Analysis of prognostic and therapeutic values of drug resistance-related genes in the lung cancer microenvironment
Introduction
Lung cancer is considered to be the most commonly reported cause of malignancy and mortality from cancer in China, accounting for 21% of all cancers and 27% of all cancer-related deaths (1). Epidemiologically, non-small cell lung cancer (NSCLC) is responsible for the predominant number of lung cancer cases. Its fatality rate is extremely high and the early five-year survival rate does not exceed 45% (2). It is becoming increasingly clear that the restrictions of the tumor nodal metastasis system for predicting prognosis in lung cancer patients. Thus, novel therapeutic targets such as kinases and immune checkpoint inhibitors have become the direction of exploration for many researchers (3,4). However, it must be acknowledged that they are not valuable enough, and there are few therapeutic targets and prognostic biomarkers for lung cancer (5,6).
Genetic alterations are considered to be an important mechanism of tumor drug resistance (7,8). In our previous study, through RNA sequencing analysis of lung cancer tissues of chemotherapy-sensitive patients and chemotherapy-insensitive patients, we found five significant drug resistance-related genes, including coiled-coil domain-containing protein 73 (CCDC73), dermatan sulfate epimerase like (DSEL), DLG associated protein 1 (DLGAP1), estrogen receptor 1 (ESR1), SEC14-like 5 (SEC14L5) (7). They had been proven to be associated with lung cancer (8-13) (Table 1). These drug resistance-related genes can directly or indirectly regulate tumor immunology and biological processes, thereby influencing tumor development, evolution, metastasis, therapeutic outcome, and patient prognosis. Therefore, these drug resistance-related genes may be potential biomarkers in lung cancer in terms of expression and prognosis. However, in the field of lung cancer, these drug resistance-related genes have not been sufficiently studied. In our study, we conducted a complex bioinformatics analysis of drug resistance-related gene expression in lung cancer and made full use of the resources of public databases to provide an insightful and extensive assessment of their potential values in lung cancer in terms of expression and prognosis, thus contributing more information for clinicians to select and design individual treatment plans and predict patient prognosis with lung cancer.
Table 1
Gene | Gene type | NCBI gene ID | Location | Exon count |
---|---|---|---|---|
CCDC73 | Protein-coding | 493860 | 11p13 | 18 |
DLGAP1 | Protein-coding | 9229 | 18p11.31 | 21 |
DSEL | Protein-coding | 92126 | 18q22.1 | 2 |
ESR1 | Protein-coding | 2099 | 6q25.1–q25.2 | 23 |
SEC14L5 | Protein-coding | 9717 | 18p11.31 | 6,579 |
CCDC73, coiled-coil domain-containing protein 73; DLGAP1, DLG associated protein 1; DSEL, dermatan sulfate epimerase like; ESR1, estrogen receptor 1; SEC14L5, SEC14-like 5.
We present the following article in accordance with the REMARK reporting checklist (available at https://tcr.amegroups.com/article/view/10.21037/tcr-21-1841/rc).
Methods
ONCOMINE
ONCOMINE (https://www.oncomine.org) is a web-based bioinformatics service available for analysis of differential gene expression and prediction of co-expressed genes (14). The expression of these drug resistance-related genes in lung cancer was assessed by extracting data. In this study, 0.05 and 2 were the threshold for P value and fold change, separately. The differential expression of these drug resistance-related genes in lung cancer was analyzed by Student’s t-test.
GEPIA
GEPIA (http://gepia.cancer-pku.cn/index.html), containing 9,736 datasets from the GTEx and the TCGA projects, can effectively conduct online analysis and mining of cancer data for RNA sequence expression (15). In this study, “Single Gene Analysis” was utilized for analysis of differential expression, phase of pathology and relevant prognosis of these genes. Moreover, “Multiple Gene Comparison” was used for multiple gene comparison analysis of these genes. The threshold for P value was 0.05. These genes were analyzed by Student’s t-test.
UALCAN
UALCAN (http://ualcan.path.uab.edu/analysis.html) functions as a versatile and robust database available for identifying biomarkers, analyzing expression profiles, and survival analysis, among others (16). In our study, the “Expression Analysis” module, “LUAD” and “LUSC” datasets were utilized to analyze data of these drug resistance-related genes. Generated by the Student’s t-test, the P value has a threshold value of 0.05.
cBioPortal
cBioPortal (https://www.cbioportal.org), a user-friendly website based on numerous databases, enables visualization of cancer genomics data in multiple dimensions (17). Genetic variation, gene network, as well as co-expression of these drug resistance-related genes were analyzed by cBioPortal. Both the z scores of mRNA and protein expression were derived from the z score threshold ±2.0.
