Molecular subtypes of m6A RNA methylation modification patterns and their clinical significance in clear cell renal cell carcinoma
Introduction
Renal cell carcinoma (RCC), also known as renal adenocarcinoma, or simply renal cancer, is a malignant tumor originating from the uriniferous tubule epithelium in the renal parenchyma. Over the last 2 decades, the incidence of renal cancer has risen by approximately 2% (1). At present, RCC cases account for 80% to 90% of all renal malignancies (2). The incidence is 1.5-fold higher in men than women, with peak incidence at 60 to 70 years of age (3). In 2016, the World Health Organization classified RCC according to origin and genetic alterations as clear cell renal cell carcinoma (ccRCC) (60–85%), chromophobe renal carcinoma (4–10%), papillary RCC (7–14%), collecting duct carcinoma (1–2%), and other rare types (4). The most prevalent RCC type is ccRCC, which is usually well-defined, without an envelope, yellow in color, and often associated with hemorrhage and necrosis. The most frequent genetic alterations include the deletion of chromosome 3p and mutations in the von Hippel-Lindau (VHL) gene on chromosome 3p25. Other tumor suppressor genes include breast cancer 1-associated protein 1 (BAP1), SET domain containing 2 (SETD2), and polybromo 1 (PBRM1) (5).
RNA modifications constitute a type of post-transcriptional regulation mechanism. To date, over 150 RNA modifications have been identified. They occur across different RNA types, such as ribosomal RNAs (rRNAs), transfer RNAs (tRNAs), messenger RNAs (mRNAs), long non-coding RNAs (lncRNAs), and small non-coding RNAs (sncRNAs). The most common methylation modification in RNA is N6-methyladenine (m6A), which occurs most commonly in the mRNA of higher organisms. The modification level of m6A in the transcripts is regulated dynamically by several enzymes, including the methyltransferases (encoders), binding proteins (readers), and demethylases (decoders). These m6A RNA methylation modifications play a major role in cancer progression processes, including migration, invasion, and proliferation. Thus, m6A modifications and their regulators are becoming putative targets for cancer diagnosis and treatment as their potential underlying mechanisms are gradually being unraveled (6).
Although previous studies have found that m6A RNA methylation regulators play an important role in the progression of ccRCC, which may be conducive to prognostic stratification (7,8). We applied different methods to construct new ccRCC subtypes and new prognostic molecular signature for ccRCC.In the present study, we used high-throughput sequencing data from The Cancer Genome Atlas (TCGA, https://www.cancer.gov) and identified the molecular subtypes of ccRCC according to expressions of m6A-related genes, including methyltransferase like 14 (METTL14), methyltransferase like 3 (METTL3), Wilms’ tumor 1-associated protein (WTAP), KIAA1429, zinc finger CCCH domain-containing protein 13 (ZC3H13), RNA binding motif protein 15 (RBM15), HNRNPC, YTHDC1, YTHDC2, YTHDF1, YTHDF2, AlkB Homolog 5 (ALKBH5), and the fat mass and obesity-associated gene (FTO). Next, comparative survival analysis was performed for the obtained subtypes, and m6A-related gene expressions were used to construct the m6A-related prognostic molecular signature for ccRCC. The findings are expected to provide new research ideas for studies on the etiology and pathogenesis of ccRCC. We present the following article in accordance with the TRIPOD reporting checklist (available at https://tcr.amegroups.com/article/view/10.21037/tcr-22-117/rc).
Methods
Data source
RNA-sequencing data for a total of 611 renal tissue samples (72 normal and 539 ccRCC tumor samples) and the corresponding clinical data for 530 patients were obtained from TCGA, including records of gender, race, age, pathological stage, smoking status, radiotherapy status, chemotherapy status, and survival. The study was conducted in accordance with the Declaration of Helsinki (as revised in 2013).
