Integrated analysis of multiple bioinformatics studies to identify microRNA-target gene-transcription factor regulatory networks in retinoblastoma
Introduction
Retinoblastoma (RB) is the most common primary intraocular tumor of childhood, seriously threatening the vision and life of children (1,2). Common signs of RB include leukocoria, strabismus, glaucoma and inflammation. To date, a range of treatments is available to RB experts, including chemotherapy, focal treatment, radiation therapy and surgery (3). Although treatment methods have improved over the past few years, mortality remains ~70% in developing countries (4). In order to find new therapeutic strategies, we need to carefully study the biological processes (BP) and related underlying mechanisms of RB.
MicroRNAs (miRNAs) are small endogenous noncoding RNAs that function as universal specificity factors in mRNA posttranscriptional gene silencing (5). MiRNAs comprise a growing class of ~22 nt long nonprotein-coding RNAs (6). Considerable evidence indicates that miRNAs and their biogenesis machinery are involved in the development of cancer. In recent years, many research groups have focused their efforts on studying the causes of aberrant miRNA expression in cancer (7). Previously, some studies have suggested that miRNAs play a role in regulating the progression of RB. For example, miR-361-3p (8), miR-330 (9) and miRNA‑874‑3p (10) are all involved in the development of RB. Some genes have also been reported to influence RB progression, for instance, taurine up-regulated 1 (TUG1) (11) and centromere protein E (CENPE) (12). Kruppel like factor 2 (KLF2), a transcription factor (TF), has also been involved in the development of RB (13). Therefore, elucidating the relationships among aberrantly expressed miRNAs and their target mRNAs and TFs in RB may provide new ideas for the study of RB molecular mechanisms and therapies. In this study, three mRNA expression profiles (GSE111168, GSE110811, GSE125903) and one miRNA expression profile (GSE41321) from the GEO database were selected, and differentially expressed genes (DEGs) and differentially expressed miRNAs (DEMs) were identified by comparing RB and noncancer samples. Then, we performed miRNA-target gene network analysis and further identified TFs related to the key DEMs from the interaction network. Our work will support the screening of key genes, miRNAs and TFs in RB and the construction of miRNA-target gene-TF regulatory networks to determine the crucial molecular mechanisms involved in RB. We present the following article in accordance with the REMARK reporting checklist (available at https://tcr.amegroups.com/article/view/10.21037/tcr-21-1748/rc).
Methods
Bioinformatics data
The Gene Expression Omnibus (GEO) database is an international public repository that archives and distributes high-throughput gene expression and genomics datasets. We downloaded both mRNA and miRNA expression profiles from the GEO database (http://www.ncbi.nlm.nih.gov/gds/). The GSE111168, GSE110811 and GSE125903 datasets were used for mRNA, while the GSE41321 dataset was used for miRNA. All data sets were screened according to the following criteria: (I) not less than 6 samples, (II) from human tissues, and (III) data sets included case controls. We extracted and summarized information from each dataset, as shown in Table 1. The study was conducted in accordance with the Declaration of Helsinki (as revised in 2013).
Table 1
Author, year | GEO | Platform | Control | Tumor |
---|---|---|---|---|
Chai et al., 2018 | GSE111168 | GPL11154 | 3 | 3 |
Hudson et al., 2018 | GSE110811 | GPL16686 | 3 | 28 |
Rajasekaran et al., 2019 | GSE125903 | GPL16791 | 3 | 7 |
Beta et al., 2013 | GSE41321 | GPL14767 | 3 | 3 |
The last one is miRNA expression profile, the rest are mRNA expression profiles. miRNA, microRNA; GEO, Gene Expression Omnibus.
Analysis of DEGs and DEMs
We conducted data analysis by using R program (V4.1.0. R Core Team (2021). R: A language and environment for statistical computing. R Foundation for Statistical Computing, Vienna, Austria. URL https://www.R-project.org/). The limma R package was used to identify aberrantly expressed genes and aberrantly expressed miRNAs in RB tissues compared to normal tissues. To decrease the false-positive rate, we utilized the Benjamini–Hochberg false discovery rate (FDR) method to obtain the adjusted P value. Genes with an adjusted P<0.05 and |log fold change (FC)| >1 were considered DEGs. Standard for the truncation of abnormal expression of miRNAs was a |log fold change (FC)| >0.5 and adjusted P<0.05. However, among DEGs, we maintained the upregulated and downregulated gene lists as Excel files. Then, they were sorted by logFC for further integration analysis. The Version of RStudio is 3.6.1.
