Development of a multiparametric model for predicting the response to neoadjuvant chemotherapy in breast cancer
Highlight box
Key findings
• A multiparametric model based on clinical-pathological-magnetic resonance imaging (MRI) features was established to forecast the effect of neoadjuvant chemotherapy (NAC) in patients with locally advanced breast cancer.
What is known and what is new?
• The histopathological features and the radiomics features of tumors are related to the efficacy of NAC efficacy in breast cancer patients.
• This was the first study to establish a multiparametric model based on clinical- pathological-MRI features to forecast the effect of NAC in patients with locally advanced breast cancer.
What is the implication, and what should change now?
• This new model, which is based on the clinical-pathological-MRI features of breast cancer, could be used as new method to predict the effect of NAC in clinical settings.
Introduction
According to worldwide cancer statistics, in 2021, breast cancer ranked first among cancers in women in new cancer cases, accounting for approximately 30% of all new cancer cases for women, and is increasing at a rate of 0.5% per year, and was the leading cause of tumor-related death in women (1). In China, the incidence rate of advanced breast cancer is 545.29 per 100,000 women every year, and 30% of patients who are initially diagnosed with early breast cancer and receive adjuvant therapy eventually develop recurrence or metastasis (2).
The increasing rate of surgical resection of tumors is important for locally advanced patients with breast cancer. Neoadjuvant chemotherapy (NAC) can lower the chance of tumor recurrence after surgery and the clinical tumor stage, improve the chance of breast-conserving surgery, and provide sensitive information on effective drugs (3-5). Breast cancer patients who respond to NAC can be prescribed sensitive drugs after underdoing radical mastectomy for breast cancer (3-5).
Predicting the efficacy of NAC early and choosing the appropriate timing for radical mastectomy are important. The histopathological features and genomic expression of tumors are used to monitor disease progression and responses to treatment, detect tumor reoccurrence, and evaluate the prognosis of breast cancer patients (6,7). Some studies have reported that the histopathologic features of tumor tissue, including programmed death-ligand 1 expression and Ki-67 status, are related to the efficacy of NAC in breast cancer patients (8,9). Moreover, the predictive effects of molecular markers and liquid biopsies in the NAC of breast cancer patients have received widespread attention (10,11). Previous studies have shown the effectiveness of NAC using molecular biomarkers and liquid biopsies (12-15).
In addition to molecular biomarkers and liquid biopsies, significant progress has been made in predicting the efficacy of NAC for breast cancer using radiomics methods. Several studies have examined the multidimensional features of tumor tissues using imaging parameters, such as ultrasound, 18F-FDG PET/CT, and magnetic resonance imaging (MRI) scanning, to predict breast cancer patients’ responses to treatment and prognosis early (16-18). Changes in the maximum size of the breast cancer combined with ultrasonography can be informative in predicting NAC efficacy and evaluating residual lesions (19). Notably, the volumetric parameters of 18F-FDG PET/CT before NAC, such as the metabolic tumor volume and total lesion glycolysis, are significantly correlated with the disease-free survival of breast cancer patients (20). Recent studies have also shown that MRI features of tumors identified by pretreatment multiparametric MRI examination are related to breast cancer patients’ responses to NAC, and can predict the sensitivity of breast cancer patients to NAC (21-24). Several studies have shown that different MRI sequences of tumors can provide microstructural information, including information about blood supply, tissue density, and the shape and size of the lesion, and these MRI sequences are widely used in the clinical diagnosis of breast cancer (25-27). Tsukada et al. found that a tumor growth pattern parallel to Cooper’s ligaments and a fast wash-out rate on pretreatment multiparametric MRI are associated with the pathological complete response (pCR) of breast cancer patients by combining multiple MRI parameters (28).
However, few studies have examined the relationship between the NAC response and multiparametric features of breast cancer patients, including the clinical, pathological, and MRI features, using a prediction model. In this study, in addition to the basic clinical features of patients with locally advanced breast cancers, we gathered pathological data and radiological characteristic data by MRI and investigated the correlation between clinical-pathological-MRI parameters and the pCR by establishing a prediction model. The least absolute shrinkage and selection operator (LASSO) regression model was used for the variable screening. Some features that were significantly associated with pCR were selected by a multivariate logistic regression analysis. A multiparametric model to predict the responses of breast cancer patients to NAC was built and the performance of this model was assessed by calibration and decision curves. The established multiparametric model, which is based on clinical-pathological-MRI features, can predict the effect of NAC for patients with locally advanced breast cancer early and enable clinicians to implement timely patient treatment regimens. We present this article in accordance with the TRIPOD reporting checklist (available at https://tcr.amegroups.com/article/view/10.21037/tcr-23-770/rc).
