NF2 is a candidate diagnosis, prognostic, and immunotherapeutic biomarker: a systematic pan-cancer analysis
Highlight box
Key findings
• Neurofibromin 2 (NF2) is upregulated in various tumors, and its expression is highly associated with the tumor immune microenvironment. NF2 also has a potential value for predicting the efficacy of immune checkpoint inhibitors therapy.
What is known and what is new?
• The abnormal expression of NF2 contributes to tumorigenesis and progression.
• NF2 is significantly correlated with immune cells and genes.
What is the implication, and what should change now?
• NF2 can be used as diagnostic, prognostic, and immunotherapeutic biomarker for pan-cancer.
Introduction
Cancer mortality is rapidly growing in every country of the world. Almost 10 million people die of cancer every year. Unfortunately, there is still no complete cure for cancer (1). Recently, the tumor immune microenvironment (TIME) has been proven essential in tumor progression. Continued detailed analysis of TIME enables the identification of potential biomarkers for clinic benefits, and multiple novel immune checkpoints have evolved into practical cancer therapy targets (2). With the generation and continuous improvement of public databases like The Cancer Genome Atlas (TCGA), it is possible to explore the occurrence and progression mechanisms of cancer through pan-cancer analysis, which may help to find potential biomarkers and immunotherapeutic targets and provide new insights for diagnosis, treatment, and prognosis of cancer (3).
The human neurofibromin 2 (NF2) is a protein-coding gene expressing a protein called Merlin (4). Merlin is a typical tumor suppressor that is known for its ability to induce contact-dependent growth inhibition. Like other multifunctional proteins, merlin plays an important role in maintaining cell stability, controlling cell proliferation, and promoting tissue and organ differentiation by interacting with cell surface proteins, proteins involved in cytoskeletal dynamics, and proteins involved in regulating ion transport (5). NF2’s malfunction results in aberrant cell proliferation causing tumorigenesis by abrogating anti-tumor immunity and modulating primary cell proliferation signaling pathways, including Hippo, WNT/β-catenin, TGF-β, receptor tyrosine kinase (RTK), and Notch pathways (6-10). Studies have manifested that mutation of NF2 is recognized in malignant tumors, such as breast cancer (BRCA), mesothelioma (MESO), prostate cancer, and glioma, and may lead to poor prognosis (5,11). In addition, some clinical trials have been conducted to explore the prognostic impact of NF2 mutations on solid tumor patients (12,13).
The occurrence of NF2 mutations can affect tumor progression or prognosis. However, there is still a lack of larger researches to evaluate the inactivation of NF2 in cancer. We still need to better understand the role of NF2 in tumor development and progression. The fact that the absence of NF2 mainly leads to the formation of tumors in Schwann, meningeal, and ependymal cells, while other cell types, although commonly expressing merlin in normal tissues, do not undergo transformation, indicating that tissue specific molecular background and tumor microenvironment dependence need further clarification (7). There is still no comprehensive pan-cancer study of NF2 conducted. Therefore, we retrieved multiple databases including TCGA, Human Protein Atlas (HPA), Cancer Cell Line Encyclopedia (CCLE), Genotype-Tissue Expression Project (GTEx), and cBioPortal to extract corresponding data for subsequent analysis. With the comparison and analysis of NF2 expression in various types of tumors, we studied associations between NF2 with immune infiltration levels, immune-related genes expression, microsatellite instability (MSI), and tumor mutational burden (TMB). Besides, we also investigated gene set enrichment analysis (GSEA) and gene set variation analysis (GSVA) to explore the biological functions of NF2 in pan-cancer. The results showed that NF2 might be a valuable diagnostic, prognostic, and immunological biomarker of pan-cancer. The combination of molecular therapies around NF2 may become a successful treatment method. Through further research and better understanding of NF2 related molecular cross signaling, may determine the optimal treatment strategy and achieve personalized precision medicine. We present this article in accordance with the REMARK reporting checklist (available at https://tcr.amegroups.com/article/view/10.21037/tcr-23-1179/rc).
Methods
Differential expression analysis
The gene expression RNA sequencing and phenotype profiles of TCGA were downloaded from Xena (https://xena.ucsc.edu/), an online platform containing private and public clinical/phenotype data, including tumor samples and corresponding normal samples (14). Data from CCLE were downloaded from DepMap Portal (https://depmap.org/portal/). GTEx (https://www.gtexportal.org/home/) is a comprehensive public dataset to study tissue-specific gene expression and regulation (15). We downloaded 31 different tissues’ gene expression data from GTEx. The differential expression gene analyses between tumor and normal samples were performed by Log2 transformation and t-tests. P<0.05 was the standard for identifying the expression difference between tumor and normal tissues. R software (Version 4.0.2; https://www.Rproject.org) was used for statistical analysis, and the R package “ggplot2” was used for drawing box plots.
