan-Meier survival curves of AC004540.2, AC009065.8, AC083809.1, AC055822.1, AP000904.1, and FBXL19-AS1 did not have statistical significance (P>0.05, Table S1).
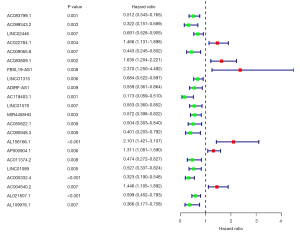
Table 2
ID | Coef | HR | HR.95L | HR.95H | P value |
---|---|---|---|---|---|
AC099343.2 | 0.648 | 1.912 | 0.842 | 4.346 | 0.122 |
LINC02446 | −0.396 | 0.673 | 0.4952 | 0.9146 | 0.0112 |
AC083809.1 | 0.833 | 2.300 | 1.6162 | 3.272 | 0.000 |
LINC01315 | −0.294 | 0.745 | 0.541 | 1.027 | 0.072 |
MIR4458HG | −0.854 | 0.426 | 0.282 | 0.643 | 0.000 |
AC090948.3 | −0.945 | 0.389 | 0.167 | 0.902 | 0.028 |
AC116312.1 | 0.739 | 2.093 | 1.531 | 2.861 | 0.000 |
AC004540.2 | 0.585 | 1.795 | 1.354 | 2.379 | 0.000 |
AL021807.1 | −0.587 | 0.556 | 0.413 | 0.750 | 0.000 |
AL109976.1 | −0.558 | 0.572 | 0.279 | 1.174 | 0.128 |
lncRNAs, long non-coding RNAs; Coef, correlation coefficient; HR, hazard ratio; HR.95L, the low limit value 95% CI of HR; HR.95H, the high limit value 95% CI of HR.
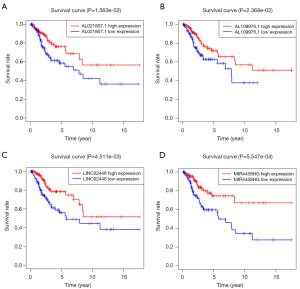
Construction of the immune-associated 10-lncRNA signature in cervical cancer patients
According to the risk score of LASSO Cox regression model, the participants were divided into 2 groups (low-risk group and high-risk group). There were 120 cervical cancer patients in the high-risk group and 121 cervical cancer patients in the low-risk group. The Kaplan-Meier survival curves of the signature had previous statistical significance (P=1.134e-10), and the 5-year survival probability was 0.444 in the high-risk group [95% confidence interval (CI): 0.334 to 0.590] and 0.884 in low-risk group (95% CI: 0.807 to 0.969) (Figure 3). The distribution of risk score, survival status with increasing the risk score, and expressions of 10 immune-associated lncRNAs in cervical cancer patients are displayed in Figure 4.
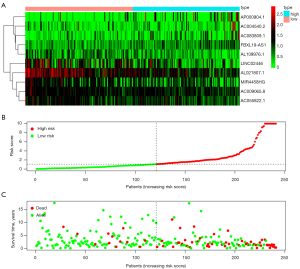
Analysis of independent risk factors with clinical characteristics
There were 241 cervical cancer patients with clinical characteristics (including age, grade, clinical stage) involved in the analysis of independent risk factors. After the univariate and multivariate Cox regression analyses with clinical characteristics, an immune-associated 10-lncRNA signature was shown to be an independent prognostic factor for predicting OS in cervical cancer patients (Figure 5). The Multi index ROC curves are shown in Figure 6. The area under curve (AUC) of the receiver operating characteristic curve of the signature was 0.833. The C-index was 0.788 (95% CI: 0.730 to 0.846, P=1.884778e-22).

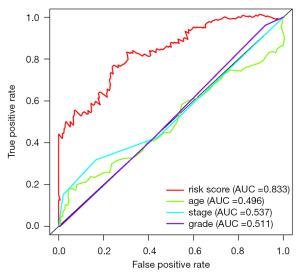
Functional analyses for high-risk group and low-risk groups
We used PCA to perform different distribution comparisons among all expression genes, immune genes, immune-associated lncRNAs, and 10 immune-associated lncRNAs between the low-risk group and high-risk group (Figure 7). Those significantly enriched on GO functional enrichment analysis and KEGG pathway analysis were up-regulated in the low-risk group (Figure 8).
