Identification of a pyroptosis related gene signature for predicting prognosis and estimating tumor immune microenvironment in bladder cancer
Introduction
Bladder cancer (BLCA) is the ninth most common malignant tumor globally, with an evaluated 430,000 newly diagnosed patients and 165,000 deaths per year (1). Approximately 70% of BLCA are first suffered with non-muscle invasive bladder cancer (nMIBC), whose recurrence may progress to muscle-invasive bladder cancer (MIBC), while some 25% of BLCA cases are first diagnosed as MIBC, which is prone to early metastasis with inferior prognosis (2). So far, the clinical prognosis of BLCA patients mainly relied on pathologic grade and Tumor Node Metastasis (TNM) clinical stage system. Although the TNM clinical stage system has an essential role in predicting prognosis and guiding treatment, it is not sufficient to assess the clinical prognosis of BLCA patients, meeting the clinical demands (3). Thus, identifying novel reliable prognostic markers and therapeutic targets that could further guide the surgeon to optimize the clinical treatment is of utmost importance.
Pyroptosis is a newly identified form of programmed cell death (PCD), mainly mediated by Gasdermin protein family. Unlike apoptosis and autophagy, pyroptosis is induced by several pathological stimulations from inside or outside, accompanied by immune response and the release of inflammatory factors (4). Recently, numerous studies have suggested that pyroptosis might have a pivotal role in cancer development and progression (5,6). As an essential process of pyroptosis, Gasdermin D (GSDMD) protein could be cleaved by CASP-1 when the cell is exposed to several stimulations (7). In their recent study, Gao et al. (8) suggested that a higher expression level of GSDMD protein is associated with more invasive malignant tumor features, including lower survival rate and larger tumor size in lung cancer. Mechanistically, the silence of GSDMD expression in non-small cell lung cancer (NSCLC) cells leads to decreased activity of the estimated glomerular filtration rate (EGFR) signaling pathway, which in turn leads to the inhibition of tumor proliferation in vivo. Oppositely, Wang proved that the expression of GSDMD protein was negatively related to the tumor formation and proliferation in gastric cancer (9). More importantly, unlike GSDMD, GSDME-mediated pyroptosis was associated with the sensitivity of chemotherapeutic drugs and tumor immunity microenvironment (10). Together, the process of inducing pyroptosis inside the tumor might be considered as a potential therapeutic strategy for tumor treatment. However, the exact function of pyroptosis in BLCA remains unclear.
In the current research, we aimed to explore the correlation of pyroptosis-related genes (PRGs) with clinical prognostic and TME in BLCA, indicating that pyroptosis might be considered as a potential target for clinical prognostic prediction and effect of immunotherapy assessment. We present the following article in accordance with the TRIPOD reporting checklist (available at https://tcr.amegroups.com/article/view/10.21037/tcr-22-177/rc).
Methods
Data download and processing
Transcriptome profiling data harmonized to the fragments per kilobase million (FPKM) and the corresponding clinical information on BLCA sample were obtain from the official The Cancer Genome Atlas (TCGA) on 28 August 2021 (https://portal.gdc.cancer.gov/repository). A total of 19 adjacent normal tissues and 411 bladder tumor tissues were examined in the current study. We identified 39 PRGs according to the previous research, which is shown in Table S1 (11-13). The expression data of BLCA samples were normalized before comparison. The “limma” package of R language was conducted to identify the difference expression of PRGs between BLCA tumors and adjacent normal tissues. In addition, the “igraph” package of R language was used to visualize the correlation network of different expression PRGs.