Methods
Patients
This retrospective study was approved by the Medical Research Ethics Committee (an institutional review board) of the First Affiliated Hospital of University of Science and Technology of China (number: 2020-P-045), and all the participants agreed to participate in the study and signed the informed consent form. The study was conducted in accordance with the Declaration of Helsinki (as revised in 2013).
In total, 91 patients with locally advanced breast cancer who received NAC and radical surgery for breast cancer at the First Affiliated Hospital of University of Science and Technology of China from 2016 to 2020 were enrolled in this study. To be eligible for inclusion in this study, the patients had to meet the following inclusion criteria: (I) have stage II–III locally advanced breast cancer without distant metastasis as confirmed by pathological biopsy and imaging examination; (II) have undergone multiparameter MRI examinations at the baseline before NAC; (III) have not received receive radiotherapy or endocrine therapy before NAC; and (IV) have undergone pathological examinations of breast cancer tumor tissues by radical surgery. Patients were excluded from the study if they met any of the following exclusion criteria: (I) had no pathology test results available; (II) had not undergone preoperative or postoperative pathological assessment; (III) had received radiotherapy or endocrine therapy before NAC; and/or (IV) had low or poor quality multiparameter MRI images, and data that could not be calculated and analyzed.
Magnetic resonance imaging
The enrolled patients received three multiparameter MRI tests before radical mastectomy at the following time points: the baseline, after two cycles of chemotherapy, and after three cycles of chemotherapy. The parameters and sequences of the multiparameter MRI included intravoxel incoherent motion, diffusion-weighted imaging, and dynamic contrast-enhanced MRI. All the patients underwent MRI using a 3.0 T MRI (GE Signa HD × T, USA) with an 8-channel dedicated breast coil. For more detailed information on the MRI images and evaluation methods of the different sequences and parameters, see our previous study (29). The apparent diffusion coefficient (ADC) values were evaluated by two senior radiologists, and the test was repeated three times.
Immunohistochemistry
We defined the values of estrogen receptor (ER) and progesterone receptor (PR) expression as positive or negative, and a cut-off value of 1% was set (30). A positive staining area ≤20% was defined as Ki-67 negative, while a positive staining area >20% was defined as Ki-67 positive (31). The histologic grade of the tumor tissue was assessed using the Bloom Richardson method. The immunohistochemical expression of the human epidermal growth factor receptor 2 (HER2) protein was examined using the BenchMark ULTRA automated stainer (Ventana, USA) using the VENTANA anti-HER2/neu (4B5) rabbit monoclonal primary antibody (Ventana, USA). We used the anti-HER2/neu (4B5) rabbit monoclonal primary antibody and the ultraView Universal DAB text kit with the BenchMark ULTRA automated stainer, and the anti-HER2/neu (4B5) rabbit monoclonal primary antibody was incubated for 12 minutes at 36 ℃. Hematoxylin 2 was used for counterstaining for 4 minutes. The expression level of the HER2 protein was scored as 0, 1+, 2+, or 3+. A score of 0 or 1+ was considered negative, while a score of 3+ was considered positive. Samples with a score of 2+ were subsequently confirmed by fluorescence in situ hybridization in accordance with the manufacturer’s instructions (32).
NAC regimen and response assessment
The enrolled patients received two to eight cycles of NAC, and chemotherapy regimens, including epirubicin plus cyclophosphamide, epirubicin plus cyclophosphamide, and docetaxel, epirubicin, plus cyclophosphamide, were determined as per the guidelines. The Miller-Payne system has five grades. pCR was defined as pathological complete remission, and non-pCR was defined as non-pathological complete remission. A grade 5 pathological reaction was considered pCR, while grade 1–4 pathological reactions were considered non-pCR. pCR was defined as the absence of invasive cancer cells in the surgical specimen after NAC for cancer, with or without the presence of ductal invasive carcinoma. Conversely, non-pCR was defined as the presence of invasive cancer cells in the surgical specimen after NAC. According to the Miller-Payne system, the efficacy of NAC was evaluated by comparing the operative specimen with the preoperative specimen (33,34).
Clinical characteristics of breast cancer patients and magnetic resonance imaging parameters of tumor tissues
In this retrospective study, the clinical, pathological, and MRI characteristics were observed and analyzed. The basic characteristics included patient age, disease history, a family history of tumor, and menstrual history. The clinical features included the clinical stage of the tumor, luminal type, Miller-Payne grade, tumor marker, chemotherapy regimen and cycle, complete blood count, liver function, creatinine, D-dimer, and left ventricular ejection fraction. The histopathological features included lymph node status, vascular and nerve status, and the nipple, basal, and tumor tissue margins. The protein expression levels were obtained by immunohistochemistry, and the proteins included ER, PR, HER2, Ki-67, CD31, CD34, CD56, D2-40, P53, P63, CK5/6, CK7, CK5, AR, P120, Syn, CgA, GATA-3, GCDFD-15, Sox-11, vimentin, calponin, E-cadherin, and lactoglobulin.