GeneMANIA
GeneMANIA (http://www.genemania.org), a comprehensive web resource, supplies information on genetic interactions and protein analysis, including Genetic Interactions, Pathways, and Shared protein domains (18). In this study, protein-protein interaction (PPI) network of these drug resistance-related genes was obtained by GeneMANIA.
STRING
STRING (https://string-db.org), covering up to 5.090 thousand total organisms and 24.6 million proteins, aims to search, evaluate, and combine all available sources of PPI data using computational predictions, knowledge transfer between organisms and so on (19). In this study, PPI network analysis of drug resistance-related genes was performed by STRING.
TIMER
TIMER (https://cistrome.shinyapps.io/timer/), an accessible and intuitive database, makes it possible to systematically assess the clinical effects of various immune cells on a variety of cancer types (20). The statistical methods it uses have been validated using pathological estimates. In this study, “Gene Module” was used to fully explore the relationship between the expression of these genes and various immune cells. And the “Clinical Module” was used to perform the survival analysis of various types of immune cells in lung cancer.
Statistical analysis
The specific statistical methods were described in detail in the database section above. In general, P≤0.05 was considered as a statistically significant difference.
Ethical statement
The study was conducted in accordance with the Declaration of Helsinki (as revised in 2013).
Results
Expression patterns of drug resistance-related genes in patients with lung cancer
There drug resistance-related genes (including CCDC73, DLGAP1, DSEL, ESR1 and SEC14L5) were first searched with ONCOMINE, a database includes 715 datasets and 86,733 samples. The database was used to analyze differences in transcriptional levels between lung cancer and normal lung tissues. In comparison with normal tissues, the transcriptional levels of DLGAP1 was lower, and those of SEC14L5 were remarkably higher in lung cancer tissues (Figure 1). Consistent with the above results, it has been indicated that the expression level of DLGAP1 (P=7.47e−08) in lung carcinoid tumor decreased with a fold change of −34.874 (Table 2) (21). The expression level of SEC14L5 (P=1.42e−04) in lung carcinoid tumor increased, and the fold change was 3.178 in Bhattacharjee’s datasets (Table 2) (21). In the Garber datasets, ESR1 transcriptional levels were significantly increased in lung adenocarcinoma (LUAD) when compared with normal lung tissues (Table 2) (22). However, Hou’s dataset suggested that ESR1 (P=1.78e−08) significantly decreased in large cell lung carcinoma (Table 2) (23). Different cancer types could account for these inconsistencies.
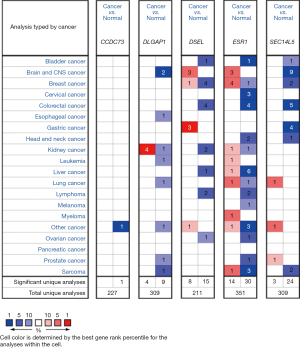
Table 2
Gene | Cancer type | Fold change | P value | t-test |
---|---|---|---|---|
DLGAP1 | Lung carcinoid tumor | −34.874 | 7.47e−08 | −6.81 |
ESR1 | Lung adenocarcinoma | 8.770 | 4.07e−04 | 5.958 |
ESR1 | Large cell lung carcinoma | −3.558 | 1.78e−08 | −8.015 |
SEC14L5 | Lung carcinoid tumor | 3.178 | 1.42e−04 | 4.37 |
DLGAP1, DLG associated protein 1; ESR1, estrogen receptor 1; SEC14L5, SEC14-like 5.
Besides, the expression levels of these drug resistance-related genes in LUAD and lung squamous cell carcinoma (LUSC) tissues as well as in normal tissues were also tested in UALCAN. The transcriptional levels of CCDC73 (P=3.693e−06), DLGAP1 (P=0.09876) and ESR1 (P=1.997e−07) in LUAD tissues were decreased (Figure 2). Meanwhile, the expression levels of all genes except CCDC73 were also decreased in LUSC (Figure 3). The relative expression levels of these drug resistance-related genes in lung cancer tissues and normal tissues were also performed for comparison (Figure 4). The results found that among all the drug resistance-related genes we evaluated, DSEL had the highest relative expression in normal tissues. Unfortunately, no significant difference in the expression of these genes in LUAD or LUSC was found.