Identification and validation of the pyroptosis signature
First, 70% of BLCA patients were classified as training cohort to establish a clinical prognostic risk score signature based on the PRGs, and the other 30% of cases were set as the testing cohort, which was conducted to evaluate the risk score model’s predictive ability. Furthermore, univariate Cox regression analysis for R’s “survival” package was employed to filter the PRGs, which were statistically correlated with the patients’ survival status. Moreover, LASSO Cox regression was employed to establish the pyroptosis risk model. Finally, 6 PRGs and their coefficients were obtained to determine the prediction signature. The risk scores of each BLCA samples were calculated by the formula: (X: coefficients, Y: gene expression). Next, the Kaplan-Meier survival analysis was employed to examine the survival outcome difference using the “survival” R language package. In addition, the correlation between risk score and clinical characters of BLCA was evaluated by univariate COX regression and multivariate Cox regression through the R package “survival”. The clinical features included the age at diagnosis, patients’ gender, tumor grade, and clinical stage.
Functional enrichment analyses of differentially expressed genes (DEGs)
The DEGs between the two subgroups with high- and low-risk in the BLCA entire cohort were screened according to specific criteria [|log2FC| ≥0.585 and false discovery rate (FDR) <0.05]. R language “clusterProfiler” package was used to perform Kyoto Encyclopedia of Genes and Genomes (KEGG) as well as Gene Ontology (GO) enrichment analyses. Besides, gene set enrichment analysis (GSEA) (C2:CP:KEGG subset) was also employed to explore the signal pathways that statistically changed between high- and low-risk subgroups.
Tumor mutational burden (TMB) analysis
TMB measures the number of mutations per megabyte in cancer tissue, which could be conducted to estimate the effect of anti-tumor immunotherapy (14). To investigate the correlation between TMB and risk score, the somatic mutation data of BLCA was obtained from the TCGA and analyzed using “maftools” package of R language.
Immune cell infiltration analysis
The estimate score, immune score, stromal score and tumor purity of each BLCA cases were measured by “estimate” package of R language to identify the immune characteristics of BLCA patients in different risk score subgroups. CIBERSORT.R was conducted to examine the infiltration level of immune cells using the “CIBERSORT” R package. Besides, the correlation of PRGs risk signature with 22 types of immune cells infiltration was also performed.
Statistical analysis
All the bioinformatics analyses were conducted using the R language software (version 4.1.0 for Mac). Wilcoxon test was employed for the two groups comparison, while Kruskal-Wallis test was employed for more than two groups comparison. In addition, Spearman correlation test was employed to determine the correlation of PRGs risk score and certain variables. The receiver operating characteristic (ROC) curve was subsequently performed to assess the predictive capacity of PRGs signature. Univariate Cox regression and multivariate Cox regression models were used to assess the association between patients’ overall survival and PRGs risk score. A P value <0.05 was considered statistically significant.
Ethical statement
The study was conducted in accordance with the Declaration of Helsinki (as revised in 2013).
Results
Different expression of PRGs in adjacent normal and tumor tissues
First, the different expression of 39 PRGs was compared between cancer and adjacent normal tissues. The obtained results revealed that the expression level of 19 PRGs were statistically different (P<0.05, Figure 1A). Among them, 15 genes (HMGB1, GPX4, PLCG1, GSDMD, CASP8, CASP3, BAK1, PYCARD, BAX, GSDMB, CASP6, NLRP2, AIM2, CASP5, and NLRP7) were up-regulated and 4 genes (ELANE, IL6, NLRP3, and NLRP1) were down-regulated in tumor tissues (Figure 1B). To further investigate the interactions of the PRGs, the correlation network containing all the 19 different expressed PRGs is presented in Figure 1C.
