Construction and validation of a novel web-based nomogram for primary ocular adnexal lymphoma: a real-world analysis based on the Surveillance, Epidemiology, and End Results database
Highlight box
Key findings
• We examined conditional survival (CS) pattern and possible prognostic factors in patients diagnosed with primary ocular adnexal lymphoma (POAL) and created a dependable nomogram for predicting their overall survival (OS).
What is known and what is new?
• Due to the low occurrence rate of POAL and the scarcity of relevant research data, there is limited analysis of CS and prognosis factors within the current literature.
• The OS of POAL patients can be predicted by the nomogram model.
What is the implication, and what should change now?
• The newly-established nomogram model proves invaluable in enabling clinical doctors to assess patients’ risk factors and formulate personalized treatment strategies, thereby enhancing survival assessment and clinical management for POAL patients.
Introduction
Primary ocular adnexal lymphoma (POAL) encompasses both intraocular and ocular adnexal lymphomas, representing a subtype of extranodal non-Hodgkin’s lymphoma (1-5). POAL typically manifests as a painless, slightly enlarged mass in the eye, which may lead to eye protrusion (4). However, it does not lead to diplopia (double vision) or any loss of vision. As there is no presence of lymphoid tissue, the incidence of POAL is low (3), only accounting for 5% to 10% of all extra-nodal lymphomas and 10% of all ocular tumors. Non-Hodgkin’s lymphomas are the main pathological type (6-8). Early diagnosis and standardized treatment are important factors affecting the prognosis of POAL (9,10); and conventional treatments for this disease include surgical resection, radiotherapy (RT), and chemotherapy (CT) (11). Due to the low incidence of this type of tumor, there are fewer reports in the literature on POAL (12-15). Our present comprehension of POAL primarily derives from a collection of retrospective analyses and case studies (16-18). And the treatment strategies and survival patterns in POALs have not been adequately analyzed at a large population level (19-21).
The Surveillance, Epidemiology, and End Results (SEER) database is a valuable resource for studying rare malignancies in cases where clinical trials or prospective data are limited (22). Nomograms have been widely used in a variety of cancers for survival prediction with a comprehensive consideration of a range of prognostic factors (23,24). Therefore, the objective of this research is to examine the survival trends and determine the prognostic elements of patients diagnosed with POAL. Subsequently, a pioneering nomogram model based on the SEER database was designed and authenticated to assess long-term survival rates using accessible clinicopathological characteristics. In addition, an online survival calculator has also been constructed to facilitate the provision of therapy suggestions and assist clinical decision-making in clinical practice. We present this article in accordance with the TRIPOD reporting checklist (available at https://tcr.amegroups.com/article/view/10.21037/tcr-23-1556/rc).
Methods
Data source
The SEER database contains demographic and clinical pathological information of American patients (25,26). We retrieved data from the SEER18 database and carefully screened suitable participants for inclusion. The eligibility criteria comprised: (I) patients diagnosed with POAL [International Classification of Disease for Oncology third edition (ICD-O-3) histology codes 9590–9599, 9650–9729]; (II) diagnosis year between 2000 and 2017; (III) primary site was restricted to ocular adnexa (site-specific code C69.0–69.9 and C44.1); and (IV) active follow-up. Patients without histological confirmation and those diagnosed only at autopsy were excluded from our study. The study was conducted in accordance with the Declaration of Helsinki (as revised in 2013).
Data collection
We extracted the following clinicopathological features and treatment information from the SEER database: (I) age (≤50, 51–60, 61–70, 71–80 or >80 years); (II) sex (male or female); (III) race (white or nonwhite); (IV) coexistence with other malignancy; (V) tumor histology [mucosa-associated lymphoid tissue (MALT), diffuse large B-cell lymphoma (DLBCL), follicular lymphoma, or others]; (VI) tumor site [conjunctiva, eyelid, orbit, lacrimal gland or others/eye, not otherwise specified (NOS)]; (VII) laterality of tumor (bilateral or unilateral); (VIII) surgery (yes or no); (IX) RT (yes or no/unknown); (X) CT (yes or no); (XI) marriage status (single, married, or unknown); (XII) rural/urban status (metropolitan counties or non-metropolitan counties); (XIII) median household income (≥$70,000 or <$70,000); and (XIV) tumor stage (locoregional or distant). The endpoints of our research were focused on the measurement of overall survival (OS), representing the duration from diagnosis to the event of passing away due to any cause.