HPA
HPA (https://www.proteinatlas.org/) is a human proteome atlas database that maps human proteins by integrating various omics technologies, such as systems biology, antibody-based imaging, and transcriptomics (16). To evaluate differences in NF2 expression at the protein level, we downloaded and analyzed immunohistochemistry images of normal and tumor tissues in six types of cancer, including cervical squamous cell carcinoma and endocervical adenocarcinoma (CESC), pancreatic adenocarcinoma (PAAD), prostate adenocarcinoma (PRAD), liver hepatocellular carcinoma (LIHC), lung squamous cell carcinoma (LUSC), and testicular germ cell tumors (TGCT). The study was conducted in accordance with the Declaration of Helsinki (as revised in 2013).
Diagnosis and survival analysis
Data from TCGA samples regarding tumor node metastasis (TNM) stage and clinical phenotype were analyzed by R packages “ggplot2” to find their correlation with NF2 expression. To assess the accuracy of NF2 for disease diagnosis, we performed the receiver operating characteristic (ROC) curve analysis using the R package “pROC” (17). Area under the curve (AUC) of ROC was classified into three ranges: high diagnostic accuracy (AUC ≥0.9), relative diagnostic accuracy (0.9< AUC ≤0.7), and low diagnostic accuracy (0.7< AUC ≤0.5). The survival data from TCGA were used to analyze the relationship between NF2 and prognosis in pan-cancer. Overall survival (OS), disease-specific survival (DSS), and progression-free interval (PFI) were considered as the prognosis indicators. The Kaplan-Meier method and log-rank test were used to conduct survival analyses. Survival curves were drawn by the R packages “survival” and “survminer”. Furthermore, R packages “Forestplot” were used to perform cox proportional hazards analysis between NF2 expression and survival.
Immunological correlation analysis
The relative scores of immunocyte abundances were analyzed by CIBERSORT (https://cibersortx.stanford.edu/), a metagene tool to estimate cell abundances through gene expression data. The correlations between NF2 and immunocytes in pan-cancer were assessed by R packages “ggplot2”, “ggpubr”, and “ggExtra”. Estimation of Stromal and Immune Cells in Malignant Tumor Tissues Using Expression Data (ESTIMATE) algorithm is able to use transcriptional profiles of cancer samples to infer the abundance of tumor cells, immune cells, and stromal cells in the tumor microenvironment (18). The relationship between immune scores and NF2 expression in each type of cancer was analyzed by R packages “estimate” and “Limma”. Furthermore, the co-expression analysis of NF2 and immune-related genes, including chemokine, immunoinhibitor, immunostimulator, chemokine receptors, and MHC genes was performed. The R package “Limma” was used to perform the analysis.
TMB is a quantitative genomic biomarker that quantifies the total number of mutations in a tumor specimen to evaluate response to immunotherapy (19). MSI is proven to correlate with better survival outcomes (20). TMB and MSI analyses were performed by Sangerbox, a platform that integrated Gene Expression Omnibus, TCGA, International Cancer Genome Consortium, and other databases for differential analysis and customizable interactive analysis (21).
The biological function enrichment analysis
GSEA and GSVA were conducted to explore biological function enrichment analyses of NF2 in each tumor. Functional analysis was performed by R packages “limma”, “org.Hs.eg.db”, “clusterProfiler”, and “enrichplot”. The GSVA gene set “h.all.v7.5.1” was from the MSigDB database (https://www.gsea-msigdb.org/). The correlation of NF2 expression with the Hallmark pathway in each tumor was analyzed using R package “GSVA”.
Alteration and RNA modification analysis
Alterations of NF2 in pan-cancer were calculated by the cBioPortal platform (http://www.cbioportal.org/). Then, we extracted the expression of NF2 as well as 44 genes involved in the three categories of RNA modification (m6A, m5C, m1A) from the TCGA database. After filtering all normal samples, all genes were performed a log2 transformation to each expression value, and next, we calculated correlations between NF2 and 44 RNA modification genes.
Statistical analysis
The statistical analysis was computed by R (version 4.2.1) in this study. These results were considered as statistically significant at P<0.05.
Results
Differential expression of NF2 between tumor and normal tissue samples
By using the GTEx datasets, we analyzed the expression levels of NF2 gene across different physiologic tissues (Figure 1A). It can be seen that NF2 expression level in testis was the highest among all of the tissues, while most other normal tissues expressed low level of NF2. The NF2 expression levels across different cell lines are presented in Figure 1B, derived from the CCLE data set. Compared with the GTEx analysis, it is evident that the expression levels of NF2 are generally increased in different tissues of cancer cell lines.