Integration of microarray data
The robust rank aggregation (RRA) R package (https://cran.rstudio.com/bin/windows/contrib/3.5/RobustRankAggreg_1.1.zip) was used to integrate the two final Excel files according to previously published work (14). The adjusted P value in the RRA tool indicates the possibility of each gene ranking highly in the final two gene lists. Genes with adjusted P value <0.05 and |logFC| >1 were considered significantly altered. Finally, a comprehensive list of up-regulated and down-regulated DEGs was saved for further analysis.
Functional and pathway enrichment analysis
Gene ontology (GO) analysis and Kyoto Encyclopedia of Genes and Genomes (KEGG) pathway analysis were performed to analyze the functions of the DEGs. Then, we used the GOplot R package to visualize the GO analysis results, and the Cytoscape (Version 3.8.0) plugin ClueGO (Version 2.5.7) was carried out to visualize the results of KEGG pathway analysis. The ClueGO plugin provides a series of biological explanations for gene lists that identify and functionally classify important KEGG pathways. A P value <0.05 was selected as a cutoff criterion for both GO terms and KEGG pathway enrichment analysis.
Protein-protein interaction (PPI) network construction and analysis of modules
Hub genes are considered key genes that play an important role in regulating disease progression. We uploaded key genes identified using the RRA method to the STRING database (http://www.string-db.org/, Version 11.5). The PPIs network was analyzed by using the combined score from STRING database, with the association score >0.4 as the cut-off criterion. Moreover, PPIs were visualized using Cytoscape software. We also used a Cytoscape plugin, CytoNCA (Version 2.1.6), to evaluate the score of key genes for centrality analysis (15). Then, molecular complex detection (MCODE) (Version 1.6.1) was used to identify densely connected regions based on topology (MCODE degree cutoff = 2, maximum depth = 100, node score cutoff = 0.2 and k Core = 2).
Prediction of TFs and miRNAs and construction of a miRNA-target gene-TF regulatory network
We utilized MiRTarBase (http://mirtarbase.mbc.nctu.edu.tw/php/index.php, Version 7.0) to predict miRNAs that possibly regulate the DEGs. The online tool miRTarBase is a manually collected, experimentally validated miRNA target gene database. Only miRNAs supported by strong evidence were included. We then identified critical miRNAs by crossing DEMs acquired from the GSE41321 profile dataset with miRNAs obtained from miRTarBase. In the present research, TFs that might be associated with the key DEMs were predicted using TransmiR (http://www.cuilab.cn/transmir, Version 2.0). Likewise, only data with evidence from the literature was identified. Ultimately, miRNA-target gene-TF regulatory networks were established.
Cell culture
Human retinal pigment epithelial cells (ARPE-19, FH0543, FuHeng Cell Center, Shanghai, China) and human RB cell lines (WERI-Rb-1, FH0300, FuHeng Cell Center) were both purchased from FuHeng Cell Center. WERI-Rb-1 cells were cultured in RPMI-1640 (Gibco, 72400047, USA) medium containing 10% fetal bovine serum (FBS) (Gibco,10099141C), 100 µg/mL penicillin, and 100 µg/mL streptomycin (Gibco,15140122, USA) at 37 ℃ and 5% CO2. ARPE-19 cells were cultured in DMEM/F12 (Gibco,11330057, USA) medium containing 10% FBS (Gibco,10099141C, USA), 100 µg/mL penicillin, and 100 µg/mL streptomycin (Gibco,15140122, USA) at 37 ℃ and 5% CO2.
Total RNA extraction and RT-qPCR validation
We used TRIzol (Invitrogen, CA, USA) to extracted RNA from the cell lines according to the manufacturer’s protocol. A NanoDrop 2000 (Thermo Fisher Scientific, USA) was used to measure RNA purity and concentration. We extracted RNA using 6-well plates with 1×107 cells in each well, and each set of experiments was repeated at least three times. Total RNA was reverse transcribed into cDNA using a cDNA Synthesis Kit (Takara, RR036A, Japan). The cDNA synthesis conditions were 37 ℃ for 15 min followed by 85 ℃ for 5 s. RNA expression was then examined by quantitative real-time PCR using SYBR® Premix Ex Taq™ II (Takara, RR820A, Japan) and MicroRNAs qPCR (BioTNT, China) kits and a Biosystems 7500 Fast Real-Time PCR System. The thermal cycling conditions were 95 ℃ for 30 s followed by 40 cycles of 95 ℃ for 5 s and 60 ℃ for 34 s for PCR. The expression of miRNA and mRNA was normalized to U6 and GAPDH expression respectively. The 2-∆∆Ct method was used to calculate the expression levels of miRNA and mRNA expression. The primers for quantitative real-time polymerase chain reaction (RT-qPCR) used in the present research are as follows: let-7a-5p, 5'- CTC AAC TGG TGT CGT GGA GTC GGC AAT TCA GTT GAG AAC TAT AC -3'; let-7b-5p, 5'- CTC AAC TGG TGT CGT GGA GTC GGC AAT TCA GTT GAG AAC CAC AC -3'; U6, 5'- AAA AAT ATG GAA CGC TTC ACG -3'; the mRNA primers were listed in Table S1.