Statistical analysis
The eligible POAL patients were randomly allocated to the training and testing groups in a 7:3 ratio. Descriptive statistics were utilized to present the characteristics of the patients, tumors, and treatments. The conditional survival (CS) trend of the POAL patients was analyzed. Univariate and multivariate Cox analyses were conducted on all predictive factors to screen for potential significant prognostic factors. Significant factors identified by the multivariate model were utilized to create a unique graphical nomogram and a dynamic online survival calculator. The nomogram model was validated in both the training and testing groups using the calibration curve, concordance index (C-index), receiver operating characteristic (ROC) curve, and time-dependent area under the ROC curve (AUC). The data were analyzed using R version 4.0.5 (The R Project for Statistical Computing, Vienna, Austria), with statistical significance defined as P<0.05. Finally, based on the significant prognosis factors, an online dynamic nomogram was created.
Results
Baseline characteristic
In accordance with the inclusion criteria set for this study, a total of 3,324 patients were included in the SEER database between 2000 and 2017. These patients were then randomly distributed into a training group (n=2,327) and a testing group (n=997), maintaining a ratio of 7:3. The baseline characteristics of the patients in both the training and validation cohorts have been summarized in Table 1. In the entire cohort, the mean age [standard deviation (SD)] was 64.6 (16.3) years and the majority of patients were over 50 years of age, with females and white patients being the predominant demographics. And the ratio of female to male was 1.23. Regarding tumor characteristics, the majority of patients were diagnosed with MALT situated in the orbit. In terms of tumor attributes, most patients were diagnosed with MALT located in the orbit. As for treatment, 41.2% of the included patients underwent surgical intervention, while 59.2% received RT and 26.4% were treated with CT.
Table 1
Variables | Training group (n=2,327) | Testing group (n=997) | Overall (n=3,324) |
---|---|---|---|
Age (years), mean ± SD | 64.6±16.3 | 64.6±16.3 | 64.6±16.3 |
Age (years), n (%) | |||
≤50 | 444 (19.1) | 184 (18.5) | 628 (18.9) |
51–60 | 415 (17.8) | 194 (19.5) | 609 (18.3) |
61–70 | 532 (22.9) | 225 (22.6) | 757 (22.8) |
71–80 | 534 (22.9) | 222 (22.3) | 756 (22.7) |
>80 | 402 (17.3) | 172 (17.3) | 574 (17.3) |
Sex, n (%) | |||
Male | 1,065 (45.8) | 425 (42.6) | 1,490 (44.8) |
Female | 1,262 (54.2) | 572 (57.4) | 1,834 (55.2) |
Race, n (%) | |||
White | 1,881 (80.8) | 823 (82.5) | 2,704 (81.3) |
Nonwhite | 418 (18.0) | 167 (16.8) | 585 (17.6) |
Unknown | 28 (1.2) | 7 (0.7) | 35 (1.1) |
Year of diagnosis, n (%) | |||
<2010 | 1,427 (61.3) | 601 (60.3) | 2,028 (61.0) |
≥2010 | 900 (38.7) | 396 (39.7) | 1,296 (39.0) |
Coexistence with other malignancy, n (%) | |||
Yes | 749 (32.2) | 340 (34.1) | 1,089 (32.8) |
No/unknown | 1,578 (67.8) | 657 (65.9) | 2,235 (67.2) |
