Identification of novel candidate genes and small molecule drugs in ovarian cancer by bioinformatics strategy
Introduction
Ovarian cancer (OC) is the second most common gynecologic cancer and most deadly gynecologic malignancy around the world, with 1.6% incidence and 2.1% mortality in 2020 and a 5-year survival rate as 47.6% (1,2). As of 2021, OC is still the fifth cause of cancer related death in females in the United States (3). There are approximately 225,500 new cases and an estimated 140,200 ovarian cancer-related deaths each year (4). The high annual mortality was attributed to late diagnosis and recurrence associated with chemotherapy-resistance. Most patients diagnosed with metastatic disease will have disease recurrence within 5 years, and the 5-year survival rate is reduced to <20% (5). At present, the most common treatment is cytoreductive surgery combined with platinum-based chemotherapy. However, 60% of patients relapsed following first-line treatment and 50% were resistant to chemotherapy. Therefore, it is crucial to discover valid biomarkers for early diagnosis and find drugs that affect the mechanism of drug resistance.
In recent years, a significant number of genes and targets related to the disease were found because of the Human Genome Project and other genomic studies. With the rapid development of gene microarray technologies and high throughput sequencing (6), more and more ovarian cancer related differentially expressed genes (DEGs) and functional pathways having exactly been identified, leading to breakthroughs in the ovarian cancer research. Thanks to the advent of bioinformatics, direct drug development for biomolecules against disease-related targets has gradually become the primary strategy for drug development (7).
In this research, GSE18520 and GSE14407 from the Gene Expression Omnibus (GEO) database were selected and the overlapped DEGs were detected. The Gene Ontology (GO) analysis and Kyoto Encyclopedia of Genes and Genome (KEGG) pathway enrichment analysis were performed to establish the PPI network of DEGs and identified the hub genes. Furthermore, we validated the expression of the identified hub genes by using Gene Expression Profiling Interactive Analysis (GEPIA), Oncomine database and Human Protein Atlas (HPA) databases. The prognostic value of them were evaluated by the Kaplan Meier plotter online tool. NCAPG is one of the identified hub gene. The expression of NCAPG was further explored by immunohistochemistry in our OC tissues. Moreover, we used the CMap database to look for prospective small compounds with therapeutic efficacy based on OC RNA-seq, indicating the way for future drug development. We present the following article in accordance with the REMARK reporting checklist (available at https://tcr.amegroups.com/article/view/10.21037/tcr-21-2890/rc).
Methods
Data resources
Two original microarray datasets were downloaded from the GEO database (http://www.ncbi.nlm.nih.gov/geo/). GSE18520, which was based on the GPL570 (HG-U133_Plus_2; Affymetrix Human Genome U133 Plus 2.0 Array), contained 53 high-grade serous papillary carcinoma samples and 10 normal ovarian surface epithelium samples. The platform for GSE14407 is also GPL570, contained 12 serous papillary ovarian cancer samples and 12 normal ovarian epithelium samples.
Data preprocessing and DEGs screening
The GEO2R online tools were utilized to identify the DEGs between OC samples and normal tissue samples with the cutoff threshold of adjust P<0.05 and |log2(fold change)| >2. The online Venn 2.1.0 software (https://bioinfogp.cnb.csic.es/tools/venny/) was applied to get the overlap genes between the two datasets.
Functional annotation and pathway analysis
GO enrichment analysis is a commonly used approach to investigate the biological process (BP), molecular function (MF) and cell component (CC) of genes or gene products. KEGG is a widely used database for identifying functional and metabolic pathways. The DAVID (Annotation, Visualization and Integrated Discovery) database (version 6.8; http://david.abcc.ncifcrf.gov/) was used to carry out GO function and KEGG pathway enrichment analysis. P<0.05 was set as the cut-off criterion for the significant enrichment.
Protein-protein interaction (PPI) network construction and module analysis
The online database Search Tool for the Retrieval of Interacting Genes (STRING, version 11.0, https://string-db.org/) was used to illustrate the potential interrelationships among the overlapping DEGs. Then the Cytoscape software (https://cytoscape.org) was utilized to construct the network and investigate the interaction relationship of the DEGs encoding proteins in OC. A combined score above 0.4 was set as the cut-off value. Finally, the MCODE (Molecular Complex Detection) plug-in of Cytoscape software was used to visualize and identify significant modules from the PPI network for the selection of hub genes (degree =5, node score =0.2, k-core =2, and maximum depth =100).
Validation of key genes
The intersecting genes from the enrichment pathways and top 10 nodes in PPI network were identified as hub genes. “Expression analysis-Box Plots” module of The Gene Expression Profiling Interactive Analysis (GEPIA, http://gepia.cancer-pku.cn) was adopted for analyzing hub genes mRNA levels in OC, considering that the default setting cut-off value are the |Log2FC| Cutoff:1, P value Cutoff: 0.01, and “Match TCGA normal and GTEx data”. The Oncomine database (https://www.oncomine.org/) were also used to analyze their expression in OC compared with levels in normal specimens. The threshold value was set as follows: fold change: 1, P value: 0.05, and gene ranking: 10%.
