Construction and validation of a pyroptosis-related gene signature in hepatocellular carcinoma based on RNA sequencing
Introduction
Primary liver cancer (PLC) is the most prevalent cause of cancer mortality worldwide, and the fifth most frequent cause in the United States, which has a 5-year relative survival rate of 20% (1). Hepatocellular carcinoma (HCC), which accounts for approximately 80% of all cases, is the most predominant type of PLC (2). Most HCC risk factors are modifiable, including obesity, excessive alcohol consumption, cigarette smoking, and infection with the hepatitis B or C virus (3). At present, surgical excision, orthotopic liver transplantation, thermal ablation, and transarterial therapy are the primary treatment modalities for HCC (4). However, HCC is a highly heterogeneous disease, both from a clinical and a molecular standpoint (5). It results in recurrence after ablation or excision, progression after effective chemoembolization, and inefficient radiological assessment. Therefore, HCC patients are advised to arrange multidisciplinary consultations, and treatment indications should be evaluated individually (6). Consequently, based on the limitations of the aforementioned HCC treatments, it is critical to build a novel signature to predict HCC patients’ survival and improve their clinical prognosis.
Pyroptosis is a type of apoptosis in which cells enlarge to the point where their membranes break, releasing pro-inflammatory substances that initiate an aggressive inflammatory response (7). Pyroptosis has been associated with carcinogenesis and metastasis in a variety of tumors, including cancers of the liver, skin, stomach, lung, and breast (8,9). However, the connection between pyroptosis and cancer is not clear. Pyroptosis not only creates an inflammatory microenvironment, but it also stimulates the transformation of normal cells into tumor cells and the death of tumor cells. Furthermore, due to the development of tumor heterogeneity and the dynamic microenvironment, patients often show inadequate responses to anticancer therapies (10). With the emergence of next-generation sequencing (NGS), recent studies have explored HCC in relation to tumor heterogeneity, the mutational gene landscape, and tumor immunology, thereby demonstrating the effectiveness and potential ability of NGS to guide patients in their treatment (5,11). Recent studies have established and validated pyroptosis-related signatures for ovarian and thyroid cancer in prognosis prediction based on RNA sequencing (RNA-seq) (12,13). Although some studies on pyroptosis and HCC have appeared (14,15), it is important to construct a novel prognostic model of pyroptosis gene.
Therefore, we conducted a thorough investigation to determine the expression levels of genes implicated in pyroptosis in normal liver tissues and HCC tumors. To investigate the prognosis of HCC and the relationship between pyroptosis and immunological infiltration, a novel signature was created and validated. We present the following article in accordance with the TRIPOD reporting checklist (available at https://tcr.amegroups.com/article/view/10.21037/tcr-21-2898/rc).
Methods
Data collection
The Cancer Genome Atlas (TCGA) database was utilized to obtain RNA sequencing (RNA-seq) data for 374 HCC cases and 50 normal samples, as well as related clinical information (downloaded on 21 October 2021, https://portal.gdc.cancer.gov/repository). The International Cancer Genome Collaboratory (ICGC) database was used to obtain the validation cohort’s RNA-seq data and clinical information (downloaded on 21 October 2021, https://dcc.icgc.org, ID: LIHC-US). The study was conducted in accordance with the Declaration of Helsinki (as revised in 2013).
Analysis of differentially expressed pyroptosis-related genes (PRGs)
From the literature (8,16-18), we identified 33 genes associating with pyroptosis, which are listed in Table S1. In brief, 50 normal liver tissues and 374 HCC samples were obtained to identify differentially expressed genes (DEGs) in normal and cancerous tissues. DEGs with a P value <0.05 were determined using R’s “limma” package. The genes related to pyroptosis in HCC were compared to normal liver tissues using a heatmap. The correlation of DEGs was analyzed with cutoff <0.2 and demonstrated by using “igraph” and “reshape2” modules within R package. Using the Search Tool for Recurring Instances of Neighbouring Genes (STRING) database (version 11.5), a protein-protein interaction (PPI) network was constructed for the DEGs (https://string-db.org).