Tumor histology, n (%) | |||
MALT | 1,265 (54.4) | 541 (54.3) | 1,806 (54.3) |
Others | 497 (21.4) | 212 (21.3) | 709 (21.3) |
DLBCL | 293 (12.6) | 129 (12.9) | 422 (12.7) |
Follicular lymphoma | 272 (11.7) | 115 (11.5) | 387 (11.6) |
Tumor site, n (%) | |||
Conjunctiva | 556 (23.9) | 264 (26.5) | 820 (24.7) |
Eyelid | 177 (7.6) | 72 (7.2) | 249 (7.5) |
Orbit | 1,168 (50.2) | 458 (45.9) | 1,626 (48.9) |
Lacrimal gland | 274 (11.8) | 124 (12.4) | 398 (12.0) |
Others/eye, NOS | 152 (6.5) | 79 (7.9) | 231 (6.9) |
Laterality, n (%) | |||
Bilateral | 190 (8.2) | 92 (9.2) | 282 (8.5) |
Unilateral | 2,137 (91.8) | 905 (90.8) | 3,042 (91.5) |
Surgery, n (%) | |||
Yes | 961 (41.3) | 407 (40.8) | 1,368 (41.2) |
No/unknown | 1,366 (58.7) | 590 (59.2) | 1,956 (58.8) |
RT, n (%) | |||
Yes | 1,388 (59.6) | 580 (58.2) | 1,968 (59.2) |
No/unknown | 939 (40.4) | 417 (41.8) | 1,356 (40.8) |
CT, n (%) | |||
Yes | 615 (26.4) | 261 (26.2) | 876 (26.4) |
No | 1,712 (73.6) | 736 (73.8) | 2,448 (73.6) |
Marital status, n (%) | |||
Single | 841 (36.1) | 360 (36.1) | 1,201 (36.1) |
Unknown | 191 (8.2) | 89 (8.9) | 280 (8.4) |
Married | 1,295 (55.7) | 548 (55.0) | 1,843 (55.4) |
Rural/urban status, n (%) | |||
Metropolitan counties | 2,088 (89.7) | 892 (89.5) | 2,980 (89.7) |
Non-metropolitan counties | 239 (10.3) | 105 (10.5) | 344 (10.3) |
Median household income, n (%) | |||
≥$70,000 | 1,112 (47.8) | 476 (47.7) | 1,588 (47.8) |
<$70,000 | 1,215 (52.2) | 521 (52.3) | 1,736 (52.2) |
Tumor stage, n (%) | |||
Locoregional | 1,966 (84.5) | 849 (85.2) | 2,815 (84.7) |
Distant | 361 (15.5) | 148 (14.8) | 509 (15.3) |
SD, standard deviation; MALT, mucosa-associated lymphoid tissue; DLBCL, diffuse large B-cell lymphoma; NOS, not otherwise specified; RT, radiotherapy; CT, chemotherapy.
We further described the CS pattern of these patients. The CS curves for all patients at 0, 1, 2, 5, 10, and 15 years are shown in Figure 1. Using CS analysis, we observed a noteworthy escalation in the survival rate among these patients for every additional year of survival.
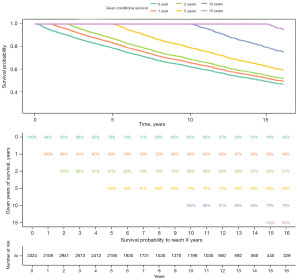
Identify independent prognostic factors
The outcomes of the Cox analysis are displayed in Table 2. There were several factors that had a significant association with OS, including race [with a hazard ratio (HR) of 0.727 and a 95% confidence interval (CI): 0.612–0.863; P<0.001], tumor site (HR, 1.247; 95% CI: 1.182–1.315; P<0.001), tumor histology (HR, 1.255; 95% CI: 1.195–1.318; P<0.001), stage (HR, 1.563; 95% CI: 1.335–1.829; P<0.001), surgery (HR, 0.777; 95% CI: 0.680–0.889; P<0.001), RT (HR, 0.701; 95% CI: 0.615–0.798; P<0.001), CT (HR, 1.258; 95% CI: 1.092–1.450; P=0.002), coexistence of other malignancy (HR, 1.515; 95% CI: 1.328–1.728; P<0.001), age (HR, 2.146; 95% CI: 2.020–2.280, P<0.001), marital status (HR, 0.741; 95% CI: 0.661–0.830, P<0.001). The sex (P=0.077), year of diagnosis (P=0.230), laterality (P=0.616), rural/urban status (P=0.079), and household income (P=0.072) were found no significant correlation with OS.