Statistical analysis
GraphPad Prism Version 8.0 was used to analyze the RT-qPCR data, and comparisons between two groups were performed using Student’s t test, with a P value <0.05.
Results
Identification of DEGs and DEMs in RB using integrated bioinformatics
Three mRNA and one miRNA expression profile datasets, including a total of 41 RB and 12 healthy samples, were downloaded from the GEO database. Aberrantly expressed genes and miRNAs were identified using the limma R package with adjusted P<0.05 and |logFC| >1. Three hundred eighteen abnormally expressed genes were obtained in the GSE110811 profile dataset, consisting of 165 upregulated genes and 153 downregulated genes. Additionally, 3,003 abnormally expressed genes were identified in the GSE111168 profile dataset, including 2,887 upregulated genes and 116 downregulated genes, and 869 abnormally expressed genes were established in the GSE125903 profile dataset, involving 336 downregulated genes and 533 downregulated genes. The miRNA expression profile (GSE41321) revealed 35 DEMs, including 18 upregulated miRNAs and 16 downregulated miRNAs. The volcano plots of the four datasets are shown in Figure 1, and Figure 2 shows the heatmaps of aberrantly expressed genes and miRNAs.
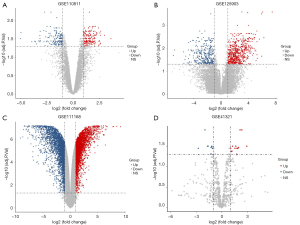
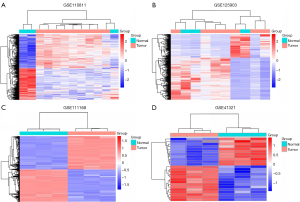
We identified the overlapping expression matrix of the three mRNA profile datasets using the limma R package. The results were sorted according to log fold change. Then, the RRA R package was used to identify integrated DEGs. Only results with adjusted P<0.05 and |logFC| >1 were included. The RRA method operates on the hypothesis that every gene in each dataset is randomly arranged. According to the gene ranks in all datasets, the higher the ranking, the lower the correlating P value and the greater the possibility of abnormally expressed genes. Using the RRA method, we obtained a total of 180 integrated DEGs, containing 109 upregulated genes and 71 downregulated genes (Table S2).
Functional enrichment analysis of DEGs
In order to understand the function and mechanism of these DEGs, KEGG pathways and GO terms, including BP, cellular component (CC), and molecular function (MF), were identified by utilizing the GOplot R package and the ClueGO plugin. The results were considered statistically significant if the P<0.05, and the three parts of the GO results are shown in Figure 3. In the BP-related category, the top 5 significantly enriched GO terms were regulation of chromosome segregation (GO: 0007059) with P=5.23E-14, nuclear division (GO: 0000280) with P=9.30E-14, organelle fission (GO: 0048285) with P=7.77E-13, mitotic nuclear division (GO: 0140014) with P=1.30E-12 and sister chromatid segregation (GO: 0000819) with P=7.31E-12. In the cell component (CC)-associated category, the most considerably enriched GO terms were spindle (GO: 0005819) with a P=8.18E-09, condensed chromosome (GO: 0000793) with a P=8.12E-09, spindle microtubule (GO: 0005876) with a P=7.04E-07, MCM complex (GO: 0042555) with a P=8.39E-05 and spindle pole (GO: 0000922) with a P=8.67E-05. In the MF-associated category, the key DEGs were enriched in DNA replication origin binding (GO: 0003688) with P=5.43E-08, ferric iron binding (GO: 0008199) with P=0.004132, coreceptor binding (GO: 0039706) with P=0.005792, heparan sulfate proteoglycan binding (GO: 0043395) with P=0.008756 and amyloid-beta binding (GO: 0001540) with P=0.032651. The KEGG pathway analysis of the combined DEGs was carried out by using Cytoscape software, and the results of the analysis are shown in Figure 4. The identified DEGs were primarily enriched in five pathways; pyrimidine metabolism, DNA replication, homologous recombination, Fanconi anemia pathway and cell cycle.
