Development of multivariable risk signature based on four immune-related RNA-binding proteins to predict survival and immune status in lung adenocarcinoma
Introduction
Lung cancer is the first leading cause of cancer-related deaths worldwide, accounting for almost a quarter of all cancer deaths (1). Lung cancer can be classified into two types based on histology: small cell lung cancer (SCLC) and non-small cell lung cancer (NSCLC); they are responsible for 15% and 85% of lung cancers, respectively. Lung adenocarcinoma (LUAD) accounts for >40% of lung cancers and is the most common type of NSCLC (2). In the last decade, immunotherapy has become an effective therapy for several cancers, and LUAD is an immune-sensitive cancer (3,4). Indeed, the immune status of cancer predicts the effectiveness of immunotherapy and is closely related to patients’ prognoses (5).
Studies have confirmed that several biomarkers are prognostic factors of LUAD, such as the epidermal growth factor receptor (EGFR), V-Ki-Ras2 Kirsten rat sarcoma 2 viral oncogene homolog (KRAS), and ALK receptor tyrosine kinase (ALK) (6-8). Many drugs have subsequently been developed to target driver gene mutations in these genes. Unfortunately, most patients will eventually develop resistance to targeted therapies after receiving them. For example, patients’ development of resistance to EGFR-tyrosine kinase inhibitors is part due to a secondary mutation in tumors (9). Moreover, changes at the genetic level of the tumor occur before the clinical characteristics, and patients with the same stage of cancer may have varying prognoses. Most patients are at an advanced stage when diagnosed and have thus missed the opportunity to undergo standard treatment. For these reasons, the 5-year survival rate of patients with LUAD is only 23% (10,11). Therefore, it is imperative to identify novel diagnostic and prognostic biomarkers of LUAD. Then, management schemes can be designed for distinct subsets of patients to allow for precise treatment.
RNA-binding protein (RBP) is a class of proteins that control RNA stabilization, degradation, and modification at the post-transcriptional level by selectively binding to RNA (12). So far, 1,542 RBP genes have been identified by genome-wide screening in the human genome, accounting for 7.5% of all protein-coding genes (13). Post-transcriptional regulation plays a critical role in cellular processes. Therefore, RBP dysregulation might lead to abnormal gene expression in cells and eventually result in various diseases, including cancer and immune disorders (14). For instance, by regulating the translation efficiency of Cyclin D1, CDK2, and CDK4, m6A modified RBP YTHDF1 deficiency inhibits the proliferation of NSCLC cells and the formation of xenograft tumors, and YTHDF1 decrease inhibits de novo LUAD (15). RBPs play important roles in various cancers (16-19). No study has tried to develop a risk signature for LUAD based on immune-related RBPs.
Lately, a study constructed a prognostic signature of liver cancer using immune-related RBPs and demonstrated good predictive power (20). To the best of our knowledge, no research has been reported to construct a risk signature for LUAD based on immune-related RBPs. Therefore, this study aimed to construct and validate a risk signature with predictive power based on immune-related RBPs for LUAD patients. Such a risk signature might help provide information for accurate prognosis and individualized immunotherapy of patients with LUAD. We present the following article in accordance with the TRIPOD reporting checklist (available at https://tcr.amegroups.com/article/view/10.21037/tcr-22-698/rc).
Methods
Data sources
We used public data from The Cancer Genome Atlas (TCGA) (https://portal.gdc.cancer.gov) and Gene-Expression Omnibus (GEO) (http://www.ncbi.nlm.nih.gov/geo) databases to conduct a comprehensive analysis. As a training cohort, the expression data and clinical information of LUAD patients (486 tumor samples and 54 normal samples) were downloaded from TCGA, after excluding those with unknown survival data, overall survival (OS) <30 days, or died from non-cancer causes (e.g., myocardial infarction, hemorrhage, infection, etc.). GSE68465 from GEO (including 443 LUAD patients) was used as a validation cohort (Table S1). Gerstberger et al. (13) reviewed the literature to create a census of RBPs and identified 1,542 RBPs, which were all examined in the present study. Based on the Immunology Database and Analysis Portal (immPort) database (https://immport.niaid.nih.gov), we acquired 2,498 immune genes (IGs) (21). The study was conducted in accordance with the Declaration of Helsinki (as revised in 2013).
Identification of differentially expressed immune-related RBPs
Using Wilcoxon’s test, the R package “limma” was used to identify the differentially expressed RBPs and IGs between the LUAD and normal samples. The adjusted P value <0.05 and |log2 fold-change| >0.5 were considered as the thresholds. Based on their expression in LUAD samples, Pearson correlation analysis was used to determine the RBPs related to IGs. The threshold was set to P value <0.01 and |correlation coefficient| >0.5. Genes that met the criteria were displayed in a regulatory network using the Cytoscape software. Gene Ontology (GO) (http://geneontology.org) is an important bioinformatics tool to annotate and illustrate genes and their biological process (BP), cellular component (CC), and molecular function (MF) (22). The Kyoto Encyclopedia of Genes and Genomes (KEGG) (http://www.genome.jp/kegg) is a comprehensive database resource for the biological interpretation of genome sequences (23). The analysis of GO and KEGG pathway enrichment was performed in the R package “clusterProfiler” (24).