Consensus clustering
The HCC samples were classified by consensus clustering using the “ConsensusClusterPlus” module within R package. Kaplan-Meier analysis of two cluster curves was performed using “survival” and “survminer” modules within R package. The relationship between clinical features and clusters was determined using the chi-square test and “pheatmap” module within R package.
Establishment and validation of the pyroptosis-related signature
To further analyze the predictive utility of PRGs, we utilized Cox regression analysis in the TCGA cohort to determine the relationship between survival status and each gene. To obtain the prognostic signature, LASSO Cox regression analysis was utilized. This approach was utilized to reduce the potential gene list and establish the prognostic signature. In regression analysis using the “glmnet” module within R package, the status and overall survival (OS) of the TCGA cohort were utilized as dependent variables. After maintaining the five genes and their associated coefficients, the penalty parameter (λ) was calculated by relying on the minimal requirements. The risk score calculation was as follows: risk score = (expression level of gene 1 × coefficient) + (coefficient × expression level of gene 2) + … + (expression level of gene n × coefficient). Based on the size of the risk score, patients in the cohort were divided into high- and low-risk groups. Kaplan-Meier curves with log-rank testing were utilized to assess the prognostic power in both TCGA and ICGC cohorts. Additionally, principal component analysis (PCA) was utilized to verify the specificity and sensitivity of the receiver operating characteristic (ROC) analyses, and a risk plot relying on the five-gene signature was built using “ggplot2” and “pheatmap” modules within R package. On an independent basis, univariate and multivariate Cox regression models were utilized to establish the predictive utility of the clinicopathological factors.
Function enrichment analysis
According to their median risk scores, individuals with HCC in the TCGA cohort were categorized into two groups. DEGs categorized as low- and high-risk were filtered in compliance with certain requirements [|log2FC| ≥1 and false discovery rate (FDR) <0.05]. Gene Ontology (GO) and Kyoto Encyclopedia of Genes and Genomes (KEGG) analysis were performed using the “ClusterProfiler” module within R package based on these DEGs. Single-sample gene set enrichment analysis (ssGSEA) was utilized for the infiltration score of immune cells and the activation of immune-related pathways using the “gsva” module within R package.
Cell culture
Cells (L02: human normal liver cells, Hep3B: liver cancer cells) from Chongqing Medical University’s Laboratory of Stem Cells and Tissue Engineering were used in this research. Cells were cultured in DMEM supplemented with 1% penicillin-streptomycin and 10% fetal bovine serum (FBS) (Gibco, USA). Cells were incubated at 37 ℃ and 5% CO2.
Quantitative real-time PCR (qRT-PCR)
According to the manufacturer’s instructions, total RNA was extracted from cells using TRIzol (Takara, Japan), followed by reverse transcription using the PrimeScript RT Reagent Kit (Takara, Japan). For qRT-PCR, the SYBR Premix Ex Taq II Kit was utilized (Takara). The 2−ΔΔCt approach was utilized to assess relative expression levels, with GAPDH serving as an internal control. Each experiment was repeated at least three independent times. Table S2 contains a list of the primers used.
Statistical analysis
To compare continuous variables among low- and high-risk groups, an independent t-test was employed. To test categorical data, the chi-square test was utilized. The log-rank test in univariate Kaplan-Meier analysis was utilized to evaluate survival, whereas multivariate survival analysis was accomplished using the Cox regression model. To examine differences in immune infiltration between two groups, the Mann-Whitney test was employed. Statistical significance in qRT-PCR was indicated by Student’s t-test P<0.05. R language (version 4.1.0) was used to perform statistical analyses.