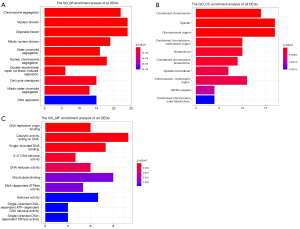
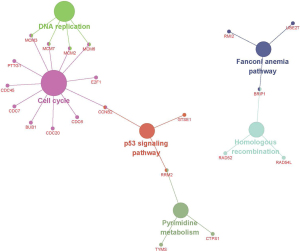
PPI network construction and analysis of modules
A PPI network was established for 180 DEGs in RB tissues compared to control tissues, containing 167 nodes and 1,115 edges (Figure S1). A network topology analysis was used to identify the top 20 nodes with high degrees. We identified top 20 nodes as hub genes according to the three centrality methods (Table 2), including genes like cell division cycle 20 (CDC20), aurora kinase B (AURKB), DNA topoisomerase II alpha (TOP2A), kinesin family member 11 (KIF11), BUB1 mitotic checkpoint serine/threonine kinase (BUB1), cyclin B2 (CCNB2), ribonucleotide reductase regulatory subunit M2 (RRM2), cell division cycle 45 (CDC45), minichromosome maintenance complex component 2 (MCM2), and kinesin family member 20A (KIF20A). Next, we performed subnetwork module analysis and obtained a total of 3 cluster modules. The top modules with the highest score (score: 39.762) included 43 nodes and 835 edges (Figure S2). All aberrantly expressed genes in the top module were included to perform GO functional enrichment and KEGG pathway analysis. According to the enrichment results of genes in the top module were primarily enriched in the cell cycle, DNA replication and p53 signaling pathways (Figure S3).
Table 2
Gene | Degree | Betweenness | Closeness |
---|---|---|---|
CDC20 | 51 | 270.05453 | 0.04576144 |
AURKB | 51 | 1034.8419 | 0.04596835 |
TOP2A | 51 | 319.67508 | 0.04576144 |
KIF11 | 51 | 731.74243 | 0.045812994 |
BUB1 | 50 | 117.515045 | 0.045727137 |
CCNB2 | 49 | 275.38492 | 0.045710005 |
RRM2 | 49 | 111.97493 | 0.045607477 |
CDC45 | 49 | 69.68993 | 0.045590434 |
MCM2 | 48 | 61.376442 | 0.04550541 |
KIF20A | 48 | 157.32498 | 0.045692883 |
UBE2C | 48 | 606.3233 | 0.045727137 |
CDC6 | 48 | 50.60078 | 0.04557340 |
CDCA8 | 47 | 66.23398 | 0.045675777 |
MCM3 | 47 | 114.58486 | 0.045471486 |
NUSAP1 | 47 | 66.23398 | 0.045675777 |
CENPF | 47 | 263.64554 | 0.045675777 |
MELK | 46 | 61.64553 | 0.04565868 |
PBK | 46 | 664.2733 | 0.045812994 |
MKI67 | 46 | 837.691 | 0.04589917 |
TYMS | 46 | 123.92797 | 0.0454207 |
DEG, differentially expressed genes; PPI, protein-protein interaction.
Prediction of TFs and establishment of a miRNA-target gene-TF regulatory network
We used miRTarBase, a miRNA-target interaction database that has been experimentally validated by reporter assay, western blot, microarray and next-generation sequencing experiments, to predict the upstream miRNAs of DEGs (16). In order to obtain more reliable prediction results, we selected only the miRNA-target interactions verified by the reporter assay for further analysis. A total of 162 miRNAs were identified. Next, we removed miRNAs whose expression levels were positively associated with that of DEGs, and we obtained 2 overlapping miRNAs that were downregulated, including let-7b-5p, predicted to regulate 1 gene which was high mobility group AT-hook 1 (HMGA1). RRM2, AURKB and enhancer of zeste 2 polycomb repressive complex 2 subunit (EZH2) were predicted to be regulated by let-7a-5p.
miRNAs and TFs are involved in transregulators that influence gene regulatory networks in different ways (17). Therefore, it is important to determine the function of miRNAs, which may allow us to better understand the interactions between TFs and miRNAs. We hypothesized that the miRNA-target gene-TF regulatory network might take part in RB pathogenesis. Therefore, we used the online tool TransmiR to predict TFs that might regulate the expression of miRNAs. Only the data with evidence from the literature was identified. As can be seen from Figure 5, let-7a-5p and let-7b-5p can be regulated by some of the same TFs. For example, interleukin 6 (IL6), argonaute RISC catalytic component 2 (EIF2C2) and tripartite motif containing 32 (TRIM32) regulate both let-7a-5p and let-7b-5p. However, lysine demethylase 2B (KDM2B) and signal transducer and activator of transcription 1 (STAT1) regulate let-7b-5p and let-7a-5p, respectively. The regulatory networks for let-7a-5p and let-7b-5p are shown in Figure 5.
