The diagnostic, prognostic, drug sensitivity and ceRNA internet of PPP4R1 in liver hepatocellular carcinoma (LIHC)
Highlight box
Key findings
• Protein phosphatase 4 regulatory subunit 1 (PPP4R1) may be a prognostic biomarker and a potential target for immunotherapy in liver hepatocellular carcinoma (LIHC).
What is known and what is new?
• LIHC has a high incidence but a poor prognosis.
• PPP4R1 can predict the prognosis of LIHC.
What is the implication, and what should change now?
• The role of PPP4R1 in LIHC needs further study.
Introduction
Protein phosphatase 4 regulatory subunit 1 (PPP4R1, also referred to as PP4R1), serves as the regulatory subunit of protein phosphatase 4 (PP4). The existing literature (1,2) suggests that targeted interference and inhibition of PP4 activity may have potential benefits for tumor immunotherapy. It has been shown that inhibition of PP4 activity enhances cisplatin sensitivity, reduces the DNA damage response, and hinders tumor growth and progression (3). Nevertheless, research on PPP4R1 in the context of tumors remains limited.
Based on available reports, it has been observed that PPP4R1 hinders activation of the nuclear factor kappa-B (NF-κB) pathway in T cells and T lymphocytes by interacting with tumor necrosis factor receptor-associated factor molecules (4,5). Moreover, PPP4R1 has been found to enhance the migration and metastasis of non-small cell lung cancer (NSCLC) by binding to the high-mobility group A2 (HMGA2) (6). Furthermore, Xiang et al. demonstrated that long non-coding RNA (lncRNA) insulin growth factor 2 antisense (IGF2-AS) facilitates the progression of thyroid cancer by modulating the miR-500a-3p/PPP4R1/phospho-vascular endothelial growth factor receptor 2 (p-VEGFR2) signalling pathway (7). However, the specific role of PPP4R1 in other cancers remains unclear, for example, liver hepatocellular carcinoma (LIHC).
In this study, exploring the expression profile, diagnostic and prognostic value of PPP4R1 were explored in pan-cancer by R software. Analysis of the correlation between PPP4R1 and 24 immune cells was performed. Additionally, RNA sequencing data and anti-cancer drug data were obtained from the CellMiner database (8) and were used to assess the correlation between PPP4R1 and anti-cancer drugs using the R software. Furthermore, prediction and construction of the competitive endogenous RNA (ceRNA) network were done through online databases in LIHC. Finally, experiments were conducted to elucidate the expression of PPP4R1 at both the tissue and cellular levels, as well as to ascertain the localization of PPP4R1 within LIHC cells. We hypothesized that PPP4R1 is a new biomarker for LIHC and may be a target for LIHC immunotherapy. We present this article in accordance with the MDAR and REMARK reporting checklists (available at https://tcr.amegroups.com/article/view/10.21037/tcr-23-1744/rc).
Methods
Analysis of differential gene expression
RNA sequencing data and clinical data of 18,102 cases were downloaded from the University of California Santa Cruz (UCSC) Xena database (https://xenabrowser.net/datapages/) in August 2022, including 7,568 cases of Genotype-Tissue Expression (GTEx) normal data, 727 cases of The Cancer Genome Atlas (TCGA) paracancer data and 9,807 cases of TCGA tumour data; both GTEx normal data and TCGA paracancer data were included in the normal group, and TCGA tumour data were included in the tumour group. The analysis of PPP4R1 expression between tumor samples and normal samples was performed using the ggplot2 package in R software (V4.2.1). The differences between the two groups of data were analyzed using the Wilcoxon rank-sum test. The threshold for statistical significance was established as P<0.05.
Diagnostic value analysis
The cancer diagnostic importance of PPP4R1 was assessed by employing a receiver operating characteristic (ROC) curve. A low diagnostic value was indicated by an area under the curve (AUC) between 0.5 and 0.7, while an intermediate diagnostic value was indicated by an AUC between 0.7 and 0.9, and a high diagnostic value was indicated by an AUC greater than 0.9.
Survival analysis
The overall survival (OS) rate of cancer patients was assessed using Kaplan-Meier survival curves to examine the influence of PPP4R1 expression. When hazard ratio (HR) >1, a higher level of PPP4R1 expression was linked to a poorer prognosis, while when HR <1, a lower level of PPP4R1 expression was linked to a poorer prognosis. The threshold for statistical significance was established as P<0.05.
Correlation between PPP4R1 expression levels and clinical factors
The samples were categorized into groups based on the median of PPP4R1 expression values, distinguishing between high-expression and low-expression groups. Afterwards, the R software was utilized to examine the disparities in clinical variables between the two groups. The threshold for statistical significance was established as P<0.05.
Prognostic factors analysis
RNA sequencing data and clinical data for TCGA-LIHC were downloaded from the TCGA database (https://portal.gdc.cancer.gov), and prognostic data were obtained from a cell article (9). The R software was used to perform univariate cox regression analysis on clinical variables, including pathologic stage, M stage, PPP4R1, histologic grade. These variables that had a P value lower than 0.05 were subsequently incorporated into the multifactorial cox regression analysis. An independent prognostic factor for the cancer was considered when the P value was below 0.05.
Protein-protein interaction (PPI) network construction and Gene Ontology (GO) and Kyoto Encyclopedia of Genes and Genomes (KEGG) enrichment analysis
The identification of differentially expressed genes (DEGs) of PPP4R1 in LIHC was conducted using R software, Volcano maps were used for visualization. The PPP4R1 co-expression genes were screened using STRING data (10) with the following conditions: network type: full STRING network; network edge meaning: evidence; active interaction sources: text mining, experiments, databases and co-expression; minimum required interaction score: medium confidence (0.400); maximum number of interactors to be displayed: no more than 50 interactors. Visualisation of co-expressed genes was done by using cytoscape software. Subsequently, the GO and KEGG enrichment analyses of the co-expression genes were performed using the clusterProfiler R package (11).