Results
Identification of DEGs in the TCGA cohort
The analysis of the expression levels of 33 PRGs was performed using TCGA database (50 normal and 374 tumor tissues), and 25 PRGs (P<0.05) were identified. Among them, 15 genes (IL6, IL1B, NLRP3, ELANE, NLRP6, CASP4, SCAF11, PRKACA, CASP6, CASP9, AIM2, NLRP7, GPX4, NOD2, and PJVK) were downregulated, while ten genes (CASP3, NOD1, CASP8, GSDMD, NLRP1, GSDME, GSDMB, PYCARD, PLCG1, and GSDMC) were more prevalent within the cancer group. Figure 1A illustrates the heatmap of the RNA expression levels of these genes. PPI analysis was employed to understand the relationship between these pyroptosis-related genes, and the results were presented in Figure 1B. High confidence (0.7) was identified as the minimum required interaction score in the PPI study. We determined that CASP3, CASP8, NOD1, IL1B, NLRP3, AIM2, PYCARD, NLRP1, GSDMD, and CASP4 were the hub proteins. Figure 1C depicts the correlation network of these PRGs.
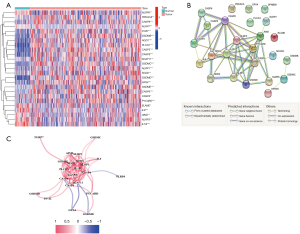
Tumor classification based on the PRGs
This classification determined the association between the expression of the 25 PRGs and the subtypes of HCC. All 374 HCC samples (TCGA cohort) were subjected to consensus clustering analysis. When the clustering variable k=2, the minimal intragroup linkage suggested that the 374 patients with HCC could be separated into two clusters (Figure 2A). The overall survival times of the two clusters were also examined, and significant differences were observed (P<0.001, Figure 2B). In the heatmap (Figure 2C), the gene expression profile and clinicopathological information are shown (online table available at: https://cdn.amegroups.cn/static/public/tcr-21-2898-1.pdf), and we found that tumor stage, tumor grade, and patient age between the two clusters were significantly different.
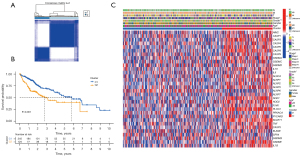
Gene signature established from the TCGA cohort
HCC patients with survival time and status were extracted from the TCGA database. The PRGs with survival times were initially screened using univariate Cox regression analysis. Six genes (CASP8, GSDMC, NLRP6, NOD1, NOD2, and PLCG1) that met the P<0.05 criterion were used for further analysis. Among these, five genes (CASP8, GSDMC, NLRP6, NOD2, and PLCG1) were linked to HRs >1, whereas NLRP6 was the protective gene with HRs <1 (Figure 3A). Using LASSO Cox regression analysis and relying on the optimum value λ, a five-gene signature was established (Figure 3B,3C). The formula was as follows: risk score = (0.225 * CASP8 exp.) + (0.305 * GSDMC exp.) + (−0.479 * NLRP6 exp.) + (0.561 * NOD2 exp.) + (0.180 * PLCG1 exp.) (exp. = expression). The median score was computed using the risk score formula that was utilized to classify HCC samples into low- and high-risk subgroups (Figure 3D). According to the findings, there was a significant variation in OS time among the low- and high-risk groups (P<0.001, Figure 3E). High-risk group patients died at a higher rate and survived for a shorter time than those in the low-risk group (Figure 3F). ROC analysis was utilized to assess the sensitivity and specificity of the prognostic model. The area under the receiver operating characteristic curve (AUC) for 1-year survival was 0.730, while the 3-year survival was 0.609, and the 5-year survival was 0.639 (Figure 3G). It was found that individuals with variable risk degrees could be categorized into two different groups using PCA (Figure 3H).