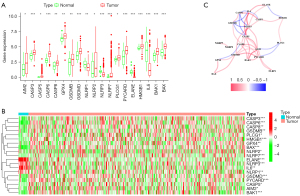
Establishment of PRGs risk model in training cohort
The total TCGA BLCA cases were randomly divided into training (70%)/validation (30%) cohort. The clinical data of BLCA patients in the training cohort were subsequently used to establish the prognostic risk score model. Univariate Cox regression was first conducted to investigate the correlation between PRGs expression and overall survival for BLCA samples. Among 39 PRGs, AIM2, CASP6, CASP8, CASP9, GSDMB, GZMA, GZMB, and IRF1 were filtered to have significant association with overall survival of BLCA patients, and all these genes could be considered as protecting factors (P<0.05, Figure 2A). Furthermore, the LASSO Cox regression analysis was performed, and a total of 6 PRGs were ultimately conducted to establish the clinical prognostic risk model using the formula: risk score = (AIM2* −0.04245) + (CASP6* −0.21147) + (CASP8* −0.05678) + (CASP9* −0.32890) + (GSDMB* −0.26059) + (GZMA* −0.13959) (Figure 2B, Table 1). Based on the median value of PRGs risk score calculated by the formula for every BLCA sample, the patients in TCGA training cohort were grouped into high- and low-risk subgroups. As shown in Figure 2C, the high-risk subgroup exhibited statistically more deaths and poorer overall survival than patients in low-risk score group (P<0.001, Figure 2D). The principal component analysis (PCA) plot showed that patients with different risk scores were well separated into two clusters (Figure 2E). In addition, as illustrated in Figure 2F, areas under the ROC curve (AUCs) of the PRGs risk score for 1, 3, and 5 years were 0.705, 0.681, and 0.698, respectively. Moreover, univariate Cox regression analysis demonstrated that age at the diagnosis, tumor stage, and PRGs risk score were potential factors impacting the survival outcome of BLCA patients in the training cohort (Figure 2G), while multivariate Cox regression analysis proved that the PRGs risk signature was an independent factor affecting the clinical outcomes of BLCA in training cohorts (HR =2.734, 95% CI: 1.807–4.137, while patient’s age was lack of statistical significance in multivariate analysis (P=0.112) Figure 2H.
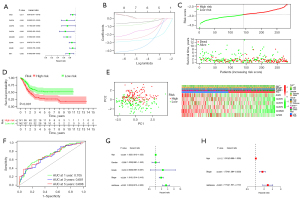
Table 1
i | Gene | Coef |
---|---|---|
1 | AIM2 | −0.04245 |
2 | CASP6 | −0.21147 |
3 | CASP8 | −0.05678 |
4 | CASP9 | −0.32890 |
5 | GSDMB | −0.26059 |
6 | GZMA | −0.13959 |
Validation of PRGs risk model in the testing and entire cohort
Furthermore, the PRGs risk score model was also validated in testing as well as the entire cohort. The BLCA samples were also grouped into low- and high-risk score subgroups in testing as well as the entire cohort based on the median valve of PRGs risk score in training cohort. Consisted with the training cohort results, the Kaplan-Meier (K-M) survival curves revealed a significant difference in survival outcome between the high- and low-risk subgroup in the testing (P<0.001, Figure 3A). In addition, as illustrated in Figure 3B, the high-risk score group had more patient deaths compared with the low-risk score group in the testing and entire cohort. In addition, ROC analysis revealed that AUC’s for 1, 3, and 5 years were 0.693, 0.686, 0.706 in testing group (Figure 3C). PCA plot showed that patients with different risk scores were well separated into two clusters (Figure 3D). Moreover, univariate and multivariate Cox regression analysis proved that the PRGs risk signature was an independent factor affecting the clinical outcomes of BLCA in testing and entire cohort respectively (P<0.05, Figure 3E,3F). The performance of the risk model in entire cohort was consisted with testing cohort (Figure 4A-4F).
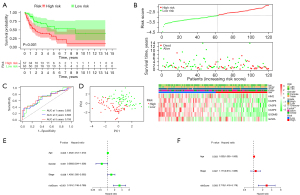
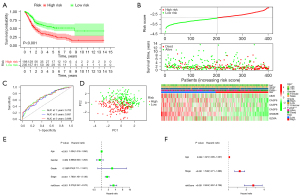
Correlations of risk signature with BLCA patients’ clinical characteristics
Furthermore, we also investigate the association between clinical characteristics, including age at diagnosis, gender, grade, clinical stage, T as well as N stage and the risk score. As a result, there was a significant correlation between high risk score and increasing age, higher clinical stage, T stage and N stage. Additionally, no statistical significance was observed between patient’s gender and tumor grade (Figure 5A-5F).