Immune cell infiltration and gene correlation analysis
The correlation between PPP4R1 and the 24 immune cells was investigated using R software and the single sample gene set enrichment analysis (ssGSEA) algorithm. Subsequently, the TISIDB (an integrated repository portal for tumor-immune system interactions) database (12) was used to identify immunoinhibitor related genes linked to PPP4R1 and to generate a molecular correlation scatter plot.
Drug sensitivity analysis
RNA sequencing data and drug data were obtained from the CellMiner database. Subsequently, the correlation between genes and drugs was examined using the impute package and limma package in the R software. Additionally, a correlation scatterplot was generated using ggplot2 and ggpubr packages.
Prediction and construction of a ceRNA network for PPP4R1
The miRWalk (mRNA-miRNA interactions) database (13) and MicroT-CDS (mRNA-miRNA interactions) database (14) were used to predict the miRNAs that targeted PPP4R1. Additionally, the Encyclopedia of RNA Interactomes (ENCORI) database (15) and LncBase (miRNA-lncRNA interactions) database (16) were used to predict lncRNAs with the ability to bind to miRNAs. Subsequently, the correlation between PPP4R1 and miRNAs was analyzed using the TCGA-LIHC dataset. Only miRNAs that exhibited a negative correlation with PPP4R1 were retained. Next, the correlation between miRNAs and lncRNAs was explored, with a focus on retaining lncRNAs that displayed a negative correlation with miRNAs. Finally, the obtained results were visualized using cytoscape software to enhance the precision of predictions, and an analysis was conducted to examine the correlation between PPP4R1 and lncRNAs. The lncRNAs exhibiting the highest positive correlation coefficients with PPP4R1 were chosen as definitive members of the regulatory network.
Tissue and cell
Nine liver cancer tissues and paracancerous tissue specimens obtained from the First Affiliated Hospital of Hainan Medical College. LX-2, HLE, Huh7, PLC, and HepG2 cells were purchased from Wuhan Procell Life Technology Co., Ltd. Cells were cultured in Dulbecco’s modified eagle medium (DMEM) (Cat #C3113-0500, Viva cell, China) supplemented with 10% fetal bovine serum, (Cat #164210-50, Procell, China) 100 µg/mL penicillin, and 0.25 µg/mL streptomycin (Cat #15140-122, Gibco, United States). The cells were then incubated at 37 ℃ with 5% CO2. Once the cell population reached 5×106, trypsin was used to digest the cells and extract total protein for western blotting experiments. Additionally, 5×104 cells were used for immunofluorescence.
Western blotting
LX-2, HLE, Huh7, PLC and HepG2 cells were collected and lysed in lysis buffer (Cat #C510003, Sangon Biotech, China) for 40 min, then centrifuged at 12,000 rpm for 15 min at 4 ℃, and the supernatant was taken as the total protein of the cells, and the concentration of the total protein was determined by using BCA Protein Concentration Assay Kit (Cat #P0009, Beyotime, China). About 20ug of cell lysates was taken for 12% sodium dodecyl sulphate-polyacrylamide gel electrophoresis, and the proteins were subsequently transferred to a polyvinylidene fluoride (PVDF) membrane. The PVDF membrane was washed three times using phosphate buffered solution (PBST) buffer and then it was closed in protein free rapid blocking buffer (Cat #PS108, Epizyme, China) for 15 min, and then the membrane was incubated with a 1:2,000 dilution of PPP4R1 antibody (Cat #67392-1-Ig, Proteintech, China) and a 1:10,000 dilution of glyceraldehyde-3-phosphate dehydrogenase (GAPDH) antibody (Cat #10494-1-AP, Proteintech) at 4 ℃ overnight. The membranes were then incubated with HPR-conjugated secondary antibodies (Cat #SA00001-2, Proteintech) at 37 ℃ for 1 h. The membranes were incubated with enhanced chemiluminescence (ECL) substrate and detected on a luminescent device. Grey scale values were analysed using image J software to quantify the relative expression of the proteins.
Immunohistochemistry
The level of PPP4R1 protein was assessed in LIHC tissue specimens using immunohistochemistry. In brief, the formalin-fixed and paraffin-embedded LIHC tissue sections were deparaffinized in xylene and rehydrated in a series of ethanol solutions (100–50%) and into tape water. The sections in a citric buffer (pH 5.0) were subjected to the antigen repair in a microwave and then incubated in normal serum-PBS (1:20 dilution) at room temperature for 1 h and then with an anti-PPP4R1 antibody (Cat #67392-1-Ig, Proteintech) at 4 ℃ overnight. The following day, the sections were washed with PBS thrice and further incubated with a secondary antibody (Cat #GB23301, Servicebio, China) at a dilution of 1:200 at room temperature for 1 h. After brief washing with PBS, the sections were incubated with a 3,3'-diaminobenzidine (DAB) kit (Cat #G1212, Servicebio) at room temperature in the dark for 10 min and then incubated with DAB solution and counterstained with hematoxylin and mounted with coverslips. The stained sections were then reviewed and scored under a light microscope (E100, Nikon, Japan). Mean density were analysed using image J software to quantify the relative expression of the proteins.