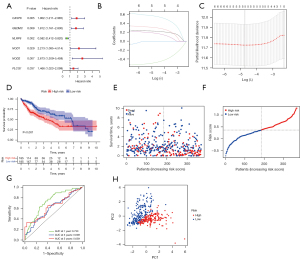
External validation of the risk signature
After removing patients with missing survival times and states, 294 HCC primary samples were included in the validation set from the ICGC cohort (ID: LIHC-US). Kaplan-Meier analysis relying on the median risk score in the TCGA cohort implied a statistically significant difference in the survival rate among low- and high-risk groups (P=0.005, Figure 4A). Our model had a high predictive efficacy when applied to the ICGC cohort, as determined by ROC curve analysis (AUC =0.682 for 1-year, 0.573 for 3-year, and 0.624 for 5-year survival) (Figure 4B). Additionally, in the ICGC cohort, 134 individuals were classed as low-risk, whereas the remaining 160 were classified as high-risk (Figure 4C). The low-risk group had a lower rate of mortality and a longer median survival time compared to the high-risk group (Figure 4D). According to PCA, the two subgroups were significantly distinct (Figure 4E).
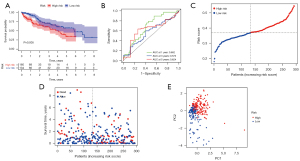
Independent prognostic predicting factor of the risk signature
Univariate and multivariate Cox regression analyses were performed to assess whether the risk score acquired from the gene signature model could be utilized independently in HCC treatment. In TCGA and ICGC cohorts, the risk score was an independent predictor of poor survival, as revealed by univariate Cox regression analysis (Figure 5A,5B). Following adjustment of additional confounding factors, patients with HCC could be accurately predicted by the risk score in both cohorts (Figure 5C,5D). A clinical parameter heatmap for the TCGA cohort (Figure 5E) demonstrated that the low- and high-risk groups were differently distributed in terms of tumor stage, tumor grade, and patient’s age (P<0.05).
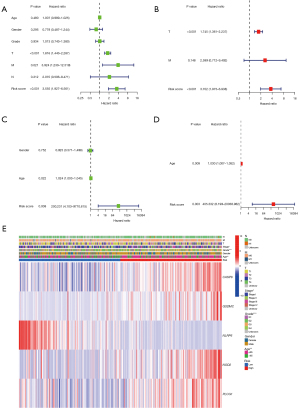
Signal pathway enrichment analysis
To further evaluate the variations in gene functions and pathways within the risk model subgroups, we extracted DEGs using the FDR <0.05 and |log2 FC| ≥1 criteria from the “limma” module of R package. Among the two groups in the TCGA cohort, 43 DEGs were detected (Table S3). On the basis of these DEGs, KEGG pathway analysis and GO enrichment were employed (details in online table available at: https://cdn.amegroups.cn/static/public/tcr-21-2898-2.pdf). The DEGs were mostly associated with metabolic signaling pathways, P450 cytochrome, and the peroxisome proliferator-activated receptor (PPAR) signaling pathway (Figure 6A,6B).
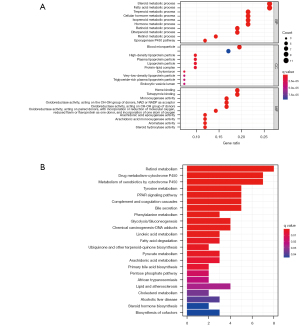
Comparison of the immune microenvironment between subgroups
ssGSEA utilized both TCGA and ICGC cohorts to assess the enrichment scores of 16 different types of immune cells among low and high-risk groups and 13 immune-related pathways. Except for natural killer (NK) cells, other immune cells were reduced in a low-risk group in the TCGA cohort (Figure 7A). With the exception of the interferon (IFN) response pathway, the others demonstrated decreased activity in the TCGA low-risk group (Figure 7B). In the ICGC cohort, a similar finding was achieved (Figure 7C,7D). In summary, our findings indicate that patients in the high-risk group had a higher active immunological state than those in the low-risk group.
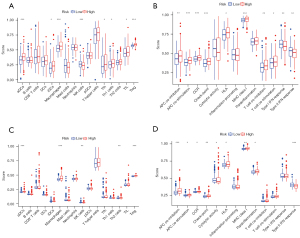
Expression levels of five prognostic genes
The expression of five prognostic genes (CASP8, GSDMC, NLRP6, NOD2, and PLCG1) in L02 and Hep3B cell lines was further validated. The mRNA expression of CASP8, GSDMC, NOD2, and PLCG1 was considerably greater in Hep3B, according to the results of qRT-PCR (P<0.05). However, in Hep3B, NLRP6 expression was reduced (P<0.05) (Figure 8). Notably, the expression patterns of the prognostic genes were compatible with the bioinformatic analysis results.