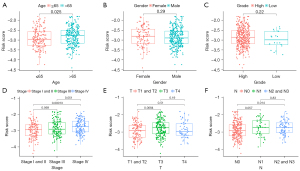
Functional analyses of DEGs in the subgroup with different risk score
To further investigate the differences of biological processes and signal pathways between the two subgroups with different risk score, GO, KEGG, and GSEA function enrichment analyses were employed. As a result, the DEGs filtered between low- and high-subgroup were mainly enriched in “Collagen-Containing Extracellular Matrix”, “Extracellular Structure Organization”, “Extracellular Matrix Organization”, “Skin Development”, “External Encapsulating Structure Organization”, etc. (P<0.05; Figure 6A). In addition, KEGG analyses results revealed that the DEGs filtered between low- and high-subgroup were highly enriched in “Phagosome”, “Focal adhesion”, “Epstein-Barr virus infection”, “Antigen processing and presentation”, “Protein digestion and absorption”, etc. (P<0.05; Figure 6B). In addition, as shown in GSEA results, the gene sets of low-risk subgroup were enriched in “Rig I Like Receptor Signaling Pathway”, and “Peroxisome”, while gene sets of high-risk subgroup were enriched in “Ecm Receptor Interaction”, “Glycosaminoglycan Biosynthesis Chondroitin Sulfate”, “Arrhythmogenic Right Ventricular Cardiomyopathy Arvc”, “Melanoma”, “Focal Adhesion” and “Dilated Cardiomyopathy” (Figure 6C).
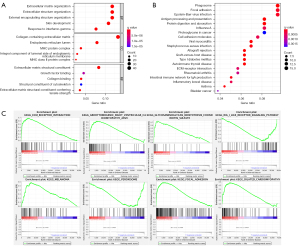
Correlations of risk signature with TMB in BLCA patients
As a well-known evaluation marker of tumor immunotherapy efficacy, we also investigated the association between the PRGs risk score and TMB in BLCA tissues. As a result, the top 10 highest mutation frequencies were TP53, TTN, KMT2D, MUC16, ARID1A, KDM6A, PIK3CA, SYNE1, RB1 and FGFR3 (Figure 7A,7B). In addition, TMB was found to be significantly lower in high-risk group compared with low-risk group (P<0.001, Figure 7C). Moreover, the PRGs risk score was also negatively associated with TMB in BLCA tissues (r=−0.18, P<0.001, Figure 7D).
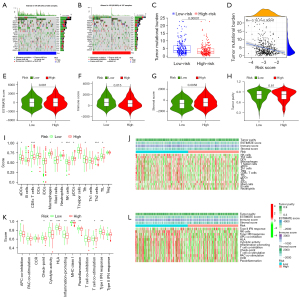
Correlations of risk signature with tumor microenvironment (TME) and immune activity in BLCA tissues
In the current study, we also identified whether the TME was associated with the score risk. Our results revealed that the low-risk subgroup exhibited a significantly lower stroma score (P=0.0058) and higher immune score (P=0.015) compared with the high-risk subgroup, while there was no statistically difference in tumor purity and estimate scores in the subgroups with different risk score in BLCA cohort (P>0.05, Figure 7E-7H).
In addition, single sample gene set enrichment analysis (ssGSEA) was also employed to identify the enrichment scores of immune cell and immune related function between the two subgroups with different risks in the entire cohort. As a result, the enrichment scores of most immune cell [including activated dendritic cell (aDC), CD8+ T cell, neutrophil, natural killer (NK) cell, plasmacytoid dendritic cells (pDCs), T follicular helper (Tfh), T helper 2 (Th2) cell, and tumor-infiltrating lymphocyte (TIL)] in low-risk score subgroup were significantly higher than in the high-risk score subgroup (P<0.05, Figure 7I,7J). Moreover, with reference to the score of the immune function analysis, besides antigen presenting cell (APC) stimulation, cytokine-cytokine receptor (CCR), type II interferon (IFN) response, and T cell co-inhibition, the other ssGSEA scores of immune function were statistically higher in the low-risk score subgroup than high-risk score subgroup, implying the immune functions related to pyroptosis might be more active in low-risk score subgroup (Figure 7K,7L).