Immunofluorescence
The HLE, Huh7 and LX-2 cells were placed in confocal dishes (Cat #BS-15-GJM, biosharp, China) and cultured overnight in an incubator, on the second day, take out the cell dishes and discard the medium and wash it 2 times with PBS, fix the cells with paraformaldehyde for 30 min, then wash 2 times with PBS, to block the non-specific signals, close it with the special sealing solution for 15 min and then wash it with PBS for 2 times, The PPP4R1 antibody (Cat #67392-1-Ig, Proteintech) was incubated overnight at 4 ℃, on the third day, the PPP4R1 antibody was recovered and incubated with the second antibody (Cat #SA00013-3, Proteintech) in the incubation room at 37 ℃ for 1 h after washing it 2 times with PBS, and then photographed by adding the 4',6-diamidino-2-phenylindole (DAPI) staining solution (Cat #C1006, Beyotime) and put into a laser confocal microscope for observation (LSM800, ZEISS, Germany).
Statistical analysis
Statistical software using R software (4.2.1). The chi-square test was used for count data and the independent samples T test was used for measurement data. The data of two groups satisfied normal distribution and homogeneity using T test, satisfied normal distribution did not satisfy homogeneity using welch t-test, and did not satisfy normal distribution using Wilcoxon rank sum test. Indicators with missing data ≥60% were excluded from the statistical analysis. P<0.05 was considered as statistically significant difference.
Ethical statement
The study was conducted in accordance with the Declaration of Helsinki (as revised in 2013). The study was approved by Ethics Committee of the First Affiliated Hospital of Hainan Medical College (No. 2023-KYS-161) and informed consent was taken from all the patients.
Results
Expression levels of PPP4R1 in pan-cancer
The expression level of PPP4R1 in pan-cancer was investigated by analyzing the pan-cancer RNA sequencing dataset with R software. The analysis revealed that PPP4R1 exhibited high expression in 11 tumors and low expression in 13 tumors in unpaired samples (Figure 1A). Furthermore, the analysis revealed that PPP4R1 exhibited high expression in four tumors and only one tumor displayed low expression (Figure 1B) in paired samples. Through the integration of PPP4R1 expression levels in tumor tissues and normal tissues, in both paired and unpaired samples, it was discovered that PPP4R1 exhibited high expression exclusively in cholangiocarcinoma (CHOL) and LIHC, while displaying low expression in kidney chromophobe (KICH).
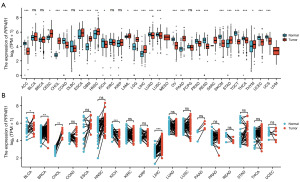
Diagnostic significance of PPP4R1 in pan-cancer
ROC curve analysis revealed that PPP4R1 exhibited diagnostic value for 14 different types of cancer, with an AUC greater than 0.7. The specific AUC values for each cancer type were as follows: LIHC (AUC =0.867); colon adenocarcinoma (COAD) (AUC =0.786); CHOL (AUC =1.000); rectum adenocarcinoma (READ) (AUC =0.858); uterine carcinosarcoma (UCS) (AUC =0.822); KICH (AUC =0.860); brain lower grade glioma (LGG) (AUC =0.869); pancreatic adenocarcinoma (PAAD) (AUC=0.950); ovarian serous cystadenocarcinoma (OV) (AUC =0.876); adrenocortical carcinoma (ACC) (AUC =0.861); oral squamous cell carcinoma (OSCC) (AUC =0.774); skin cutaneous melanoma (SKCM) (AUC =0.882); glioblastoma multiforme (GBM) (AUC =0.888); and testicular germ cell tumors (TGCT) (AUC =0.953) (Figure 2). According to these results, PPP4R1 has high diagnostic value in CHOL, PAAD, and TGCT (AUC >0.9), medium diagnostic value in LIHC, READ, UCS, KICH, LGG, OV, ACC, SKCM and GBM (AUC >0.8), low diagnostic value in COAD and OSCC (AUC >0.7).
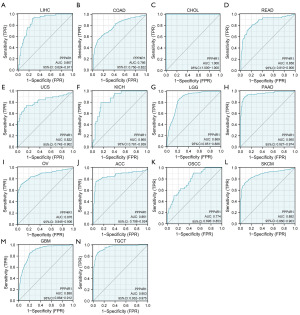
Prognostic significance of PPP4R1 in pan-cancer
Kaplan-Meier survival curves for LIHC, PAAD, sarcoma (SARC), and ACC demonstrated a significant association between PPP4R1 expression and OS in these cancer types. Specifically, high expression of PPP4R1 was found to be indicative of poor prognosis in patients with LIHC (HR =1.64, P=0.006), PAAD (HR =1.66, P=0.019) and SARC (HR =1.51, P=0.043), as depicted in Figure 3A-3C. However, in kidney renal clear cell carcinoma (KIRC), PPP4R1 was shown to be a protective factor for prognosis (HR =0.69, P=0.017) (Figure 3D). In Figure 3E, it is shown that PPP4R1 was a poor prognostic factor for ACC (HR =3.29, P=0.004).
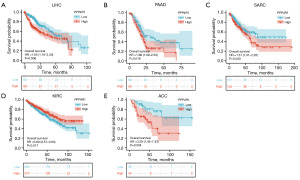
Association between PPP4R1 expression levels and different clinical features of LIHC
Based on our comprehensive analysis across multiple cancer types, it was observed that PPP4R1 was significantly upregulated specifically in LIHC. Furthermore, PPP4R1 demonstrated diagnostic and prognostic potential exclusively in the context of LIHC. Consequently, our subsequent investigation primarily focused on elucidating the association between PPP4R1 and LIHC. The baseline datasheet analysis revealed a statistically significant association between PPP4R1 expression levels and various clinical factors in LIHC, including pathologic T stage (P=0.664), pathologic M stage (P=0.123), gender (P=0.097), age (P=0.008), pathological stage (P=0.024), histological grading (P=0.004) and alpha-fetoprotein (AFP) levels (P<0.001) (Table 1). Notably, patients with age (≤60 years), pathological stages (III and IV), histological grades (G3 and G4), and AFP levels (>400 ng/mL) exhibited increased PPP4R1 expression levels (Figure 4).