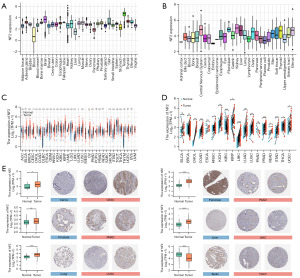
Next, through the GTEx-TCGA data of Xena, we compared the expression of NF2 gene in normal tissues and tumor samples (Figure 1C). Excluding the cancers with no or few normal samples, the expression of NF2 in a total of 25 cancers was statistically different from normal tissues. NF2 was upregulated in BRCA, cervical squamous cell carcinoma and CESC, cholangiocarcinoma (CHOL), colon adenocarcinoma (COAD), diffuse large B-cell lymphoma (DLBC), esophageal carcinoma (ESCA), head and neck squamous cell carcinoma (HNSC), kidney chromophobe (KICH), kidney renal papillary cell carcinoma (KIRP), acute myeloid leukemia (LAML), brain lower grade glioma (LGG), LIHC, lung adenocarcinoma (LUAD), LUSC, ovarian serous cystadenocarcinoma (OV), PAAD, prostate adenocarcinoma (PRAD), skin cutaneous melanoma (SKCM), stomach adenocarcinoma (STAD), thyroid carcinoma (THCA), thymoma (THYM). Contrary to the upregulated tumors, NF2 levels had low expression in adrenocortical carcinoma (ACC), glioblastoma multiforme (GBM), kidney renal clear cell carcinoma (KIRC), and TGCT. Besides, NF2 expressed no significant difference in bladder urothelial carcinoma (BLCA), pheochromocytoma and paraganglioma (PCPG), rectum adenocarcinoma (READ), uterine corpus endometrial carcinoma (UCEC), and uterine carcinosarcoma (UCS). For paired tumors and corresponding normal samples, NF2 expression was upregulated in 11 types of tumors (Figure 1D). These results suggested that NF2 may have a potentially crucial role in cancer diagnosis.
Furthermore, in order to analyze the expression of NF2 at protein level, we collected immunohistochemistry images from the HPA dataset and compared the images with the TCGA expression data (Figure 1E). The analysis indicated that the results from two databases were consistent.
Prognostic significance of NF2 in pan-cancer
To elucidate the relationship between NF2 expression level and prognosis, we performed survival association analysis in pan-cancer, including OS, DSS, and PFI. Cox proportional hazards model analysis showed that NF2 expression levels were associated with OS in ACC (P<0.001), LIHC (P=0.04), KIRC (P=0.0017), KIRP (P=0.009), LGG (P=0.02), PCPG (P=0.04). NF2 was a low-risk factor in KIRC, KIRP, LGG, and PCPG, while it was a high-risk factor in other types of cancer (Figure 2A).
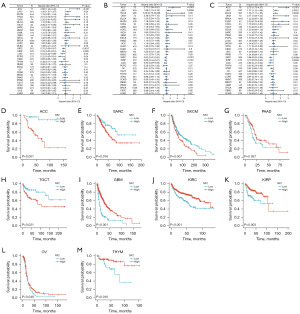
Next, Figure 2B reveals the NF2 expression significantly correlated with DSS in ACC (P<0.001), LIHC (P=0.0056), KIRC (P<0.001), KIRP (P<0.001) and LGG (P=0.01). Regarding associations between NF2 expression and PFI, forest plots showed associations in ACC (P<0.001), LIHC (P=0.027), BLCA (P=0.0032), UVM (P=0.04), KIRC (P<0.001), KIRP (P=0.0055), LGG (P=0.02) and THYM (P=0.02) (Figure 2C).
Kaplan-Meier survival analysis demonstrated that high NF2 expression was associated with better OS among patients with GBM, KIRC, KIRP, OV, PAAD, and THYM. On the contrary, in ACC, sarcoma (SARC), SKCM and TGCT, high expression of NF2 was associated with poor OS (Figure 2D-2M). In addition, Kaplan-Meier survival analysis suggested the correlation between low NF2 expression level and better DSS in ACC, SARC, and SKCM. At the same time, patients in PAAD, GBM, KIRC, MESO, KIRP, THYM, and STAD showed the opposite (Figure S1). Low expression of NF2 was associated with poor PFI in patients with ESCA, GBM, KIRC, KIRP, and LGG (Figure S2).
Diagnosis value of NF2 in pan-cancer
By analyzing the expression of NF2 in different stages of tumors, we found that the expression of NF2 increased significantly in the early stages of 9 cancers, including CHOL, COAD, HNSC, LIHC, LUSC, KIRP, oral squamous cell carcinoma (OSCC), PRAD and STAD (Figure 3A). The analysis indicated that NF2 might have a potential clinical value in the early diagnosis of the tumors mentioned above. Furthermore, the ROC curve demonstrated the performance of the gene signature for diagnostic accuracy. Results were divided into three groups: high diagnostic accuracy with the AUC >0.9, relative diagnostic accuracy (0.9< AUC <0.7), or low diagnostic accuracy (0.7< AUC <0.5). As Figure 3B shows, there were 4 types of cancer with high diagnostic accuracy, 9 types with relative diagnostic accuracy 14 types with low diagnostic accuracy. By the way, NF2 expression had the best diagnostic accuracy in CHOL, with 1.0 AUC achieved.