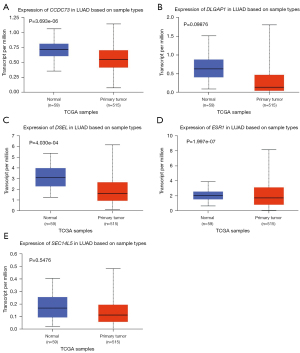
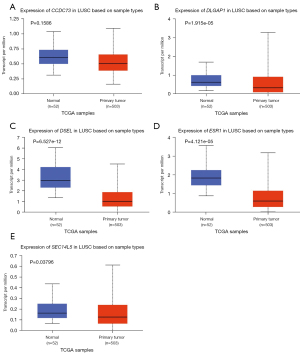
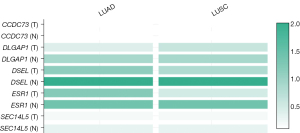
To search for genes closely associated with the development, evolution of lung cancer, we further evaluated the relationships between the expression of these drug resistance-related genes and the pathological stage of LUAD (Figure 5) and LUSC (Figure 6). The expression of SEC14L5 (P=0.00523) showed significant correlation with the pathological stage of LUAD (Figure 5). Unfortunately, none of the genes is significantly related to the pathological staging of LUSC (Figure 6). These data suggested that these drug resistance-related genes might significantly lead to tumor development and progression of LUAD.
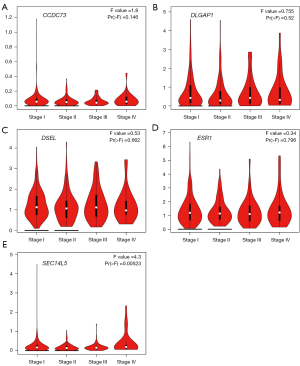
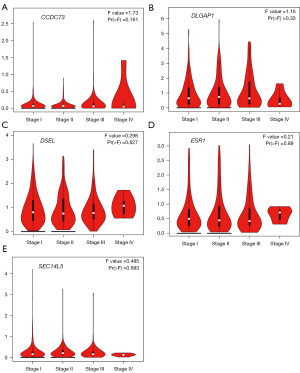
The prognostic values of drug resistance-related genes in patients with lung cancer
The relationships between drug resistance-related genes and clinical effects of lung cancer patients were evaluated using the GEPIA database to further illuminate the potential value of these drug resistance-related genes in lung cancer progression. LUAD patients with low DSEL (P=0.046) transcription levels and high SEC14L5 (P=0.046) transcription levels tended to have longer disease-free survival (DFS) (Figure 7). Unfortunately, there was no gene whose transcript levels were strongly correlated with DFS in LUSC (Figure 8). In addition, we also evaluated the role of drug resistance-related genes in the overall survival (OS) of lung cancer patients. However, none of the genes demonstrated a significant correlation with the OS of patients with LUAD (Figure 9). But the OS of LUSC patients with high CCDC73 transcriptional levels was remarkably prolonged (Figure 10).
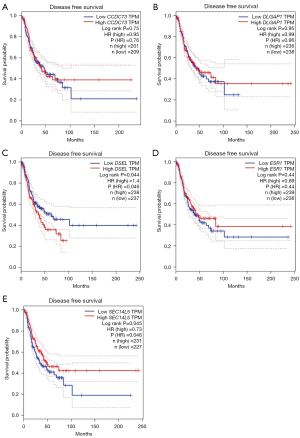
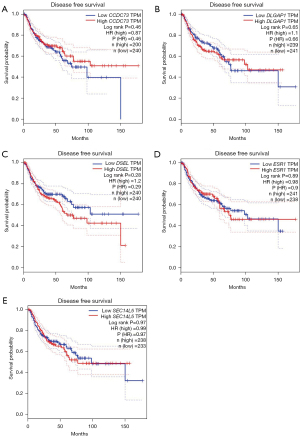
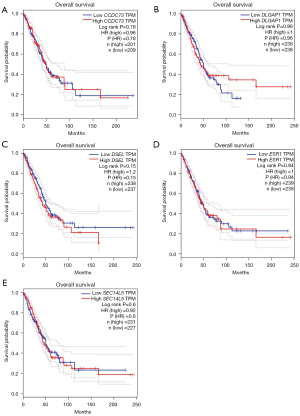
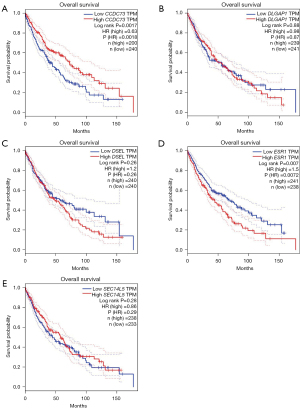
Genetic alteration, PPI network and interaction analyses of these drug resistance-related genes in patients with lung cancer
A detailed and extensive molecular characterization was obtained by analysis of drug resistance-related genes to characterize their genetics and PPI. Genetic alterations in drug resistance-related genes were derived by analysis using a temporary dataset from TCGA. As a result, CCDC73, DLGAP1, ESR1, DSEL and SEC14L5 were altered in 9%, 9%, 9%, 7%, and 2.3% of the tested LUAD samples, separately (Figure 11A). And, DLGAP1, CCDC73, DSEL, ESR1 and SEC14L5 were altered in 11%, 10%, 10%, 10%, and 2.7% of the tested LUSC samples, separately (Figure 11B). What followed was a further exploration of potential co-expression analysis of drug resistance-related genes. In LUAD, there was a relatively weak link between the expression of CCDC73, DLGAP1 and DSEL, and the similar link between the expression of DLGAP1 and