Table 1
Characteristics | Low expression of PPP4R1 (N=187) | High expression of PPP4R1 (N=187) | P value |
---|---|---|---|
Pathologic T stage, n (%) | 0.664 | ||
T1 | 95 (25.4) | 88 (23.5) | |
T2 | 48 (12.8) | 47 (12.6) | |
T3 | 35 (9.4) | 45 (12.0) | |
T4 | 6 (1.6) | 7 (1.9) | |
Missing | 3 (0.8) | 0 | |
Pathologic M stage, n (%) | 0.123 | ||
M0 | 130 (34.7) | 138 (36.9) | |
M1 | 4 (1.1) | 0 | |
Missing | 53 (14.2) | 49 (13.1) | |
Gender, n (%) | 0.097 | ||
Female | 53 (14.2) | 68 (18.2) | |
Male | 134 (35.8) | 119 (31.8) | |
Age, n (%) | 0.008 | ||
≤60 years | 76 (20.3) | 101 (27.0) | |
>60 years | 111 (29.7) | 85 (22.7) | |
Missing | 0 | 1 (0.3) | |
Pathologic stage, n (%) | 0.024 | ||
Stage I | 89 (23.8) | 84 (22.5) | |
Stage II | 45 (12.0) | 42 (11.2) | |
Stage III | 33 (8.8) | 52 (13.9) | |
Stage IV | 5 (1.4) | 0 | |
Missing | 15 (4.0) | 9 (2.4) | |
Histologic grade, n (%) | 0.004 | ||
G1 | 35 (9.4) | 20 (5.3) | |
G2 | 97 (25.9) | 81 (21.7) | |
G3 | 50 (13.4) | 74 (19.8) | |
G4 | 3 (0.8) | 9 (2.4) | |
Missing | 2 (0.5) | 3 (0.8) | |
AFP, n (%) | <0.001 | ||
≤400 ng/mL | 126 (33.7) | 89 (23.8) | |
>400 ng/mL | 20 (5.3) | 45 (12.0) | |
Missing | 41 (11.0) | 53 (14.2) |
The significance level was set at P<0.05. PPP4R1, protein phosphatase 4 regulatory subunit 1; LIHC, liver hepatocellular carcinoma; AFP, alpha-fetoprotein.
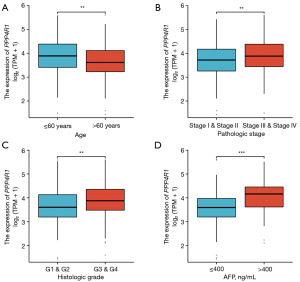
Cox regression analysis—single and multiple factors
To explore the prognostic factors for PPP4R1 in LIHC, cox regression analyses were performed. Univariate cox regression analysis revealed that pathological stage (HR =2.09, 95% CI: 1.429–3.055, P<0.001), M stage (HR =4.077, 95% CI: 1.281–12.973, P=0.017), and PPP4R1 (HR =1.643, 95% CI: 1.157–2.335, P=0.006) were associated with poor prognosis in LIHC (Table 2). In the multivariate cox regression analysis, pathological stage (HR =2.504, 95% CI: 1.570–3.994, P<0.001), M stage (HR =3.865, CI: 1.164–12.826, P=0.027), and PPP4R1 (HR =1.776, 95% CI: 1.131–2.787, P=0.013) were independent prognostic factors for the OS of LIHC patients (Table 2).
Table 2
Characteristics | Total (N) | Univariate analysis | Multivariate analysis | |||
---|---|---|---|---|---|---|
Hazard ratio (95% CI) | P value | Hazard ratio (95% CI) | P value | |||
Pathologic stage | 349 | |||||
Stage I | 173 | Reference | ||||
Stage II & III & IV | 176 | 2.090 (1.429–3.055) | <0.001 | 2.504 (1.570–3.994) | <0.001 | |
M stage | 272 | |||||
M0 | 268 | Reference | ||||
M1 | 4 | 4.077 (1.281–12.973) | 0.017 | 3.865 (1.164–12.826) | 0.027 | |
PPP4R1 | 374 | |||||
Low | 187 | Reference | ||||
High | 187 | 1.643 (1.157–2.335) | 0.006 | 1.776 (1.131–2.787) | 0.013 | |
Histologic grade | 368 | |||||
G1 & G2 | 233 | Reference | ||||
G3 & G4 | 135 | 1.091 (0.761–1.564) | 0.636 |
The significance level was set at P<0.05. LIHC, liver hepatocellular carcinoma; CI, confidence interval; PPP4R1, protein phosphatase 4 regulatory subunit 1.
PPP4R1 differential expression analysis, PPI network construction and GO and KEGG enrichment analysis in LIHC
To investigate the biological function of PPP4R1, R software was used to screen the DEGs of PPP4R1 in LIHC and the results were visualized by volcano plot (Figure 5A), then PPP4R1 co-expression genes were explored using STRING database, and a total of 50 co-expression genes were obtained, which were visualised by cytoscape (Figure 5B). Finally, these 50 genes were analysed by GO and KEGG enrichment, and the results of GO enrichment analysis showed that the biological processes (BP) mainly included dephosphorylation, negative regulation of phosphate metabolism process, cell growth, mitotic cell cycle phase transition and calcineurin-NFAT signalling cascade; cellular components (CC) mainly included protein serine/threonine phosphatase complex, protein phosphatase type 2A complex, chromosome, centromeric region, spindle and glutamatergic synapse; molecular functions (MF) mainly included protein phosphatase regulator activity, phosphoric ester hydrolase activity, protein phosphatase binding, tau protein binding and cyclosporin A binding (Figure 5C). KEGG pathway enrichment was mainly associated with phosphatidylinositol 3-kinase (PI3K)-Akt signalling pathway, AMP-activated protein kinase (AMPK) signalling pathway, mRNA surveillance signalling pathway, Hippo signalling pathway, Wnt signalling pathway, programmed cell death-ligand 1 (PD-L1) expression and programmed cell death protein 1 (PD-1) checkpoint pathway in cancer, and NF-κB signalling pathway. In addition, KEGG enrichment was observed to associate PPP4R1 with hepatitis C, cellular senescence and natural killer (NK) cell-mediated cytotoxicity (Figure 5D).