The immunohistochemical results of the real hub genes were obtained from the Human Protein Atlas (version 18, https://www.proteinatlas.org/). The Kaplan-Meier-plotter (http://kmplot.com/analysis) online database was used to evaluate survival analysis of these 10 hub genes. A total of 1,656 OC patients for overall survival (OS) analysis. The genes and Affy ID are as follows: CCNB1 (214710_s_at), TOP2A (201291_s_at), NUSAP1 (218039_at), NCAPG (218662_s_at), KIF20A (218755_at), DLGAP5 (203764_at), CDK1 (203468_at), FOXM1 (202580_x_at), RRM2 (202580_x_at), AURKB (209464_at).
Median expression of hub genes of all samples was chosen as a cut-off to divide samples into the high expression group and low expression group. The Kaplan-Meier analysis was performed to investigate the prognosis of patients in the two groups.
Identification of candidate small molecules
The Connectivity Map (CMap) database (http://www.broadinstitute.org/cmap/), which is based on the gene signature of OC was used to find potential drugs for patients. The common dysregulated probe sets were used to query the CMap database.
Using the perl language, convert the up- regulated genes and down-regulated genes in Table 1 to GPL571 probe IDs and enter them into the CMap official online “rapid query” tool. Tag files can be found in the Supplementary Material. As a result, the enrichment scores that represent similarity were calculated (ranging from –1 to 1). A positive connectivity score (closer to 1) shows that a drug is capable to induce the input signature of OC cells, whereas a negative connectivity score (closer to −1) shows that a drug is capable to reverse the input signature. The negative connectivity scores were confirmed as candidate molecules with potential therapeutic value. Tomographs of these candidate small molecular drugs were inquired in the Pubchem database (https://pubchem.ncbi.nlm.nih.gov/).
Table 1
Characteristics | Malignant (n=69) | Borderline (n=15) | Benign (n=35) |
---|---|---|---|
Age, years, n (%) | |||
≤60 | 48 (69.6) | 12 (80.0) | 27 (77.1) |
>60 | 21 (30.4) | 3 (20.0) | 8 (22.9) |
FIGO stage, n (%) | |||
I | 8 (11.6) | ||
II | 10 (14.5) | ||
III | 45 (65.2) | ||
IV | 6 (8.7) | ||
Histologic grade, n (%) | |||
Low grade | 31 (44.9) | ||
High grade | 38 (55.1) | ||
CA125, n (%) | |||
≤500 | 27 (39.1) | ||
>500 | 42 (60.9) | ||
HE4, n (%) | |||
≤500 | 39 (56.5) | ||
>500 | 30 (43.5) | ||
Ascites | |||
Yes | 45 (65.2) | ||
No | 24 (34.8) | ||
Lymph node metastasis | |||
Yes | 37 (53.6) | ||
No | 29 (42.0) | ||
Ki 67, n (%) | |||
≤50% | 19 (27.5) | ||
>50% | 50 (72.5) |
FIGO, International Federation of Gynecology and Obstetrics.
Human tissue samples
Totally 69 serous ovarian cancer, 35 benign serous ovarian cystadenoma tissues and 15 borderline serous ovarian cystadenoma tissues were obtained, all of them underwent first surgery at the Department of Obstetrics and Gynecology of the First Hospital of Lanzhou University from January 2015 to December 2020. None of them had received any chemoradiation, radiation, or other treatments before the surgery. The diagnoses of the pathological sections were confirmed by pathology department consultation (Table 1). This study was approved by the Ethics Committee of the First Hospital of Lanzhou University. All the patients were informed and signed informed consent.
Immunohistochemical analysis
Each tissue was embedded in paraffin blocks and continues processed into 4-µm thick paraffin sections. Specimens were deparaffinized and hydrated, 3% H2O2 were used for 15 min to eliminate endogenous peroxidase and then citrate buffer solution (pH=6) were used to repair antigens. The sections were then blocked with 10% serum for 60 min and incubated with rabbit anti-NCAPG antibody (1:100; Abcam, ab251864, Cambridge, UK) at 4 ℃ overnight followed by incubation with secondary antibody goat anti-rabbit IgG (Zhongshan Golden bridge Biotechnology, SAP-9100, Beijing, China) for 30 mins at 37 ℃. Then diaminobenzidine and hematoxylin were employed to stain these sections, and they were sealed with neutral resins. The results were evaluated by two pathologists blindly and randomly. The positive staining of NCAPG was located in the cytoplasm and membrane. The intensity of NCAPG expression was scored as follows: 0, negative staining; 1, mild staining; 2, moderate staining; 3, strong staining. The percentage of NCAPG expression was scored as follows: 0, <10% positive cancer cells; 1, 11–25% positive cancer cells; 2, 26–50% positive cancer cells; 3, 51–75% positive cancer cells; 4, >75% positive cancer cells. The final scores of each staining were calculated by multiplying the two scores. The section with a score of 0–4 was defined as a low expression, whereas the section with a score of 5–12 was defined as high expression.