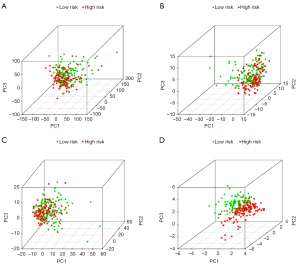
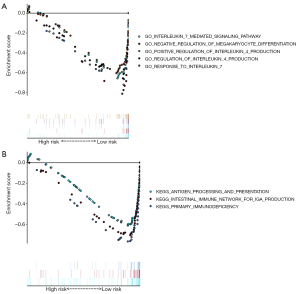
Discussion
Immune status is closely associated with human health, especially among cancer-related patients. The immune system plays an important role in cancer immunoediting (26). The immune response is characterized by its durable and adaptive features, and its self-propagating possibilities after activation (27). It has been reported that lncRNAs have abilities to control the differentiation of human monocytes into dendritic cells (DCs) (28). The DCs provide a connection in the innate and adaptive arms of the immune system as primary antigen presentation cells (APCs) for T cells (29). The lncRNAs acquired from CD8(+) T cells perform important roles in adaptive immunity (30), and lncRNAs have been defined as fine-tuners and key drivers in T cell differentiation (31).
In recent years, some immune-related lncRNAs and establishment of some prognostic models based on immune-related lncRNAs have provided us with a certain understanding of the immune status in cancer-related patients. There were 7 immune-related lncRNAs show to be independent prognostic risk factors in low-grade glioma (16). The lncRNA OSTN-AS1 was shown to be a potential immune-related prognostic marker for triple-negative breast cancer (32). Immune-related lncRNAs have been associated with prognostication in kidney renal clear cell carcinoma (33). An immune-related 6-lncRNA signature was verified as an independent prognostic factor for glioblastoma multiforme(14). A 9-immune-related lncRNA signature was closely associated with the immune status of pancreatic cancer (17). A 9-immune-related lncRNA signature was correlated with prognostication in anaplastic gliomas based on different immune status (34).
In this study, a total of 685 statistically significant immune-associated lncRNAs were identified from co-expression networks of lncRNAs and immune-associated genes. Through univariate Cox regression analysis, we identified 22 prognostic potential immune-related lncRNAs, among which, according to the hazard ratio (HR), 6 high-risk immune-related lncRNAs (HR >1) and 16 low-risk immune-related lncRNAs (HR <1) were associated with latent prognostic value. Finally, through Cox regression analyses and the LASSO Cox regression model, 10 immune-related lncRNAs (AC004540.2, AC083809.1, AP000904.1, FBXL19-AS1, LINC02446, AL109976.1, MIR4458HG, AC009065.8, AL021807.1, and AC055822.1) significantly have latent prognostic value in cervical cancer patients. Among the 10 immune-associated lncRNAs, only FBXL19-AS1 has been reported to be associated with cancers, and no other lncRNAs have been reported. The lncRNA FBXL19-AS1 is a potential oncogenic lncRNA and has been shown to act as a molecular sponge in colorectal cancer (35), osteosarcoma (36), breast cancer (37), and lung cancer (38,39).