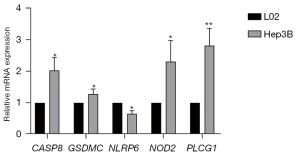
Discussion
PLC is widely considered as a leading cause of mortality worldwide. Hepatocellular carcinoma (HCC), hepatoblastoma (HB), intrahepatic cholangiocarcinoma (ICC), and HCC combined with ICC (cHCC-ICC) are the four primary subtypes of PLC (19). HCC accounts for nearly 80% of all types of PLC and is characterized by both phenotypic and molecular heterogeneity (20). Patients with HCC at different stages of progression often require different treatments, but only half receive systematic treatment (21). Indeed, HCC is a highly drug-resistant cancer that associates with inefficient treatment. With advancements in genomic, transcriptomic, and epigenomic studies, important insights into the biology of the disease have been obtained. Thus, precise diagnosis and therapy for HCC is an important medical requirement, and the construction of novel prognostic signatures is urgently needed.
Pyroptosis is a type of apoptosis that arises when pathogens invade cells, triggering the body’s inflammatory response (7,8). In 2001, Cookson and Brennan first proposed the term “pyroptosis” to describe pro-inflammatory programmed cell death (PCD) (22). Subsequent studies have shown the differences, characteristics, and mechanisms of pyroptosis and apoptosis (23,24). Both types of PCDs are activated in the same way in the early stages of PCD. GSDMD was identified as having a crucial role in the activation of pyroptosis (25), and the gasdermins (GSDM) family is increasingly being explored in pyroptosis and cancer (26). The recent explosion in knowledge has demonstrated the importance of pyroptosis in pro- or anti-tumor functions, as well as in sensitization or resistance to oncological treatments (27). As a result, accumulating evidence indicates that the tumor immune microenvironment (TME) plays a multifaceted role in cancer progression. In addition to the expression of pyroptosis genes in HCC patients, which we used to establish the predictive signature, we evaluated both high- and low-risk groups, immune cells, and immune-related pathways. Additionally, our study indicates that pyroptosis in combination with immunotherapy may be an option for patients with a poor prognosis.
The expression of 33 PRGs in HCC and normal tissues were examined in this research. Patients were classified into two categories based on consensus clustering analysis. Interestingly, based on the PRGs, the two clusters could distinguish differences in clinical characteristics. By considering the relationship between patient heterogeneity and expression of pyroptosis genes that affected prognosis, we built a reliable signature. To further investigate the predictive values of these pyroptosis-related regulators, we designed a five-gene risk signature utilizing univariate Cox and LASSO Cox regression analysis, which was subsequently confirmed in a suitable external dataset.
Five genes were constructed for our signature (CASP8, GSDMC, NLRP6, NOD2, and PLCG1). CASP8, a cysteine-aspartate protease, is the initiator caspase of extrinsic apoptosis. The CASP8 level is associated with poor prognosis and metastatic development of HCC (28). Furthermore, CASP8 activation occurs in response to death receptor stimulation, which activates downstream components of another caspase family effector, amplifying the apoptotic signal potency (29). In a murine model study, it was reported that silencing of CASP8 can inhibit the development of early HCC development (30), indicating that it may become a promising diagnostic marker for HCC. GSDMC belongs to the GSDM family. It plays roles in a variety of biological processes, most notably pyroptosis, as well as the development and progression of various types of tumors. GSDMC mRNA expression was significantly increased in HCC tumor tissue compared with normal tissue, which was found to be linked with tumor grade (31). GSDMC silencing significantly diminishes colorectal cancer cell proliferation, which suggested its role as a carcinogenic gene (32). On the other hand, NLRP6 is a member of the NLR family, which detects pathogens in the cytoplasm as well as cell damage-associated factors (33). According to Chen et al., NLRP6 prevents aberrant inflammation and colon tumor development (34). Subsequently, a study found NLRP6 is a negative regulatory factor in the development of intestinal tumors (35). Moreover, several studies have reported that NLRP6 negatively correlates with tumor development, which is consistent with the coefficients in our model (36,37). In HCC samples, NOD2, a pattern recognition receptor, was upregulated and activated, and high expression of NOD2 has been linked with a poor prognosis in patients with HCC (38). Furthermore, a previous study has shown that NOD2 inhibitors have anticancer activity in vivo (39). PLCG1 is widely expressed in various tissues in humans. According to Tang et al., overexpression of PLCG1 in liver cancer cells and clinical HCC tissues was associated with poor clinical characteristics in HCC patients (40). As a result, we verified the reliability of the risk scores using the relative expression of signature genes in HCC cells and normal liver cells.