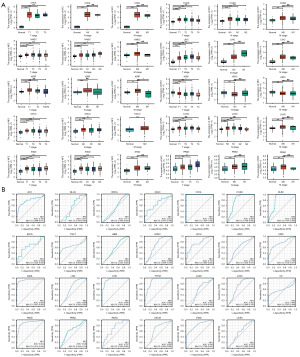
Relationship between NF2 expression levels and tumor immune infiltration
Tumor immune cell infiltration is a critical factor in tumor progression and immune escape. The analysis by CIBERSORT revealed the correlation between NF2 expression and immune cell infiltration. The results showed that NF2 expression level had a significant association with immune cell infiltration of a variety of cancers. In particular, NF2 expression was associated with Th2 cells in 19 types of cancer, pDc cells in 18 types, and cytotoxic cells in 16 types (Figure 4A). We further explored the relationship between TIME and NF2 expression by the ESTIMATE algorithm. The immune cell scores were calculated in 33 types of tumors. The results revealed that NF2 expression was significantly negatively correlated with immune score in GBM, LGG, CESC, BRCA, ACC, ESCA, SARC, PRAD, UCEC, KIRC, LUSC, THYM, THCA, and SKCM, and contrarily in PAAD, COAD, DLBC, and UVM (Figure 4B).
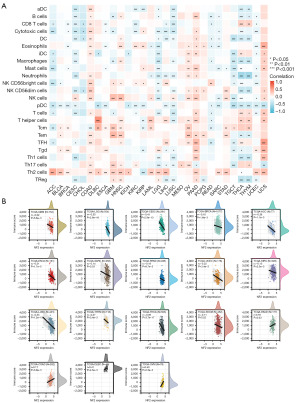
Relationship between NF2 expression with immune-related genes
In order to explore the relationship between NF2 expression and immune-related genes, gene co-expression analyses were performed between NF2 expression and immune-related genes, including chemokines, chemokine receptors, immunostimulators, immunoinhibitors, and major histocompatibility complex (MHC) genes (Figure 5A-5E). It can be seen that NF2 was positively correlated with the expression of most immune-related genes, especially with VEGFR, TGFBR1, IL10RB, TAP1, TAP2, CD276, PVR, and IL6R. In addition, immune-related genes significantly correlated with NF2 expression in DLBC, OV, PAAD, and UVM.
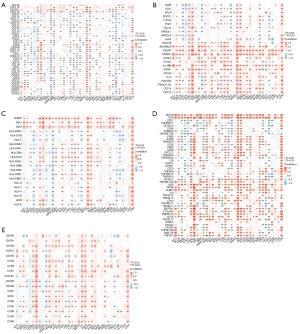
NF2 mutations and correlation of NF2 with RNA modification genes
The gene alteration of NF2 in pan-cancer was analyzed through the cBioPortal platform. The TCGA pan-cancer atlas studies, covering 10,967 patients from 32 types of cancer, were used for gene alteration analysis. cBioPortal revealed that mutation was the largest proportion of alteration in NF2 and occurred most frequently in pleural MESO (22.99%), CHOL (5.56%), and endometrial cancer (3.75%) (Figure 6A). The integration data of the NF2 mutation sites are shown in Figure 6B. Besides, we further analyzed the correlation between NF2 expression and RNA modification genes, including m6A/m1A/m5C regulated genes (Figure 6C-6E). The result indicated that the expression of NF2 was positively correlated with most m6A/m1A/m5C regulated genes, especially in DLBC and OV.
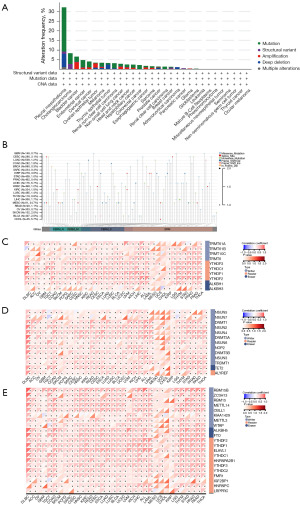
Biological functions analysis of NF2 in pan-cancer
To investigate the potential biological pathways correlated with NF2, we conducted GSEA and GSVA in pan-cancer. The visualized results of GO and KEGG pathway analysis are shown in Figure 7A. The enriched pathways mainly focused on the mechanism of immune regulation, such as regulation of lymphocyte activation (BLCA, DLBC, COAD, LIHC), immune response regulation signaling pathway (DLBC, GBM, LIHC), and intestinal immune network for immunoglobulin A production (COAD). Besides, NF2 was also closely associated with MAPK signaling pathways, pathways in cancer, and neuroactive ligand-receptor interaction in various tumors. For GSVA results, the top 5 hallmark pathways significantly positively and negatively associated with NF2 expression in various tumors are presented in Figure 7B. NF2 expression has the highest positive correlation with WNT/β-catenin pathway and the highest negative correlation with interferon-α response. In summary, these results suggested that NF2 may play a critical role in tumor immunity regulation, tumorigenesis, and tumor progression.