Screening and analysis of prognostic immune-related RBPs
Univariable Cox regression analyses were used to determine the immune-related RBPs that were associated with LUAD prognosis. Using the R package “glmnet”, Lasso regression analysis was used to exclude genes with high correlation, thus reducing the complexity of the risk signature (25). Multivariable Cox regression analysis was ultimately performed to evaluate the immune-related RBPs as independent predictive indicators for LUAD (P<0.05). The final immune-related RBPs were defined as risk genes for the construction of the risk signature. A series of online databases were selected for systematic analysis of the above risk genes. First, genetic alteration conditions of risk genes in LUAD were downloaded from cBioPortal (http://www.cbioportal.org) (26). Subsequently, mRNA expression levels of risk genes in LUAD and normal tissues were compared in the UALCAN (http://ualcan.path.uab.edu/) database (27). Finally, we conducted OS analysis using The Gene Expression Profiling Interactive Analysis 2 (GEPIA2) (http://gepia2.cancer-pku.cn) database (28).
Construction and verification of prognostic risk model
The risk score was derived from the following formula: Risk score = Exp1 * B1 + Exp2 * B2 + Expi * Bi. Expi was the expression level of risk genes, and Bi was the multivariable Cox regression coefficient of risk genes. According to the median risk score, LUAD patients were divided into the low- and high-risk groups. The R package “survival” was used to compare the survival differences between the low- and the high-risk groups. External validation of the prognostic model was performed using the GEO data set GSE68465 to test stability. Group-based survival analyses were performed based on age, sex, stage, and TNM to determine the predictive power of the risk signature for different patients. In order to investigate whether the risk signature can be used as an independent prognostic factor, we integrated the risk score with clinical parameters for univariable and multivariable Cox regression analyses. The R package “survivalROC” was used to calculate the area under the curve (AUC) to verify the prognostic performance. A nomogram was drawn using the R package “rms”.
Assessment of tumor immune microenvironment, stemness index, and immune checkpoint
Understanding the tumor immune microenvironment is a prerequisite for effective tumor immunotherapy. Using the R package “estimate”, the tumor stromal cell and immune cell scores were calculated based on the ESTIMATE algorithm to analyze the purity of the tumor. A correlation analysis between the risk score and DNA stemness score (DNAss) and RNA stemness score (RNAss) was performed through the Spearman method. Tumor immune evasion is involved in immune checkpoint pathways, and anticancer immunity can be enhanced by immune checkpoint inhibitors (29). Thus, we analyzed the expression differences of some common immune checkpoints between low- and high-risk groups.
Immune infiltrates analysis
The tumor-infiltrated immune cells are an important component of the tumor immune microenvironment. The tumor immune estimation resource (TIMER) database (https://cistrome.shinyapps.io/timer/) can comprehensively analyze the infiltrating immune cells in tumors (30). According to the data of immune infiltration in LUAD patients in TIMER, we analyzed the correlation between risk score and infiltrating immune cells. Based on CIBERSORT (http://cibersort.stanford.edu/), the deconvolution algorithm was employed to evaluate the proportion of 22 immune cell subtypes in LUAD patients (31) to compare the differences in immune cell subtypes between the low- and high-risk groups, as well as the association between immune cells.
Principal component analysis (PCA) and gene set enrichment analysis (GSEA)
PCA was used to show the distribution patterns of different groups. The 3-dimensional graph was plotted by the R package “pca3d”. GSEA was used to assess whether there were differences in immune-related pathways between low- and high-risk patients. GSEA was used to analyze the KEGG enrichment pathways in the low- and high-risk groups. Each analyzed genome was repeated 1,000 times (32).
Drug sensitivity
Finally, we wanted to explore which drugs were sensitive to the immune-related RBPs constructed in the risk signature. Drug sensitivity processed data was obtained from the CellMiner database (https://discover.nci.nih.gov/cellminer/home.do). According to the analysis results, the top 16 sensitive drugs were selected for display using the R package “ggplot2”.
Statistical analysis
Unless otherwise specified, P<0.05 was considered statistically significant. All analyses were performed using R software (version 4.0.4), as described above.
Results
Differentially expressed genes
First, the differentially expressed RBPs and IGs between LUAG and normal samples were identified. Based on the threshold, 245 up-regulated and 139 down-regulated differentially expressed RBPs were identified (Figure 1A). In addition, 484 up-regulated and 337 down-regulated IGs were identified (Figure 1B).
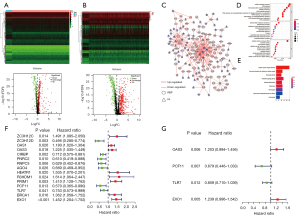
Co-expression regulatory network and enrichment analysis
Then, the role of the RBPs in the immune system was examined. A total of 278 pairs of interacting genes were identified through Pearson correlation analysis, among which the number of immune-related RBPs was 91 (90 significantly up-regulated genes, and one significantly down-regulated gene). A co-expression regulatory network was constructed to show the regulatory relationship between differentially expressed RBPs and IGs by Cytoscape (Figure 1C). GO enrichment (Figure 1D) and KEGG pathway (Figure 1E) analysis of immune-related RBPs were performed to understand their roles better. GO enrichment analysis indicated that the BPs were mainly associated with the non-coding RNA (ncRNA) metabolic process and RNA splicing. The CCs were abundant in the external side of the plasma membrane and spliceosomal complex. The MFs were most significantly enriched in catalytic activity, acting on RNA and nuclease activity (Table S2). Moreover, the KEGG pathways analysis showed that the RBPs were mainly enriched in cytokine-cytokine receptor interaction and viral protein interaction with cytokine and cytokine receptor (Table S3).