Research methodology
Differential analysis of expressions of m6A-related genes in ccRCC tumor and normal renal tissues
The expressions of m6A-related genes were extracted using the edgeR package (https://www.bioconductor.org/packages/release/bioc/html/edgeR) in R (version: 4.0.3) (https://bioconductor.org/install/index.html#install-R). The differences between tumor and normal renal tissues were analyzed. Heat maps and violin plots were constructed, and correlation analysis was performed among the m6A-related genes. The criteria for determining the differential gene expressions in this study were as follows: an absolute value of fold change ≥2 and a corrected false discovery rate (FDR) <0.05.
Construction of ccRCC molecular subtypes according to m6A-related gene expressions
Unsupervised clustering analysis of the m6A-related gene expressions in ccRCC was performed using the ConsensusClusterPlus package (https://www.bioconductor.org/packages/release/bioc/html/ConsensusClusterPlus). The ccRCC molecular subtypes identified according to m6A-related gene patterns were used for comparative prognoses of patients with different subtypes.
Construction of a ccRCC prognostic molecular signature according to m6A-related gene expression patterns
Least absolute shrinkage and selector operation (LASSO)-Cox regression analyses were used to identify the genes significantly associated with prognosis in patients with ccRCC. A molecular signature was constructed using Cox regression coefficients. The calculation formula was as follows:
where EXP represented the expression level of genes and β the regression correlation coefficient. After substituting the relevant data, the molecular signature scores were calculated. According to the median of the molecular signature scores, patients with ccRCC were classified into high- and low-expression groups, and the prognoses for the 2 groups were compared.
Statistical analysis
Continuous variables use t-test or one-way ANOVA, and categorical variables use chi-square test or Fisher-exact. Univariate and multivariate Cox regression analyses were performed to demonstrate whether genetic traits were independent of other clinical features. All statistics are made in R software 4.1.1. The edgeR package is used for difference analysis, and the survival package and glmnet package are used for Cox regression analysis and Lasso regression analysis.
Results
Basic characteristics of patients
The data of a total of 530 patients, including 186 (35.09%) females and 344 (64.91%) males, were analyzed. A total of 177 (33.4%) of them did not survive, while 353 (66.6%) of them did (Table 1).
Table 1
Clinical variables | Case (n) | (%) |
---|---|---|
Survival status | ||
Surviving | 353 | 66.60 |
Dead | 177 | 33.40 |
Age (years) | ||
<50 | 107 | 20.19 |
50–59 | 138 | 26.04 |
60–69 | 142 | 26.79 |
70–79 | 110 | 20.75 |
≥80 | 24 | 4.53 |
Gender | ||
Female | 186 | 35.09 |
Male | 344 | 64.91 |
History of radiotherapy | ||
Yes | 6 | 1.13 |
No | 524 | 98.87 |
History of medication | ||
Yes | 75 | 14.15 |
No | 455 | 85.85 |
Pathological grade of the tumor | ||
G1 | 14 | 2.64 |
G2 | 227 | 42.83 |
G3 | 208 | 39.25 |
G4 | 75 | 14.15 |
GX | 6 | 1.13 |
Tumor stage | ||
Stage I | 267 | 50.38 |
Stage II | 57 | 10.75 |
Stage III | 124 | 23.40 |
Stage IV | 82 | 15.47 |
Tumor T-stage | ||
T1 | 271 | 51.13 |
T2 | 69 | 13.02 |
T3 | 179 | 33.77 |
T4 | 11 | 2.08 |
Tumor N-stage | ||
N0 | 239 | 45.09 |
N1 | 16 | 3.02 |
NX | 275 | 51.89 |
Tumor M-stage | ||
M0 | 420 | 79.25 |
M1 | 78 | 14.72 |
MX | 32 | 6.04 |
ccRCC, clear cell renal cell carcinoma.