Cervical cancer patients were divided into a high-risk group and low-risk group according to the HR of immune-related lncRNA. The HR of some immune-related lncRNA was larger than 1 (including AC004540.2, AC083809.1, AP000904.1, and FBXL19-AS1), which meant that these immune-associated lncRNAs may be harmful to the OS of cervical cancer patients. The HR of some immune-related lncRNA was less than 1 (including LINC02446, AL109976.1, MIR4458HG, AC009065.8, AL021807.1, and AC055822.1), which meant that these immune-associated lncRNAs may be protective factors the OS of cervical cancer patients. The Kaplan-Meier survival curves of AL021807.1, AL109976.1, LINC02446, and MIR4458HG were associated with the OS in cervical cancer patients. The Kaplan-Meier survival curves of the survival probability of 5 years were 0.444 in the high-risk group (95% CI: 0.334 to 0.590) and 0.884 in the low-risk group (95% CI: 0.807 to 0.969), which meant that the OS of cervical cancer patients was better in the low-risk group compared with the high-risk group. Multi index ROC curves showed that the immune-associated 10-lncRNA signature prognostic model could efficiently stratify the OS of cervical cancer patients as an independent factor, compared with other clinical characteristics. The area under the curve (AUC) of the prognostic model for 5-year survival was 0.833, combined with C-index (0.788, 95% CI: 0.730 to 0.846, P=1.884778e-22), indicating the efficient accuracy of the signature prognostic model. The PCA results showed that AC004540.2, AC083809.1, AP000904.1, and FBXL19-AS1 promote cervical cancer development while LINC02446, AL109976.1, MIR4458HG, AC009065.8, AL021807.1 and AC055822.1 has a protective effect on cervical cancer. The signature could adequately distinguish the high-risk patients and the low-risk patients.
For the sake of discovering the potential functions of the signature, signature-related protein coding genes (PCGs) co-expression networks were performed by GSEA. Surprisingly, we discovered that 5/20 GO functional enrichment analyses and 3/20 KEGG pathway analyses were up-regulated in low-risk groups in cervical cancer patients. These functional analyses were directly or indirectly associated with immunity. All these enriched gene sets indicated that the signature-related PCGs may participate in immunologic biological processes and pathways. Patients in the high-risk group and the low-risk group had previously different OS. The OS of the high-risk group was poorer than that of the low-risk group. The reason might be attributable to the enriched immunologic biological processes and pathways.
Conclusions
In summary, we identified an immune-associated 10-lncRNA signature prognostic model containing 10 lncRNAs (AC004540.2, AC083809.1, AP000904.1, FBXL19-AS1, LINC02446, AL109976.1, MIR4458HG, AC009065.8, AL021807.1, and AC055822.1). The signature is a dependable and measurable method to predict the prognosis of cervical cancer patients acting as an independent factor, and provides strong evidence for individualized immunotherapy for cervical cancer patients.
Acknowledgments
Funding: The present study was supported by the National Natural Science Foundation of China (81760466). The funders had no role in study design, data collection and analysis, decision to publish, or preparation of the manuscript.
Footnote
Reporting Checklist: The authors have completed the REMARK reporting checklist. Available at https://dx.doi.org/10.21037/tcr-21-2390
Conflicts of Interest: Both authors have completed the ICMJE uniform disclosure form (available at https://dx.doi.org/10.21037/tcr-21-2390). The authors have no conflicts of interest to declare.
Ethical Statement: The authors are accountable for all aspects of the work in ensuring that questions related to the accuracy or integrity of any part of the work are appropriately investigated and resolved. The study was conducted in accordance with the Declaration of Helsinki (as revised in 2013).
Open Access Statement: This is an Open Access article distributed in accordance with the Creative Commons Attribution-NonCommercial-NoDerivs 4.0 International License (CC BY-NC-ND 4.0), which permits the non-commercial replication and distribution of the article with the strict proviso that no changes or edits are made and the original work is properly cited (including links to both the formal publication through the relevant DOI and the license). See: https://creativecommons.org/licenses/by-nc-nd/4.0/.