Statistical analysis
Statistical analysis was performed using SPSS software (version 26.0). In our tissue samples, Chi-square test was used to analyze the difference between three groups. The P<0.05 was considered to indicate a statistically significant difference.
Ethical statement
The study was conducted in accordance with the Declaration of Helsinki (as revised in 2013). The study was approved by the Ethics Committee of the First Hospital of Lanzhou University (No. LDYYLL2019-275) and individual consent for this retrospective analysis was waived.
Results
Identification of DEGs in OC
A total of 433 overlapping genes (Table 2) were extracted to be differentially expressed. 159 up-regulated genes (Figure 1A) and 274 down-regulated genes (Figure 1B) as shown respectively in the Venn diagrams.
Table 2
DEGs | Genes name |
---|---|
Upregulated (159) | HMGA1 LZTS3 MECOM SUSD2 CLUH CD24 KLHL14 DUXAP10 SOX17 MCM10 LOC101060391 C1orf186 CLDN3 GRHL2 ELF3 LYPD6B WFDC2 NEK2 DLX6-AS1 CP E2F1 MPZL2 FOXM1 NES SMIM22 ESM1 ARHGAP11B SLC52A2 KLF12 KIF14 CLDN4 C1orf106 TIMELESS CYYR1 FOLR1 SUSD4 PSAT1 DLGAP5 EHF NRXN1 PAX8 KIF20A SCGB2A1 FILIP1 BUB1 GPM6B LPAR3 FAM83D TTK AIF1L TRIP13 CENPF CEP55 NCAPG SLC4A11 RNF157-AS1 DTL IGF2BP3 CATIP-AS1 CDCA5 KIF4A S100A1 SLC2A1 EPCAM KIF11 EPHX4 STON2 BUB1B MELK UBE2C LIMS3 CRABP2 MUC1 SULT1C2 CENPA SOX9 PROM2 CBS SORT1 ESRP1 MXD3 CKS2 CDK1 PRC1 FZD10 CDC20 CXXC5 TOP2A CENPK CDH6 MMP7 LOC101928554 FOXQ1 ECT2 LINC00511 ESCO2 C8orf4 CCNB2 NUSAP1 S100A2 KLK6 LYNX1 PRR11 KIAA0101 C12orf56 LYPD1 EPB41L5 RGS1 PRSS2 LRP8 CENPU NR2F6 SLC26A7 HMMR CCNB1 RAD51AP1 S100A13 RRM2 PRAME ST6GALNAC1 MTHFD2 MTFR2 MAL TTC39A INHBB KLK8 AURKB KIAA1217 LOC150051 DCDC2 DIS3L2 HEY2 GLDC TMTC1 LCN2 DUXAP10 DEPDC1 RAPGEF3 DIRAS2 MAGEA11 HIST1H1C PTX3 KCCAT333 PCDH7 NRTN TFAP2A SCGB1D2 HMGA2 MEOX1 FAM107A DEFB1 PTH2R LOC613266 COL12A1 WDR72 LIX1 SST PDCL2 |
Downregulated (274) | LINC01105 BNC1 ITLN1 LHX9 MAF PDE8B GADL1 PEX5L SVEP1 ABCA8 REEP1 NKX3-1 METTL7A HAND2-AS1 HBB SNX29P2 SNCAIP OGN GFPT2 SYT4 SNX13 ANTXR2 SLC4A4 MNDA AADACL2 DIRAS3 CHGB WNT2B CLDN15 HBA1 NPY1R AOX1 GPRASP1 BCHE PRG4 PPM1K TBX3 LGALS2 CLEC4M DOCK5 HELQ TCEAL2 AGTR1 SMPD3 TCEAL7 S100A10 SFRP1 PCOLCE2 SNCA PKD2 ADH1B SHISA3 DDR2 PTPRZ1 DFNA5 B3GALT2 MTUS1 LGALS8 ADH1C PRSS35 EIF1 ALDH1A2 DSC3 AKAP12 TCF21 PCDH9 PROCR NBR1 CMAHP CALB2 CPB1 CSGALNACT1 ARHGAP18 GATA4 FLRT2 SEMA5A THBD OGFOD1 DST BTD FAM153B MGARP COL8A1 LHX2 WNT16 LSAMP HBG1 ABHD12B ANKRD29 CHRDL1 SORBS2 HLF DAB2 ADGRD1 PTGDR OMD GAS1RR RYR2 SERTM1 KLF2 RTN1 MGC24103 LOC100996760 MCTP2 KCNT2 TMEM37 PDGFD SPOCK1 PLEKHH2 VGLL3 TMEM255A ILDR2 ARX ANXA8 LINC01133 ANXA1 NELL2 DMD