Next, the multiparametric MRI features were analyzed. First, the type of breast and the morphology of the tumor were described. Breast types included heterogeneously dense breast, scattered fibroglandular breast and extremely dense breast. The morphology of the tumor included the size and quadrant of the lesion, the shape of the lesion (circular, extent, satellite, or irregular), and the pattern of the lesion (burr, necrosis, or hole).
The features of the blood vessels, muscles, and axillary lymph nodes around the tumor were also observed. The internal mammary, axillary, thoracic, and intercostal arteries were described and analyzed. The pectoralis major muscle, pectoralis minor muscle, and intercostal muscle were also observed and assessed. The features of the axillary lymph nodes were described, including the number, size, and signal parameters of the lymph nodes. The features of skin edema, mammary duct, subcutaneous fascia, areola of the nipple, retro-mammary space, ulcer, and Cooper’s ligaments were also studied and evaluated.
Other features included the Breast Imaging Reporting and Data System grade of the tumor, pleural effusion, contralateral breast diseases, and disease of other organs. T1- or T2-weighted sequences of the MRI were examined, and the above data were recorded. The MRI parameters also included different ADC values (standard, fast, or slow ADC values) at different time points, the type of the time-signal intensity curve, and the curve variation type.
Statistical analysis
In this study, the correlation between clinical, pathological, or MRI features and Miller-Payne grades were assessed by the Chi-squared test or Fisher’s exact test. The LASSO binary logistic regression model was used to analyze the clinical, pathological, and MRI features. According to the logistic regression analysis, the odds ratio (OR) and coefficients were estimated. P values less than 0.05 were considered statistically significant.
Development of the multiparametric model
The correlation between the multiparametric characteristics and NAC efficacy was analyzed. A LASSO regression model was used for the dimension reduction of the data, feature selection, and feature building. With the 10-fold cross-validation, the optimization target would be a binomial deviance of no more than the simplest models that would provide the minimum nominal deviance standard deviation. A generalized and linear model with a L2 penalty was used to fit the model, and a multiparametric model was established.
A LASSO regression model can be used to study the relationship between the dependent variable (target) and the independent variable (predictor), and thus has a wide range of uses in clinical applications. For example, LASSO regression models can be used for: (I) disease prediction: a LASSO regression model can be used to predict the occurrence and progression of diseases. By choosing appropriate independent variables, prediction models can be built to help doctors identify high-risk patients and implement appropriate interventions. (II) Biomarker research: a LASSO regression model can be used to screen the biomarkers associated with diseases. By analyzing large-scale biological data, key biomarkers related to diseases can be identified, which can help in the early diagnosis and treatment of diseases. (III) Drug discovery and developments: a LASSO regression model can be used to build effect prediction models for screening new drugs. A LASSO regression model can be built to predict the efficacy of some new drugs. Drug development can help clinicians formulate individualized treatment schemes.
Performance of the multiparametric model
A nomogram was used to visualize the multiparametric model. To further evaluate the performance of the model, calibration and decision curves were plotted. To verify the effect of the model, we repeatedly used 5-fold cross-validation for the data. The cross-validation data were independently selected and fitted with the model by processing the whole data. Through multiple cross-validations, the values and 95% confidence intervals (CIs) of accuracy, the area under the curve (AUC), positive predictive value (PPV), negative predictive value (NPV), sensitivity, and specificity were calculated to assess the model.
Results
Clinical characteristics
The clinical characteristics of 91 breast cancer patients who received NAC were analyzed and are shown in Table 1. In the primary cohort, the breast cancer patients had an average age of 47.33±18.56 years in the pCR group and 48.56±11.85 years in the non-pCR group (P=0.894). In the validation cohort, the breast cancer patients had an average age of 45.91±9.45 years in the pCR group and 49.88±10.45 years in the non-pCR group (P=0.260) (Table 1). As Table 1 shows, 7 (26.9%) and 19 (73.1%) patients had pCR and non-pCR in the primary cohort, respectively. The pathological types of tumor tissue included invasive carcinoma, high-grade intraductal carcinoma, ductal carcinoma, invasive lobular carcinoma, and mucinous adenocarcinoma. In the pCR group, 71.4% and 78.6% had the invasive type in the primary and validation cohorts, respectively. In terms of the clinical stage of the primary cohort, 85.7% had stage II and 14.3% had stage III in the pCR group, and 26.3% had stage II and 73.7% had stage III in non-pCR groups, and the difference between the two groups was significant (P=0.007). A significant difference (P=0.014) between the pCR and non-pCR groups was also observed in the validation cohort (Table 1).