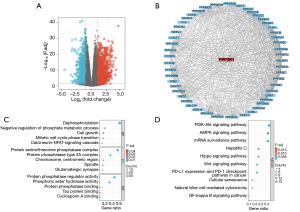
Immune infiltration and gene correlation analysis
Using the R package, we employed the ssGSEA algorithm to investigate the correlation between PPP4R1 and 24 different types of immune cells (Figure 6A). The results showed that PPP4R1 had a strong positive relationship with Th2 cells, helper T cells, Tem, Tcm, macrophages, NK CD56bright cells and follicular helper T cell (TFH), with correlation coefficients (R-values) of 0.382 (P<0.001), 0.367 (P<0.001), 0.270 (P<0.001), 0.227 (P<0.001), 0.190 (P<0.001), 0.188 (P<0.001) and 0.156 (P<0.01), respectively. In addition, PPP4R1 had a strong negative relationship with Th17 cells, with correlation coefficients (R-value) of −0.394 (P<0.001). Furthermore, the association between PPP4R1 and genes related to immunoinhibitors was examined by utilizing the TISIDB database. The findings showed notable associations between PPP4R1 and programmed cell death protein 1 (PDCD1), hepatitis A virus-cellular receptor 2 (HAVCR2), cytotoxic T lymphocyte antigen 4 (CTLA4), and transforming growth factor-beta1 (TGF-β1), with correlation coefficients of 0.19 (P<0.001), 0.172 (P<0.001), 0.153 (P=0.0031), and 0.22 (P<0.001), respectively (Figure 6B-6E).
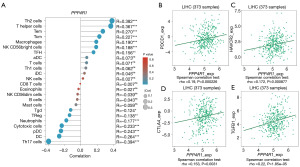
Drug sensitivity analysis
Anti-cancer drugs are important in the treatment of cancer; however, resistance often develops during treatment, so it is crucial to study the relationship between genes and the sensitivity of anti-cancer drugs. As shown in Figure 7, the expression of PPP4R1 was negatively correlated with the half maximal inhibitory concentration (IC50) values of tamoxifen, actinomycin D, vemurafenib, ergenyl, crizotinib, PF-06463922, homoharringtonine, Denileukin diftitox ontology, and LDK-378 (P<0.05). Conversely, a positive correlation was observed between the expression of PPP4R1 and the IC50 value of fludarabine (P<0.05). This suggests that higher expression levels of PPP4R1 are associated with increased resistance to fludarabine.
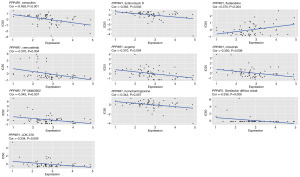
Predicting and constructing ceRNA regulatory networks
The importance of ceRNA regulatory networks in LIHC has been established by previous research. Hence, the process of forecasting and building ceRNA networks was carried out. The miRWalk and MicroT-CDS databases predicted a total of 122 and 199 miRNAs, respectively. The findings shown in Figure 8A demonstrated a group of miRNAs that were shared by both databases, specifically hsa-miR-320b, hsa-miR-1298-3p, hsa-miR-3120-3p, hsa-miR-5189-5p, hsa-miR-22-3p, hsa-miR-488-5p, hsa-miR-6738-3p, and hsa-miR-6738-3p. Further examinations of the connection between miRNAs and PPP4R1 expression levels unveiled a noteworthy inverse relationship between hsa-miR-22-3p and PPP4R1 in LIHC (R=−0.434, P<0.001).
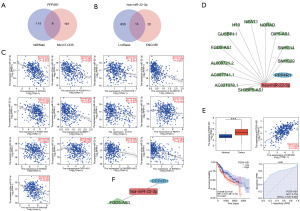
Furthermore, the ENCORI and Lncbase databases were employed for the prediction of lncRNAs that targeted hsa-miR-22-3p, revealing predictions of 34 and 844 lncRNAs, respectively. Figure 8B displayed a group of lncRNAs, including AC021078.1, AC087741.1, AL008721.2, AL390294.1, FGD5 antisense RNA 1 (FGD5-AS1), GUSBP11, H19, LINC00963, NEAT1, NORAD, OIP5-AS1, SH3BP5-AS1, SNHG14, and SNHG29, which were found in both databases. Afterwards, the association between these 14 long non-coding RNAs (lncRNAs) and the level of expression of hsa-miR-22-3p was investigated. A total of 12 lncRNAs were found to have a negative correlation with hsa-miR-22-3p, specifically AC087741.1 (R=−0.393, P<0.001), AC021078.1 (R=−0.211, P<0.001), AL008721.2 (R=−0.408, P<0.001), FGD5-AS1 (R=−0.324, P<0.001), GUSBP11 (R=−0.302, P<0.001), H19 (R=−0.238, P<0.001), NEAT1 (R=−0.117, P=0.025), NORAD (R=−0.217, P<0.001), OIP5-AS1 (R=−0.199, P<0.001), SH3BP5-AS1 (R=−0.179, P<0.001), SNHG14 (R=−0.383, P<0.001), and SNHG29 (R=−0.312, P<0.001) (Figure 8C) were observed. Figure 8D displays the maps of the ceRNA regulatory network.