Correlations of risk signature and Immune cell infiltration in BLCA patients
CIBERSORT was subsequently employed to explore the whether the infiltration level of 22 immune cell was associated with the score risk (Figure 8A). The obtained results indicated that the infiltration levels of CD8 T cells, follicular helper T cells and activated CD4 T cells in the high-risk score subgroup were significantly low than in the high-risk score subgroup (P<0.05, Figure 8B), while the levels of macrophages M2 and M0, as well as mast cells activated in a high-risk score subgroup, were statistically higher than that in the high-risk score subgroup (P<0.05, Figure 8B).
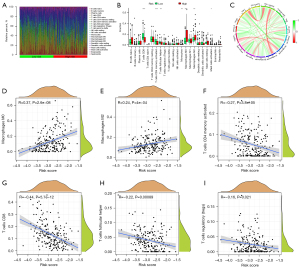
Meanwhile, the correlation between infiltration level of immune cells and the PRGs risk score was also analyzed. As a result, the infiltration proportion of the macrophages M0 and M2 cells were positively associated with the risk score, while the levels of activated CD4 T cells, T cells regulatory (Tregs), CD8 T cells, and follicular helper T cells, had a negative associated with PRGs risk score (Figure 8C-8I). In addition, among these immune cell types, high infiltration levels of B cells memory, macrophages M0, macrophages M2, mast resting cells, and neutrophils were significantly associated with poor survival outcomes, while increased infiltration levels T cells follicular helper, T cells CD8, activated CD4 T cell, and plasma cells were related to better OS (all P<0.05, Figure 9). Based on the PRGs prognostic model, these results suggested that the high-risk score group has less CD8 and CD4 T cells infiltration and more anti-inflammatory macrophages M2 cells than the low-risk score group, which led to a worse tumor clinical prognosis.
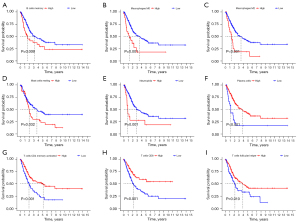
Discussion
Growing evidence has indicated that pyroptosis play a critical role in the development of many diseases. Of particular importance is its dual role in tumor formation and microenvironment (15). On one hand, the various pre-inflammatory mediators released during pyroptosis process are related to tumor formation and progression. On the other hand, pyroptosis could also suppress the incidence and progression of tumors (16). Thus, pyroptosis may become a potential therapy for drug-resistant cancers in the future (17). However, the exact function of pyroptosis in the development and microenvironment of BLCA remains unclear.
In the current study, we first explored the different expression of PRGs between adjacent normal and BLCA tissues, finding that the expression level 19 PRGs was statistically different between BLCA and adjacent normal tissues in the TCGA BLCA cohort. Except for ELANE, IL6, NLRP3, and NLRP1, the other differently expressed PRGs were up-regulated in BLCA tissues, thus indicating that the occurrence of pyroptosis might be more prevalent in BLCA tissues. One possible explanation is that the inflammatory mediators released during the pyroptosis process might promote tumor formation, leading to the high expression of PRGs (18). However, whether the number of inflammatory mediators released by pyroptosis activation could induce the occurrence of cancer needs to be further studied. Since pyroptosis might have an opposite role in promoting and suppressing tumor progression in different tissues and genetic backgrounds, we further investigated whether the expression level of PRGs was correlated with the OS of the BLCA patients. Univariate Cox regression analyses revealed that several PRGs were related to the clinical prognosis of BLCA patients. Interestingly, the high expression levels of these PRGs predicted a good prognosis, thus suggesting that the PRGs could be considered as protective factors in BLCA patients. These results were also consistent with recent studies on the other different types of cancer (11,19).