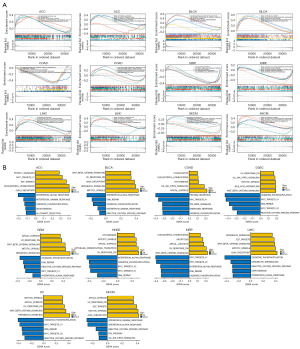
TMB and MSI analysis of NF2 expression in pan-cancer
TMB and MSI are closely related to the sensitivity of immune checkpoint inhibitors (ICIs), such as programmed cell death protein 1 (PD-1)/programmed cell death ligand 1 (PD-L1). By Spearman rank correlation coefficient, we calculated the TMB and MSI of each tumor sample and analyzed the correlation between NF2 with TMB and MSI in 33 tumors. The results revealed that NF2 was positively correlated with TMB in ACC, BRCA, COAD, LAML, SARC, STAD, UCEC, and conversely in THCA and UVM (Figure 8A). NF2 expression was positively correlated with MSI in 7 tumors, including BLCA, CESC, COAD, KICH, SARC, STAD, and UCEC. Additionally, NF2 was negatively correlated with MSI in DLBC, HNSC, PRAD, and THCA (Figure 8B). The above results showed that high expression of NF2 is extensively associated with tumor immunity.
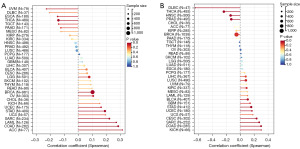
Discussion
The activation of developmental signaling pathways is a similarity between embryonic tissue growth and tumorigenesis. WNT/β, TGF-β, RTK, Hippo, and Notch pathways are key participants in normal developmental biology (22). Loss of restriction on developmental related signaling pathways can cause damage to tissue development, manifested as developmental syndrome. Similarly, by promoting cell proliferation, migration, and stem cell like phenotype, the activity imbalance of these pathways can promote cancer occurrence and progression. As a spatiotemporal-dependent manner, NF2 contributes to either activation or inhibition of developmental pathways in order to maintain cell integrity, tissue organization, and adequate different stages of organism development. The abnormality of NF2 deregulates the activity of these pathways and promotes carcinogenesis and cancer progression. However, NF2 has not received much attention in cancers (11). In our study, there are significant differences in NF2 expression of 25 cancer types compared with normal tissues. Among them, NF2 expression levels were upregulated in 21 tumors, such as BRCA, CESC, CHOL, and COAD. Although some cancer analyses could not be conducted due to the lack of normal tissue data, they are still worth further exploration with accumulating datasets. For example, genetic inactivation of NF2 was confirmed as a frequent tumorigenic event in MESO (23). In paired samples analysis, NF2 was overexpressed in 11 cancer types, and IHC analysis confirmed the high-level expression of NF2 in most cancer types at the protein level. These results indicate the potential of NF2 as a tumor biomarker. To investigate the association between NF2 expression levels and prognosis, survival association analysis was performed using Kaplan-Meier survival curves for each type of cancer, including OS, DSS, and PFI. Combining these results, we found that high NF2 expression had a good prognosis in ESCA, LGG, PAAD, GBM, KIRC, MESO, KIRP, THYM, STAD, OV, and a poor prognosis in ACC, CHOL, LIHC, SARC, SKCM, and TGCT. Given the above, we also investigated the expression of NF2 in different TNM stages to explore the value of NF2 in early cancer screening. Based on the collected data marked with staging information, we found that NF2 expression had early elevations in 9 cancers. Besides, NF2 also showed a superior diagnosis value in the AUC of ROC curve. NF2 showed high diagnostic accuracy in 4 forms of cancer (AUC ≥0.9), including COAD, with 1.0 AUC achieved. Nine forms of cancer showed a relative diagnostic accuracy (0.9< AUC ≤0.7). To understand the relationship between NF2 expression level and cancer prognosis, we performed Cox proportional hazards model analysis and Kaplan-Meier survival curves, including OS, PFI, and DSS. All combined, high NF2 expression had a better prognosis in GBM, KIRC, KIRP, MESO, THYM, STAD, OV, ESCA, and LGG, and a worse prognosis in ACC, SARC, SKCM, BLCA, and CHOL. Overall, these findings suggest that NF2 has potential value in early diagnosing and assessing prognosis.
The occurrence and progression of cancer are closely related to its surrounding stroma (24). Cancer cells and inflammatory cells with their surrounding stroma constitute the TIME. Cells within the TIME are highly plastic, continuously changing their phenotypic and biological functions (25). Growing evidence suggests that TIME can be exploited to assess the response of tumor cells to immunotherapies (26). So, it is essential to understand the TIME status of patients to select the appropriate immunotherapy strategy. In the present study, we found that high expression of NF2 is associated with high expression of TH2 cells and the inhibition of pDC cells. In addition, in COAD, OV, and PAAD, high expression of NF2 is correlated with high expression of various immune cells. We also analyzed the correlation between NF2 expression and immune score calculated by the ESTIMATE algorithm. The results indicate that NF2 expression negatively correlates with immune cell infiltration in most cancers. The co-expression analysis unraveled a positive correlation between NF2 and immune-related genes, such as chemokines, immunosuppressive genes, immunostimulatory genes, MHC genes, and their receptors in most tumor types. We found that NF2 correlated with T cell exhaustion marker genes such as VEGFR, TGFBR1, KDR, IL-10RB, and PDCD1LG2 in pan-cancer (27-29). Previous studies prove that NF2 can affect the TGF-β signaling pathway, which regulates Treg cells, effector T cells, NK cells, macrophages, and multiple immune response processes (30). VEGFR plays a crucial role in tumor neo-angiogenesis and induces immunosuppression by modulating Treg cells, dendritic cells, cytotoxic T lymphocytes, and M2-like macrophages, resulting in tumor immune escape (31).