The results of immune cell infiltrations and pathways activation revealed that the risk scores might be an effective tool for immunotherapy. All of them were significantly different between the low- and high-risk groups. Additionally, we discovered that there were significantly higher quantities of immune cells entering the body and immunological-related pathways in the high-risk group, suggesting that robust active immune cell infiltration leads to a poor response to immunotherapy in individuals with higher scores. However, we only considered the whole microenvironment. In the future, we will explore the prognostic risks associated with a certain gene and TME changes in the model. Consequently, our model has advantages in promoting treatment from a new immune perspective that involves pyroptosis.
In summary, our research established a strong correlation between pyroptosis and the clinical risk of HCC patients. We identified differentially expressed pyroptosis genes between normal and HCC tissues, constructed a predictive model, and explored differences in immune infiltration. In addition, in vitro experiments, we verified the correlation of five hub genes in the model. However, the absence of in vivo experiments led to limitations in our study. This will prompt us to dig deeper into the clinical significance of the pyroptosis genes.
Acknowledgments
Funding: This study was supported by the Technology Research Program of Chongqing Municipal Education Commission (No. KJZD-K201802701 to Dilong Chen).
Footnote
Reporting Checklist: The authors have completed the TRIPOD reporting checklist. Available at https://tcr.amegroups.com/article/view/10.21037/tcr-21-2898/rc
Conflicts of Interest: All authors have completed the ICMJE uniform disclosure form (available at https://tcr.amegroups.com/article/view/10.21037/tcr-21-2898/coif). The authors have no conflicts of interest to declare.
Ethical Statement: The authors are accountable for all aspects of the work in ensuring that questions related to the accuracy or integrity of any part of the work are appropriately investigated and resolved. The study was conducted in accordance with the Declaration of Helsinki (as revised in 2013).
Open Access Statement: This is an Open Access article distributed in accordance with the Creative Commons Attribution-NonCommercial-NoDerivs 4.0 International License (CC BY-NC-ND 4.0), which permits the non-commercial replication and distribution of the article with the strict proviso that no changes or edits are made and the original work is properly cited (including links to both the formal publication through the relevant DOI and the license). See: https://creativecommons.org/licenses/by-nc-nd/4.0/.