SEC14L5 (Figure 11C). However, in LUSC, there was a low to moderate correlation between DLGAP1 and CCDC73, DSEL and DLGAP1, and a low to moderate correlation was identified between the expression of DSEL and ESR1 (Figure 11D). In addition, we also carried out PPI network analysis of drug resistance-related genes and strings to explore the potential interaction between them. Unfortunately, the PPI network in the result did not gain new nodes, and thus there were no edges (Figure 11E). The results of GeneMANIA showed that the function of these genes was mainly related to RNA polymerization, which may be related to the high metabolism of tumor cells (Figure 11F).
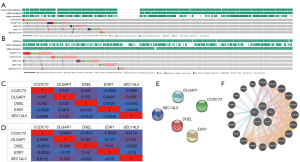
Immune cell infiltration of these drug resistance-related genes in patients with lung cancer
One important mechanism by which these genes affected the clinical outcome of lung cancer patients was the immune response. Therefore, TIMER database was used to conduct an extensive study on the relationship between these drug resistance-related genes and immune cell infiltration. In LUAD, both B cell infiltration (P=2.50e−3) and CD4+ T cell infiltration (P=7.46e−3) were positively associated with the expression of CCDC73 (Figure 12A). A statistical association of DLGAP1 with any immune cell infiltration was not found here (Figure 12B). As the expression of DSEL increased, the infiltration of CD8+ T cell (cor =0.092, P=4.34e−2) and macrophage (cor =0.108, P=1.74e−2) progressed (Figure 12C). All six immune cell types were positively associated with the expression of ESR1 (all P<0.05; Figure 12D). The expression of SEC14L5 was positively correlated with other five kinds of immune cells (all P<0.05) except CD8+ T cell (Figure 12E).
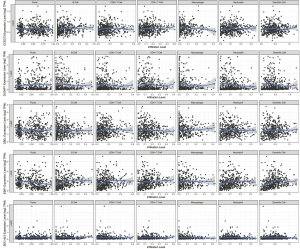
In LUSC, significant associations were not found between CCDC73 and six immune cell infiltrates (Figure 13A). Higher DLGAP1 expression in LUSC patients contributed to deeper B cell infiltration (cor =0.167, P=2.60e−4; Figure 13B). In addition to CD4+ T cells, DSEL expression and other five kinds of immune cells (all P<0.05; Figure 13C). LUSC patients with higher ESR1 gene expression tended to have deeper infiltration of all studied immune cells (Figure 13D). In LUSC, CD8+ T cells (cor =−0.114, P=1.29e−2) as well as neutrophils (cor =−0.111, P=1.51e−2; Figure 13E) were negatively correlated with SEC14L5 gene expression. The Cox proportional hazards model was utilized to further assess the degree of correlation between these drug resistance-related genes and immune cell infiltration. B cells (P<0.001) and CD4+ T cells (P=0.040) were remarkably correlated with the clinical effects of patients with LUAD (Table 3). Unfortunately, none of immune cells or these drug resistance-related genes was remarkably associated with the clinical effects of patients with LUSC lung cancer (Table 4).