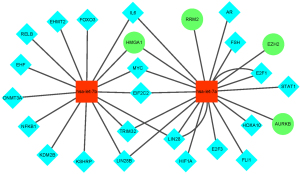
Verification of potential biomarker expression by RT-qPCR
Twenty hub genes and two miRNAs were verified. Then, the selected biomarkers, including let-7a-5p and let-7b-5p, were validated in RB cells and RPE cells using RT-qPCR analysis. Consistent with the prediction, the results showed that expression levels of the top 5 hub genes were higher in RB cells than in RPE cells. Furthermore, validation of the two miRNAs and their associated genes also confirmed the results from the GEO dataset (Figure 6).
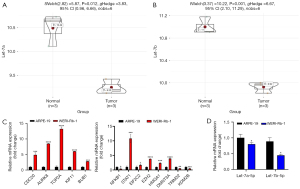
Discussion
RB is a rare pediatric cancer of the developing retina that occurs either as an inherited or as a sporadic disease. However, the precise pathological mechanisms of RB remain unclear. In addition, more effective therapies are still needed. Gene therapy takes therapeutic genes or disease-related genes as therapeutic targets to treat diseases and has a good application prospect in various tumor treatments. (18,19). Therefore, it is very important to investigate the underlying mechanism of RB development and progression. In recent years, researchers have widely used microarray technology to explore whether there are differences in the expression of various tumor genes, which provides a new method for identifying key genes and lays a foundation for further research on development and progression of tumors. Therefore, we assume that the differentially expressed mRNAs in RB compared to nontumor tissues might provide potential targets for RB treatments. In this research, we carried out RRA method to analyze the GSE111168, GSE110811, GSE125903 and GSE42321 datasets, and total 180 DEGs were identified. We then conducted BP, CC and MF enrichment analysis for these DEGs. These DEGs were primarily enriched in the regulation of chromosome segregation (ontology: BP), spindle (ontology: CC), and DNA replication origin binding (ontology: MF). These results suggest that these DEGs are involved in the cell cycle of RB cells. We can see from the results of KEGG pathway analysis that these DEGs are mainly enriched in the following top five pathways: pyrimidine metabolism, DNA replication, homologous recombination, Fanconi anemia pathway and cell cycle.
We also identified 20 hub genes, and the top 5 were CDC20, AURKB, TOP2A, KIF11, and BUB1. Among these DEGs, certain genes have previously been reported to be involved in tumorigenesis of various cancers. High expression of BUB1 and CDC20 in tumors was significantly associated with poorer overall survival in pancreatic ductal adenocarcinoma (20). Moreover, knockdown of CDC20 can be used for therapeutic benefit and represents an effective adjuvant anticancer treatment to eliminate CSCs during prostate cancer progression (21). Aurora B, a member of the Aurora family of serine/threonine protein kinases, plays a crucial role in chromosome segregation. Previous studies have shown that it regulates nearly every stage of cell division, including chromosome condensation, chromosome biorientation, spindle checkpoint formation, chromosome segregation, and cytokinesis (22,23). Aurora B has been reported to induce EMT to promote breast cancer metastasis through the OCT4/AKT/GSK3β/Snail1 signaling pathway. (24). Wu et al. showed that AURKB silencing promotes apoptosis of osteosarcoma 143B cells through unc-51 like autophagy activating kinase 1 (ULK1) phosphorylation-induced autophagy (25). Recently, it was revealed that AURKB plays an important role in the regulation of mitosis and is gaining prominence as a therapeutic target in RB (26). A previous study overexpressed TOP2A in bladder urothelial carcinoma (BLCA) and concluded that TOP2A represents a diagnostic marker of BLCA (27). The analysis also predicted RRM2 as a vital factor associated with RB prognosis. Ribonucleotide reductase M2 subunit (RRM2) plays important roles in many vital cellular processes (28). Some studies have found that RRM2 is critical for DNA synthesis and DNA repair. Therefore, there is much evidence suggesting that RRM2 may be an effective target for tumor therapy. For instance, expression levels of RRM2 are high in breast cancer patients, and the higher the expression of RRM2 was, the worse the prognosis (29). Furthermore, Wilson et al. repressed RRM2 in ER-negative breast cancer cells, which led to RRM2 being confirmed as a potential treatment for breast cancer (30). In hepatocellular carcinoma, RRM2 was also reported to be a crucial diagnostic marker. Jin et al. revealed that overexpression of RRM2 promotes the proliferation of LUAD cells and that RRM2 is an independent prognostic factor for patients with lung cancer (31).