References
- Siegel RL, Miller KD, Fuchs HE, et al. Cancer Statistics, 2021. CA Cancer J Clin 2021;71:7-33. Erratum in: CA Cancer J Clin 2021 Jul;71(4):359. [Crossref] [PubMed]
- Llovet JM, Kelley RK, Villanueva A, et al. Hepatocellular carcinoma. Nat Rev Dis Primers 2021;7:6. [Crossref] [PubMed]
- Villanueva A. Hepatocellular Carcinoma. N Engl J Med 2019;380:1450-62. [Crossref] [PubMed]
- Marrero JA, Kulik LM, Sirlin CB, et al. Diagnosis, Staging, and Management of Hepatocellular Carcinoma: 2018 Practice Guidance by the American Association for the Study of Liver Diseases. Hepatology 2018;68:723-50. [Crossref] [PubMed]
- Schulze K, Nault JC, Villanueva A. Genetic profiling of hepatocellular carcinoma using next-generation sequencing. J Hepatol 2016;65:1031-42. [Crossref] [PubMed]
- Forner A, Reig M, Bruix J. Hepatocellular carcinoma. Lancet 2018;391:1301-14. [Crossref] [PubMed]
- Fang Y, Tian S, Pan Y, et al. Pyroptosis: A new frontier in cancer. Biomed Pharmacother 2020;121:109595. [Crossref] [PubMed]
- Xia X, Wang X, Cheng Z, et al. The role of pyroptosis in cancer: pro-cancer or pro-"host"? Cell Death Dis 2019;10:650. [Crossref] [PubMed]
- Chu Q, Jiang Y, Zhang W, et al. Pyroptosis is involved in the pathogenesis of human hepatocellular carcinoma. Oncotarget 2016;7:84658-65. [Crossref] [PubMed]
- Dagogo-Jack I, Shaw AT. Tumour heterogeneity and resistance to cancer therapies. Nat Rev Clin Oncol 2018;15:81-94. [Crossref] [PubMed]
- Harding JJ, Nandakumar S, Armenia J, et al. Prospective Genotyping of Hepatocellular Carcinoma: Clinical Implications of Next-Generation Sequencing for Matching Patients to Targeted and Immune Therapies. Clin Cancer Res 2019;25:2116-26. [Crossref] [PubMed]
- Ye Y, Dai Q, Qi H. A novel defined pyroptosis-related gene signature for predicting the prognosis of ovarian cancer. Cell Death Discov 2021;7:71. [Crossref] [PubMed]
- Wu P, Shi J, Sun W, et al. Identification and validation of a pyroptosis-related prognostic signature for thyroid cancer. Cancer Cell Int 2021;21:523. [Crossref] [PubMed]
- Liu S, Shao R, Bu X, et al. Identification of the Pyroptosis-Related Gene Signature for Overall Survival Prediction in Patients With Hepatocellular Carcinoma. Front Cell Dev Biol 2021;9:742994. [Crossref] [PubMed]
- Chen Z, Zou Y, Zhang Y, et al. A Pyroptosis-Based Prognostic Model for Immune Microenvironment Estimation of Hepatocellular Carcinoma. Dis Markers 2022;2022:8109771. [Crossref] [PubMed]
- Karki R, Kanneganti TD. Diverging inflammasome signals in tumorigenesis and potential targeting. Nat Rev Cancer 2019;19:197-214. [Crossref] [PubMed]
- Wang B, Yin Q. AIM2 inflammasome activation and regulation: A structural perspective. J Struct Biol 2017;200:279-82. [Crossref] [PubMed]
- Man SM, Kanneganti TD. Regulation of inflammasome activation. Immunol Rev 2015;265:6-21. [Crossref] [PubMed]
- Wang G, Wang Q, Liang N, et al. Oncogenic driver genes and tumor microenvironment determine the type of liver cancer. Cell Death Dis 2020;11:313. [Crossref] [PubMed]
- Xue R, Chen L, Zhang C, et al. Genomic and Transcriptomic Profiling of Combined Hepatocellular and Intrahepatic Cholangiocarcinoma Reveals Distinct Molecular Subtypes. Cancer Cell 2019;35:932-947.e8. [Crossref] [PubMed]
- Llovet JM, Montal R, Sia D, et al. Molecular therapies and precision medicine for hepatocellular carcinoma. Nat Rev Clin Oncol 2018;15:599-616. [Crossref] [PubMed]