PTGIS NR2F1-AS1 HHIP LOC286191 CXorf57 SLC30A4 PAPSS2 FGF1 PLCE1 BDH2 TFPI BCAR3 RBMS3 NEFH PEG3 PMP22 IL18 FAM153B C7 RERG MEOX2 HSD17B2 MARCO PRDM5 RAB27B GHR IRAK3 AKT3 FGF13 ABI3BP CALCRL MEDAG CPED1 NT5E HPSE EZR OLFML1 SLC41A2 ALDH1A1 NEGR1 PTGER3 BAMBI PCDH17 CFI GIPC2 DOCK11 CELF2 MUM1L1 SBSPON NLGN4X CFC1 DPP10 RNASE4 AQP9 CYP39A1 LY75 DTNA RGS4 SCD5 EFEMP1 MMP28 LIMA1 GPM6A SDPR PRRX1 NAP1L2 CLMP GABRB2 RUNX1T1 RNF128 NXPH2 AKR1C2 CYP2U1 CNTN1 PTHLH NAP1L3 SIGLEC11 FABP4 PITPNC1 CAV1 DSE CNTN4 KLF4 PDPN FLRT3 C1orf168 FAM134B ARHGAP44 CBLN4 GATM HSD17B6 ECM2 PGR PKHD1L1 CFH SFRP2 HPGD ADAMTS3 TMEM150C DPYD MGP MCOLN3 LAMA4 CYS1 NKAIN2 COL14A1 S100A8 TRPC1 FAM13C MCC RARRES1 TBX18 COL3A1 BNC2 LRP2 DCN LINC01279 NBEA SLITRK5 ITM2A FRY GNG11 FGF9 FAM155A CCDC80 LINC01116 ZFPM2 GAS1 FRAS1 TMEM98 WDR17 TSPAN8 RASSF3 SLIT2 PRKAR2B NDN TFPI2 DPP6 MDFIC TLE4 PROS1 PHLDB2 CFH NRXN3 MSRB3 BEX1 MEIS2 ARRDC4 GATA6 STK26 MAOA CRNDE WNT5A RSPO1 MICU3 PCDH20 |
DEGs, differentially expressed genes.
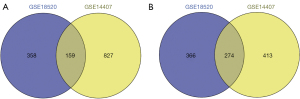
Enrichment analyses
The bubble map was revealed via the ggplot2.R package for the top 10 significant GO and pathway based on P value. For BP of GO enrichment, GO analysis results showed that DEGs were markedly enriched in negative regulation of transcription (Figure 2A). As for CC of GO enrichment, DEGs were particularly involved in extracellular region (Figure 2B). In addition to MF, the overlapping DEGs were particularly enriched in calcium ion binding (Figure 2C). Furthermore, KEGG pathway enrichment analysis demonstrated that these DEGs were mainly enriched in pathways in cancer and proteoglycans in cancer (Figure 2D).
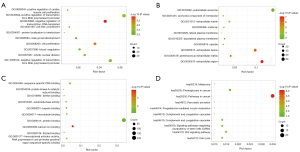
PPI network construction and module analysis
There were 344 nodes and 1,596 edges found in the network (Figure 3A). The module genes CDK1, FOXM1, CCNB1, RRM2, AURKB, TOP2A, NUSAP1, NCAPG, KIF20A and DLGAP5 were the most significant 10 node degree genes and identified as the hub genes (Figure 3B). Additionally, the most important PPI network module was detected using MCODE (Figure 3C). Pathway enrichment analysis showed that the most top module genes were primarily enriched in cell cycle, p53 signaling pathway, progesterone-mediated oocyte maturation and oocyte meiosis (Table 3).
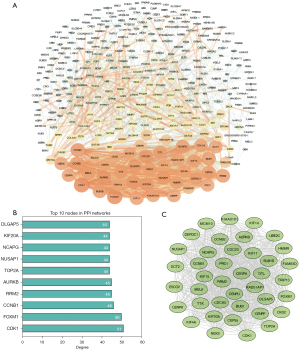
Table 3
Pathway ID | Pathway description | Gene counts | P value |
---|---|---|---|
4110 | Cell cycle | 7 | 1.39E-08 |
4115 | p53 signaling pathway | 4 | 1.33E-04 |
4914 | Progesterone-mediated oocyte maturation | 4 | 3.06E-04 |
4114 | Oocyte meiosis | 4 | 5.99E-04 |
KEGG, Kyoto Encyclopedia of Genes and Genome.