The m6A-related genes differentially expressed between ccRCC tumor tissues and normal kidney tissues
The RNA sequencing data for 611 renal tissue samples, including 72 normal samples and 539 ccRCC tumor samples, were downloaded from TCGA. If patients had multiple tumor sample data, the average values was taken. The differences between ccRCC tumor tissue and normal kidney tissue expressions of 13 m6A-related genes (METTL14, METTL3, WTAP, HNRNPC, FTO, KIAA1429, RBM15 ZC3H13, YTHDF1, YTHDF2, YTHDC1, YTHDC2, and ALKBH5) were analyzed, and heat maps and violin plots were generated (Figure 1). Next, a correlation analysis between the expressions of m6A-related genes was performed (Figure 2). Eight of the 13 m6A-related genes (YTHDC1, YTHDF2, HNRNPC, FTO, METTL14, YTHDC2, ZC3H13, and YTHDF1) were significantly differentially expressed between normal and tumor renal tissues.
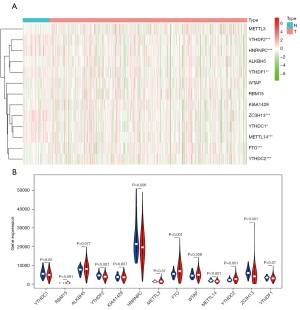
Molecular subtypes of ccRCC identified according to m6A-related gene expressions
Unsupervised clustering of ccRCC samples based on the expressions of m6A-related genes was performed using the ConsensusClusterPlus package (Figure 3A-3J). Based on the consistency matrix and results of the delta region, clustering was found to be stable, and the overfitting effect was reduced when k=2 or 3. The expectation maximization-principal component analysis (EM-PCA) algorithm was used to perform dimensionality reduction analysis of the principal components to concomitantly reduce gene expressions to PCA1 or PCA2, thereby representing the full gene expression information. Overlap was smaller when k=2 (Figure 3K) than when k=3 (Figure 3L). Moreover, when k=2, tumor samples could be better differentiated. The prognoses of patients with different ccRCC types at k=2 were also analyzed and compared, and significant differences were observed between the groups (P=0.016) (Figure 4A). Further exploring the tumor microenvironment and immune infiltrating cells of the two subtypes, The stromal score of subtype 2 was higher than that of subtype 1 (P<0.01) (Figure 4B,4C). There were significant differences in Memory B cells, T regulatory cells (Tregs), NK cells resetting and macrophages M1 between the two subtypes (Figure 4D).
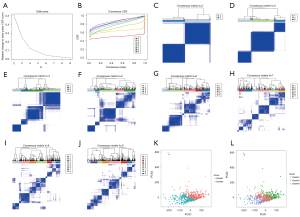
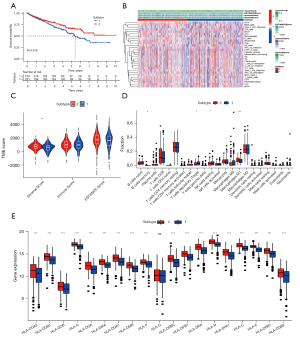
The expression levels of HLA genes of subtype 2 between two subtypes almost all higher than subtype 1 (Figure 4E).
Screening of prognostic markers and construction of a molecular signature for ccRCC
Univariate Cox regression analysis for 13 m6A-related gene expressions in combination with survival data revealed that 7 genes (ZC3H13, FTO, METTL3, METTL14, KIAA1429, YTHDC1, and YTHDF2) were significantly correlated with prognosis in patients with ccRCC (P<0.05).
The transcriptomic data of 530 ccRCC cases were randomly divided into 2 cohorts in a ratio of 30:70, with the latter cohort used as the training dataset for constructing the prognostic molecular signature and the former used as the testing dataset for validating the prognostic value of the constructed molecular signature.
The 7 genes related to prognosis in patients with ccRCC that were screened in the univariate Cox regression analysis were further analyzed by LASSO regression and multivariate Cox regression. Finally, 5 genes (KIAA1429, METTL14, METTL3, YTHDF2, and ZC3H13) (Figure 5, Table 2) were further identified and used to construct a molecular signature as in Eq. [1].