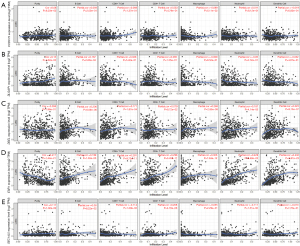
Table 3
Cells and genes | Coef. | HR | 95% CI_l | 95% CI_u | P value |
---|---|---|---|---|---|
B cell | −5.256 | 0.005 | 0.000 | 0.068 | 0.000*** |
CD8+ T cell | 0.202 | 1.224 | 0.195 | 7.664 | 0.829 |
CD4+ T cell | 2.827 | 16.901 | 1.133 | 252.102 | 0.040* |
Macrophage | 0.014 | 1.014 | 0.077 | 13.307 | 0.991 |
Neutrophil | −0.177 | 0.838 | 0.019 | 36.589 | 0.927 |
Dendritic | −0.339 | 0.713 | 0.187 | 2.711 | 0.619 |
CCDC73 | −0.194 | 0.823 | 0.404 | 1.677 | 0.592 |
DLGAP1 | 0.150 | 1.161 | 0.956 | 1.411 | 0.132 |
DSEL | 0.150 | 1.162 | 0.964 | 1.401 | 0.115 |
ESR1 | 0.045 | 1.046 | 0.914 | 1.196 | 0.516 |
SEC14L5 | 0.088 | 1.091 | 0.733 | 1.626 | 0.667 |
*, P<0.05; ***, P<0.001. LUAD, lung adenocarcinoma; coef., coefficient; HR, hazard ratio; 95% CI_I, lower bound of 95% confidence interval; 95% CI_u, upper bound of 95% confidence interval; CCDC73, coiled-coil domain-containing protein 73; DLGAP1, DLG associated protein 1; DSEL, dermatan sulfate epimerase like; ESR1, estrogen receptor 1; SEC14L5, SEC14-like 5.
Table 4
Cells and genes | Coef. | HR | 95% CI_I | 95% CI_u | P value |
---|---|---|---|---|---|
B cell | 0.933 | 2.541 | 0.226 | 28.576 | 0.450 |
CD8+ T cell | −1.213 | 0.297 | 0.051 | 1.735 | 0.178 |
CD4+ T cell | 0.371 | 1.449 | 0.118 | 17.762 | 0.772 |
Macrophage | 0.889 | 0.503 | 0.047 | 5.382 | 0.570 |
Neutrophil | 0.658 | 1.930 | 0.072 | 52.063 | 0.696 |
Dendritic | 0.607 | 1.835 | 0.448 | 7.523 | 0.399 |
CCDC73 | 0.016 | 0.984 | 0.538 | 1.802 | 0.959 |
DLGAP1 | 0.017 | 1.018 | 0.874 | 1.185 | 0.822 |
DSEL | 0.063 | 1.065 | 0.884 | 1.283 | 0.508 |
ESR1 | 0.058 | 1.060 | 0.860 | 1.306 | 0.585 |
SEC14L5 | 0.201 | 0.818 | 0.563 | 1.187 | 0.290 |
LUSC, lung squamous cell carcinoma; coef., coefficient; HR, hazard ratio; 95% CI_I, lower bound of 95% confidence interval; 95% CI_u, upper bound of 95% confidence interval; CCDC73, coiled-coil domain-containing protein 73; DLGAP1, DLG associated protein 1; DSEL, dermatan sulfate epimerase like; ESR1, estrogen receptor 1; SEC14L5, SEC14-like 5.
Discussion
Drug resistance-related genes such as DLGAP1, ESR1, SEC14L5, DSEL, CCDC73 can make vital contributions to tumor development, tumor progression, and tumor metastasis. It has been reported that there is a correlation between these drug resistance-related genes and tumor microenvironment and tumor therapy, indicating that these drug resistance-related genes may regulate tumor progression and immunotherapy effect. Therefore, these drug resistance-related genes may be potential biomarkers in lung cancer in terms of expression and prognosis. However, the potential of these drug resistance-related genes as therapeutic targets and prognostic biomarkers in lung cancer have not been well described.
We first discussed the expression of these drug resistance-related genes in lung cancer and their relationships with pathological staging. As a result, the expression of DLGAP1 was significantly down-regulated, but the expression of SEC14L5 was significantly up-regulated in lung cancer tissues. The expression of DLGAP1 was down-regulated in lung carcinoid tumor. The expression of ESR1 in LUAD tissue was up-regulated, but the expression of ESR1 in large cell lung carcinoma was down-regulated. Lung cancer patients with high CCDC73 expression tended to have longer OS. In LUAD patients, SEC14L5 expression was positively correlated with the pathological stage of the tumor, which was consistent with the result that SEC14L5 expression was increased in lung cancer. These data indicated that these drug resistance-related genes may contribute to the development and progression of lung cancer.