Prognostic immune-related RBPs
Univariable Cox regression was used to analyze genes associated with OS. From 91 immune-related RBPs, we identified 15 RBPs with prognostic value (Figure 1F and Table S4). After lasso Cox regression and multivariable Cox regression analysis, we finally got four independent prognostic RBPs (Figure 1G and Table S5). Then the risk genes were validated from multiple angles. First, gene mutations of the risk genes were detected in the cBioPortal database. Risk genes expression patterns were altered in 23% of 586 LUAD patients. EXO1 had the highest mutation rate of 10%. The most common forms of genetic alterations were amplification and missense mutations (Figure S1A). Next, we verified the differences in the expression of risk genes between tumor and normal tissues. Based on UALCAN database, the mRNA expressions of OAS3 and EXO1 in tumor tissues were increased while the mRNA expressions of PCF11 and TLR7 were opposite (Figure S1B). Based on the GEPIA2 database, we compared the prognosis of high and low expression of risk genes in LUAD patients (Figure S1C). We can see that the expression of risk genes and the results of their survival analysis were consistent with the effect of the signs of these coefficients. This validated our previous analysis.
Validation of the immune-RBP-based prognostic risk model for LUAD
According to the expression of the risk genes and regression coefficients, the formula for constructing a risk signature was: risk score = (OAS3*0.185096492873965) + (PCF11*−0.387198865656384) + (TLR7*−0.15166316298057) + (EXO1*0.214581605857755). The training group consisted of 486 LUAD patients from TCGA, then divided into low- and high-risk groups based on the median risk score. The median value of TCGA was between 0.987 and 0.935. The median value of GEO was between 0.986 and 0.988. Then, the risk score range of TCGA patients was 0.303 to 3.045, and the risk score range of GEO patients was 0.248 to 3.306. Compared with the high-risk group, OS was significantly higher in the low-risk group (Figure 2A). The AUC of the training group model was 0.731 (Figure 2B). We analyzed the survival distribution among patients in the low- and high-risk groups and observed that mortality increased with the increase in risk score (Figure 2C). Finally, a heat map showed the expression changes of risk genes in different groups (Figure 2D).
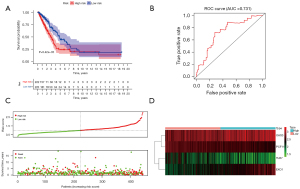
Independent external verification was performed using GSE68465 from the GEO database, which contains a total of 443 LUAD patients. The above formula was used again to obtain a risk score for each patient. The low-risk group also showed a better prognosis (Figure 3A). The AUC of the test group model was 0.707 (Figure 3B). Besides, the survival overview and gene expression heat map were shown in Figure 3C,3D. Therefore, the above validation results proved the stability of the model prediction and its good predictive ability of LUAD patients’ OS. Finally, we further analyzed the relationship between the risk signature and the grouping of patients with different clinical parameters. Except for metastasis, all the others showed significant differences (Figure 3E).
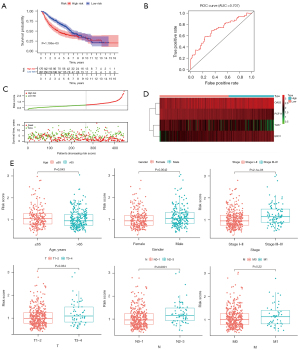
Construction of a nomogram for clinical practice
The prognostic value of the risk model was further evaluated using hazard ratios (HRs) of the risk scores in univariable and multivariable Cox regression analyses (Figure 4A,4B, and Table S6). The HRs were 2.11 and 1.86 in the univariable and multivariable Cox regression analyses, respectively (P<0.001). This means that the risk signature can be used as an independent prognostic factor, and the higher the risk score, the worse the prognosis. ROC curves were used to evaluate the specificity and sensitivity of our risk signature, and the AUC was 0.733, suggesting the risk signature for LUAD was highly reliable (Figure 4C). We used four immune-related RBPs to construct a nomogram for predicting prognosis, which can easily calculate the OS of LUAD patients at 1, 3, and 5 years (Figure 4D).

Tumor immune microenvironment, stemness index, and immune checkpoint
We performed risk signature analysis on tumor immune microenvironment, Stemness index, and immune checkpoints. The result showed that stromal cell score (R=−0.29, P<0.001) and immune cell score (R=−0.35, P<0.001) were negatively correlated with a risk score. Moreover, the risk score was positively correlated with DNAss (R=0.21, P<0.001) and RNAss (R=0.55, P<0.001) (Figure 5A). Cancer stemness represents the similarity of cancer cells with stem cells. Immune checkpoints are proteins involved in tumor immune escape. Among traditional immune checkpoints such as PD-L1 (Figure S2A), PD-1 (Figure S2B), and CTLA4, only CTLA4 (P=0.046, Figure 5B) showed significant differences. Still, other potential emerging immune checkpoints under investigation all showed significant differences. For instance, LAG3 (P=0.0044), TIGIT (P=0.04), and TIM3 (P=0.0015) (Figure 5B).
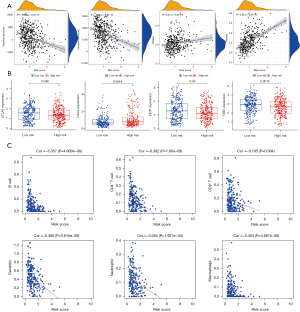
Immune infiltration and immune cell subtypes
Based on the data downloaded from the TIMER database, the Spearman correlation test was performed with the R software to obtain the correlation between the risk signature and the level of immune cell infiltration in LUAD. All immune cells were negatively correlated with risk score: B cell (Cor =−0.357, P<0.001), CD4+ T cell (Cor =−0.392, P<0.001), CD8+ T cell (Cor =−0.195, P=0.004), dendritic cells (Cor =−0.380, P<0.001), neutrophil (Cor =−0.284, P<0.001), and macrophage (Cor =−0.303, P<0.001) (Figure 5C). Using CIBERSORT, we further assessed whether there were differences in the abundance of 22 different immune cell infiltrates based on the risk signature. In the relatively high-risk group, B cell memory macrophages M2, resting dendritic cells, resting mast cells, and eosinophils were highly expressed in the low-risk group, while natural killer cells resting, Macrophages M0 and dendritic cells activated were the opposite (P<0.05; Figure 6A). Heatmap showed the interaction between different cells based on Pearson correlation analysis (Figure 6B).