- Cookson BT, Brennan MA. Pro-inflammatory programmed cell death. Trends Microbiol 2001;9:113-4. [Crossref] [PubMed]
- Tsuchiya K. Switching from Apoptosis to Pyroptosis: Gasdermin-Elicited Inflammation and Antitumor Immunity. Int J Mol Sci 2021;22:426. [Crossref] [PubMed]
- Bertheloot D, Latz E, Franklin BS. Necroptosis, pyroptosis and apoptosis: an intricate game of cell death. Cell Mol Immunol 2021;18:1106-21. [Crossref] [PubMed]
- Shi J, Zhao Y, Wang K, et al. Cleavage of GSDMD by inflammatory caspases determines pyroptotic cell death. Nature 2015;526:660-5. [Crossref] [PubMed]
- De Schutter E, Roelandt R, Riquet FB, et al. Punching Holes in Cellular Membranes: Biology and Evolution of Gasdermins. Trends Cell Biol 2021;31:500-13. [Crossref] [PubMed]
- Sarrió D, Martínez-Val J, Molina-Crespo Á, et al. The multifaceted roles of gasdermins in cancer biology and oncologic therapies. Biochim Biophys Acta Rev Cancer 2021;1876:188635. [Crossref] [PubMed]
- Mandal R, Barrón JC, Kostova I, et al. Caspase-8: The double-edged sword. Biochim Biophys Acta Rev Cancer 2020;1873:188357. [Crossref] [PubMed]
- Salvesen GS, Abrams JM. Caspase activation - stepping on the gas or releasing the brakes? Lessons from humans and flies. Oncogene 2004;23:2774-84. [Crossref] [PubMed]
- Liedtke C, Zschemisch NH, Cohrs A, et al. Silencing of caspase-8 in murine hepatocellular carcinomas is mediated via methylation of an essential promoter element. Gastroenterology 2005;129:1602-15. [Crossref] [PubMed]
- Hu K, Xu Z, Yao L, et al. Integrated analysis of expression, prognostic value and immune infiltration of GSDMs in hepatocellular carcinoma. Aging (Albany NY) 2021;13:24117-35. [Crossref] [PubMed]
- Miguchi M, Hinoi T, Shimomura M, et al. Gasdermin C Is Upregulated by Inactivation of Transforming Growth Factor β Receptor Type II in the Presence of Mutated Apc, Promoting Colorectal Cancer Proliferation. PLoS One 2016;11:e0166422. [Crossref] [PubMed]
- Ghimire L, Paudel S, Jin L, et al. The NLRP6 inflammasome in health and disease. Mucosal Immunol 2020;13:388-98. [Crossref] [PubMed]
- Chen GY, Liu M, Wang F, et al. A functional role for Nlrp6 in intestinal inflammation and tumorigenesis. J Immunol 2011;186:7187-94. [Crossref] [PubMed]
- Normand S, Delanoye-Crespin A, Bressenot A, et al. Nod-like receptor pyrin domain-containing protein 6 (NLRP6) controls epithelial self-renewal and colorectal carcinogenesis upon injury. Proc Natl Acad Sci U S A 2011;108:9601-6. [Crossref] [PubMed]
- Domblides C, Soubeyran I, Lartigue L, et al. Prognostic Role of Inflammasome Components in Human Colorectal Cancer. Cancers (Basel) 2020;12:3500. [Crossref] [PubMed]
- Wang Q, Wang C, Chen J. NLRP6, decreased in gastric cancer, suppresses tumorigenicity of gastric cancer cells. Cancer Manag Res 2018;10:6431-44. [Crossref] [PubMed]
- Zhou Y, Hu L, Tang W, et al. Hepatic NOD2 promotes hepatocarcinogenesis via a RIP2-mediated proinflammatory response and a novel nuclear autophagy-mediated DNA damage mechanism. J Hematol Oncol 2021;14:9. [Crossref] [PubMed]
- Dong Y, Wang S, Wang C, et al. Antagonizing NOD2 Signaling with Conjugates of Paclitaxel and Muramyl Dipeptide Derivatives Sensitizes Paclitaxel Therapy and Significantly Prevents Tumor Metastasis. J Med Chem 2017;60:1219-24. [Crossref] [PubMed]
- Tang W, Zhou Y, Sun D, et al. Oncogenic role of phospholipase C-γ1 in progression of hepatocellular carcinoma. Hepatol Res 2019;49:559-69. [Crossref] [PubMed]