In order to enhance the precision of the prediction, an analysis was conducted on the relationship between lncRNAs and PPP4R1 in LIHC. The findings indicated that only FGD5-AS1 expression was increased, displaying a positive correlation with PPP4R1, and possessing diagnostic (AUC =0.774) and prognostic (HR =1.51, P=0.021) significance in LIHC (Figure 8E). The growth of LIHC may be influenced by the FGD5-AS1–hsa-miR-22-3p–PPP4R1 ceRNA network, as suggested by these findings. Figure 8F displays the ultimate depiction of the ceRNA network.
Experimental verification
To validate the concordance between the PPP4R1 expression level and the findings from TCGA data analysis, western blotting experiments was performed at the cellular level and IHC experiments was performed at the tissue level. The results of western blotting experiments demonstrated that the expression level of PPP4R1 was elevated in HLE, Huh7, and HepG2 cells compared to that in LX-2 cells, however, PPP4R1 is lowly expressed in PLC cells (Figure 9A). Then, image J software was used to calculate the gray value of PPP4R1 expression in LIHC cells and hepatocytes, and a histogram was made (Figure 9B). IHC experiments analysis revealed a higher expression level of PPP4R1 in tumor tissues than in paracancerous tissues (Figure 9C,9D), which aligns with the findings of TCGA analysis. To examine the cellular localization of PPP4R1, immunofluorescence experiments were conducted on LX-2, Huh7, and HLE cells. The results demonstrated that PPP4R1 was predominantly localized in the cytoplasm and the cell membrane (Figure 10).
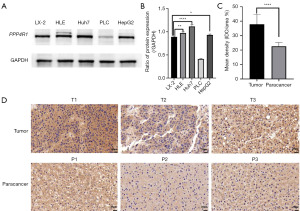
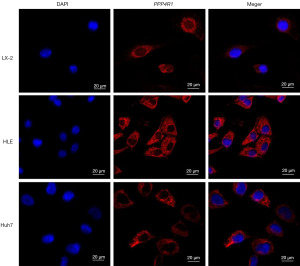
Discussion
Recent research indicates that the removal of phosphate groups from proteins raises the likelihood of tumor formation. One such example is the activation of the src homology-2 domain-containing protein tyrosine phosphatase-2 (SHP2) protein (17), which contains the src homology 2 domain and acts as a tyrosine phosphatase. This protein, encoded by PTPN11, significantly increases the susceptibility to leukemia in children and is now being targeted for cancer treatment. The primary role of PPP4R1 is protein dephosphorylation, thus, increased PPP4R1 expression may facilitate tumor advancement. Previous research has shown that PPP4R1 expression is higher in lung cancer tissues compared to normal lung tissues, and it can enhance the migration and metastasis of NSCLC (6). The findings indicate that cancer therapy could potentially target PPP4R1 as well.
In this research, the levels of PPP4R1 expression were investigated in different cancer types, and its increase was observed in CHOL and LIHC. Additionally, through the integration of clinical data, PPP4R1 was found to have diagnostic value in 14 cancers and prognostic value in 5 cancers, but with both diagnostic and prognostic value only in LIHC. Consequently, subsequent investigations focused on elucidating the specific role of PPP4R1 in LIHC. Clinical parameters indicated that PPP4R1 was primarily linked to pathological stage, age, histological grade, and AFP level. AFP is a well-established diagnostic indicator for LIHC and is recognized for promoting growth, infiltration, spread, and evasion of the immune system (18,19). As a result, it has a vital function in the development and advancement of LIHC. Thus, it is plausible to suggest that PPP4R1 might also facilitate the development and advancement of LIHC. The ROC curve indicated that PPP4R1 exhibited a moderate diagnostic significance in LIHC. Consequently, utilizing PPP4R1 and AFP in combination as a diagnostic indicator for LIHC might enhance the diagnostic precision of LIHC. According to the cox regression analysis, the prognosis in LIHC can be predicted independently by factors such as pathological stage, M stage, and PPP4R1.The findings offer valuable insights for the treatment and identification of LIHC in a clinical setting.
Furthermore, the GO enrichment analysis indicated that PPP4R1 was primarily linked to cellular proliferation and the cell cycle. According to the KEGG enrichment analysis, the signaling pathways linked to PPP4R1 primarily included PI3K-Akt, Wnt, AMPK, Hippo, and NF-κB, which have been demonstrated to facilitate the advancement of cancer (20-23). This implies that PPP4R1 might also contribute to the progression of cancer. Furthermore, the KEGG enrichment analysis revealed a correlation between PPP4R1 and NK cell-induced cytotoxicity, as well as PD-L1 expression within tumors and the PD-1 checkpoint pathway. These findings strongly suggest the potential involvement of PPP4R1 in immunotherapy. In order to further examine this hypothesis, an analysis of immune infiltration was performed in LIHC, revealing a strong association between PPP4R1 and NK CD56bright cells. The results indicated that PPP4R1 may have the ability to regulate NK cells in order to modify immunotherapy in tumors. Subsequent correlation analysis was conducted between PPP4R1 and genes related to immunoinhibition, revealing a positive association between PPP4R1 and PDCD1, HAVCR2, CTLA4, and TGF-β1. The PDCD1 gene produces PD-1 proteins in T cells, and the PD-1 receptor on T cells' surface can interact with PD-L1 ligands on tumour cells’ surface, inhibiting T cell activity (24). Additionally, KEGG enrichment analysis revealed PPP4R1’s association with PD-1 and PD-L1 checkpoint signaling pathways, As PD-L1 has been shown to promote tumour immune escape (25), thus, PPP4R1 might also facilitate immune evasion by tumour cells. Tim-3, also referred to as HAVCR2, was found to hinder the PI3K signaling pathway, consequently obstructing NK cell immune surveillance against LIHC cells and promoting their growth (26). This suggests that PPP4R1 might play a role in regulating the immune function of NK cells, aligning with the KEGG enrichment analysis. CTLA4, a gene associated with checkpoints, has a crucial function in suppressing the activation of T cells and has been utilized as a focal point for tumor immunotherapy (27). Therefore, PPP4R1 may also be a potential target for tumour immunotherapy. TGF-β1, the primary constituent of the TGF-β family, has been identified as a significant marker for the advancement of hepatitis C to cirrhosis and hepatocellular carcinoma (28). The KEGG enrichment analysis findings indicated that PPP4R1 was also linked to hepatitis C. Hence, PPP4R1 might possess the potential to facilitate the progression of hepatitis C to hepatocellular carcinoma.