Table 2
Variables | HR | Lower 95% CI | Upper 95% CI | P value |
---|---|---|---|---|
Race | 0.727 | 0.612 | 0.863 | <0.001*** |
Sex | 0.889 | 0.781 | 1.013 | 0.077 |
Year of diagnosis | 0.990 | 0.975 | 1.006 | 0.230 |
Tumor site | 1.247 | 1.182 | 1.315 | <0.001*** |
Laterality | 0.940 | 0.739 | 1.196 | 0.616 |
Tumor histology | 1.255 | 1.195 | 1.318 | <0.001*** |
Surgery | 0.777 | 0.680 | 0.889 | <0.001*** |
RT | 0.701 | 0.615 | 0.798 | <0.001*** |
CT | 1.258 | 1.092 | 1.450 | 0.002** |
Coexistence of other malignancy | 1.515 | 1.328 | 1.728 | <0.001*** |
Age | 2.146 | 2.020 | 2.280 | <0.001*** |
Marital status | 0.741 | 0.661 | 0.830 | <0.001*** |
Rural/urban status | 1.195 | 0.979 | 1.459 | 0.079 |
Household income | 0.887 | 0.779 | 1.011 | 0.072 |
Tumor stage | 1.563 | 1.335 | 1.829 | <0.001*** |
**, P<0.010; ***, P<0.001. HR, hazard ratio; CI, confidence interval; RT, radiotherapy; CT, chemotherapy.
And the multivariable Cox analysis showed age (P<0.001), sex (P<0.001), tumor site (P<0.001), tumor histology (P<0.001), coexistence of other malignancy (P=0.018), surgery (P=0.01), RT (P<0.001), and marital status (P<0.001) were identified as independent prognosis factors (Figure 2). The Kaplan-Meier curve analysis was depicted to further verify the prognostic abilities of these factors (Figure 3), showing that longer OS was related to age (P<0.0001), tumor site (P<0.0001), tumor histology (P<0.0001), coexistence of other malignancy (P<0.0001), surgery (P=0.00022), and RT (P<0.0001).
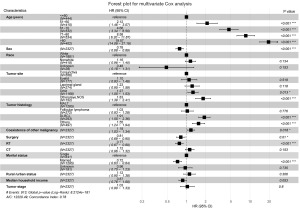
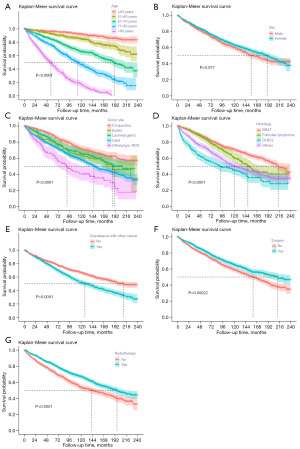
Establishment and validation of the nomogram
To predict the rates of OS for the periods of 2, 5, 10, and 15 years, a nomogram was created based on seven independent prognosis factors that showed significance. These factors included age, gender, tumor site, tumor histology, presence of other malignancy, surgical intervention, and RT for patients with POAL. The nomogram, shown in Figure 4, revealed that age was the most prominent contributor to prognosis, followed by tumor histology, tumor site, RT, surgical intervention, and gender. Each prognostic parameter was attributed a score based on the corresponding scoring table. After adding up the individual scores and consulting the comprehensive score table, the calculated likelihood of survival at each given time interval could be readily ascertained by tracing a vertical line down the chart. To assess the validity of the nomogram’s predictive capabilities, we utilized calibration curves and C-indexes to evaluate its performance. Calibration curves were generated for survival probabilities at 24, 60, 120, and 180 months for both training (Figure 5) and testing (Figure 6) samples, demonstrating favorable agreement between ideal and calibration curves. C-indexes were 0.77 (95% CI: 0.74–0.80) and 0.77 (95% CI: 0.72–0.81) for the training and testing groups, respectively, indicating strong accuracy of the model. The ROC curve was used to evaluate the sensitivity and specificity of the nomogram for the training and testing samples at survival probabilities of 24, 60, 120, and 180 months, as shown in Figures 7,8. The C-indexes for the training and testing groups were 0.77 (95% CI: 0.74–0.80) and 0.77 (95% CI: 0.72–0.81), respectively, indicating a high level of accuracy for the model. The ROC curve was employed to assess the sensitivity and specificity of the nomogram in the training and testing samples, with survival probabilities of 24, 60, 120, and 180 months, illustrated in Figures 7,8. Figure 9 displays the AUC curves over time for both the training and testing groups. AUC values served as a standard metric for evaluating a model’s predictive prowess, with higher scores indicating superior performance. The time-based AUC values for both the training and testing groups consistently remained above 0.75 in this nomogram., indicating the favorable performance of our survival prediction model.