Validation of hub genes
GEPIA and Oncomine tool verified that the expressions of CDK1, FOXM1, CCNB1, RRM2, AURKB, TOP2A, NUSAP1, NCAPG, KIF20A and DLGAP5 were significant elevated in OC compared with normal ovarian tissues (Figure 4A,4B). It was found that higher expressions of CCNB1, TOP2A, NUSAP1, NCAPG, KIF20A and DLGAP5 were significantly correlated with worse overall survival for OC patients (Figure 5). Via the Human Protein Atlas database, these six genes’ expression were higher in OC compared than in normal tissues (Figure 6).
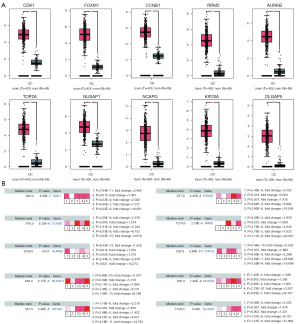
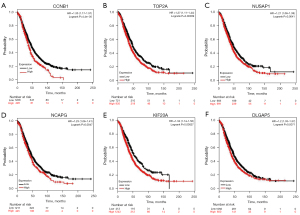
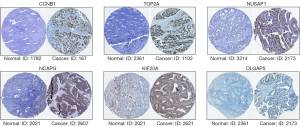
Validation of NCAPG expression in patient tissues
As shown in Table 4 and Figure 7, NCAPG was mainly located in the cell cytoplasm and membrane. The scoring system results demonstrated that NCAPG high expression rate was 58/69 (84.1%) in serous ovarian cancer (SOC) samples, which was significantly higher than that in benign serous ovarian cystadenoma (5/35, 14.3%) and borderline serous ovarian cystadenoma tissues (2/15, 13.3%) (P<0.05).
Table 4
Group | Cases | NCAPG expression | |||
---|---|---|---|---|---|
High expression cases | Low expression cases | χ2 | P value | ||
Malignant | 69 | 58 (84.1%) | 11 (15.9%) | 57.412 | 0.000 |
Benign | 35 | 5 (14.3%) | 30 (85.7%) | ||
Borderline | 15 | 2 (13.3%) | 13 (86.7%) |
NCAPG, non-structural maintenance of chromosomes condensin I complex subunit G.
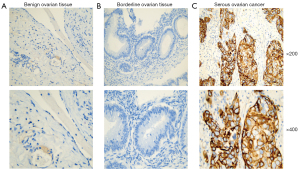
Identification of related small molecule drugs
According to the P value, the top 10 related small molecules which matched to the OC gene expression changes are listed in (Table 5). Six of these small molecules indicated a negative correlation and showed that latent capacity of tumor inhibition in clinical applications, four of them indicated a positively correlation. Among them, trichostatin A, vorinostat, phenoxybenzamine, 8-azaguanine showed a significantly negative correlation (P<0.05) and the tomography of them shown in (Figure 8).
Table 5
Rank | CMap name | Mean | N | Enrichment | P value |
---|---|---|---|---|---|
1 | Trichostatin A | –0.469 | 182 | –0.428 | 0.00000 |
2 | Vorinostat | –0.598 | 12 | –0.621 | 0.00000 |
3 | Phenoxybenzamine | –0.835 | 4 | –0.937 | 0.00000 |
4 | 8-azaguanine | –0.768 | 4 | –0.917 | 0.00010 |
5 | Resveratrol | –0.694 | 9 | –0.669 | 0.00016 |
6 | Podophyllotoxin | 0.672 | 4 | 0.849 | 0.00074 |
7 | Chenodeoxycholic acid | 0.595 | 4 | 0.846 | 0.00080 |
8 | Thioperamide | 0.563 | 5 | 0.798 | 0.00082 |
9 | Vinburnine | 0.576 | 4 | 0.83 | 0.00127 |
10 | Luteolin | –0.734 | 4 | –0.835 | 0.00131 |
Cmap, Connectivity Map; OC, ovarian cancer.
Discussion
Ovarian cancer remains the most lethal gynecologic cancer due to delayed diagnosis, which could explain the dismal prognosis of OC patients. Early diagnosis of OC will improve the overall survival. Therefore, to discover reliable biomarkers and to reveal the possible molecular mechanisms of OC is especially important for diagnosis and prognosis. In the present study, we utilized bioinformatics methods to identify novel biomarkers and potential small molecule drugs in OC by integrating two expression profile datasets GSE18520 and GSE14407. 433 common DEGs were identified, among which 159 genes were upregulated and 274 genes were downregulated. Applied GO function and KEGG pathway enrichment analysis, we found that the biological processes involved in DEGs were mainly acted in negative regulation of transcription and DNA-templated. The DEGs were mainly enriched in Pathways in cancer and Proteoglycans in cancer. These DEGs might involve in the carcinogenesis of OC by means of affect the biological behavior of OC cells via these pathways.