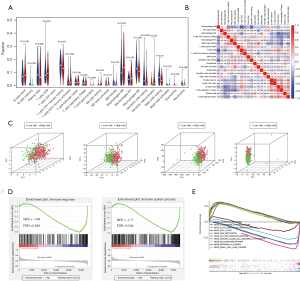
Comparison of patients in the high- and low-risk groups
We used PCA to show the different distribution patterns of the high- and low-risk groups. Based on our immune-related RBP, the low- and high-risk groups were clearly distinguished from each other (Figure 6C). According to other standards, such as whole RBPs, whole IGs, and whole-genome sets (Figure 6C), all fail to distinguish between low- and high-risk groups of patients better. Normalized enrichment score (NES) is the standardized result, with positive values indicating that the pathway is associated with the first phenotype group and negative values indicating that the pathway is associated with the second phenotype. As shown in Figure 6D, immune response [NES =−1.69, false discovery rate (FDR) =0.049] and immune system process (NES =−1.71, FDR =0.044) were more annotated in the low-risk group. These results are consistent with our previous immune-related analyses, indicating that the low-risk scores were correlated with the enhanced immunophenotype. We further performed GSEA on the gene sets of patients in the low- and high-risk groups to identify the KEGG pathways involved. The top five enrichment pathways were selected to display, respectively. The high-risk group was mainly enriched in cell cycle, DNA replication, pathways in cancer, p53 signaling pathway, and SCLC. The low-risk group was mainly enriched in allograft rejection, autoimmune thyroid, B cell receptor signaling, jak stat signaling, and natural killer cell-mediated cytotoxicity (Figure 6E). Different enrichment pathways of patients in low- and high-risk groups may indicate different prognoses and appropriate treatment methods.
Drug sensitivity
In order to explore the potential correlation between immune-related RBPs in the signature and drug sensitivity, we used human cancer cell lines from the CellMiner database for correlation analysis. TLR7 was positively correlated with alectinib, denileukin diftitox (ontak), fluphenazine, isotretinoin, LDK-378, imiquimod, megestrol acetate, nelfinavir, estramustine, celecoxib, dromostanolone propionate, and negatively correlated with irofulven. EXO1 was positively correlated with vorinostat and 6-thioguanine. PCF11 was positively correlated with nelarabine and vorinostat (Figure 7). All these drugs modulate the tumor immune microenvironment to induce cancer cell death (33-46).
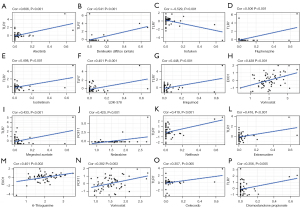
Discussion
RBPs play critical roles in cancer. Immunotherapy is effective in many cancer types, LUAD. This study aimed to construct and validate a risk signature with predictive power based on immune-related RBPs for LUAD patients. The results suggest that the risk signature of immune-related RBPs established in this study can provide the basis for clinical decisions regarding diagnosis, prognosis, and immunotherapy in LUAD patients.
The expression level of oncogenes and tumor suppressor genes can be affected by changes in RBPs expression and location. In addition, various cancer-associated phenotypes such as metastasis, invasion, aging, angiogenesis, apoptosis, and proliferation can be regulated by RBP-mediated genes. Therefore, there must be some relationship between cancer prognosis and RBP (47). Researchers are committed to developing anti-cancer drugs that target RBP, which is expected to become a new direction for cancer treatment in the future (48). Still, the biological roles and mechanisms of most RBPs have not been fully elucidated in LUAD. RBPs are also important regulators of the immune response. More and more scholars have focused on the study of RBP in tumor immunotherapy (49). For instance, it has been reported recently that cold-inducible RBP can enhance immunotherapeutic responses against hepatocellular carcinoma (50). The specific deletion of RBP PCBP1 in T cells facilitates the differentiation of Treg cells and generates a variety of inhibitory immune checkpoints, including VISTA, TIGIT, and PD-1 on tumor-infiltrating lymphocytes, thereby inactivating anti-tumor immunity (51). Nevertheless, many immunomodulatory RBPs have not been identified in LUAD. Consequently, we aimed to investigate the immune-related RBPs in LUAD diagnosis, prognosis, and immunity.
Based on the TCGA and immPort databases, we identified 384 RBPs and 488 IGs that were differentially expressed in LUAD and normal tissues. Through the correlation analysis between IGs and RBPs, 91 immune-related RBPs in LUAD were identified. GO enrichment analysis revealed the immune-related RBPs were primarily enriched in ncRNA metabolic process, external side of the plasma membrane, catalytic activity, and acting on RNA. KEGG enrichment analysis showed that the immune-related RBPs were mainly enriched in cytokine-cytokine receptor interaction.