References
- Chen W, Zheng R, Baade PD, et al. Cancer statistics in China, 2015. CA Cancer J Clin 2016;66:115-32. [Crossref] [PubMed]
- Bray F, Ferlay J, Soerjomataram I, et al. Global cancer statistics 2018: GLOBOCAN estimates of incidence and mortality worldwide for 36 cancers in 185 countries. CA Cancer J Clin 2018;68:394-424. [Crossref] [PubMed]
- Wang M, Sun X, Wang H, et al. Long non-coding RNAs in non-small cell lung cancer: functions and distinctions from other malignancies. Transl Cancer Res 2019;8:2636-53. [Crossref]
- Guttman M, Amit I, Garber M, et al. Chromatin signature reveals over a thousand highly conserved large non-coding RNAs in mammals. Nature 2009;458:223-7. [Crossref] [PubMed]
- Yu WD, Wang H, He QF, et al. Long noncoding RNAs in cancer-immunity cycle. J Cell Physiol 2018;233:6518-23. [Crossref] [PubMed]
- Chan AWH, Zhong J, Berhane S, et al. Development of pre and post-operative models to predict early recurrence of hepatocellular carcinoma after surgical resection. J Hepatol 2018;69:1284-93. [Crossref] [PubMed]
- Marrero JA, Feng Z, Wang Y, et al. Alpha-fetoprotein, des-gamma carboxyprothrombin, and lectin-bound alpha-fetoprotein in early hepatocellular carcinoma. Gastroenterology 2009;137:110-8. [Crossref] [PubMed]
- Liu GM, Xie WX, Zhang CY, et al. Identification of a four-gene metabolic signature predicting overall survival for hepatocellular carcinoma. J Cell Physiol 2020;235:1624-36. [Crossref] [PubMed]
- Ma C, Zhang W, Wu Q, et al. Identification of a microRNA signature associated with survivability in cervical squamous cell carcinoma. PLoS One 2018;13:e0193625. [Crossref] [PubMed]
- Ding TT, Ma H, Feng JH. A three-gene novel predictor for improving the prognosis of cervical cancer. Oncol Lett 2019;18:4907-15. [Crossref] [PubMed]
- Mao X, Qin X, Li L, et al. A 15-long non-coding RNA signature to improve prognosis prediction of cervical squamous cell carcinoma. Gynecol Oncol 2018;149:181-7. [Crossref] [PubMed]
- Mao Y, Dong L, Zheng Y, et al. Prediction of Recurrence in Cervical Cancer Using a Nine-lncRNA Signature. Front Genet 2019;10:284. [Crossref] [PubMed]
- Cai S, Yu X, Gu Z, et al. A 10-gene prognostic methylation signature for stage I-III cervical cancer. Arch Gynecol Obstet 2020;301:1275-87. [Crossref] [PubMed]
- Zhou M, Zhang Z, Zhao H, et al. An Immune-Related Six-lncRNA Signature to Improve Prognosis Prediction of Glioblastoma Multiforme. Mol Neurobiol 2018;55:3684-97. [PubMed]
- Wu S, Dai X, Xie D. Identification and Validation of an Immune-Related RNA Signature to Predict Survival of Patients With Head and Neck Squamous Cell Carcinoma. Front Genet 2019;10:1252. [Crossref] [PubMed]
- Li X, Meng Y. Survival analysis of immune-related lncRNA in low-grade glioma. BMC Cancer 2019;19:813. [Crossref] [PubMed]
- Wei C, Liang Q, Li X, et al. Bioinformatics profiling utilized a nine immune-related long noncoding RNA signature as a prognostic target for pancreatic cancer. J Cell Biochem 2019;120:14916-27. [Crossref] [PubMed]
- Ye J, Chen X, Lu W. Identification and Experimental Validation of Immune-Associate lncRNAs for Predicting Prognosis in Cervical Cancer. Onco Targets Ther 2021;14:4721-34. [Crossref] [PubMed]
- Subramanian A, Tamayo P, Mootha VK, et al. Gene set enrichment analysis: a knowledge-based approach for interpreting genome-wide expression profiles. Proc Natl Acad Sci U S A 2005;102:15545-50. [Crossref] [PubMed]
- Wang W, Zhao Z, Yang F, et al. An immune-related lncRNA signature for patients with anaplastic gliomas. J Neurooncol 2018;136:263-71. [Crossref] [PubMed]