Furthermore, a prognostic risk score model based on 6 PRGs (AIM2, CASP6, CASP8, CASP9, GSDMB, and GZMA) was moderately performed in prognostic predictions of BLCA patients. Survival analysis indicated that BLCA samples with low-risk scores were related to a better prognosis compared with high-risk patients. Moreover, multivariable Cox regression analysis shown that the PRGs risk score could result as an independent factor affecting the overall survival of BLCA patients in training, testing, as well as total cohorts. Furthermore, our results also proved that the PRGs risk score was correlated with the following clinical features of BLCA patients: age, clinical stage, and TNM status. These results further confirmed that pyroptosis could inhibit tumor progression by promoting cell death, thus acting as a protective factor in BLCA patients.
Pyroptosis, also called GSDM mediated PCD, was first discovered in epithelial tissues (20). As a major part of the GSDM family, GSDMB protein can be cleaved by CASP-1, leading to cell pyroptosis by the release of the N-terminal domain (21). Several studies have shown that GSDMB could promote pyroptosis and suppress the growth of tumor cells (7,22). Consistent with the previous study (7), our results indicated high expression level of GSDMB predicted a good clinical prognosis in BLCA patients. However, the precise function of GSDMB in cancer is still controversial. A recent study reported that the expression level of GSDMB protein was down-regulated in normal tissue compared with BLCA tissues, while overexpressed GSDMB facilitated tumor progression by interacting with STAT3 and enhancing the glycolysis of BLCA cells (23). In addition, the clinical outcomes of HER2-positive breast cancer with high expression level of GSDMB were found to significantly predict a poor prognosis, accompanied by trastuzumab resistance (24). AIM2, which is known as a tumor suppressor, has been found in many types of malignant tumors, including gastric, endometrial, and colon cancers, due to its inactivation (25). In their recent study, Kumari proved that AIM2 activates CASP-1 through apoptosis-associated speck-like protein containing a CARD (ASC) mediated junctional proteins to promote tumor cell pyroptosis, accompanied by the release of pre-inflammatory mediators such as IL-1β and IL-18 (26). CASP-6 has been proven to regulate inflammation activation and promote GSDMD-induced cell pyroptosis (27). Except for pyroptosis, CASP-6 also has a critical role in promoting apoptosis and necroptosis (28). In general, our results proved that the PRGs in the risk model acted as a cancer suppressor, and due to high expression levels of these genes predicted a good prognosis of BLCA patients. Yet, the molecular mechanism of these genes needs to be further studied.
The cellular components of TMEs include endothelial cells, stromal cells, tumor cells, and immune cells. Accumulating evidence indicated that inflammatory cytokines released during pyroptosis process, including ATP, HMGB1, IL-1β, and IL-18, could exert an important influence on the TME (29). A recent study conducted by Zhang proved that malignant tumors with the high expression level of GSDME exhibited increased infiltration level of immune cells, including NK cells and CD8+ T cells, whereas GSDME-deficient malignant tumors exhibited reduced infiltration of immune cell (30). Consistent with these previous studies, by analyzing the immune cell and immune pathways in TME, our results proved that the activation of pyroptosis increased the infiltration level of CD4+ and CD8+ T cells, whereas it reduced M0 and M2 cells populations, which have been indicated to suppress anti-tumor immunity system and to be related to poor prognosis in previous studies (31).
However, there are several limitations in our study. First, the data in the study was obtained from public databases. Second, the definitive functions of PRGs in BLCA require further experiments. In addition, the performance of the PRGs model was not verified in another independent cohort.
Taken together, our study revealed that pyroptosis was closely related to BLCA as most of the PRGs were highly expressed in tumor tissues. Moreover, a novel prognostic model based on 6 PRGs was constructed, and the PRGs signature considered as an independent risk factor for predicting BLCA prognosis. In addition, the PRGs risk score was also associated with the level of anti-tumor infiltrating immune cells; thus, pyroptosis might be regarded as a novel strategy in cancer diagnosis and therapy. However, future studies need to investigate further the adverse and beneficial influence of pyroptosis on malignant tumors.
Acknowledgments
We are grateful to the TCGA for providing all the data.
Funding: This work was supported by funds from the National Science Foundation of Beijing (Nos. 7192053, 7172068).