Following this, an analysis of drug sensitivity demonstrated a direct association between the PPP4R1 expression level and the IC50 value of fludarabine. According to a prior investigation (29), it was found that fludarabine increased the harmful effects of NK cells on LIHC cells. Hence, combining fludarabine with NK cells during the treatment of LIHC patients might boost the effectiveness of therapy. Furthermore, the suppression of PPP4R1 expression may lead to further improvements in therapeutic efficacy. The discovery highlights the role of PPP4R1 in LIHC immunotherapy. Online databases were utilized to predict and construct ceRNA networks in order to identify upstream targets of PPP4R1. In conclusion, the ceRNA network consists of FGD5-AS1, hsa-miR-22-3p, and PPP4R1, with FGD5-AS1 and hsa-miR-22-3p identified as upstream targets of PPP4R1. According to a research, it was found that FGD5-AS1 contributes to the development of lenvatinib resistance in hepatocellular carcinoma (30), and PPP4R1 is associated with fludarabine resistance, which implies that FGD5-AS1–hsa-miR-22-3p–PPP4R1 ceRNA network might be linked to drug resistance in LIHC. Finally, experiments were conducted to verify the expression of PPP4R1 protein in both cells level and tissues level. According to the western blotting experimental findings, the PPP4R1 protein exhibited significant elevation in three LIHC cell lines (HLE, Huh7, and HepG2) in comparison to normal hepatocytes (LX-2). The experimental results from IHC also demonstrated a notable increase in PPP4R1 expression in cancerous tissues when compared to adjacent paracancerous tissues. Furthermore, immunofluorescence experiments revealed that PPP4R1 was present in both the cytoplasm and cell membrane. This finding further supports the potential of PPP4R1 as an immunotherapeutic target for LIHC, as it is located on the cell membrane. These results indicate that PPP4R1, a cancer-causing gene, could potentially serve as a new indicator for LIHC.
This paper examined the mRNA and protein levels of PPP4R1, investigates its role in the clinic, and reveals its biological functions, drug sensitivity, and upstream targets. Nevertheless, the majority of these findings were anticipated based on publicly available information. Subsequent investigations will conduct cellular assays to delve deeper into the impact of PPP4R1 on the characteristics of LIHC cells. To detect alterations in NK cell toxicity to LIHC cells, co-culturing of NK cells with LIHC cells will be conducted. The impact of fludarabine on apoptosis of LIHC cells will be assessed using flow cytometry. Using a dual luciferase reporter assay to verify the FGD5-AS1–hsa-miR-22-3p–PPP4R1 ceRNA network.
Conclusions
To summarize, the research indicates a notable link between increased PPP4R1 levels and the diagnosis of LIHC, as well as a negative outlook for the patient’s prognosis. Moreover, this heightened manifestation could potentially lead to resistance against drugs and impede the effectiveness of immunotherapy in LIHC. Hence, targeting PPP4R1 could be an innovative method for treating LIHC. The results offer valuable perspectives on the clinical treatment of patients with LIHC.
Acknowledgments
Funding: This work was supported by
Footnote
Reporting Checklist: The authors have completed the MDAR and REMARK reporting checklists. Available at https://tcr.amegroups.com/article/view/10.21037/tcr-23-1744/rc
Data Sharing Statement: Available at https://tcr.amegroups.com/article/view/10.21037/tcr-23-1744/dss
Peer Review File: Available at https://tcr.amegroups.com/article/view/10.21037/tcr-23-1744/prf
Conflicts of Interest: All authors have completed the ICMJE uniform disclosure form (available at https://tcr.amegroups.com/article/view/10.21037/tcr-23-1744/coif). The authors have no conflicts of interest to declare.
Ethical Statement: The authors are accountable for all aspects of the work in ensuring that questions related to the accuracy or integrity of any part of the work are appropriately investigated and resolved. The study was conducted in accordance with the Declaration of Helsinki (as revised in 2013). The study was approved by Ethics Committee of the First Affiliated Hospital of Hainan Medical College (No. 2023-KYS-161) and informed consent was taken from all the patients.
Open Access Statement: This is an Open Access article distributed in accordance with the Creative Commons Attribution-NonCommercial-NoDerivs 4.0 International License (CC BY-NC-ND 4.0), which permits the non-commercial replication and distribution of the article with the strict proviso that no changes or edits are made and the original work is properly cited (including links to both the formal publication through the relevant DOI and the license). See: https://creativecommons.org/licenses/by-nc-nd/4.0/.