In view of the significant differences in the expression of multiple drug resistance-related genes in lung cancer, we explored detailed and extensive molecular characteristics. Among all the mutant genes associated with the differential expression of drug resistance in LUAD and LUSC, CCDC73 was the most susceptible gene to be mutated. In contrast, SEC14L5 was the least mutated gene among all mutated genes associated with differential expression of drug resistance in LUAD and LUSC. Mutations in these genes might be associated with tumor heterogeneity and tumor drug resistance. For example, ESR1 mutations in breast cancer were an essential mechanism for anti-endocrine therapy for breast cancer (24,25). The development and progression of lung cancer were complex and multifaceted, and the exact mechanisms were not fully understood, but it was certain that genetic alterations were of vital importance (25). In this study, no strong correlation was found between these drug resistance-related genes, which might suggest that these drug resistance-related genes lead to the development and progression of lung cancer by different mechanisms and pathways. These drug resistance-related genes can affect the prognosis of a wide range of tumors. It was demonstrated that CCDC73 was strongly associated with the prognosis and progression of bladder cancer (26), hepatocellular carcinoma (27) and endometrial cancer (28). DLGAP1 is involved in the metastasis and recurrence of malignant melanoma by pathways like cell migration, extracellular matrix and cytoskeletal network, leading to a significant reduction in OS (28). Inhibition of DLGAP1 expression reduces the proliferation and aggression of cancer cells and improves chemotherapy sensitivity (29). DSEL can also function as a validated biomarker to predict OS in bladder cancer (30). SEC14L5 was thought to be closely associated with rectum adenocarcinoma (31), lung cancer (8) and others. This study revealed a statistically significant relationship between the expression of SEC14L5 and the progression of LUAD.
A growing number of studies pointed to the fact that inflammatory responses and immune reactions make vital contributions in the development and progression of cancer, and could even have a significant impact on immunotherapy effect and clinical outcome (32,33). As the main effector cells of antitumor immunity, CD8+ T cells could selectively target mutant or tumor cells and generate lethal effects through various pathways. CD4+ T cells not only helped CD8+ T and macrophages activation, but also produced cytokines and chemokines that indirectly participated in antitumor immune effects (34). This study suggested that drug resistance-related genes could be used as an important indicator of immune status, resulting from our discovery that the expression of drug resistance-related genes was significantly correlated with the infiltration of six types of immune cells (dendritic cells, macrophages, neutrophils, B cells, CD4+ T cells, and CD8+ T cells). Notably, the increased immune cell infiltration but not superior prognosis of LUAD compared to LUSC may be related to the different origin of the database and some potential possible mechanisms to be discovered by further studies. Similarly, in LUAD patients, high DSEL was shown to be associated with increased immune cell infiltration in the GEPIA database, but worse outcomes in the TIMER database. It is thought that differences in study data and other factors affecting outcome may play a role. Therefore, the differential expression of these drug resistance-related genes not only correlated with lung cancer in terms of progression and prognosis, but also closely linked with the immune microenvironment, which might serve as an important biomarker to assist in tumor treatment.
There are also some limitations in this study. The immune status was only partially characterized by the analysis of the transcriptional levels, but cannot be interpreted in comprehensive details. Furthermore, it would be more persuasive if our findings were further verified in independent in vivo or in vitro biological experiments.
Conclusions
To sum up, the resources of public databases were fully utilized to analyze the correlation between drug resistance-related genes in lung cancer selected by RNA sequencing and the development and prognosis of lung cancer. Based on these databases, we found increased DLGAP1 expression and decreased SEC14L5 expression in lung cancer, high CCDC73 associated with better outcome of LUSC, SEC14L5 expression associated with LUAD pathological staging, molecular characterization of these genes, and their correlations with immune cell infiltration. Our results could be helpful in select new immunotherapy targets and prognostic biomarkers for lung cancer, thus providing clinicians with new perspectives for designing individualized therapeutic plans and predicting survival of lung cancer patients.
Acknowledgments
Funding: This study was supported by ‘Dream Tutor’ Outstanding Young Talents Program (fkyq1901), National Key R&D Program of China (2018YFC0910500), and the Neil Shen’s SJTU Medical Research Fund.