- Lossos IS, Czerwinski DK, Alizadeh AA, et al. Prediction of survival in diffuse large-B-cell lymphoma based on the expression of six genes. N Engl J Med 2004;350:1828-37. [Crossref] [PubMed]
- Shannon P, Markiel A, Ozier O, et al. Cytoscape: A software environment for integrated models of biomolecular interaction networks. Genome Res 2003;13:2498-504. [Crossref] [PubMed]
- Zhang W, Zhang J, Yan W, et al. Whole-genome microRNA expression profiling identifies a 5-microRNA signature as a prognostic biomarker in Chinese patients with primary glioblastoma multiforme. Cancer 2013;119:814-24. [Crossref] [PubMed]
- Shi X, Le X, Li X, et al. A Practical Prognostic lncRNA Signature for Lung Squamous Cell Carcinoma. J Thorac Oncol 2017;12:S2099. [Crossref]
- Cai J, Zhang W, Yang P, et al. Identification of a 6-cytokine prognostic signature in patients with primary glioblastoma harboring M2 microglia/macrophage phenotype relevance. PLoS One 2015;10:e0126022. [Crossref] [PubMed]
- Bao ZS, Li MY, Wang JY, et al. Prognostic value of a nine-gene signature in glioma patients based on mRNA expression profiling. CNS Neurosci Ther 2014;20:112-8. [Crossref] [PubMed]
- Boussadia Z, Zanetti C, Parolini I. Role of microenvironmental acidity and tumor exosomes in cancer immunomodulation. Transl Cancer Res 2020;9:5775-86. [Crossref]
- Hamid O, Robert C, Daud A, et al. Safety and tumor responses with lambrolizumab (anti-PD-1) in melanoma. N Engl J Med 2013;369:134-44. [Crossref] [PubMed]
- Wang P, Xue Y, Han Y, et al. The STAT3-binding long noncoding RNA lnc-DC controls human dendritic cell differentiation. Science 2014;344:310-3. [Crossref] [PubMed]
- Steinman RM, Hemmi H. Dendritic cells: translating innate to adaptive immunity. Curr Top Microbiol Immunol 2006;311:17-58. [Crossref] [PubMed]
- Pang KC, Dinger ME, Mercer TR, et al. Genome-wide identification of long noncoding RNAs in CD8+ T cells. J Immunol 2009;182:7738-48. [Crossref] [PubMed]
- Ranzani V, Arrigoni A, Rossetti G, et al. Next-Generation Sequencing Analysis of Long Noncoding RNAs in CD4+ T Cell Differentiation. Methods Mol Biol 2017;1514:173-85. [Crossref] [PubMed]
- Liu Z, Mi M, Li X, et al. lncRNA OSTN-AS1 May Represent a Novel Immune-Related Prognostic Marker for Triple-Negative Breast Cancer Based on Integrated Analysis of a ceRNA Network. Front Genet 2019;10:850. [Crossref] [PubMed]
- Khadirnaikar S, Kumar P, Pandi SN, et al. Immune associated LncRNAs identify novel prognostic subtypes of renal clear cell carcinoma. Mol Carcinog 2019;58:544-53. [Crossref] [PubMed]
- Shen B, Yuan Y, Zhang Y, et al. Long non-coding RNA FBXL19-AS1 plays oncogenic role in colorectal cancer by sponging miR-203. Biochem Biophys Res Commun 2017;488:67-73. [Crossref] [PubMed]
- Pan R, He Z, Ruan W, et al. lncRNA FBXL19-AS1 regulates osteosarcoma cell proliferation, migration and invasion by sponging miR-346. Onco Targets Ther 2018;11:8409-20. [Crossref] [PubMed]
- Ding Z, Ye P, Yang X, et al. LncRNA FBXL19-AS1 promotes breast cancer cells proliferation and invasion via acting as a molecular sponge to miR-718. Biosci Rep 2019;39:BSR20182018. [Crossref] [PubMed]
- Jiang Q, Cheng L, Ma D, et al. FBXL19-AS1 exerts oncogenic function by sponging miR-431-5p to regulate RAF1 expression in lung cancer. Biosci Rep 2019;39:BSR20181804. [Crossref] [PubMed]
- Wang L, Zhang X, Liu Y, et al. Long noncoding RNA FBXL19-AS1 induces tumor growth and metastasis by sponging miR-203a-3p in lung adenocarcinoma. J Cell Physiol 2020;235:3612-25. [Crossref] [PubMed]
(English Language Editor: J. Jones)