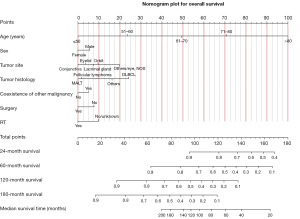
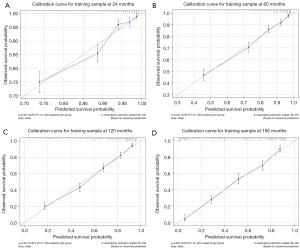
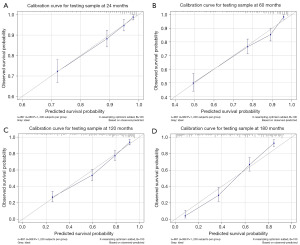
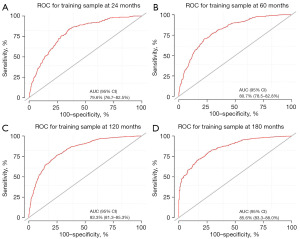
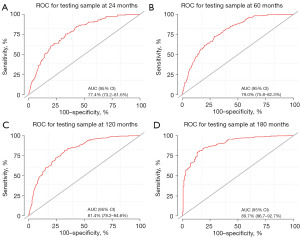
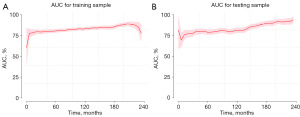
Online dynamic nomogram establishment
Finally, we further created an online dynamic survival calculator based on the nomogram model. Healthcare professionals can access the nomogram easily through our website (https://helloshinyweb.shinyapps.io/eye_dynamic_nomogram/) to draw individual survival curves and predict survival rates at different time points. Figure 10 represents a screenshot of the online tool.
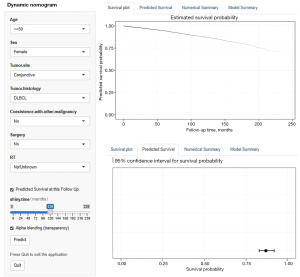
Discussion
Due to the low occurrence rate of POAL and the scarcity of relevant research data, there is limited analysis of CS and prognosis factors within the current literature (27). As POAL is not commonly understood, it can often be misdiagnosed in the early stages, and medical professionals may struggle to provide relatively accurate survival predictions for affected patients (19,28,29). Therefore, it is essential to develop a novel predictive tool based on common clinical characteristics to evaluate patient survival. This research examines CS among patients with POAL using data from the SEER database in the United States. Univariate and multivariate Cox analyses were employed to identify potential prognostic factors. Utilizing significant prognostic factors, we have succeeded in constructing and validating a new POAL patient nomogram model to assist clinicians in providing personalized survival predictions.
The cause of POALs is not fully understood, which makes treatment challenging and highlights the need for further research (19). Some researchers suggested that chronic inflammation or autoimmune disorders might contribute to the development of extranodal reactive lymphoid hyperplasia (30). And prolonged exposure to such stimuli may lead to genetic instability and subsequent lymphocyte clonal transformation, ultimately resulting in POALs. While Ferreri et al. identified an association between Chlamydophila psittaci (Cps) and POALs, it is worth noting that the reported prevalence of Cps can vary significantly across different regions (28,31).
There are many prognostic factors related to POALs, including pathological type, primary site, clinical stage, etc. (32-34). Among them, the pathological type is the key factor in determining prognosis. Elderly patients often have more comorbidities and cannot tolerate highly toxic intensive treatment, which may directly have a negative impact on survival time.
Different pathological subtypes of lymphoma cells have varying levels of invasiveness, leading to differing degrees of malignancy and outcomes. Currently, there is no standardized protocol or set of guidelines for the treatment of POAL (19,28,30). Researches have shown that surgical treatment alone was more likely to result in long-term recurrence (28,35). Thus, a combination of surgery, radiation, and CT is commonly utilized in the treatment of POAL (27). The treatment plan for POAL is based on the patient’s age, cancer stage, histologic type, and clinical manifestations at the time of diagnosis (19,36). The particular surgical method employed depends on the location, scope, and type of the lesion. Radiation therapy is now considered a feasible option for treating low-grade malignant POAL, providing a high rate of local remission whilst avoiding systemic side effects often associated with CT (35,36). Patients with various POAL subtypes have seen higher remission rates after receiving radiation therapy. In cases where there is highly malignant ocular adnexal lymphoma and/or systemic involvement, CT is necessary to reduce side effects and prolong survival (35). There are now numerous new treatment methodologies being employed for POAL treatment, providing patients with an increasing number of options. Cps infection is one of the potential pathogenic factors associated with POALs, making the treatment of primary infections a major focus of research for managing POALs (31). Radioimmunotherapy, which involves monoclonal antibodies coupled with radioactive nuclides targeted at human lesions, has been shown to provide maximum anti-tumor effects with minimal risk of damage to other areas of the body (37,38). This is a viable treatment alternative for patients with refractory and recurrent B-cell-derived ocular lymphomas. However, this therapy is yet to become a standard treatment, and as such, its use is relatively limited.