miRNAs are noncoding RNA molecules that play a crucial role in regulating a range of basic cellular processes. They may induce RNA silencing and act as post-DNA transcription regulators (32). miRNAs involve in a variety of physiological and pathological processes by regulating the expression of multiple downstream target genes. It has been reported that abnormal regulation of miRNAs may be associated with the progression of RB. miR-146a has been reported to play a key role in proliferation and metastasis by inhibiting NOVA alternative splicing regulator 1 (NOVA1) (33). Many miRNAs have been reported to be abnormally expressed in RB, like miR-192, miR-34a and miR-376a (34-37). In RB, miR-188-5p promotes epithelial–mesenchymal transition by targeting DNA binding 4 via Wnt/β–catenin signaling pathway (38). This study screened DEMs in RB samples in comparison to nontumor retina samples. Then, a miRNA target gene regulatory network was established based on the DEMs and their overlaps among the DEGs. Two key DEMs, let-7a and let-7b, which were downregulated in RB samples in comparison to noncancer retina samples, were identified. Let-7a was reported to be associated with type 2 diabetes, asthma, and breast cancer (39-41). Let-7a has been reported that it acts as a tumor suppressor in hepatocellular carcinoma (42). The underlying mechanism of let-7a’s role in breast cancer may relate to a complicated network of solute carrier family 2 member 12 (GLUT12) (43). Therefore, we assume that let-7a may be an important factor in tumor progression, including RB. Some studies have reported that let-7b is involved in the development of multiple cancers, including ovarian cancer (44), prostate cancer (45), and breast cancer (46). Let-7b has been found to inhibit cell proliferation via upregulation of p21 in hepatocellular carcinoma (47). However, whether let-7a and let-7b expressed differently in RB has not been verified in previous studies. Thus, RT-qPCR was carried out to confirm the results of bioinformatics analysis and showed that expression of the miRNAs and key genes was consistent with our analysis. However, our current study only existed to verify and built some possible regulatory networks, which needs to be confirmed by subsequent and more detailed basic experiments. Due to the complexity of crosstalk of miRNA, TF and hub genes, the regulatory relationship between them needs to be studied very carefully. Furthermore, the underlying regulatory patterns between them may contribute to the discovery of new molecular targets for RB diagnosis and treatment.
Conclusions
We constructed some miRNA-gene-TFs regulatory networks by analyzing bioinformatics data, and found that let-7a and let-7b may serve as markers for the treatment and diagnosis of RB.
Acknowledgments
We sincerely appreciate the valuable advice and support from Dr. Xu Wang.
Funding: This work was supported by National Natural Science Foundation of China (No. 81770933) and National Natural Science Foundation of China (No. 81770964).
Footnote
Reporting Checklist: The authors have completed the REMARK reporting checklist. Available at https://tcr.amegroups.com/article/view/10.21037/tcr-21-1748/rc
Data Sharing Statement: Available at https://tcr.amegroups.com/article/view/10.21037/tcr-21-1748/dss
Peer Review File: Available at https://tcr.amegroups.com/article/view/10.21037/tcr-21-1748/prf
Conflicts of Interest: All authors have completed the ICMJE uniform disclosure form (available at https://tcr.amegroups.com/article/view/10.21037/tcr-21-1748/coif). The authors have no conflicts of interest to declare.
Ethical Statement: The authors are accountable for all aspects of the work in ensuring that questions related to the accuracy or integrity of any part of the work are appropriately investigated and resolved. The study was conducted in accordance with the Declaration of Helsinki (as revised in 2013).
Open Access Statement: This is an Open Access article distributed in accordance with the Creative Commons Attribution-NonCommercial-NoDerivs 4.0 International License (CC BY-NC-ND 4.0), which permits the non-commercial replication and distribution of the article with the strict proviso that no changes or edits are made and the original work is properly cited (including links to both the formal publication through the relevant DOI and the license). See: https://creativecommons.org/licenses/by-nc-nd/4.0/.