Additionally, our GSEA and GSVA results demonstrated the correlation of NF2 expression with immune regulatory functions, such as lymphocyte activation, immune response pathway, MAPK pathway, and WNT/β-catenin pathway. MAPK pathway has been shown to regulate inflammatory responses by inducing the expression of multiple cytokines, including interleukins and interferons (32-34). Wnt/β-catenin signaling pathway has been proven to regulate the differentiation and development of various immune cells, such as macrophages and B cells (35). In summary, these results indicate that NF2 expression level is involved in the regulation and activation of immune cells and is highly correlated with the TIME.
ICIs, such as PD-1/PD-L1 inhibitors and CTLA-4 inhibitors, have demonstrated lasting anti-tumor effects in the treatment of multiple cancer types (36). However, only a tiny percentage of patients could have long-term clinical benefits from ICI therapies, and ICIs may cause immune toxicities or even worsen tumor prognosis (37). TMB is a predictive biomarker for identifying patients with better survival upon ICI treatment (38). NF2 has a significantly positive correlation with TMB in ACC, BRCA, COAD, LAML, SARC, STAD, and UCEC. MSI is also a predictive biomarker of ICIs (39), and it has been confirmed as an independent predictor of clinical characteristics and prognosis in COAD (40). NF2 positively correlates with MSI in BLCA, CESC, COAD, KICH, SARC, STAD, and UCEC. Based on existing research and our findings, we speculated that patients with high NF2 expression might benefit from ICIs therapy in the cancers mentioned above.
RNA modification is a critical method of regulating gene expression at post-transcription (41). Aberrant RNA modifications promote the activation of multiple cancer phenotypes, such as stress adaptation, differentiation, invasion, and resistance to therapies (42). In our study, the results suggest a positive correlation between NF2 expression and major RNA modification genes of m6A, m5A, and m1C, such as METTL3, METTL14, and NSUN2. METTL3 and METTL14 complex was demonstrated as critical factor of cell proliferation (43). NSUN2 has been reported to be able to modulate MYC-dependent proliferation and stabilize oncogenic mRNAs (44,45). These results indicated that NF2 expression could affect RNA modification and may broaden ideas for anticancer epigenetic drugs.
In brief, our first pan-cancer analysis of NF2 confirms the differential expression of NF2 between tumor and normal tissues, and NF2 expression is correlated with TIME and clinical prognosis. Our findings identify NF2 as a potential early diagnostic biomarker and independent prognostic factor in pan-cancer. Different expression levels of NF2 will contribute to different prognostic outcomes, which still need further targeted analyses of NF2 in each type of cancer. Besides, the expression of NF2 is associated with TMB and MSI in a variety of cancers, suggesting that NF2 may be a potential biomarker for predicting the efficacy of ICI therapy.
However, this study has some limitations, which cannot be ignored. First, specific systematic biases may exist because the data used for analysis were derived from different databases (46). Second, although we found that the expression of NF2 is related to tumor diagnosis, diagnostic, and immunity, we could not prove the causal relationship. In addition, we found that even as a tumor suppressor, some patients still have poor prognosis when NF2 is highly expressed. This may be due to the heterogeneity and complexity of tumor occurrence and development, as well as the imbalance and crosstalk between various signaling pathways. However, since our research mainly focuses on bioinformatics analysis methods, we can only analyze whether there is a correlation between the expression levels of genes or proteins, but cannot judge whether there is a causal relationship between them. In the next work, we will continue to increase the in-depth study on the function of NF2 in the occurrence and metastasis of different cancers and conduct functional experiments on NF2.
Conclusions
In summary, NF2 is related to early diagnosis and prognosis of cancer patients and the immune infiltration in different cancers. The expression of NF2 is also associated with MSI, TMB, and RNA methylation genes in various cancers. Our study reveals that NF2 is a promising diagnosis, prognostic, and immunotherapeutic biomarker for many types of tumors. These findings may help elucidate the biological functions of NF2 in tumorigenesis and progression, ultimately impacting precision medicine and personalized immunotherapy in the future.
Acknowledgments
Funding: This study was supported by
Footnote
Reporting Checklist: The authors have completed the REMARK reporting checklist. Available at https://tcr.amegroups.com/article/view/10.21037/tcr-23-1179/rc
Peer Review File: Available at https://tcr.amegroups.com/article/view/10.21037/tcr-23-1179/prf
Conflicts of Interest: Both authors have completed the ICMJE uniform disclosure form (available at https://tcr.amegroups.com/article/view/10.21037/tcr-23-1179/coif). J.L. reports the funding from National Natural Science Foundation of China (82274103) and Shanghai Academic Research Leader (21XD1403400). The other author has no conflicts of interest to declare.
Ethical Statement: The authors are accountable for all aspects of the work in ensuring that questions related to the accuracy or integrity of any part of the work are appropriately investigated and resolved. The study was conducted in accordance with the Declaration of Helsinki (as revised in 2013).