Due to the limited incidence rate of POALs, there is insufficient POAL data in literature and a lack of prognostic models at present (39). Recently, nomogram models have been recommended as crucial tools for personalized patient survival prediction in clinical management (34,40,41). Consequently, following multivariate analysis, we identified seven optimal variables and developed high-performance predictive models for POAL patients over 2, 5, 10, and 15 years. Our nomogram demonstrated excellent predictive proficiency for the clinical outcomes of these patients in both the training and testing groups. Furthermore, the reliability and accuracy of our prediction model were confirmed with the use of the C-index, ROC, AUC, and calibration curve to verify the predicted values. To facilitate the model’s clinical application, we developed an online survival calculator, accessible through the webpage (https://helloshinyweb.shinyapps.io/eye_dynamic_nomogram/). By inputting patient variables and time, survival probability with a 95% CI can be quickly obtained.
There are a few limitations worth noting in this study. To begin with, as a retrospective study, some degree of selection bias is unavoidable. Secondly, to ensure more reliable results, it is advisable to use another large-scale independent dataset for external validation. Moreover, the SEER database lacks detailed data on certain critical variables, such as quality of life, vision, and preoperative laboratory results, which are significant for POAL patient prognosis and may impact the predictive model. Lastly, advancements in lymphoma examination and diagnostic techniques may affect our findings. Nonetheless, we validated our results in the validation cohort and observed the nomogram’s good performance, delivering useful information on POAL prognostic factors and patient survival.
Conclusions
In this study, we examined possible prognostic factors in patients diagnosed with POAL and created a dependable nomogram for predicting their OS. The nomogram demonstrated clinical utility and could assist healthcare professionals in evaluating risk factors for poor prognosis and developing personalized treatment strategies. Future research is necessary to validate our findings.
Acknowledgments
The authors would like to thank the SEER database for the availability of the data.
Funding: None.
Footnote
Reporting Checklist: The authors have completed the TRIPOD reporting checklist. Available at https://tcr.amegroups.com/article/view/10.21037/tcr-23-1556/rc
Peer Review File: Available at https://tcr.amegroups.com/article/view/10.21037/tcr-23-1556/prf
Conflicts of Interest: All authors have completed the ICMJE uniform disclosure form (available at https://tcr.amegroups.com/article/view/10.21037/tcr-23-1556/coif). The authors have no conflicts of interest to declare.
Ethical Statement: The authors are accountable for all aspects of the work in ensuring that questions related to the accuracy or integrity of any part of the work are appropriately investigated and resolved. The study was conducted in accordance with the Declaration of Helsinki (as revised in 2013).
Open Access Statement: This is an Open Access article distributed in accordance with the Creative Commons Attribution-NonCommercial-NoDerivs 4.0 International License (CC BY-NC-ND 4.0), which permits the non-commercial replication and distribution of the article with the strict proviso that no changes or edits are made and the original work is properly cited (including links to both the formal publication through the relevant DOI and the license). See: https://creativecommons.org/licenses/by-nc-nd/4.0/.