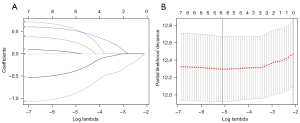
Table 2
m6A-related genes | Univariate analysis | Multivariate analysis | |||||
---|---|---|---|---|---|---|---|
Hazard ratio | Z value | P value | Hazard ratio | Z value | P value | ||
KIAA1429 | 0.789318 | –2.41701 | 0.015649 | 1.550374 | 1.728097 | 0.083971 | |
METTL14 | 0.696207 | –4.26616 | 0.0000199 | 0.34411 | –3.80809 | 0.00014 | |
METTL3 | 1.248753 | 2.087501 | 0.036843 | 1.828659 | 3.983522 | 0.0000679 | |
YTHDF2 | 0.77696 | –2.20015 | 0.027796 | 2.187476 | 2.334648 | 0.019562 | |
ZC3H13 | 0.776113 | –3.27685 | 0.00105 | 0.585816 | –2.18477 | 0.028905 |
where EXP represented the expression levels of the genes and β the regression correlation coefficient. After substituting the relevant data, the molecular signature scores were calculated.
The expression of the molecular signature was as calculated as follows:
After substituting the relevant data, the molecular signature scores were calculated. According to the median molecular signature score, patients were categorized into 2 groups, namely high- and low-score, and survival curves were plotted for the training and testing datasets after combining the survival time and survival status of the patients from TCGA. Next, we determined whether the differences in survival curves between the high- and low-score groups were significant. The molecular signature scores for the entire dataset and the training and testing datasets were correlated significantly with the survival of ccRCC patients. The higher the molecular signature score, the worse the prognosis of the patient (Figure 3). Subsequently, the receiver operator characteristic (ROC) curves were plotted using 1-, 3-, and 5-year survival rates (Figure 6).
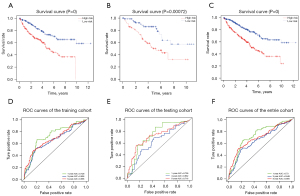
Discussion
Found in both mRNAs and lncRNAs, m6A RNA modifications are reversible epigenetic modifications and are closely associated with the stimulation and attenuation of cancer-related signaling cascades. They affect processes of transcription, localization, metabolism, RNA maturation, and translation and are involved in multiple biological processes in mammals, including the development of the nervous system, regulation of the circadian rhythms, heat shock response, DNA damage response, and tumor progression (9,10). Meanwhile, m6A serves as an important regulatory mechanism for gene expression in several physiological and pathological processes, including cancer progression. Genes related to m6A include METTL14, METTL3, WTAP, HNRNPC, KIAA1429, ALKBH5, RBM15, ZC3H13, FTO, YTHDF1, YTHDF2, YTHDC1, and YTHDC2. Among them, METTL14, METTL3, WTAP, RBM15, KIAA1429, and ZC3H13 are methyltransferase-related genes, while FTO and ALKBH5 are demethylase genes, whose primary role is to remove the m6A methyl groups from RNA. The main role of methylation recognition enzymes is to encode genes by binding to the m6A modification sites in the RNAs. The first identified methylation recognition enzymes were the proteins containing the YTH domains, including the YTH domain-containing protein (YTHDC) and YTH domain family protein (YTHDF) subtypes, such as YTHDC1, YTHDC2, YTHDF1, YTHDF2, and HNRNPC (10,11).
Cancer cell fates are altered by m6A modifications, which influences several different facets of cancer progression processes. In addition, m6A acts as a promoter or inhibitor at different stages of cancer (12). One previous study showed that m6A-related RNA in the peripheral blood might serve as an effective biomarker for the diagnosis of breast cancer and benign breast diseases (13). Another study reported the impact of m6A modifications on cancer treatment resistance and their implications in chemotherapy, radiotherapy, and immunotherapy (14). The m6A-related METTL3 gene is an oncogenic promoter in thymic tumors and may be a promising therapeutic target for the treatment of patients with thymic tumors (15).