Open Access Statement: This is an Open Access article distributed in accordance with the Creative Commons Attribution-NonCommercial-NoDerivs 4.0 International License (CC BY-NC-ND 4.0), which permits the non-commercial replication and distribution of the article with the strict proviso that no changes or edits are made and the original work is properly cited (including links to both the formal publication through the relevant DOI and the license). See: https://creativecommons.org/licenses/by-nc-nd/4.0/.
References
- Pavet V, Portal MM, Moulin JC, et al. Towards novel paradigms for cancer therapy. Oncogene 2011;30:1-20. [Crossref] [PubMed]
- Gajewski TF, Schreiber H, Fu YX. Innate and adaptive immune cells in the tumor microenvironment. Nat Immunol 2013;14:1014-22. [Crossref] [PubMed]
- Blum A, Wang P, Zenklusen JC. SnapShot: TCGA-Analyzed Tumors. Cell 2018;173:530. [Crossref] [PubMed]
- Asthagiri AR, Parry DM, Butman JA, et al. Neurofibromatosis type 2. Lancet 2009;373:1974-86. [Crossref] [PubMed]
- Pećina-Šlaus N. Merlin, the NF2 gene product. Pathol Oncol Res 2013;19:365-73. [Crossref] [PubMed]
- McClatchey AI, Saotome I, Ramesh V, et al. The Nf2 tumor suppressor gene product is essential for extraembryonic development immediately prior to gastrulation. Genes Dev 1997;11:1253-65. [Crossref] [PubMed]
- Mota M, Shevde LA. Merlin regulates signaling events at the nexus of development and cancer. Cell Commun Signal 2020;18:63. [Crossref] [PubMed]
- Wei Y, Yee PP, Liu Z, et al. NEDD4L-mediated Merlin ubiquitination facilitates Hippo pathway activation. EMBO Rep 2020;21:e50642. [Crossref] [PubMed]
- Perugorria MJ, Olaizola P, Labiano I, et al. Wnt-β-catenin signalling in liver development, health and disease. Nat Rev Gastroenterol Hepatol 2019;16:121-36. [Crossref] [PubMed]
- Basson MA. Signaling in cell differentiation and morphogenesis. Cold Spring Harb Perspect Biol 2012;4:a008151. [Crossref] [PubMed]
- Petrilli AM, Fernández-Valle C. Role of Merlin/NF2 inactivation in tumor biology. Oncogene 2016;35:537-48. [Crossref] [PubMed]
- Devarakonda S, Pellini B, Verghese L, et al. A phase II study of everolimus in patients with advanced solid malignancies with TSC1, TSC2, NF1, NF2 or STK11 mutations. J Thorac Dis 2021;13:4054-62. [Crossref] [PubMed]
- Schroeder RD, Angelo LS, Kurzrock R. NF2/merlin in hereditary neurofibromatosis 2 versus cancer: biologic mechanisms and clinical associations. Oncotarget 2014;5:67-77. [Crossref] [PubMed]
- Caicedo HH, Hashimoto DA, Caicedo JC, et al. Overcoming barriers to early disease intervention. Nat Biotechnol 2020;38:669-73. [Crossref] [PubMed]
- The GTEx Consortium atlas of genetic regulatory effects across human tissues. Science 2020;369:1318-30. [Crossref] [PubMed]
- Karlsson M, Zhang C, Méar L, et al. A single-cell type transcriptomics map of human tissues. Sci Adv 2021;7:eabh2169. [Crossref] [PubMed]
- Smoot BJ, Wong JF, Dodd MJ. Comparison of diagnostic accuracy of clinical measures of breast cancer-related lymphedema: area under the curve. Arch Phys Med Rehabil 2011;92:603-10. [Crossref] [PubMed]
- Yoshihara K, Shahmoradgoli M, Martínez E, et al. Inferring tumour purity and stromal and immune cell admixture from expression data. Nat Commun 2013;4:2612. [Crossref] [PubMed]
- Choucair K, Morand S, Stanbery L, et al. TMB: a promising immune-response biomarker, and potential spearhead in advancing targeted therapy trials. Cancer Gene Ther 2020;27:841-53. [Crossref] [PubMed]
- van Velzen MJM, Derks S, van Grieken NCT, et al. MSI as a predictive factor for treatment outcome of gastroesophageal adenocarcinoma. Cancer Treat Rev 2020;86:102024. [Crossref] [PubMed]
- Shen W, Song Z, Zhong X, et al. Sangerbox: A comprehensive, interaction-friendly clinical bioinformatics analysis platform. iMeta 2022;1. [Crossref]
- Michie KA, Bermeister A, Robertson NO, et al. Two Sides of the Coin: Ezrin/Radixin/Moesin and Merlin Control Membrane Structure and Contact Inhibition. Int J Mol Sci 2019;20:1996. [Crossref] [PubMed]
- Wu J, Minikes AM, Gao M, et al. Intercellular interaction dictates cancer cell ferroptosis via NF2-YAP signalling. Nature 2019;572:402-6. [Crossref] [PubMed]