References
- Ortiz MV, Dunkel IJ. Retinoblastoma. J Child Neurol 2016;31:227-36. [Crossref] [PubMed]
- Yang J, Dang Y, Zhu Y, et al. Diffuse anterior retinoblastoma: current concepts. Onco Targets Ther 2015;8:1815-21. [PubMed]
- Rao R, Honavar SG. Retinoblastoma. Indian J Pediatr 2017;84:937-44. [Crossref] [PubMed]
- Abramson DH, Shields CL, Munier FL, et al. Treatment of Retinoblastoma in 2015: Agreement and Disagreement. JAMA Ophthalmol 2015;133:1341-7. [Crossref] [PubMed]
- Liu B, Li J, Cairns MJ. Identifying miRNAs, targets and functions. Brief Bioinform 2014;15:1-19. [Crossref] [PubMed]
- Bartel DP. MicroRNAs: genomics, biogenesis, mechanism, and function. Cell 2004;116:281-97. [Crossref] [PubMed]
- Acunzo M, Romano G, Wernicke D, et al. MicroRNA and cancer--a brief overview. Adv Biol Regul 2015;57:1-9. [Crossref] [PubMed]
- Zhao D, Cui Z. MicroRNA-361-3p regulates retinoblastoma cell proliferation and stemness by targeting hedgehog signaling. Exp Ther Med 2019;17:1154-62. [PubMed]
- Wang L, Wang L, Li L, et al. MicroRNA-330 is downregulated in retinoblastoma and suppresses cell viability and invasion by directly targeting ROCK1. Mol Med Rep 2019;20:3440-7. [Crossref] [PubMed]
- Zhang Y, Wang X, Zhao Y. MicroRNA-874 prohibits the proliferation and invasion of retinoblastoma cells by directly targeting metadherin. Mol Med Rep 2018;18:3099-105. [Crossref] [PubMed]
- Xiu C, Song R, Jiang J. TUG1 promotes retinoblastoma progression by sponging miR-516b-5p to upregulate H6PD expression. Transl Cancer Res 2021;10:738-47. [Crossref] [PubMed]
- Shi K, Zhu X, Wu J, et al. Centromere protein E as a novel biomarker and potential therapeutic target for retinoblastoma. Bioengineered 2021;12:5950-70. [Crossref] [PubMed]
- Wu N, Chen S, Luo Q, et al. Kruppel-like factor 2 acts as a tumor suppressor in human retinoblastoma. Exp Eye Res 2022;216:108955. [Crossref] [PubMed]
- Gao X, Chen Y, Chen M, et al. Identification of key candidate genes and biological pathways in bladder cancer. PeerJ 2018;6:e6036. [Crossref] [PubMed]
- Tang Y, Li M, Wang J, et al. CytoNCA: a cytoscape plugin for centrality analysis and evaluation of protein interaction networks. Biosystems 2015;127:67-72. [Crossref] [PubMed]
- Chou CH, Shrestha S, Yang CD, et al. miRTarBase update 2018: a resource for experimentally validated microRNA-target interactions. Nucleic Acids Res 2018;46:D296-302. [Crossref] [PubMed]
- Zhao Q, Liu H, Yao C, et al. Effect of Dynamic Interaction between microRNA and Transcription Factor on Gene Expression. Biomed Res Int 2016;2016:2676282. [Crossref] [PubMed]
- Lumbroso-Le Rouic L. Retinoblastoma Rev Prat 2017;67:888-91. [PubMed]
- Philiponnet A, Grange JD, Baggetto LG. Application of gene therapy to oncologic ophthalmology. J Fr Ophtalmol 2014;37:155-65. [Crossref] [PubMed]
- Dong S, Huang F, Zhang H, et al. Overexpression of BUB1B, CCNA2, CDC20, and CDK1 in tumor tissues predicts poor survival in pancreatic ductal adenocarcinoma. Biosci Rep 2019;39:BSR20182306. [Crossref] [PubMed]
- Zhang Q, Huang H, Liu A, et al. Cell division cycle 20 (CDC20) drives prostate cancer progression via stabilization of β-catenin in cancer stem-like cells. EBioMedicine 2019;42:397-407. [Crossref] [PubMed]
- Portella G, Passaro C, Chieffi P. Aurora B: a new prognostic marker and therapeutic target in cancer. Curr Med Chem 2011;18:482-96. [Crossref] [PubMed]
- Krenn V, Musacchio A. The Aurora B Kinase in Chromosome Bi-Orientation and Spindle Checkpoint Signaling. Front Oncol 2015;5:225. [Crossref] [PubMed]
- Zhang J, Lin X, Wu L, et al. Aurora B induces epithelial-mesenchymal transition by stabilizing Snail1 to promote basal-like breast cancer metastasis. Oncogene 2020;39:2550-67. [Crossref] [PubMed]
- Wu X, Liu J, Song H, et al. Aurora kinase-B silencing promotes apoptosis of osteosarcoma 143B cells by ULK1 phosphorylation-induced autophagy. Nan Fang Yi Ke Da Xue Xue Bao 2020;40:1273-9. [PubMed]