Table 1
Characteristic | Primary cohort | Validation cohort | |||||
---|---|---|---|---|---|---|---|
non-pCR | pCR | P | non-pCR | pCR | P | ||
Age, years | 48.56±11.85 | 47.33±18.56 | 0.894 | 49.88±10.45 | 45.91±9.45 | 0.260 | |
Histological type* | 0.211 | 0.397 | |||||
Invasive | 17 (89.5) | 5 (71.4) | 43 (84.3) | 11 (78.6) | |||
Mixed invasive | 2 (10.5) | 2 (28.6) | 8 (15.7) | 3 (21.4) | |||
Clinical stage* | 0.007 | 0.014 | |||||
II | 5 (26.3) | 6 (85.7) | 18 (35.3) | 10 (71.4) | |||
III | 14 (73.7) | 1 (14.3) | 33 (64.7) | 4 (28.6) | |||
HER2 status | 0.548 | 0.541 | |||||
Positive | 10 (55.6) | 3 (42.9) | 19 (37.3) | 7 (50.0) | |||
Negative | 8 (44.4) | 4 (57.1) | 32 (62.7) | 7 (50.0) | |||
Unkown | 1 | 0 | |||||
Ki-67 status | 0.369 | 0.527 | |||||
≤20% | 7 (38.9) | 4 (57.1) | 16 (31.4) | 6 (42.9) | |||
>20% | 11 (61.1) | 3 (42.9) | 35 (68.6) | 8 (57.1) | |||
Unkown | 1 | 0 |
Data are presented as mean ± SD or number (percentage). *, data are measured at baseline. HER2, human epidermal growth factor receptor 2; pCR, pathologic complete response; SD, standard deviation.
The HER2 and Ki-67 status of the tumor tissues was also analyzed. The data indicated that 55.6% and 37.3% of the non-pCR patients had positive HER2 expression in the primary and validation cohorts, respectively. As Table 1 shows, 61.1% and 68.6% of the non-pCR patients had positive Ki-67 expression in the primary and validation cohorts, respectively. There were no significant differences between HER2 and Ki-67 statuses in the two cohorts, and the P values for the HER2 expression difference between the two groups were 0.548 and 0.541 in the primary and validation cohorts, respectively. The P values for the Ki-67 expression difference between the two groups were 0.369 and 0.527 in the primary and validation cohorts, respectively.
Feature selection and multiparametric model construction
In our study, 102 clinical, pathological, and MRI features were analyzed using the LASSO binary logistic regression model (Figure 1). The selected λ value and log(λ) were 0.1466567 and −1.919661, respectively. The optimal five features were selected to build the multiparametric model (Figure 1). The features of the established model included lymph node metastasis, the first standard ADC at the baseline, the change in the standard ADC at the first follow-up, the change in tumor volume at the first follow-up, and the clinical stage at the baseline.
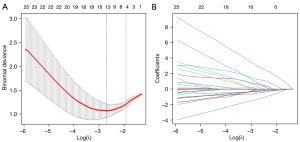
Development and evaluation of the nomogram
By multiple logistic and linear regression analyses, a multiparametric and predictive model was established based on the five features, and a nomogram was developed based on the model (Figure 2A). As Figure 2B shows, the gray, long and dashed line (ideal) represents perfect prediction, while the gray, short and dashed line (apparent) shows the predicted probability of the nomogram, which was close to the ideal line. The results indicated that the two curves had a good correlation, and that the nomogram had good predictive performance (Figure 2B). The bias correction (as represented by the solid black line) was estimated using internal verification and bootstrap methods (Figure 2B). Figure 2C displays the decision curves for the nomogram, and the cost-to-benefit ratio was 1:10. The established nomogram showed good performance regardless of whether the patients had pCR or non-pCR.