We eventually identified four independent prognostic immune-related RBPs (OAS3, PCF11, TLR7, and EXO1) as risk genes to construct the risk signature. The expression levels of OAS3 and EXO1 were up-regulated in LUAD, while PCF11 and TLR7 were down-regulated in LUAD. Simultaneously, higher expression of OAS3 and EXO1 and lower expression of PCF11 and TLR7 in LUAD patients were associated with worse OS. These results suggest that they may become potential biomarkers for the diagnosis and prognosis of LUAD. OAS3 (2'-5'-oligoadenylate synthetase 3) has an antiviral effect on alphavirus (52). Recent study has identified several molecules as inhibitors of the OAS family, especially interacting with Tyr230, Asp75, Asp77, and Asp75 in OAS3, indicating that OAS3 could be expected to become a new drug target (53). PCF11 (PCF11 cleavage and polyadenylation factor subunit) directs neural differentiation, apoptosis, proliferation, and cell cycle by shaping the inputs that converge on WNT signaling. A recent study reported that down-regulation of postnatal PCF11 promotes neurodifferentiation, and low levels of PCF11 were associated with spontaneous tumor regression and good prognosis (54). Toll-like receptor (TLR) ligand’s effect on immune activation makes it a drug target for treating cancer and infectious diseases (55). Because TLRs bind to cell membranes and present a wide range of expression patterns. Even local application may cause the release of systemic cytokines, resulting in inhibitory side effects (56). In contrast, TLR7 has a more limited range of action, including only certain types of immune cells, and can serve as a treatment target (57). Preclinical studies have shown that TLR7 agonists have antitumor and immunomodulatory effects. For example, studies have demonstrated that the TLR7 agonist R-848 can enhance anti-tumor effects in cetuximab-coated colorectal cancer (CRC) cell lines through antibody-dependent cellular cytotoxicity (ADCC) (58). EXO1 is an evolutionarily highly conserved exonuclease (59). Compared with the above genes, EXO1 had the highest mutation rate. As previously reported, abnormal expression of EXO1 may cause genomic instability, including homologous recombination, telomere maintenance, and DNA mismatch repair (60-62). Some studies claimed that increased expression of EXO1 promotes tumor invasion and metastasis, leading to a worse prognosis for cancer patients (63,64). In terms of immune infiltrate, overexpression of EXO1 might lead to decreased antibody diversity and immunoglobulin maturation malfunction, lowering the number of infiltrating B cells even further (62).
Through gradual screening, a risk signature was finally established based on the above four immune-related RBPs. According to the median risk score, LUAD patients were divided into the high- and low-risk groups. Low-risk patients had better OS than high-risk patients. It was validated in another independent cohort from the GEO database. In order to explore the feasibility of the prognostic model in clinical application, we compared this prognostic model with the clinical parameters of LUAD patients, such as age, gender, and pathological stage. After univariable and multivariable Cox and ROC analyses, the risk signature was an independent prognostic factor of LUAD. To enable our prognostic model to gain more reliable and valuable predictive power in clinical applications, we developed a nomogram that included OAS3, PCF11, TLR7, and EXO1 to calculate the scores of different patients to predict the prognosis.
Recent studies have associated the immune microenvironment with the immunotherapeutic response of several cancers, including LUAD (65-67). By calculating immune scores, we could accurately determine the tumor purity and immune cell infiltration in the tumor microenvironment. DNAss and RNAss reflect epigenetic characteristics and gene expression, respectively (68). They were found to be positively correlated with the risk score in our analysis. An increase in stemness index indicates that the BPs of cancer stem cells were active, which might cause the dedifferentiation of differentiated tumors. Interestingly, a study found that elevated DNAss in some tumors led to decreased PD-L1 expression and leukocyte fraction, including BLCA, HNSC, LUSC, and GBM. Because of inherent down-regulation of the PD-L1 pathway or insufficient immune cell infiltration of tumors, such tumors were expected to be less susceptible to immune checkpoint blockade (68), supporting the present study.
With the successful application of immunotherapy, immune checkpoints have become the new focus of molecular oncologists. Although anti-cytotoxic T lymphocyte-associated protein 4 (anti-CTLA4) and anti-PD-1 therapies have achieved initial success, limitations were also exposed, such as the emergence of drug resistance in most patients. Other immune checkpoints that have the potential to become targets for immunotherapy have aroused the interest of researchers and were under study, such as TIM3, LAG3, and TIGIT (69). Coincidentally, our results suggested significant differences in immune checkpoints between the high- and low-risk groups, especially emerging immune checkpoints.
Based on the CellMiner database, we explored drugs with higher sensitivity to immune-related RBPs. Drug sensitivity was proportional to the amount of gene expression. Among them, TLR7 showed a close correlation with various drugs, and recent study has shown a link. For example, studies indicated that imiquimod, as a TLR7 agonist, promotes the immunogenicity of mesenchymal stem cells (70). In addition, EXO1 was positively correlated with vorinostat and 6-thioguanine. PCF11 was positively correlated with nelarabine and vorinostat. Of course, we only selected the top 16 sensitive drugs, and there are still many sensitive drugs worth exploring. Therefore, detecting the expression of TLR7, EXO1, and PCF11 has a certain guiding significance for selecting clinical drugs.
As far as we know, this is the first study to establish a risk signature based on immune-related RBPs in LUAD. This risk signature should be examined in the future for guiding immunotherapy in patients with LUAD. Nevertheless, several limitations of this study should also be noted. First, patient information was taken from the TCGA and GEO databases, and more sufficient clinical samples were needed for verification. Second, transcriptome analysis can only reflect certain aspects of the immune status and lacks overall changes. Third, this study was a retrospective study, and prospective studies were needed to confirm our conclusions.
In conclusion, this study identified differentially expressed and prognostic immune-related RBPs and used them to construct a risk signature of LUAD. Low-risk LUAD patients showed a better prognosis and better immunotherapy responses than high-risk patients. This study provided several biomarkers and a potential model for the prognosis and individualized immunotherapy of LUAD patients.