References
- Baldini L, Blini M, Guffanti A, et al. Treatment and prognosis in a series of primary extranodal lymphomas of the ocular adnexa. Ann Oncol 1998;9:779-81. [Crossref] [PubMed]
- Hsu CR, Chen YY, Yao M, et al. Orbital and ocular adnexal lymphoma: a review of epidemiology and prognostic factors in Taiwan. Eye (Lond) 2021;35:1946-53. [Crossref] [PubMed]
- Holm F, Mikkelsen LH, Kamper P, et al. Ocular adnexal lymphoma in Denmark: a nationwide study of 387 cases from 1980 to 2017. Br J Ophthalmol 2021;105:914-20. [Crossref] [PubMed]
- Rubinstein TJ, Aziz HA, Bellerive C, et al. Ocular/adnexal lymphoma: dissimilar to systemic lymphoma. Surv Ophthalmol 2018;63:381-8. [Crossref] [PubMed]
- Olsen TG, Heegaard S. Orbital lymphoma. Surv Ophthalmol 2019;64:45-66. [Crossref] [PubMed]
- Raderer M, Kiesewetter B, Ferreri AJ. Clinicopathologic characteristics and treatment of marginal zone lymphoma of mucosa-associated lymphoid tissue (MALT lymphoma). CA Cancer J Clin 2016;66:153-71. [Crossref] [PubMed]
- Sasai K, Yamabe H, Dodo Y, et al. Non-Hodgkin's lymphoma of the ocular adnexa. Acta Oncol 2001;40:485-90. [Crossref] [PubMed]
- Moslehi R, Devesa SS, Schairer C, et al. Rapidly increasing incidence of ocular non-hodgkin lymphoma. J Natl Cancer Inst 2006;98:936-9. [Crossref] [PubMed]
- Aronow ME, Portell CA, Rybicki LA, et al. Ocular adnexal lymphoma: assessment of a tumor-node-metastasis staging system. Ophthalmology 2013;120:1915-9. [Crossref] [PubMed]
- Sassone M, Ponzoni M, Ferreri AJ. Ocular adnexal marginal zone lymphoma: Clinical presentation, pathogenesis, diagnosis, prognosis, and treatment. Best Pract Res Clin Haematol 2017;30:118-30. [Crossref] [PubMed]
- Peng F, Su W, Zhang A, et al. Investigation of epidemiological characteristics and development of a nomogram to predict survival in primary ocular adnexal lymphoma. Clin Exp Ophthalmol 2022;50:615-31. [Crossref] [PubMed]
- Rafizadeh SM, Ghahvehchian H, Rajabi MT, et al. Anaplastic Large Cell Lymphoma With Intraocular or Ocular Adnexal Involvement: A Case Report and Systematic Review. Ophthalmic Plast Reconstr Surg 2023. [Epub ahead of print]. doi:
10.1097/IOP.0000000000002520 .10.1097/IOP.0000000000002520 - Baykal C, Atci T. Eyelid involvement of primary cutaneous lymphomas: A clinical perspective. Australas J Dermatol 2023;64:463-75. [Crossref] [PubMed]
- Wei M, Chen H, Wang JH, et al. Intraocular invasion of bilateral primary ocular adnexal mantle cell lymphoma: a case report. Zhonghua Yan Ke Za Zhi 2023;59:52-6. [PubMed]
- Manta AI, Wilson-Pogmore AA, Sullivan TJ. Thirty Years of Experience With Ocular Adnexal T-Cell Lymphoma. Ophthalmic Plast Reconstr Surg 2023;39:266-74. [Crossref] [PubMed]
- Al Jabri S, Laiq S, Matah P, et al. Primary unilateral conjunctival small lymphocytic lymphoma in a child: Case report and literature review. Oman J Ophthalmol 2023;16:318-21. [PubMed]
- Tagami M, Nishio M, Katsuyama-Yoshikawa A, et al. Machine Learning Model with Texture Analysis for Automatic Classification of Histopathological Images of Ocular Adnexal Mucosa-associated Lymphoid Tissue Lymphoma of Two Different Origins. Curr Eye Res 2023;48:1195-202. [Crossref] [PubMed]
- Iuliano A, Fossataro F, Laezza MP, et al. Primary cutaneous anaplastic large-cell lymphoma of the eyelid: report of two cases and review of the literature. Orbit 2021;40:481-7. [Crossref] [PubMed]
- Yen MT, Bilyk JR, Wladis EJ, et al. Treatments for Ocular Adnexal Lymphoma: A Report by the American Academy of Ophthalmology. Ophthalmology 2018;125:127-36. [Crossref] [PubMed]
- Yang Y, Jin X, Wang Y, et al. Clinical characteristics and treatment outcomes of secondary ocular adnexal lymphoma. Clin Exp Ophthalmol 2023;51:154-61. [Crossref] [PubMed]
- Pellicelli AM, Zoli V, Remotti D. Ocular adnexal lymphoma and infectious agents. Indian J Ophthalmol 2009;57:72-3; author reply 73. [Crossref] [PubMed]