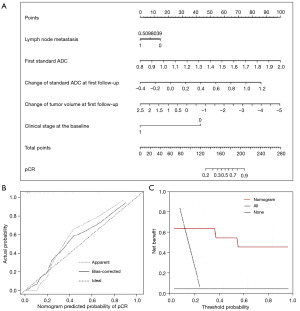
Performance of the multiparametric model
Next, the performance of the established model was examined by a receiver operating characteristic (ROC) analysis (Figure 3A,3B). As Figure 3 show, the AUCs were 0.984 (95% CI: 0.958–1) and 0.815 (95% CI: 0.509–1) in the primary and validation cohorts, respectively. The accuracy rates of the predictive model were 0.945 (95% CI: 0.865–1) and 0.753 (95% CI: 0.583–0.833) in the primary and validation cohorts, respectively. As Table 2 shows, the PPVs were 0.832 (95% CI: 0.619–1) and 0.535 (95% CI: 0.333–0.667) in the primary and validation cohorts, respectively. The NPVs of the predictive model were 0.990 (95% CI: 0.951–1) and 0.871 (95% CI: 0.778–1) in the primary and validation cohorts, respectively (Table 2). The sensitivity and specificity of the model were also analyzed, and the results are set out in Table 2. The model had a sensitivity of 0.871 (95% CI: 0.778–1) and 0.955 (95% CI: 0.769–1), and a specificity of 0.642 (95% CI: 0.333–1) and 0.938 (95% CI: 0.816–1) in the primary and validation cohorts, respectively.
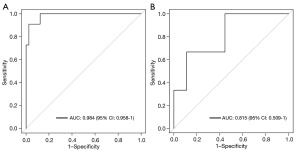
Table 2
Metrics | Clinical prediction model | |
---|---|---|
Primary cohort | Validation cohort | |
Accuracy (95% CI) | 0.945 (0.865–1) | 0.753 (0.583–0.833) |
AUC (95% CI) | 0.984 (0.958–1) | 0.815 (0.509–1) |
PPV (95% CI) | 0.832 (0.619–1) | 0.535 (0.333–0.667) |
NPV (95% CI) | 0.990 (0.951–1) | 0.871 (0.778–1) |
Sensitivity (95% CI) | 0.871 (0.778–1) | 0.955 (0.769–1) |
Specificity (95% CI) | 0.642 (0.333–1) | 0.938 (0.816–1) |
AUC, area under the curve; CI, confidence interval; NPV, negative predictive value; PPV, positive predictive value.
According to the logistic regression analysis, lymph node metastasis (OR: 0.442; 95% CI: 0.072–2.707; P=0.377), the first standard ADC at the baseline (OR: 3.165; 95% CI: 1.396–7.175; P=0.006), the change in the standard ADC at the first follow-up (OR: 2.304; 95% CI: 0.915–5.804; P=0.077), the change in tumor volume at the first follow-up (OR: 0.572; 95% CI: 0.254–1.287; P=0.177), and the clinical stage at the baseline (OR: 0.089; 95% CI: 0.013–0.611; P=0.014) were five predictive factors for NAC in the established model (Table 3).
Table 3
Variable | Clinical prediction model | ||
---|---|---|---|
Coefficient | Odds ratio (95% CI) | P | |
Lymph node metastasis | −0.817 | 0.442 (0.072–2.707) | 0.377 |
First standard ADC at the baseline | 1.152 | 3.165 (1.396–7.175) | 0.006 |
Change in standard ADC at the first follow-up | 0.835 | 2.304 (0.915–5.804) | 0.077 |
Change in tumor volume at the first follow-up | −0.560 | 0.572 (0.254–1.287) | 0.177 |
Clinical stage at baseline | −2.424 | 0.089 (0.013–0.611) | 0.014 |
ADC, apparent diffusion coefficient.
Discussion
The efficacy of chemotherapy in breast cancer patients is often evaluated by pathological and imaging results, and the Response Evaluation Criteria in Solid Tumors (RECIST) 1.1 are often recommended for assessing the efficacy of chemotherapy in breast cancer patients (35,36). The Miller-Payne system is widely used to evaluate NAC for breast cancer patients. The standard is based on the postoperative pathological results and thus it cannot predict the effect of chemotherapy before breast cancer surgery. In clinical practice, new methods are needed to predict NAC efficacy and to choose optimal treatment plans early for breast cancer patients. Dammu et al. established a new neural network based on MRI omics for predicting lymph node metastasis and the survival time of breast cancer patients (37). Some scholars have established predictive models using multiparametric MRI sequences that can predict pCR early and prognosis after NAC for patients with breast cancer (38,39).
The correlation between lymph node metastasis and NAC efficacy for breast cancer has also been confirmed by other studies using computed tomography, B-ultrasound, and MRI methods (40-42). In this retrospective study, lymph node metastasis was related to NAC efficacy, and some other parameters (e.g., the first standard ADC value at the baseline, the change in the standard ADC, and tumor volume at the first follow-up) were also found to be predictors of NAC efficacy. These results provide useful information for predicting the efficacy of NAC in patients with breast cancer. Unfortunately, our study showed that pathological features were not significantly associated with pCR in the predictive model.