Acknowledgments
We would like to thank the ImmPort, TIMER, CIBERSORT, GEO, and TCGA databases for data availability.
Funding: This work was supported by The National Natural Science Foundation of China (No. 81974015).
Footnote
Reporting Checklist: The authors have completed the TRIPOD reporting checklist. Available at https://tcr.amegroups.com/article/view/10.21037/tcr-22-698/rc
Conflicts of Interest: Both authors have completed the ICMJE uniform disclosure form (available at https://tcr.amegroups.com/article/view/10.21037/tcr-22-698/coif). The authors have no conflicts of interest to declare.
Ethical Statement: The authors are accountable for all aspects of the work in ensuring that questions related to the accuracy or integrity of any part of the work are appropriately investigated and resolved. The study was conducted in accordance with the Declaration of Helsinki (as revised in 2013).
Open Access Statement: This is an Open Access article distributed in accordance with the Creative Commons Attribution-NonCommercial-NoDerivs 4.0 International License (CC BY-NC-ND 4.0), which permits the non-commercial replication and distribution of the article with the strict proviso that no changes or edits are made and the original work is properly cited (including links to both the formal publication through the relevant DOI and the license). See: https://creativecommons.org/licenses/by-nc-nd/4.0/.
References
- Siegel RL, Miller KD, Jemal A. Cancer statistics, 2020. CA Cancer J Clin 2020;70:7-30. [Crossref] [PubMed]
- Shi J, Hua X, Zhu B, et al. Somatic Genomics and Clinical Features of Lung Adenocarcinoma: A Retrospective Study. PLoS Med 2016;13:e1002162. [Crossref] [PubMed]
- Samstein RM, Lee CH, Shoushtari AN, et al. Tumor mutational load predicts survival after immunotherapy across multiple cancer types. Nat Genet 2019;51:202-6. [Crossref] [PubMed]
- Chan TA, Yarchoan M, Jaffee E, et al. Development of tumor mutation burden as an immunotherapy biomarker: utility for the oncology clinic. Ann Oncol 2019;30:44-56. [Crossref] [PubMed]
- Yang S, Wu Y, Deng Y, et al. Identification of a prognostic immune signature for cervical cancer to predict survival and response to immune checkpoint inhibitors. Oncoimmunology 2019;8:e1659094. [Crossref] [PubMed]
- Mitsudomi T, Yatabe Y. Epidermal growth factor receptor in relation to tumor development: EGFR gene and cancer. FEBS J 2010;277:301-8. [Crossref] [PubMed]
- Slebos RJ, Kibbelaar RE, Dalesio O, et al. K-ras oncogene activation as a prognostic marker in adenocarcinoma of the lung. N Engl J Med 1990;323:561-5. [Crossref] [PubMed]
- Karnoub AE, Weinberg RA. Ras oncogenes: split personalities. Nat Rev Mol Cell Biol 2008;9:517-31. [Crossref] [PubMed]
- Liu Q, Yu S, Zhao W, et al. EGFR-TKIs resistance via EGFR-independent signaling pathways. Mol Cancer 2018;17:53. [Crossref] [PubMed]
- Pao W, Girard N. New driver mutations in non-small-cell lung cancer. Lancet Oncol 2011;12:175-80. [Crossref] [PubMed]
- Miller KD, Nogueira L, Mariotto AB, et al. Cancer treatment and survivorship statistics, 2019. CA Cancer J Clin 2019;69:363-85. [Crossref] [PubMed]
- Scaturrok M, Sala A, Cutrona G, et al. Purification by affinity chromatography of H1o RNA-binding proteins from rat brain. Int J Mol Med 2003;11:509-13. [PubMed]
- Gerstberger S, Hafner M, Tuschl T. A census of human RNA-binding proteins. Nat Rev Genet 2014;15:829-45. [Crossref] [PubMed]
- Mino T, Takeuchi O. Post-transcriptional regulation of immune responses by RNA binding proteins. Proc Jpn Acad Ser B Phys Biol Sci 2018;94:248-58. [Crossref] [PubMed]
- Shi Y, Fan S, Wu M, et al. YTHDF1 links hypoxia adaptation and non-small cell lung cancer progression. Nat Commun 2019;10:4892. [Crossref] [PubMed]
- Wurth L. Versatility of RNA-Binding Proteins in Cancer. Comp Funct Genomics 2012;2012:178525. [Crossref] [PubMed]
- Qin H, Ni H, Liu Y, et al. RNA-binding proteins in tumor progression. J Hematol Oncol 2020;13:90. [Crossref] [PubMed]
- Hua X, Ge S, Chen J, et al. Effects of RNA Binding Proteins on the Prognosis and Malignant Progression in Prostate Cancer. Front Genet 2020;11:591667. [Crossref] [PubMed]
- Pereira B, Billaud M, Almeida R. RNA-Binding Proteins in Cancer: Old Players and New Actors. Trends Cancer 2017;3:506-28. [Crossref] [PubMed]
- Yang S, Lin S, Liu K, et al. Identification of an immune-related RNA-binding protein signature to predict survival and targeted therapy responses in liver cancer. Genomics 2021;113:795-804. [Crossref] [PubMed]
- Bhattacharya S, Andorf S, Gomes L, et al. ImmPort: disseminating data to the public for the future of immunology. Immunol Res 2014;58:234-9. [Crossref] [PubMed]
- Ashburner M, Ball CA, Blake JA, et al. Gene ontology: tool for the unification of biology. The Gene Ontology Consortium. Nat Genet 2000;25:25-9. [Crossref] [PubMed]
- Kanehisa M, Furumichi M, Tanabe M, et al. KEGG: new perspectives on genomes, pathways, diseases and drugs. Nucleic Acids Res 2017;45:D353-61. [Crossref] [PubMed]
- Yu G, Wang LG, Han Y, et al. clusterProfiler: an R package for comparing biological themes among gene clusters. OMICS 2012;16:284-7. [Crossref] [PubMed]
- Xia JC, Wang YF, Xu GQ, et al. Clinical analysis of cerebral angiography via transradial access. Chinese Neurosurgical Journal 2019;35:1121-3.