- Yang L, Zhang Y. Tumor-associated macrophages: from basic research to clinical application. J Hematol Oncol 2017;10:58. [Crossref] [PubMed]
- Hinshaw DC, Shevde LA. The Tumor Microenvironment Innately Modulates Cancer Progression. Cancer Res 2019;79:4557-66. [Crossref] [PubMed]
- Greten FR, Grivennikov SI. Inflammation and Cancer: Triggers, Mechanisms, and Consequences. Immunity 2019;51:27-41. [Crossref] [PubMed]
- Ravi VM, Neidert N, Will P, et al. T-cell dysfunction in the glioblastoma microenvironment is mediated by myeloid cells releasing interleukin-10. Nat Commun 2022;13:925. [Crossref] [PubMed]
- Yang ZZ, Grote DM, Xiu B, et al. TGF-β upregulates CD70 expression and induces exhaustion of effector memory T cells in B-cell non-Hodgkin's lymphoma. Leukemia 2014;28:1872-84. [Crossref] [PubMed]
- Cader FZ, Schackmann RCJ, Hu X, et al. Mass cytometry of Hodgkin lymphoma reveals a CD4(+) regulatory T-cell-rich and exhausted T-effector microenvironment. Blood 2018;132:825-36. [Crossref] [PubMed]
- Sanjabi S, Oh SA, Li MO. Regulation of the Immune Response by TGF-β: From Conception to Autoimmunity and Infection. Cold Spring Harb Perspect Biol 2017;9:a022236. [Crossref] [PubMed]
- Liu G, Chen T, Ding Z, et al. Inhibition of FGF-FGFR and VEGF-VEGFR signalling in cancer treatment. Cell Prolif 2021;54:e13009. [Crossref] [PubMed]
- Fang JY, Richardson BC. The MAPK signalling pathways and colorectal cancer. Lancet Oncol 2005;6:322-7. [Crossref] [PubMed]
- Lee S, Rauch J, Kolch W. Targeting MAPK Signaling in Cancer: Mechanisms of Drug Resistance and Sensitivity. Int J Mol Sci 2020;21:1102. [Crossref] [PubMed]
- Yi C, Troutman S, Fera D, et al. A tight junction-associated Merlin-angiomotin complex mediates Merlin's regulation of mitogenic signaling and tumor suppressive functions. Cancer Cell 2011;19:527-40. [Crossref] [PubMed]
- Nusse R, Clevers H. Wnt/β-Catenin Signaling, Disease, and Emerging Therapeutic Modalities. Cell 2017;169:985-99. [Crossref] [PubMed]
- Goodman AM, Kato S, Bazhenova L, et al. Tumor Mutational Burden as an Independent Predictor of Response to Immunotherapy in Diverse Cancers. Mol Cancer Ther 2017;16:2598-608. [Crossref] [PubMed]
- Haslam A, Prasad V. Estimation of the Percentage of US Patients With Cancer Who Are Eligible for and Respond to Checkpoint Inhibitor Immunotherapy Drugs. JAMA Netw Open 2019;2:e192535. [Crossref] [PubMed]
- Wu Y, Xu J, Du C, et al. The Predictive Value of Tumor Mutation Burden on Efficacy of Immune Checkpoint Inhibitors in Cancers: A Systematic Review and Meta-Analysis. Front Oncol 2019;9:1161. [Crossref] [PubMed]
- Schrock AB, Ouyang C, Sandhu J, et al. Tumor mutational burden is predictive of response to immune checkpoint inhibitors in MSI-high metastatic colorectal cancer. Ann Oncol 2019;30:1096-103. [Crossref] [PubMed]
- Fan A, Wang B, Wang X, et al. Immunotherapy in colorectal cancer: current achievements and future perspective. Int J Biol Sci 2021;17:3837-49. [Crossref] [PubMed]
- Shi H, Wei J, He C. Where, When, and How: Context-Dependent Functions of RNA Methylation Writers, Readers, and Erasers. Mol Cell 2019;74:640-50. [Crossref] [PubMed]
- Nombela P, Miguel-López B, Blanco S. The role of m6A, m5C and Ψ RNA modifications in cancer: Novel therapeutic opportunities. Mol Cancer 2021;20:18. [Crossref] [PubMed]
- Barbieri I, Tzelepis K, Pandolfini L, et al. Promoter-bound METTL3 maintains myeloid leukaemia by m(6)A-dependent translation control. Nature 2017;552:126-31. [Crossref] [PubMed]
- Frye M, Watt FM. The RNA methyltransferase Misu (NSun2) mediates Myc-induced proliferation and is upregulated in tumors. Curr Biol 2006;16:971-81. [Crossref] [PubMed]
- Chen X, Li A, Sun BF, et al. 5-methylcytosine promotes pathogenesis of bladder cancer through stabilizing mRNAs. Nat Cell Biol 2019;21:978-90. [Crossref] [PubMed]
- Yoshizaki H, Okuda S. Elucidation of the evolutionary expansion of phosphorylation signaling networks using comparative phosphomotif analysis. BMC Genomics 2014;15:546. [Crossref] [PubMed]