The AUC values for the ROC curves were 0.984 (95% CI: 0.958–1) and 0.815 (95% CI: 0.509–1) in the primary and validation cohorts, respectively. A penalty was adopted in the model, and the 95% probability of the AUC values in the two cohorts was greater than 0.8. The results indicated that there was no obvious overfitting and that the established model had predictive validity in the two cohorts. The PPV value in the validation cohort was 0.535 (95% CI: 0.333–0.667). The specificity of the model in the primary cohort was 0.642 (95% CI: 0.333–1). Due to the small sample size of our study, the PPV and specificity were not high. In future research, we will increase the sample size to improve the predictive efficiency of our established model.
Conclusions
In conclusion, our study established a multiparametric and predictive model for breast cancer patients. The established model could be used early for preoperative and individualized predictions of NAC efficacy in patients with breast cancer and could help clinicians to select treatment regimens in a timely manner.
Acknowledgments
Funding: This work was supported by
Footnote
Reporting Checklist: The authors have completed the TRIPOD reporting checklist. Available at https://tcr.amegroups.com/article/view/10.21037/tcr-23-770/rc
Data Sharing Statement: Available at https://tcr.amegroups.com/article/view/10.21037/tcr-23-770/dss
Peer Review File: Available at https://tcr.amegroups.com/article/view/10.21037/tcr-23-770/prf
Conflicts of Interest: All authors have completed the ICMJE uniform disclosure form (available at https://tcr.amegroups.com/article/view/10.21037/tcr-23-770/coif). The authors have no conflicts of interest to declare.
Ethical Statement: The authors are accountable for all aspects of the work in ensuring that questions related to the accuracy or integrity of any part of the work are appropriately investigated and resolved. This retrospective study was approved by the Medical Research Ethics Committee (an institutional review board) of the First Affiliated Hospital of University of Science and Technology of China (number: 2020-P-045), and all the participants agreed to participate in the study and signed the informed consent form. The study was conducted in accordance with the Declaration of Helsinki (as revised in 2013).
Open Access Statement: This is an Open Access article distributed in accordance with the Creative Commons Attribution-NonCommercial-NoDerivs 4.0 International License (CC BY-NC-ND 4.0), which permits the non-commercial replication and distribution of the article with the strict proviso that no changes or edits are made and the original work is properly cited (including links to both the formal publication through the relevant DOI and the license). See: https://creativecommons.org/licenses/by-nc-nd/4.0/.
References
- Siegel RL, Miller KD, Fuchs HE, et al. Cancer Statistics, 2021. CA Cancer J Clin 2021;71:7-33. [Crossref] [PubMed]
- Zheng R, Zhang S, Zeng H, et al. Cancer incidence and mortality in China, 2016. Journal of the National Cancer Center 2022;2:1-9. [Crossref]
- Yau C, Osdoit M, van der Noordaa M, et al. Residual cancer burden after neoadjuvant chemotherapy and long-term survival outcomes in breast cancer: a multicentre pooled analysis of 5161 patients. Lancet Oncol 2022;23:149-60. [Crossref] [PubMed]
- Geyer CE, Sikov WM, Huober J, et al. Long-term efficacy and safety of addition of carboplatin with or without veliparib to standard neoadjuvant chemotherapy in triple-negative breast cancer: 4-year follow-up data from BrighTNess, a randomized phase III trial. Ann Oncol 2022;33:384-94. [Crossref] [PubMed]
- Shepherd JH, Ballman K, Polley MC, et al. CALGB 40603 (Alliance): Long-Term Outcomes and Genomic Correlates of Response and Survival After Neoadjuvant Chemotherapy With or Without Carboplatin and Bevacizumab in Triple-Negative Breast Cancer. J Clin Oncol 2022;40:1323-34. [Crossref] [PubMed]
- Wang H, Mao X. Evaluation of the Efficacy of Neoadjuvant Chemotherapy for Breast Cancer. Drug Des Devel Ther 2020;14:2423-33. [Crossref] [PubMed]
- Chen F, Yang J, Fang M, et al. Necroptosis-related lncRNA to establish novel prognostic signature and predict the immunotherapy response in breast cancer. J Clin Lab Anal 2022;36:e24302. [Crossref] [PubMed]