The most common pathological type of RCC is ccRCC, which has characteristic common genetic alterations, including the deletion of chromosome 3p and mutations in the VHL gene on chromosome 3p25. Other tumor suppressor genes include SETD2, BAP1, and PBRM1 (5). A recent study showed that FTO could selectively inhibit the growth and survival of VHL-deficient cells (16). A study have shown that knockdown of METTL3 gene will increase the phosphorylation level of phosphatidylinositol-3-kinase/protein kinase B/mammalian rapamycin target protein pathway and affect the progress of RCC (17). The down-regulation of METTL3 will promote cell proliferation, migration, invasion and epithelial mesenchymal transformation, induce Go/G phase arrest and promote the increase of p21 expression, so as to promote the growth of RCC. In the present study, patients’ clinical data, along with the transcriptomics of 530 cases of ccRCC, were extracted from TCGA. Then, expressions of m6A-related genes were recorded, and the differences in m6A-related gene expressions between normal and tumor renal tissues were analyzed. Expressions of 8 genes among the 13 m6A -related genes identified in this study (YTHDC1, YTHDF2, HNRNPC, FTO, METTL14, YTHDC2, ZC3H13, and YTHDF1) differed significantly between the tumor and normal renal tissues. The molecular subtypes of ccRCC were identified according to the expressions of m6A-related genes, and the survival rates associated with different molecular subtypes were compared. Different molecular subtypes of ccRCC based on m6A RNA methylation were found to have different prognoses, which suggested that m6A RNA methylation modifications could have important implications in ccRCC and serve as a potential therapeutic target. We further explored the difference of tumor microenvironment between the two subtypes. There were significant differences in Memory B cells, T regulatory cells (Tregs), NK cells resetting and macrophages M1 between the two subtypes. The expression levels of HLA genes of subtype 2 between two subtypes almost all higher than subtype 1. We further combined the expression of 13 m6A related genes with survival data for univariate Cox analysis. It was found that univariate analysis of 7 genes (METTL14, ZC3H13, FTO, YTHDC1, KIAA1429, YTHDF2, METTL3) was related to the prognosis of renal clear cell carcinoma. The seven genes screened by univariate regression analysis and the genes related to the prognosis of ccRCC were further analyzed by lasso regression analysis and multivariate Cox analysis. Finally, the prognostic molecular tag value composed of five genes was constructed. The newly constructed molecular tag can well predict the prognosis of patients with renal clear cell carcinoma. All these suggested that m6A RNA methylation modifications could have important implications in ccRCC and serve as a potential therapeutic target.
This study had some limitations. First, there is no research was carried out on the mechanism of the screened RNA in this study. How these RNAs affect the growth and apoptosis of renal clear cell carcinoma and then affect the prognosis of renal clear cell carcinoma remains to be studied. Second, whether the constructed molecular tags can predict the prognosis of renal clear cell carcinoma outside TCGA database remains to be verified. These problems need further study.
Acknowledgments
Funding: This study received fundings from
Footnote
Reporting Checklist: The authors have completed the TRIPOD reporting checklist. Available at https://tcr.amegroups.com/article/view/10.21037/tcr-22-117/rc
Conflicts of Interest: All authors have completed the ICMJE uniform disclosure form (available at https://tcr.amegroups.com/article/view/10.21037/tcr-22-117/coif). The authors have no conflicts of interest to declare.
Ethical Statement: The authors are accountable for all aspects of the work in ensuring that questions related to the accuracy or integrity of any part of the work are appropriately investigated and resolved. The study was conducted in accordance with the Declaration of Helsinki (as revised in 2013).
Open Access Statement: This is an Open Access article distributed in accordance with the Creative Commons Attribution-NonCommercial-NoDerivs 4.0 International License (CC BY-NC-ND 4.0), which permits the non-commercial replication and distribution of the article with the strict proviso that no changes or edits are made and the original work is properly cited (including links to both the formal publication through the relevant DOI and the license). See: https://creativecommons.org/licenses/by-nc-nd/4.0/.