Based on the identified DEGs, we constructed a PPI network with 344 nodes and 1596 interactions. We extracted the most important module with the most significant degree from the PPI network. The top ten module genes CDK1, FOXM1, CCNB1, RRM2, AURKB, TOP2A, NUSAP1, NCAPG, KIF20A, and DLGAP5 were chosen as hub genes based on their degree rankings. Survival analysis for 1,656 OC patients from Kaplan Meier plotter database showed that patients with higher expression of CCNB1, TOP2A, NUSAP1, NCAPG, KIF20A and DLGAP5 experienced worse survival outcomes than those with low expression. These results provide signs for us to create an ovarian cancer prognosis model, allow us to more correctly identify high-risk patients. The GEPIA, Oncomine and the Human Protein Atlas database showed that they were all overexpressed in OC tissue.
NCAPG (non-structural maintenance of chromosomes condensin I complex subunit G), a crucial component of the chromosomes condensin complex, which plays an important role in the condensation and stabilization of chromosomes during meiosis and mitosis (8). In hepatocellular carcinoma (HCC), Wang et al. found that elevated NCAPG expression was remarkably associated with worse disease-free survival and overall survival in patients suffering from HCC. NCAPG could be regard as a novel prognostic biomarker to predict HCC early recurrence after surgical resection (9). Arai et al. reported that high NCAPG expression was closely related to prognosis and clinical stage in patients with prostate cancer. Knockdown of NCAPG significantly inhibited the ability of proliferation, migrationand invasion in prostate cancer cells (10). In our studies, we verified the expression of NCAPG by the immunohistochemistry analysis in ovarian cancer tissues. The results showed that the expression rate of NCAPG in OC tissues was significantly higher than that in benign serous ovarian cystadenoma and borderline serous ovarian cystadenoma tissues that obtained from clinical patients, and the tendency was consistent with the results discussed above. Taken all these studies together, we assumed that NCAPG may be a valuable biomarker for the diagnosis and prognosis of various cancer patients.
CCNB1 (Cyclin B1), one member of the cyclin family, an important regulator in normal cell cycle progresses at the G2/M transitions by binding to CDK1 (11), plays a key role in various human malignancies. Han et al. found that knockdown long non-coding RNA SOX2OT could inhibit ovarian cancer cell migration and cell invasion by downregulated CCNB1 (12).
TOP2A (topoisomerase II alpha), is a key enzyme in DNA transcription and replication, repair, chromosome condensation and separation (13), which has been identified overexpression in several cancer types. Researchers found that TOP2A is the prime therapeutic target of many anticancer therapy regimens. It was proved that inhibitors targeting human topoisomerase II alpha were useful chemotherapy option for the treatment of many patients suffering from all kinds of cancers (14).
NUSAP1 (nucleolar and spindle associated protein 1) played a role in mitotic progression, spindle formation, and stability overexpression of NUSAP1 has been found in a variety of human malignancies, and it is commonly linked to tumor invasiveness. Zhang et al. reported that the expression of NUSAP1 in OC tissues and cells was upregulated, which could promote the proliferation and invasiveness of OC cells (15).
KIF20A (Kinesin family member 20A), as a mitotic kinesin-like protein, belongs to the kinesin perfamily-6. Depending on microtubule-dependent plus-end motility, KIF20A is contributed to different cellular processes including formation of the mitotic spindle and chromosome partitioning. In the field of oncology, increasing studies illustrated its carcinogenic properties in various types of cancers.
DLGAP5 (Discs large-associated protein 5), also known as HURP, is a mitotic spindle protein that plays a crucial role in the formation of novel tubulin sheet, the formation of facilitating spindle, and forming a connection between the centrosome and kinetochore (16). A large number of studies has shown DLGAP5 serve as oncogenic role in many types of human cancers. Chen et al. reported that DLGAP5 silencing could suppress tumorigenicity and cellular proliferation by arresting the cell cycle at the G2/M phase in ovarian cancer cells (17). All results above suggested that these 6 hub genes may relate to the occurrence and development of OC.
In this study, we also identified several small molecules with potential therapeutic efficacy against OC. Trichostatin A (TSA), as a potent inhibitor of histone deacetylase (HDAC), was considered to be the most significant small molecule with strong dose-dependent antitumor activity. Drug resistance is the main cause of the high mortality in patients with OC. Combination therapy is a possible method of overcoming drug resistance. More and more studies confirmed that TSA could enhance the antitumor effects when combinated with other anticancer drugs in multifarious cancer cells (18-20). Lambert (21) found that TSA could reduce cell viability instigates apoptosis, autophagy, inhibition of cell cycle progression in human ovarian cancer cells. Zhang (22) confirmed that TSA caused significant cytotoxicity and induced apoptosis in SKOV3 cells. Vorinostat is a small molecule inhibitor of both class I and class II HDAC enzymes. It can directly activate p21WAF1/CIP1 gene transcription, which is one of the most important cyclin-dependent kinase (CDK) inhibitors in ovarian cancer cells (23). Vorinostat has been reported to produce specific modifications in the pattern of acetylation and methylation of lysines in H3 and H4 histones that are associated with the p21WAF1/CIP1 gene promoter (24). It played an important role in antiproliferative and proapoptotic effects by inducing the expression of IL-8/CXCL8 in OC cells (25). In ovarian cancer cell lines, the combination of vorinostat and paclitaxel was superior to either drug individually (26). Phenoxybenzamine, is approved for the treatment of hypertensive emergencies associated with the adrenal medulla tumors and pheochromocytomas by the US Food and Drug Administration. At present, more and more studies have reported that phenoxybenzamine can inhibit the growth, invasion, and migration of human tumor cells as a histone deacetylase inhibitor. Inchiosa (27) reported that Phenoxybenzamine could inhibitory the activity of OVCAR-8 cells. 8-Azaguanin (8-AG), originally developed as an antineoplastic agent, but recent study has found it to be a novel immunomodulatory drug (28). 8-AG as an immunomodulatory drug, increased the functionality of NK cells via NK-target cell conjugate formation and cytolytic granule polarization. But it has not yet been demonstrated to have effectiveness against OC, and therefore further research is required.