- Huang Z, Shao W, Han Z, et al. Artificial intelligence reveals features associated with breast cancer neoadjuvant chemotherapy responses from multi-stain histopathologic images. NPJ Precis Oncol 2023;7:14. [Crossref] [PubMed]
- Lan A, Chen J, Li C, et al. Development and Assessment of a Novel Core Biopsy-Based Prediction Model for Pathological Complete Response to Neoadjuvant Chemotherapy in Women with Breast Cancer. Int J Environ Res Public Health 2023;20:1617. [Crossref] [PubMed]
- Asghar US, Kanani R, Roylance R, et al. Systematic Review of Molecular Biomarkers Predictive of Resistance to CDK4/6 Inhibition in Metastatic Breast Cancer. JCO Precis Oncol 2022;6:e2100002. [Crossref] [PubMed]
- Papakonstantinou A, Gonzalez NS, Pimentel I, et al. Prognostic value of ctDNA detection in patients with early breast cancer undergoing neoadjuvant therapy: A systematic review and meta-analysis. Cancer Treat Rev 2022;104:102362. [Crossref] [PubMed]
- Janssen LM, Suelmann BBM, Elias SG, et al. Improving prediction of response to neoadjuvant treatment in patients with breast cancer by combining liquid biopsies with multiparametric MRI: protocol of the LIMA study - a multicentre prospective observational cohort study. BMJ Open 2022;12:e061334. [Crossref] [PubMed]
- Ma G, Wang JY, Liu XA, et al. Prediction of neoadjuvant chemotherapeutic efficacy by CTC and cfDNA in patients with locally advanced breast cancer. J Clin Oncol 2019;37:551. [Crossref]
- Pore AA, Dhanasekara CS, Navaid HB, et al. Comprehensive Profiling of Cancer-Associated Cells in the Blood of Breast Cancer Patients Undergoing Neoadjuvant Chemotherapy to Predict Pathological Complete Response. Bioengineering (Basel) 2023;10:485. [Crossref] [PubMed]
- Freitas AJA, Causin RL, Varuzza MB, et al. Liquid Biopsy as a Tool for the Diagnosis, Treatment, and Monitoring of Breast Cancer. Int J Mol Sci 2022;23:9952. [Crossref] [PubMed]
- Taleghamar H, Jalalifar SA, Czarnota GJ, et al. Deep learning of quantitative ultrasound multi-parametric images at pre-treatment to predict breast cancer response to chemotherapy. Sci Rep 2022;12:2244. [Crossref] [PubMed]
- Bhardwaj D, Dasgupta A, DiCenzo D, et al. Early Changes in Quantitative Ultrasound Imaging Parameters during Neoadjuvant Chemotherapy to Predict Recurrence in Patients with Locally Advanced Breast Cancer. Cancers (Basel) 2022;14:1247. [Crossref] [PubMed]
- Li YL, Wang LZ, Shi QL, et al. CT Radiomics for Predicting Pathological Complete Response of Axillary Lymph Nodes in Breast Cancer After Neoadjuvant Chemotherapy: A Prospective Study. Oncologist 2023;28:e183-90. [Crossref] [PubMed]
- Liu Q, Tang L, Chen M. Ultrasound Strain Elastography and Contrast-Enhanced Ultrasound in Predicting the Efficacy of Neoadjuvant Chemotherapy for Breast Cancer A Nomogram Integrating Ki-67 and Ultrasound Features. J Ultrasound Med 2022;41:2191-201. [Crossref] [PubMed]
- Quartuccio N, Alongi P, Urso L, et al. (18)F-FDG PET-Derived Volume-Based Parameters to Predict Disease-Free Survival in Patients with Grade III Breast Cancer of Different Molecular Subtypes Candidates to Neoadjuvant Chemotherapy. Cancers (Basel) 2023;15:2715. [Crossref] [PubMed]
- Liu C, Huang X, Chen X, et al. Use of Pretreatment Multiparametric MRI to Predict Tumor Regression Pattern to Neoadjuvant Chemotherapy in Breast Cancer. Acad Radiol 2023;30:S62-70. [Crossref] [PubMed]
- Huang Y, Zhu T, Zhang X, et al. Longitudinal MRI-based fusion novel model predicts pathological complete response in breast cancer treated with neoadjuvant chemotherapy: a multicenter, retrospective study. EClinicalMedicine 2023;58:101899. [Crossref] [PubMed]
- Panthi B, Adrada BE, Candelaria RP, et al. Assessment of Response to Neoadjuvant Systemic Treatment in Triple-Negative Breast Cancer Using Functional Tumor Volumes from Longitudinal Dynamic Contrast-Enhanced MRI. Cancers (Basel) 2023;15:1025. [Crossref] [PubMed]
- Kovacevic L, Petrovecki M, Korsa L, et al. Early Assessment of Neoadjuvant Chemotherapy Response Using Multiparametric Magnetic Resonance Imaging in Luminal B-like Subtype of Breast Cancer Patients: A Single-Center Prospective Study. Diagnostics (Basel) 2023;13:694. [Crossref] [PubMed]