Footnote
Reporting Checklist: The authors have completed the REMARK reporting checklist. Available at https://tcr.amegroups.com/article/view/10.21037/tcr-21-1841/rc
Peer Review File: Available at https://tcr.amegroups.com/article/view/10.21037/tcr-21-1841/prf
Conflicts of Interest: All authors have completed the ICMJE uniform disclosure form (available at https://tcr.amegroups.com/article/view/10.21037/tcr-21-1841/coif). The authors have no conflicts of interest to declare.
Ethical Statement: The authors are accountable for all aspects of the work in ensuring that questions related to the accuracy or integrity of any part of the work are appropriately investigated and resolved. The study was conducted in accordance with the Declaration of Helsinki (as revised in 2013).
Open Access Statement: This is an Open Access article distributed in accordance with the Creative Commons Attribution-NonCommercial-NoDerivs 4.0 International License (CC BY-NC-ND 4.0), which permits the non-commercial replication and distribution of the article with the strict proviso that no changes or edits are made and the original work is properly cited (including links to both the formal publication through the relevant DOI and the license). See: https://creativecommons.org/licenses/by-nc-nd/4.0/.
References
- Goss PE, Strasser-Weippl K, Lee-Bychkovsky BL, et al. Challenges to effective cancer control in China, India, and Russia. Lancet Oncol 2014;15:489-538. [Crossref] [PubMed]
- Almendro V, Marusyk A, Polyak K. Cellular heterogeneity and molecular evolution in cancer. Annu Rev Pathol 2013;8:277-302. [Crossref] [PubMed]
- Duma N, Santana-Davila R, Molina JR. Non-Small Cell Lung Cancer: Epidemiology, Screening, Diagnosis, and Treatment. Mayo Clin Proc 2019;94:1623-40. [Crossref] [PubMed]
- Herbst RS, Morgensztern D, Boshoff C. The biology and management of non-small cell lung cancer. Nature 2018;553:446-54. [Crossref] [PubMed]
- Jiang M, Wu C, Zhang L, et al. FOXP3-based immune risk model for recurrence prediction in small-cell lung cancer at stages I-III. J Immunother Cancer 2021;9:e002339. [Crossref] [PubMed]
- He Y, Yu H, Rozeboom L, et al. LAG-3 Protein Expression in Non-Small Cell Lung Cancer and Its Relationship with PD-1/PD-L1 and Tumor-Infiltrating Lymphocytes. J Thorac Oncol 2017;12:814-23. [Crossref] [PubMed]
- Kuang P, Chen P, Wang L, et al. RNA sequencing analysis of small cell lung cancer reveals candidate chemotherapy insensitivity long noncoding RNAs and microRNAs. Ann Transl Med 2020;8:121. [Crossref] [PubMed]
- Zhang S, Thakur A, Liang Y, et al. Polymorphisms in C-reactive protein and Glypican-5 are associated with lung cancer risk and Gartrokine-1 influences Cisplatin-based chemotherapy response in a Chinese Han population. Dis Markers 2015;2015:824304. [Crossref] [PubMed]
- He Y, Song L, Wang H, et al. Mutational Profile Evaluates Response and Survival to First-Line Chemotherapy in Lung Cancer. Adv Sci (Weinh) 2020;8:2003263. [Crossref] [PubMed]
- Yang C, Ren J, Li B, et al. Identification of clinical tumor stages related mRNAs and miRNAs in cervical squamous cell carcinoma. Pathol Res Pract 2018;214:1638-47. [Crossref] [PubMed]
- Teng C, Huang G, Luo Y, et al. Differential long noncoding RNAs expression in cancer-associated fibroblasts of non-small-cell lung cancer. Pharmacogenomics 2019;20:143-53. [Crossref] [PubMed]
- Namani A, Cui QQ, Wu Y, et al. NRF2-regulated metabolic gene signature as a prognostic biomarker in non-small cell lung cancer. Oncotarget 2017;8:69847-62. [Crossref] [PubMed]
- Li J, Ji Z, Luo X, et al. Urinary bisphenol A and its interaction with ESR1 genetic polymorphism associated with non-small cell lung cancer: findings from a case-control study in Chinese population. Chemosphere 2020;254:126835. [Crossref] [PubMed]