Footnote
Reporting Checklist: The authors have completed the TRIPOD reporting checklist. Available at https://tcr.amegroups.com/article/view/10.21037/tcr-22-177/rc
Peer Review File: Available at https://tcr.amegroups.com/article/view/10.21037/tcr-22-177/prf
Conflicts of Interest: All authors have completed the ICMJE uniform disclosure form (available at https://tcr.amegroups.com/article/view/10.21037/tcr-22-177/coif). The authors have no conflicts of interest to declare.
Ethical Statement: The authors are accountable for all aspects of the work in ensuring that questions related to the accuracy or integrity of any part of the work are appropriately investigated and resolved. The study was conducted in accordance with the Declaration of Helsinki (as revised in 2013).
Open Access Statement: This is an Open Access article distributed in accordance with the Creative Commons Attribution-NonCommercial-NoDerivs 4.0 International License (CC BY-NC-ND 4.0), which permits the non-commercial replication and distribution of the article with the strict proviso that no changes or edits are made and the original work is properly cited (including links to both the formal publication through the relevant DOI and the license). See: https://creativecommons.org/licenses/by-nc-nd/4.0/.
References
- Antoni S, Ferlay J, Soerjomataram I, et al. Bladder Cancer Incidence and Mortality: A Global Overview and Recent Trends. Eur Urol 2017;71:96-108. [Crossref] [PubMed]
- Farling KB. Bladder cancer: Risk factors, diagnosis, and management. Nurse Pract 2017;42:26-33. [Crossref] [PubMed]
- Jordan B, Meeks JJ. T1 bladder cancer: current considerations for diagnosis and management. Nat Rev Urol 2019;16:23-34. [Crossref] [PubMed]
- Ruan J, Wang S, Wang J. Mechanism and regulation of pyroptosis-mediated in cancer cell death. Chem Biol Interact 2020;323:109052. [Crossref] [PubMed]
- Fang Y, Tian S, Pan Y, et al. Pyroptosis: A new frontier in cancer. Biomed Pharmacother 2020;121:109595. [Crossref] [PubMed]
- Zheng Z, Li G. Mechanisms and Therapeutic Regulation of Pyroptosis in Inflammatory Diseases and Cancer. Int J Mol Sci 2020;21:1456. [Crossref] [PubMed]
- Li L, Li Y, Bai Y. Role of GSDMB in Pyroptosis and Cancer. Cancer Manag Res 2020;12:3033-43. [Crossref] [PubMed]
- Gao J, Qiu X, Xi G, et al. Downregulation of GSDMD attenuates tumor proliferation via the intrinsic mitochondrial apoptotic pathway and inhibition of EGFR/Akt signaling and predicts a good prognosis in non-small cell lung cancer. Oncol Rep 2018;40:1971-84. [Crossref] [PubMed]
- Wang WJ, Chen D, Jiang MZ, et al. Downregulation of gasdermin D promotes gastric cancer proliferation by regulating cell cycle-related proteins. J Dig Dis 2018;19:74-83. [Crossref] [PubMed]
- Zhao P, Wang M, Chen M, et al. Programming cell pyroptosis with biomimetic nanoparticles for solid tumor immunotherapy. Biomaterials 2020;254:120142. [Crossref] [PubMed]
- Ye Y, Dai Q, Qi H. A novel defined pyroptosis-related gene signature for predicting the prognosis of ovarian cancer. Cell Death Discov 2021;7:71. [Crossref] [PubMed]
- Li XY, Zhang LY, Li XY, et al. A Pyroptosis-Related Gene Signature for Predicting Survival in Glioblastoma. Front Oncol 2021;11:697198. [Crossref] [PubMed]