- Gao J, Aksoy BA, Dogrusoz U, et al. Integrative analysis of complex cancer genomics and clinical profiles using the cBioPortal. Sci Signal 2013;6:pl1. [Crossref] [PubMed]
- Chandrashekar DS, Bashel B, Balasubramanya SAH, et al. UALCAN: A Portal for Facilitating Tumor Subgroup Gene Expression and Survival Analyses. Neoplasia 2017;19:649-58. [Crossref] [PubMed]
- Tang Z, Kang B, Li C, et al. GEPIA2: an enhanced web server for large-scale expression profiling and interactive analysis. Nucleic Acids Res 2019;47:W556-60. [Crossref] [PubMed]
- Postow MA, Callahan MK, Wolchok JD. Immune Checkpoint Blockade in Cancer Therapy. J Clin Oncol 2015;33:1974-82. [Crossref] [PubMed]
- Li T, Fan J, Wang B, et al. TIMER: A Web Server for Comprehensive Analysis of Tumor-Infiltrating Immune Cells. Cancer Res 2017;77:e108-10. [Crossref] [PubMed]
- Newman AM, Liu CL, Green MR, et al. Robust enumeration of cell subsets from tissue expression profiles. Nat Methods 2015;12:453-7. [Crossref] [PubMed]
- Subramanian A, Tamayo P, Mootha VK, et al. Gene set enrichment analysis: a knowledge-based approach for interpreting genome-wide expression profiles. Proc Natl Acad Sci U S A 2005;102:15545-50. [Crossref] [PubMed]
- Tomasini P, Egea J, Souquet-Bressand M, et al. Alectinib in the treatment of ALK-positive metastatic non-small cell lung cancer: clinical trial evidence and experience with a focus on brain metastases. Ther Adv Respir Dis 2019;13:1753466619831906. [Crossref] [PubMed]
- Yamada Y, Aoyama A, Tocco G, et al. Differential effects of denileukin diftitox IL-2 immunotoxin on NK and regulatory T cells in nonhuman primates. J Immunol 2012;188:6063-70. [Crossref] [PubMed]
- Xu F, Xia Y, Feng Z, et al. Repositioning antipsychotic fluphenazine hydrochloride for treating triple negative breast cancer with brain metastases and lung metastases. Am J Cancer Res 2019;9:459-78. [PubMed]
- Dahl AR, Grossi IM, Houchens DP, et al. Inhaled isotretinoin (13-cis retinoic acid) is an effective lung cancer chemopreventive agent in A/J mice at low doses: a pilot study. Clin Cancer Res 2000;6:3015-24. [PubMed]
- Li S, Qi X, Huang Y, et al. Ceritinib (LDK378): a potent alternative to crizotinib for ALK-rearranged non-small-cell lung cancer. Clin Lung Cancer 2015;16:86-91. [Crossref] [PubMed]
- Dajon M, Iribarren K, Cremer I. Dual roles of TLR7 in the lung cancer microenvironment. Oncoimmunology 2015;4:e991615. [Crossref] [PubMed]
- Ulutin HC, Arpaci F, Pak Y. Megestrol acetate for cachexia and anorexia in advanced non-small cell lung cancer: a randomized study comparing two different doses. Tumori 2002;88:277-80. [Crossref] [PubMed]
- Rengan R, Mick R, Pryma DA, et al. Clinical Outcomes of the HIV Protease Inhibitor Nelfinavir With Concurrent Chemoradiotherapy for Unresectable Stage IIIA/IIIB Non-Small Cell Lung Cancer: A Phase 1/2 Trial. JAMA Oncol 2019;5:1464-72. [Crossref] [PubMed]
- Bergh J, Björk P, Westlin JE, et al. Expression of an estramustine-binding associated protein in human lung cancer cell lines. Cancer Res 1988;48:4615-9. [PubMed]
- Abou-Issa H, Alshafie G. Celecoxib: a novel treatment for lung cancer. Expert Rev Anticancer Ther 2004;4:725-34. [Crossref] [PubMed]
- Choudhary MI, Siddiqui M. Bio-Catalytic Structural Transformation of Anti-cancer Steroid, Drostanolone Enanthate with Cephalosporium aphidicola and Fusarium lini, and Cytotoxic Potential Evaluation of Its Metabolites against Certain Cancer Cell Lines. Front Pharmacol 2017;8:900. [Crossref] [PubMed]
- Sherman CA, Herndon JE 2nd, Watson DM, et al. A phase II trial of 6-hydroxymethylacylfulvene (MGI-114, irofulven) in patients with relapsed or refractory non-small cell lung cancer. Lung Cancer 2004;45:387-92. [Crossref] [PubMed]
- Gray JE, Saltos A, Tanvetyanon T, et al. Phase I/Ib Study of Pembrolizumab Plus Vorinostat in Advanced/Metastatic Non-Small Cell Lung Cancer. Clin Cancer Res 2019;25:6623-32. [Crossref] [PubMed]
- DeAngelo DJ, Yu D, Johnson JL, et al. Nelarabine induces complete remissions in adults with relapsed or refractory T-lineage acute lymphoblastic leukemia or lymphoblastic lymphoma: Cancer and Leukemia Group B study 19801. Blood 2007;109:5136-42. [Crossref] [PubMed]
- Kang D, Lee Y, Lee JS. RNA-Binding Proteins in Cancer: Functional and Therapeutic Perspectives. Cancers (Basel) 2020;12:2699. [Crossref] [PubMed]