References
- Ljungberg B, Albiges L, Abu-Ghanem Y, et al. European Association of Urology Guidelines on Renal Cell Carcinoma: The 2019 Update. Eur Urol 2019;75:799-810. [Crossref] [PubMed]
- Zheng J, Wang L, Peng Z, et al. Low level of PDZ domain containing 1 (PDZK1) predicts poor clinical outcome in patients with clear cell renal cell carcinoma. EBioMedicine 2017;15:62-72. [Crossref] [PubMed]
- Hidayat K, Du X, Zou SY, et al. Blood pressure and kidney cancer risk: meta-analysis of prospective studies. J Hypertens 2017;35:1333-44. [Crossref] [PubMed]
- Moch H, Cubilla AL, Humphrey PA, et al. The 2016 WHO Classification of Tumours of the Urinary System and Male Genital Organs-Part A: Renal, Penile, and Testicular Tumours. Eur Urol 2016;70:93-105. [Crossref] [PubMed]
- Brugarolas J. Molecular genetics of clear-cell renal cell carcinoma. J Clin Oncol 2014;32:1968-76. [Crossref] [PubMed]
- Sun T, Wu R, Ming L. The role of m6A RNA methylation in cancer. Biomed Pharmacother 2019;112:108613. [Crossref] [PubMed]
- Zhang QJ, Luan JC, Song LB, et al. m6A RNA methylation regulators correlate with malignant progression and have potential predictive values in clear cell renal cell carcinoma. Exp Cell Res 2020;392:112015. [Crossref] [PubMed]
- Gu Y, Niu S, Wang Y, et al. DMDRMR-Mediated Regulation of m6A-Modified CDK4 by m6A Reader IGF2BP3 Drives ccRCC Progression. Cancer Res 2021;81:923-34. [Crossref] [PubMed]
- Chen XY, Zhang J, Zhu JS. The role of m6A RNA methylation in human cancer. Mol Cancer 2019;18:103. [Crossref] [PubMed]
- Robinson M, Shah P, Cui YH, et al. The Role of Dynamic m6 A RNA Methylation in Photobiology. Photochem Photobiol 2019;95:95-104. [Crossref] [PubMed]
- Zhou Z, Lv J, Yu H, et al. Mechanism of RNA modification N6-methyladenosine in human cancer. Mol Cancer 2020;19:104. [Crossref] [PubMed]
- Yang G, Sun Z, Zhang N. Reshaping the role of m6A modification in cancer transcriptome: a review. Cancer Cell Int 2020;20:353. [Crossref] [PubMed]
- Xiao H, Fan X, Zhang R, et al. Upregulated N6-Methyladenosine RNA in Peripheral Blood: Potential Diagnostic Biomarker for Breast Cancer. Cancer Res Treat 2021;53:399-408. [Crossref] [PubMed]
- Shriwas O, Mohapatra P, Mohanty S, et al. The Impact of m6A RNA Modification in Therapy Resistance of Cancer: Implication in Chemotherapy, Radiotherapy, and Immunotherapy. Front Oncol 2021;10:612337. [Crossref] [PubMed]
- Iaiza A, Tito C, Ianniello Z, et al. METTL3-dependent MALAT1 delocalization drives c-Myc induction in thymic epithelial tumors. Clin Epigenetics 2021;13:173. [Crossref] [PubMed]
- Xiao Y, Thakkar KN, Zhao H, et al. The m6A RNA demethylase FTO is a HIF-independent synthetic lethal partner with the VHL tumor suppressor. Proc Natl Acad Sci U S A 2020;117:21441-9. [Crossref] [PubMed]
- Li X, Tang J, Huang W, et al. The M6A methyltransferase METTL3: acting as a tumor suppressor in renal cell carcinoma. Oncotarget 2017;8:96103-16. [Crossref] [PubMed]
(English Language Editor: LJ. Roberts)