- Shao W, Yang Z, Fu Y, et al. The Pyroptosis-Related Signature Predicts Prognosis and Indicates Immune Microenvironment Infiltration in Gastric Cancer. Front Cell Dev Biol 2021;9:676485. [Crossref] [PubMed]
- Liu L, Bai X, Wang J, et al. Combination of TMB and CNA Stratifies Prognostic and Predictive Responses to Immunotherapy Across Metastatic Cancer. Clin Cancer Res 2019;25:7413-23. [Crossref] [PubMed]
- Jia C, Chen H, Zhang J, et al. Role of pyroptosis in cardiovascular diseases. Int Immunopharmacol 2019;67:311-8. [Crossref] [PubMed]
- Nagarajan K, Soundarapandian K, Thorne RF, et al. Activation of Pyroptotic Cell Death Pathways in Cancer: An Alternative Therapeutic Approach. Transl Oncol 2019;12:925-31. [Crossref] [PubMed]
- Shen X, Wang H, Weng C, et al. Caspase 3/GSDME-dependent pyroptosis contributes to chemotherapy drug-induced nephrotoxicity. Cell Death Dis 2021;12:186. [Crossref] [PubMed]
- Xia X, Wang X, Cheng Z, et al. The role of pyroptosis in cancer: pro-cancer or pro-"host"? Cell Death Dis 2019;10:650. [Crossref] [PubMed]
- Ju A, Tang J, Chen S, et al. Pyroptosis-Related Gene Signatures Can Robustly Diagnose Skin Cutaneous Melanoma and Predict the Prognosis. Front Oncol 2021;11:709077. [Crossref] [PubMed]
- Tamura M, Tanaka S, Fujii T, et al. Members of a novel gene family, Gsdm, are expressed exclusively in the epithelium of the skin and gastrointestinal tract in a highly tissue-specific manner. Genomics 2007;89:618-29. [Crossref] [PubMed]
- Panganiban RA, Sun M, Dahlin A, et al. A functional splice variant associated with decreased asthma risk abolishes the ability of gasdermin B to induce epithelial cell pyroptosis. J Allergy Clin Immunol 2018;142:1469-1478.e2. [Crossref] [PubMed]
- Zhou Z, He H, Wang K, et al. Granzyme A from cytotoxic lymphocytes cleaves GSDMB to trigger pyroptosis in target cells. Science 2020;368:eaaz7548. [Crossref] [PubMed]
- He H, Yi L, Zhang B, et al. USP24-GSDMB complex promotes bladder cancer proliferation via activation of the STAT3 pathway. Int J Biol Sci 2021;17:2417-29. [Crossref] [PubMed]
- Hergueta-Redondo M, Sarrio D, Molina-Crespo Á, et al. Gasdermin B expression predicts poor clinical outcome in HER2-positive breast cancer. Oncotarget 2016;7:56295-308. [Crossref] [PubMed]
- Sharma BR, Karki R, Kanneganti TD. Role of AIM2 inflammasome in inflammatory diseases, cancer and infection. Eur J Immunol 2019;49:1998-2011. [Crossref] [PubMed]
- Kumari P, Russo AJ, Shivcharan S, et al. AIM2 in health and disease: Inflammasome and beyond. Immunol Rev 2020;297:83-95. [Crossref] [PubMed]
- Zheng M, Karki R, Vogel P, et al. Caspase-6 Is a Key Regulator of Innate Immunity, Inflammasome Activation, and Host Defense. Cell 2020;181:674-687.e13. [Crossref] [PubMed]
- Mengying Z, Yiyue X, Tong P, et al. Apoptosis and necroptosis of mouse hippocampal and parenchymal astrocytes, microglia and neurons caused by Angiostrongylus cantonensis infection. Parasit Vectors 2017;10:611. [Crossref] [PubMed]
- Du T, Gao J, Li P, et al. Pyroptosis, metabolism, and tumor immune microenvironment. Clin Transl Med 2021;11:e492. [Crossref] [PubMed]
- Zhang Z, Zhang Y, Xia S, et al. Gasdermin E suppresses tumour growth by activating anti-tumour immunity. Nature 2020;579:415-20. [Crossref] [PubMed]
- Wang Q, Wang Y, Ding J, et al. A bioorthogonal system reveals antitumour immune function of pyroptosis. Nature 2020;579:421-6. [Crossref] [PubMed]