- Rhodes DR, Yu J, Shanker K, et al. ONCOMINE: a cancer microarray database and integrated data-mining platform. Neoplasia 2004;6:1-6. [Crossref] [PubMed]
- Tang Z, Li C, Kang B, et al. GEPIA: a web server for cancer and normal gene expression profiling and interactive analyses. Nucleic Acids Res 2017;45:W98-W102. [Crossref] [PubMed]
- Chandrashekar DS, Bashel B, Balasubramanya SAH, et al. UALCAN: A Portal for Facilitating Tumor Subgroup Gene Expression and Survival Analyses. Neoplasia 2017;19:649-58. [Crossref] [PubMed]
- Gao J, Aksoy BA, Dogrusoz U, et al. Integrative analysis of complex cancer genomics and clinical profiles using the cBioPortal. Sci Signal 2013;6:pl1. [Crossref] [PubMed]
- Warde-Farley D, Donaldson SL, Comes O, et al. The GeneMANIA prediction server: biological network integration for gene prioritization and predicting gene function. Nucleic Acids Res 2010;38:W214-20. [Crossref] [PubMed]
- Szklarczyk D, Gable AL, Lyon D, et al. STRING v11: protein-protein association networks with increased coverage, supporting functional discovery in genome-wide experimental datasets. Nucleic Acids Res 2019;47:D607-13. [Crossref] [PubMed]
- Li T, Fan J, Wang B, et al. TIMER: A Web Server for Comprehensive Analysis of Tumor-Infiltrating Immune Cells. Cancer Res 2017;77:e108-10. [Crossref] [PubMed]
- Bhattacharjee A, Richards WG, Staunton J, et al. Classification of human lung carcinomas by mRNA expression profiling reveals distinct adenocarcinoma subclasses. Proc Natl Acad Sci U S A 2001;98:13790-5. [Crossref] [PubMed]
- Garber ME, Troyanskaya OG, Schluens K, et al. Diversity of gene expression in adenocarcinoma of the lung. Proc Natl Acad Sci U S A 2001;98:13784-9. [Crossref] [PubMed]
- Hou J, Aerts J, den Hamer B, et al. Gene expression-based classification of non-small cell lung carcinomas and survival prediction. PLoS One 2010;5:e10312. [Crossref] [PubMed]
- Jeselsohn R, Buchwalter G, De Angelis C, et al. ESR1 mutations—a mechanism for acquired endocrine resistance in breast cancer. Nat Rev Clin Oncol 2015;12:573-83. [Crossref] [PubMed]
- Yap NY, Rajandram R, Ng KL, et al. Genetic and Chromosomal Aberrations and Their Clinical Significance in Renal Neoplasms. Biomed Res Int 2015;2015:476508. [Crossref] [PubMed]
- Liu L, Zhong X, Cui H, et al. Identification of an Individualized Prognostic Signature Based on the RWSR Model in Early-Stage Bladder Carcinoma. Biomed Res Int 2020;2020:9186546. [Crossref] [PubMed]
- Gao HJ, Chen YJ, Zuo D, et al. Quantitative proteomic analysis for high-throughput screening of differential glycoproteins in hepatocellular carcinoma serum. Cancer Biol Med 2015;12:246-54. [PubMed]
- O'Mara TA, Glubb DM, Amant F, et al. Identification of nine new susceptibility loci for endometrial cancer. Nat Commun 2018;9:3166. [Crossref] [PubMed]
- Rendleman J, Shang S, Dominianni C, et al. Melanoma risk loci as determinants of melanoma recurrence and survival. J Transl Med 2013;11:279. [Crossref] [PubMed]
- Lin J, Yang J, Xu X, et al. A robust 11-genes prognostic model can predict overall survival in bladder cancer patients based on five cohorts. Cancer Cell Int 2020;20:402. [Crossref] [PubMed]
- Huang W, Li G, Wang Z, et al. A Ten-N6-Methyladenosine (m6A)-Modified Gene Signature Based on a Risk Score System Predicts Patient Prognosis in Rectum Adenocarcinoma. Front Oncol 2021;10:567931. [Crossref] [PubMed]
- Bindea G, Mlecnik B, Tosolini M, et al. Spatiotemporal dynamics of intratumoral immune cells reveal the immune landscape in human cancer. Immunity 2013;39:782-95. [Crossref] [PubMed]
- Liu X, Wu S, Yang Y, et al. The prognostic landscape of tumor-infiltrating immune cell and immunomodulators in lung cancer. Biomed Pharmacother 2017;95:55-61. [Crossref] [PubMed]
- Lin P, Guo YN, Shi L, et al. Development of a prognostic index based on an immunogenomic landscape analysis of papillary thyroid cancer. Aging (Albany NY) 2019;11:480-500. [Crossref] [PubMed]