In summary, by means of bioinformatics analysis, we identified six real hub genes of OC, including CCNB1, TOP2A, NUSAP1, NCAPG, KIF20A and DLGAP5. Survival analysis showed these genes were significantly associated with negative survival of patients with OC. They might play crucial roles and as novel biomarkers in OC diagnosis, prognosis and treatment. In this study, we also indicated several important signaling pathways associated with the OC onset and progression, and identified a group of candidate small molecule drugs as adjunctive agents for OC. However, further studies are needed to determine the relationship between OC and these pathways and these small molecule drugs.
Acknowledgments
Funding: The present study was supported by the Fundamental Research Funds for the Central Universities (No. lzujbky-2021-kb39), the Research Program of the First Hospital of Lanzhou University (Nos. ldyyyn2019-83, ldyyyn2020-105).
Footnote
Reporting Checklist: The authors have completed the REMARK reporting checklist. Available at https://tcr.amegroups.com/article/view/10.21037/tcr-21-2890/rc
Data Sharing Statement: Available at https://tcr.amegroups.com/article/view/10.21037/tcr-21-2890/dss
Conflicts of Interest: All authors have completed the ICMJE uniform disclosure form (available at https://tcr.amegroups.com/article/view/10.21037/tcr-21-2890/coif). The authors have no conflicts of interest to declare.
Ethical Statement: The authors are accountable for all aspects of the work in ensuring that questions related to the accuracy or integrity of any part of the work are appropriately investigated and resolved. The study was conducted in accordance with the Declaration of Helsinki (as revised in 2013). The study was approved by the Ethics Committee of the First Hospital of Lanzhou University (No. LDYYLL2019-275) and individual consent for this retrospective analysis was waived.
Open Access Statement: This is an Open Access article distributed in accordance with the Creative Commons Attribution-NonCommercial-NoDerivs 4.0 International License (CC BY-NC-ND 4.0), which permits the non-commercial replication and distribution of the article with the strict proviso that no changes or edits are made and the original work is properly cited (including links to both the formal publication through the relevant DOI and the license). See: https://creativecommons.org/licenses/by-nc-nd/4.0/.
References
- Siegel RL, Miller KD, Jemal A. Cancer statistics, 2019. CA Cancer J Clin 2019;69:7-34. [Crossref] [PubMed]
- Sung H, Ferlay J, Siegel RL, et al. Global Cancer Statistics 2020: GLOBOCAN Estimates of Incidence and Mortality Worldwide for 36 Cancers in 185 Countries. CA Cancer J Clin 2021;71:209-49. [Crossref] [PubMed]
- Siegel RL, Miller KD, Fuchs HE, et al. Cancer Statistics, 2021. CA Cancer J Clin. 2021;71:7-33. [Crossref] [PubMed]
- Lisio MA, Fu L, Goyeneche A, et al. High-Grade Serous Ovarian Cancer: Basic Sciences, Clinical and Therapeutic Standpoints. Int J Mol Sci 2019;20:952. [Crossref] [PubMed]
- Torre LA, Trabert B, DeSantis CE, et al. Ovarian cancer statistics, 2018. CA Cancer J Clin 2018;68:284-96. [Crossref] [PubMed]
- Kulasingam V, Diamandis EP. Strategies for discovering novel cancer biomarkers through utilization of emerging technologies. Nat Clin Pract Oncol 2008;5:588-99. [Crossref] [PubMed]
- Brogi S. Computational Approaches for Drug Discovery. Molecules 2019;24:3061. [Crossref] [PubMed]
- Sutani T, Sakata T, Nakato R, et al. Condensin targets and reduces unwound DNA structures associated with transcription in mitotic chromosome condensation. Nat Commun 2015;6:7815. [Crossref] [PubMed]
- Wang Y, Gao B, Tan PY, et al. Genome-wide CRISPR knockout screens identify NCAPG as an essential oncogene for hepatocellular carcinoma tumor growth. FASEB J 2019;33:8759-70. [Crossref] [PubMed]