- Borah NA, Sradhanjali S, Barik MR, et al. Aurora Kinase B Expression, Its Regulation and Therapeutic Targeting in Human Retinoblastoma. Invest Ophthalmol Vis Sci 2021;62:16. [Crossref] [PubMed]
- Zeng S, Liu A, Dai L, et al. Prognostic value of TOP2A in bladder urothelial carcinoma and potential molecular mechanisms. BMC Cancer 2019;19:604. [Crossref] [PubMed]
- Nordlund P, Reichard P. Ribonucleotide reductases. Annu Rev Biochem 2006;75:681-706. [Crossref] [PubMed]
- Chen WX, Yang LG, Xu LY, et al. Bioinformatics analysis revealing prognostic significance of RRM2 gene in breast cancer. Biosci Rep 2019;39:BSR20182062. [Crossref] [PubMed]
- Wilson EA, Sultana N, Shah KN, et al. Molecular Targeting of RRM2, NF-κB, and Mutant TP53 for the Treatment of Triple-Negative Breast Cancer. Mol Cancer Ther 2021;20:655-64. [Crossref] [PubMed]
- Jin CY, Du L, Nuerlan AH, et al. High expression of RRM2 as an independent predictive factor of poor prognosis in patients with lung adenocarcinoma. Aging (Albany NY) 2020;13:3518-35. [Crossref] [PubMed]
- Catalanotto C, Cogoni C, Zardo G. MicroRNA in Control of Gene Expression: An Overview of Nuclear Functions. Int J Mol Sci 2016; [Crossref] [PubMed]
- Liu XM, Li XF, Li JC. MiR-146a functions as a potential tumor suppressor in retinoblastoma by negatively regulate neuro-oncological ventral antigen-1. Kaohsiung J Med Sci 2021;37:286-93. [Crossref] [PubMed]
- Castro-Magdonel BE, Orjuela M, Camacho J, et al. miRNome landscape analysis reveals a 30 miRNA core in retinoblastoma. BMC Cancer 2017;17:458. [Crossref] [PubMed]
- Feng S, Cong S, Zhang X, et al. MicroRNA-192 targeting retinoblastoma 1 inhibits cell proliferation and induces cell apoptosis in lung cancer cells. Nucleic Acids Res 2011;39:6669-78. [Crossref] [PubMed]
- Dalgard CL, Gonzalez M, deNiro JE, et al. Differential microRNA-34a expression and tumor suppressor function in retinoblastoma cells. Invest Ophthalmol Vis Sci 2009;50:4542-51. [Crossref] [PubMed]
- Zhang Y, Wu JH, Han F, et al. Arsenic trioxide induced apoptosis in retinoblastoma cells by abnormal expression of microRNA-376a. Neoplasma 2013;60:247-53. [Crossref] [PubMed]
- Yang M, Li Y, Wei W. MicroRNA-188-5p Promotes Epithelial-Mesenchymal Transition by Targeting ID4 Through Wnt/β-catenin Signaling in Retinoblastoma. Onco Targets Ther 2019;12:10251-62. [Crossref] [PubMed]
- Uhr K, Prager-van der Smissen WJC, Heine AAJ, et al. MicroRNAs as possible indicators of drug sensitivity in breast cancer cell lines. PLoS One 2019;14:e0216400. [Crossref] [PubMed]
- Huang Z, Cao Y, Zhou M, et al. Hsa_circ_0005519 increases IL-13/IL-6 by regulating hsa-let-7a-5p in CD4+ T cells to affect asthma. Clin Exp Allergy 2019;49:1116-27. [Crossref] [PubMed]
- Mononen N, Lyytikäinen LP, Seppälä I, et al. Whole blood microRNA levels associate with glycemic status and correlate with target mRNAs in pathways important to type 2 diabetes. Sci Rep 2019;9:8887. [Crossref] [PubMed]
- Mostafa SM, Gamal-Eldeen AM, Maksoud NAE, et al. Epigallocatechin gallate-capped gold nanoparticles enhanced the tumor suppressors let-7a and miR-34a in hepatocellular carcinoma cells. An Acad Bras Cienc 2020;92:e20200574. [Crossref] [PubMed]
- Shi Y, Zhang Y, Ran F, et al. Let-7a-5p inhibits triple-negative breast tumor growth and metastasis through GLUT12-mediated warburg effect. Cancer Lett 2020;495:53-65. [Crossref] [PubMed]
- Deng L, Huang S, Chen B, et al. Tumor-Linked Macrophages Promote HCC Development by Mediating the CCAT1/Let-7b/HMGA2 Signaling Pathway. Onco Targets Ther 2020;13:12829-43. [Crossref] [PubMed]
- Rong J, Xu L, Hu Y, et al. Inhibition of let-7b-5p contributes to an anti-tumorigenic macrophage phenotype through the SOCS1/STAT pathway in prostate cancer. Cancer Cell Int 2020;20:470. [Crossref] [PubMed]
- Zou X, Xia T, Li M, et al. MicroRNA profiling in serum: Potential signatures for breast cancer diagnosis. Cancer Biomark 2021;30:41-53. [Crossref] [PubMed]
- Hui L, Zheng F, Bo Y, et al. MicroRNA let-7b inhibits cell proliferation via upregulation of p21 in hepatocellular carcinoma. Cell Biosci 2020;10:83. [Crossref] [PubMed]