- Ma Z, Yang S, Yang Y, et al. Development and validation of prediction models for the prognosis of colon cancer with lung metastases: a population-based cohort study. Front Endocrinol (Lausanne) 2023;14:1073360. [Crossref] [PubMed]
- Wen Y, Bai J, Zheng C, et al. A nomogram for predicting the risk of male breast cancer for overall survival. Front Oncol 2023;13:1068187. [Crossref] [PubMed]
- Wu J, Zhang H, Li L, et al. A nomogram for predicting overall survival in patients with low-grade endometrial stromal sarcoma: A population-based analysis. Cancer Commun (Lond) 2020;40:301-12. [Crossref] [PubMed]
- Zhang W, Ji L, Zhong X, et al. Two Novel Nomograms Predicting the Risk and Prognosis of Pancreatic Cancer Patients With Lung Metastases: A Population-Based Study. Front Public Health 2022;10:884349. [Crossref] [PubMed]
- Zhang Y, Zhang Z, Wei L, et al. Construction and validation of nomograms combined with novel machine learning algorithms to predict early death of patients with metastatic colorectal cancer. Front Public Health 2022;10:1008137. [Crossref] [PubMed]
- Pereira-Da Silva MV, Di Nicola ML, Altomare F, et al. Radiation therapy for primary orbital and ocular adnexal lymphoma. Clin Transl Radiat Oncol 2022;38:15-20. [Crossref] [PubMed]
- Ferreri AJ, Ponzoni M, Guidoboni M, et al. Regression of ocular adnexal lymphoma after Chlamydia psittaci-eradicating antibiotic therapy. J Clin Oncol 2005;23:5067-73. [Crossref] [PubMed]
- Ponzoni M, Govi S, Licata G, et al. A reappraisal of the diagnostic and therapeutic management of uncommon histologies of primary ocular adnexal lymphoma. Oncologist 2013;18:876-84. [Crossref] [PubMed]
- Ferreri AJ, Assanelli A, Crocchiolo R, et al. Therapeutic management of ocular adnexal MALT lymphoma. Expert Opin Pharmacother 2007;8:1073-83. [Crossref] [PubMed]
- Husain A, Roberts D, Pro B, et al. Meta-analyses of the association between Chlamydia psittaci and ocular adnexal lymphoma and the response of ocular adnexal lymphoma to antibiotics. Cancer 2007;110:809-15. [Crossref] [PubMed]
- Nola M, Lukenda A, Bollmann M, et al. Outcome and prognostic factors in ocular adnexal lymphoma. Croat Med J 2004;45:328-32. [PubMed]
- McKelvie PA. Ocular adnexal lymphomas: a review. Adv Anat Pathol 2010;17:251-61. [Crossref] [PubMed]
- Frimmel SA, Kniestedt C, Töteberg-Harms M, et al. Lymphoma of the ocular adnexa. Klin Monbl Augenheilkd 2014;231:394-404. [Crossref] [PubMed]
- Rehn S, Elsayad K, Oertel M, et al. Radiotherapy Dose and Volume De-escalation in Ocular Adnexal Lymphoma. Anticancer Res 2020;40:4041-6. [Crossref] [PubMed]
- Fung CY, Tarbell NJ, Lucarelli MJ, et al. Ocular adnexal lymphoma: clinical behavior of distinct World Health Organization classification subtypes. Int J Radiat Oncol Biol Phys 2003;57:1382-91. [Crossref] [PubMed]
- Cheuk W, Yuen HK, Chan AC, et al. Ocular adnexal lymphoma associated with IgG4+ chronic sclerosing dacryoadenitis: a previously undescribed complication of IgG4-related sclerosing disease. Am J Surg Pathol 2008;32:1159-67. [Crossref] [PubMed]
- Yoon J, Kim KJ, Sa HS. Clinical analysis of ocular adnexal mucosa-associated lymphoid tissue lymphoma with IgG4-related ophthalmic disease. Orbit 2022;41:551-7. [Crossref] [PubMed]
- Moslehi R, Schymura MJ, Nayak S, et al. Ocular adnexal non-Hodgkin's lymphoma: a review of epidemiology and risk factors. Expert Rev Ophthalmol 2011;6:181-93. [Crossref] [PubMed]
- Zhang W, Ji L, Wang X, et al. Nomogram Predicts Risk and Prognostic Factors for Bone Metastasis of Pancreatic Cancer: A Population-Based Analysis. Front Endocrinol (Lausanne) 2022;12:752176. [Crossref] [PubMed]
- Wang J, Tang J, Chen T, et al. A web-based prediction model for overall survival of elderly patients with early renal cell carcinoma: a population-based study. J Transl Med 2022;20:90. [Crossref] [PubMed]