- Arai T, Okato A, Yamada Y, et al. Regulation of NCAPG by miR-99a-3p (passenger strand) inhibits cancer cell aggressiveness and is involved in CRPC. Cancer Med 2018;7:1988-2002. [Crossref] [PubMed]
- Park TJ, Kim JY, Oh SP, et al. TIS21 negatively regulates hepatocarcinogenesis by disruption of cyclin B1-Forkhead box M1 regulation loop. Hepatology 2008;47:1533-43. [Crossref] [PubMed]
- Han L, Zhang W, Zhang B, et al. Long non-coding RNA SOX2OT promotes cell proliferation and motility in human ovarian cancer. Exp Ther Med 2018;15:2182-8. [PubMed]
- Nuncia-Cantarero M, Martinez-Canales S, Andrés-Pretel F, et al. Functional transcriptomic annotation and protein-protein interaction network analysis identify NEK2, BIRC5, and TOP2A as potential targets in obese patients with luminal A breast cancer. Breast Cancer Res Treat 2018;168:613-23. [Crossref] [PubMed]
- Wang W, Tse-Dinh YC. Recent Advances in Use of Topoisomerase Inhibitors in Combination Cancer Therapy. Curr Top Med Chem 2019;19:730-40. [Crossref] [PubMed]
- Zhang Y, Huang K, Cai H, et al. The role of nucleolar spindle-associated protein 1 in human ovarian cancer. J Cell Biochem 2020;121:4397-405. [Crossref] [PubMed]
- Wang Q, Chen Y, Feng H, et al. Prognostic and predictive value of HURP in non small cell lung cancer. Oncol Rep 2018;39:1682-92. [Crossref] [PubMed]
- Chen X, Thiaville MM, Chen L, et al. Defining NOTCH3 target genes in ovarian cancer. Cancer Res 2012;72:2294-303. [Crossref] [PubMed]
- Spartalis E, Athanasiadis DI, Chrysikos D, et al. Histone Deacetylase Inhibitors and Anaplastic Thyroid Carcinoma. Anticancer Res 2019;39:1119-27. [Crossref] [PubMed]
- Tang D, Yao R, Zhao D, et al. Trichostatin A reverses the chemoresistance of lung cancer with high IGFBP2 expression through enhancing autophagy. Sci Rep 2018;8:3917. [Crossref] [PubMed]
- Vigushin DM, Ali S, Pace PE, et al. Trichostatin A is a histone deacetylase inhibitor with potent antitumor activity against breast cancer in vivo. Clin Cancer Res 2001;7:971-6. [PubMed]
- Lambert IH, Nielsen D, Stürup S. Impact of the histone deacetylase inhibitor trichostatin A on active uptake, volume-sensitive release of taurine, and cell fate in human ovarian cancer cells. Am J Physiol Cell Physiol 2020;318:C581-97. [Crossref] [PubMed]
- Zhang XF, Huang FH, Zhang GL, et al. Novel biomolecule lycopene-reduced graphene oxide-silver nanoparticle enhances apoptotic potential of trichostatin A in human ovarian cancer cells (SKOV3). Int J Nanomedicine 2017;12:7551-75. [Crossref] [PubMed]
- Sasakawa Y, Naoe Y, Inoue T, et al. Effects of FK228, a novel histone deacetylase inhibitor, on human lymphoma U-937 cells in vitro and in vivo. Biochem Pharmacol 2002;64:1079-90. [Crossref] [PubMed]
- Gui CY, Ngo L, Xu WS, et al. Histone deacetylase (HDAC) inhibitor activation of p21WAF1 involves changes in promoter-associated proteins, including HDAC1. Proc Natl Acad Sci U S A 2004;101:1241-6. [Crossref] [PubMed]
- Gatla HR, Zou Y, Uddin MM, et al. Histone Deacetylase (HDAC) Inhibition Induces IκB Kinase (IKK)-dependent Interleukin-8/CXCL8 Expression in Ovarian Cancer Cells. J Biol Chem 2017;292:5043-54. [Crossref] [PubMed]
- Cooper AL, Greenberg VL, Lancaster PS, et al. In vitro and in vivo histone deacetylase inhibitor therapy with suberoylanilide hydroxamic acid (SAHA) and paclitaxel in ovarian cancer. Gynecol Oncol 2007;104:596-601. [Crossref] [PubMed]
- Inchiosa MA Jr. Anti-tumor activity of phenoxybenzamine and its inhibition of histone deacetylases. PLoS One 2018;13:e0198514. [Crossref] [PubMed]
- Kim N, Choi JW, Song AY, et al. Direct potentiation of NK cell cytotoxicity by 8-azaguanine with potential antineoplastic activity. Int Immunopharmacol 2019;67:152-9. [Crossref] [PubMed]