- Mohibi S, Chen X, Zhang J. Cancer the'RBP'eutics-RNA-binding proteins as therapeutic targets for cancer. Pharmacol Ther 2019;203:107390. [Crossref] [PubMed]
- Newman R, McHugh J, Turner M. RNA binding proteins as regulators of immune cell biology. Clin Exp Immunol 2016;183:37-49. [Crossref] [PubMed]
- Silva L, Egea J, Villanueva L, et al. Cold-Inducible RNA Binding Protein as a Vaccination Platform to Enhance Immunotherapeutic Responses Against Hepatocellular Carcinoma. Cancers (Basel) 2020;12:3397. [Crossref] [PubMed]
- Ansa-Addo EA, Huang HC, Riesenberg B, et al. RNA binding protein PCBP1 is an intracellular immune checkpoint for shaping T cell responses in cancer immunity. Sci Adv 2020;6:eaaz3865. [Crossref] [PubMed]
- Henrik Gad H, Paulous S, Belarbi E, et al. The E2-E166K substitution restores Chikungunya virus growth in OAS3 expressing cells by acting on viral entry. Virology 2012;434:27-37. [Crossref] [PubMed]
- Gonzalez KJ, Moncada-Giraldo DM, Gutierrez JB. In silico identification of potential inhibitors against human 2'-5'- oligoadenylate synthetase (OAS) proteins. Comput Biol Chem 2020;85:107211. [Crossref] [PubMed]
- Ogorodnikov A, Levin M, Tattikota S, et al. Transcriptome 3'end organization by PCF11 links alternative polyadenylation to formation and neuronal differentiation of neuroblastoma. Nat Commun 2018;9:5331. [Crossref] [PubMed]
- Vacchelli E, Eggermont A, Sautès-Fridman C, et al. Trial Watch: Toll-like receptor agonists for cancer therapy. Oncoimmunology 2013;2:e25238. [Crossref] [PubMed]
- Engelhardt R, Mackensen A, Galanos C. Phase I trial of intravenously administered endotoxin (Salmonella abortus equi) in cancer patients. Cancer Res 1991;51:2524-30. [PubMed]
- Witt PL, Ritch PS, Reding D, et al. Phase I trial of an oral immunomodulator and interferon inducer in cancer patients. Cancer Res 1993;53:5176-80. [PubMed]
- Butchar JP, Mehta P, Justiniano SE, et al. Reciprocal regulation of activating and inhibitory Fc{gamma} receptors by TLR7/8 activation: implications for tumor immunotherapy. Clin Cancer Res 2010;16:2065-75. [Crossref] [PubMed]
- Sertic S, Quadri R, Lazzaro F, et al. EXO1: A tightly regulated nuclease. DNA Repair (Amst) 2020;93:102929. [Crossref] [PubMed]
- Amin NS, Nguyen MN, Oh S, et al. exo1-Dependent mutator mutations: model system for studying functional interactions in mismatch repair. Mol Cell Biol 2001;21:5142-55. [Crossref] [PubMed]
- Xue Y, Marvin ME, Ivanova IG, et al. Rif1 and Exo1 regulate the genomic instability following telomere losses. Aging Cell 2016;15:553-62. [Crossref] [PubMed]
- Tomimatsu N, Mukherjee B, Harris JL, et al. DNA-damage-induced degradation of EXO1 exonuclease limits DNA end resection to ensure accurate DNA repair. J Biol Chem 2017;292:10779-90. [Crossref] [PubMed]
- Luo F, Wang YZ, Lin D, et al. Exonuclease 1 expression is associated with clinical progression, metastasis, and survival prognosis of prostate cancer. J Cell Biochem 2019; [Epub ahead of print]. [Crossref] [PubMed]
- Qi L, Zhou B, Chen J, et al. Significant prognostic values of differentially expressed-aberrantly methylated hub genes in breast cancer. J Cancer 2019;10:6618-34. [Crossref] [PubMed]
- Herbst RS, Soria JC, Kowanetz M, et al. Predictive correlates of response to the anti-PD-L1 antibody MPDL3280A in cancer patients. Nature 2014;515:563-7. [Crossref] [PubMed]
- Rizvi NA, Hellmann MD, Snyder A, et al. Cancer immunology. Mutational landscape determines sensitivity to PD-1 blockade in non-small cell lung cancer. Science 2015;348:124-8. [Crossref] [PubMed]
- Topalian SL, Taube JM, Anders RA, et al. Mechanism-driven biomarkers to guide immune checkpoint blockade in cancer therapy. Nat Rev Cancer 2016;16:275-87. [Crossref] [PubMed]
- Malta TM, Sokolov A, Gentles AJ, et al. Machine Learning Identifies Stemness Features Associated with Oncogenic Dedifferentiation. Cell 2018;173:338-354.e15. [Crossref] [PubMed]
- Kondratova M, Barillot E, Zinovyev A, et al. Modelling of Immune Checkpoint Network Explains Synergistic Effects of Combined Immune Checkpoint Inhibitor Therapy and the Impact of Cytokines in Patient Response. Cancers (Basel) 2020;12:3600. [Crossref] [PubMed]
- Zhang L, Liu D, Pu D, et al. The TLR7 agonist Imiquimod promote the immunogenicity of mesenchymal stem cells. Biol Res 2015;48:6. [Crossref] [PubMed]