- Ba R, Wang X, Zhang Z, et al. Diffusion-time dependent diffusion MRI: effect of diffusion-time on microstructural mapping and prediction of prognostic features in breast cancer. Eur Radiol 2023;33:6226-37. [Crossref] [PubMed]
- Goto M, Sakai K, Toyama Y, et al. Use of a deep learning algorithm for non-mass enhancement on breast MRI: comparison with radiologists' interpretations at various levels. Jpn J Radiol 2023;41:1094-103. [Crossref] [PubMed]
- Verburg E, van Gils CH, van der Velden BHM, et al. Validation of Combined Deep Learning Triaging and Computer-Aided Diagnosis in 2901 Breast MRI Examinations From the Second Screening Round of the Dense Tissue and Early Breast Neoplasm Screening Trial. Invest Radiol 2023;58:293-8. [Crossref] [PubMed]
- Tsukada H, Tsukada J, Schrading S, et al. Accuracy of multi-parametric breast MR imaging for predicting pathological complete response of operable breast cancer prior to neoadjuvant systemic therapy. Magn Reson Imaging 2019;62:242-8. [Crossref] [PubMed]
- Lu N, Dong J, Fang X, et al. Predicting pathologic response to neoadjuvant chemotherapy in patients with locally advanced breast cancer using multiparametric MRI. BMC Med Imaging 2021;21:155. [Crossref] [PubMed]
- Vermij L, Jobsen JJ, León-Castillo A, et al. Prognostic refinement of NSMP high-risk endometrial cancers using oestrogen receptor immunohistochemistry. Br J Cancer 2023;128:1360-8. [Crossref] [PubMed]
- Lee J, Lee YJ, Bae SJ, et al. Ki-67, 21-Gene Recurrence Score, Endocrine Resistance, and Survival in Patients With Breast Cancer. JAMA Netw Open 2023;6:e2330961. [Crossref] [PubMed]
- Wolff AC, Hammond MEH, Allison KH, et al. Human Epidermal Growth Factor Receptor 2 Testing in Breast Cancer: American Society of Clinical Oncology/College of American Pathologists Clinical Practice Guideline Focused Update. J Clin Oncol 2018;36:2105-22. [Crossref] [PubMed]
- Wang W, Liu Y, Zhang H, et al. Prognostic value of residual cancer burden and Miller-Payne system after neoadjuvant chemotherapy for breast cancer. Gland Surg 2021;10:3211-21. [Crossref] [PubMed]
- Hagenaars SC, de Groot S, Cohen D, et al. Tumor-stroma ratio is associated with Miller-Payne score and pathological response to neoadjuvant chemotherapy in HER2-negative early breast cancer. Int J Cancer 2021;149:1181-8. [Crossref] [PubMed]
- Agarwal S, Kapur N, Chaudhary P. Evaluation of Neoadjuvant Response Index (NRI) and Its Correlation with Response Evaluation Criteria in Solid Tumors (RECIST) in Locally Advanced Breast Cancer. Indian J Surg Oncol 2023;14:405-10. [Crossref] [PubMed]
- Wang J, Wang X, Chen R, et al. Circulating tumor cells may serve as a supplement to RECIST in neoadjuvant chemotherapy of patients with locally advanced breast cancer. Int J Clin Oncol 2022;27:889-98. [Crossref] [PubMed]
- Dammu H, Ren T, Duong TQ. Deep learning prediction of pathological complete response, residual cancer burden, and progression-free survival in breast cancer patients. PLoS One 2023;18:e0280148. [Crossref] [PubMed]
- Shi Z, Huang X, Cheng Z, et al. MRI-based Quantification of Intratumoral Heterogeneity for Predicting Treatment Response to Neoadjuvant Chemotherapy in Breast Cancer. Radiology 2023;308:e222830. [Crossref] [PubMed]
- Li C, Lu N, He Z, et al. A Noninvasive Tool Based on Magnetic Resonance Imaging Radiomics for the Preoperative Prediction of Pathological Complete Response to Neoadjuvant Chemotherapy in Breast Cancer. Ann Surg Oncol 2022;29:7685-93. [Crossref] [PubMed]
- Zhang K, Zhu Q, Sheng D, et al. A New Model Incorporating Axillary Ultrasound After Neoadjuvant Chemotherapy to Predict Non-Sentinel Lymph Node Metastasis in Invasive Breast Cancer. Cancer Manag Res 2020;12:965-72. [Crossref] [PubMed]
- Wang L, Li Y, Li J, et al. Computed tomography reconstruction for evaluating response in axillary lymph nodes of breast cancer after neoadjuvant chemotherapy. Clin Transl Oncol 2021;23:240-5. [Crossref] [PubMed]
- Al-Hattali S, Vinnicombe SJ, Gowdh NM, et al. Breast MRI and tumour biology predict axillary lymph node response to neoadjuvant chemotherapy for breast cancer. Cancer Imaging 2019;19:91. [Crossref] [PubMed]