References
- Raja R, Wu C, Bassoy EY, et al. PP4 inhibition sensitizes ovarian cancer to NK cell-mediated cytotoxicity via STAT1 activation and inflammatory signaling. J Immunother Cancer 2022;10:e005026. [Crossref] [PubMed]
- Walton J, Blagih J, Ennis D, et al. CRISPR/Cas9-Mediated Trp53 and Brca2 Knockout to Generate Improved Murine Models of Ovarian High-Grade Serous Carcinoma. Cancer Res 2016;76:6118-29. [Crossref] [PubMed]
- Park J, Lee DH. Functional roles of protein phosphatase 4 in multiple aspects of cellular physiology: a friend and a foe. BMB Rep 2020;53:181-90. [Crossref] [PubMed]
- Tikhonova A, Aifantis I. The taming of the NF-κB: PP4R1 navigates while PP4c dephosphorylates. Immunity 2012;37:594-6. [Crossref] [PubMed]
- Hadweh P, Habelhah H, Kieff E, et al. The PP4R1 subunit of protein phosphatase PP4 targets TRAF2 and TRAF6 to mediate inhibition of NF-κB activation. Cell Signal 2014;26:2730-7. [Crossref] [PubMed]
- Wang B, Pan LY, Kang N, et al. PP4R1 interacts with HMGA2 to promote non-small-cell lung cancer migration and metastasis via activating MAPK/ERK-induced epithelial-mesenchymal transition. Mol Carcinog 2020;59:467-77. [Crossref] [PubMed]
- Xiang Y, Zong QB, Liu H, et al. lncRNA IGF2-AS regulates miR-500a-3p/PPP4R1/p-VEGFR2 signalling pathway to promote thyroid carcinoma progression and tubulogenesis. Clin Transl Med 2023;13:e1240. [Crossref] [PubMed]
- Reinhold WC, Sunshine M, Liu H, et al. CellMiner: a web-based suite of genomic and pharmacologic tools to explore transcript and drug patterns in the NCI-60 cell line set. Cancer Res 2012;72:3499-511. [Crossref] [PubMed]
- Liu J, Lichtenberg T, Hoadley KA, et al. An Integrated TCGA Pan-Cancer Clinical Data Resource to Drive High-Quality Survival Outcome Analytics. Cell 2018;173:400-416.e11. [Crossref] [PubMed]
- Szklarczyk D, Kirsch R, Koutrouli M, et al. The STRING database in 2023: protein-protein association networks and functional enrichment analyses for any sequenced genome of interest. Nucleic Acids Res 2023;51:D638-46. [Crossref] [PubMed]
- Yu G, Wang LG, Han Y, et al. clusterProfiler: an R package for comparing biological themes among gene clusters. OMICS 2012;16:284-7. [Crossref] [PubMed]
- Ru B, Wong CN, Tong Y, et al. TISIDB: an integrated repository portal for tumor-immune system interactions. Bioinformatics 2019;35:4200-2. [Crossref] [PubMed]
- Sticht C, De La Torre C, Parveen A, et al. miRWalk: An online resource for prediction of microRNA binding sites. PLoS One 2018;13:e0206239. [Crossref] [PubMed]
- Paraskevopoulou MD, Georgakilas G, Kostoulas N, et al. DIANA-microT web server v5.0: service integration into miRNA functional analysis workflows. Nucleic Acids Res 2013;41:W169-73. [Crossref] [PubMed]
- Li JH, Liu S, Zhou H, et al. starBase v2.0: decoding miRNA-ceRNA, miRNA-ncRNA and protein-RNA interaction networks from large-scale CLIP-Seq data. Nucleic Acids Res 2014;42:D92-7. [Crossref] [PubMed]
- Karagkouni D, Paraskevopoulou MD, Tastsoglou S, et al. DIANA-LncBase v3: indexing experimentally supported miRNA targets on non-coding transcripts. Nucleic Acids Res 2020;48:D101-10. [PubMed]
- Yuan X, Bu H, Zhou J, et al. Recent Advances of SHP2 Inhibitors in Cancer Therapy: Current Development and Clinical Application. J Med Chem 2020;63:11368-96. [Crossref] [PubMed]
- Zheng Y, Zhu M, Li M. Effects of alpha-fetoprotein on the occurrence and progression of hepatocellular carcinoma. J Cancer Res Clin Oncol 2020;146:2439-46. [Crossref] [PubMed]
- Zhang M, Liu K, Zhang Q, et al. Alpha fetoprotein promotes polarization of macrophages towards M2-like phenotype and inhibits macrophages to phagocytize hepatoma cells. Front Immunol 2023;14:1081572. [Crossref] [PubMed]
- Paskeh MDA, Ghadyani F, Hashemi M, et al. Biological impact and therapeutic perspective of targeting PI3K/Akt signaling in hepatocellular carcinoma: Promises and Challenges. Pharmacol Res 2023;187:106553. [Crossref] [PubMed]
- He S, Tang S. WNT/β-catenin signaling in the development of liver cancers. Biomed Pharmacother 2020;132:110851. [Crossref] [PubMed]
- Cui J, Shen HM, Lim LHK. The Role of Autophagy in Liver Cancer: Crosstalk in Signaling Pathways and Potential Therapeutic Targets. Pharmaceuticals (Basel) 2020;13:432. [Crossref] [PubMed]
- Shi H, Zou Y, Zhong W, et al. Complex roles of Hippo-YAP/TAZ signaling in hepatocellular carcinoma. J Cancer Res Clin Oncol 2023;149:15311-22. [Crossref] [PubMed]
- Palamarchuk A, Tsyba L, Tomasello L, et al. PDCD1 (PD-1) is a direct target of miR-15a-5p and miR-16-5p. Signal Transduct Target Ther 2022;7:12. [Crossref] [PubMed]
- Suresh S, Chen B, Zhu J, et al. eIF5B drives integrated stress response-dependent translation of PD-L1 in lung cancer. Nat Cancer 2020;1:533-45. [Crossref] [PubMed]
- Tan S, Xu Y, Wang Z, et al. Tim-3 Hampers Tumor Surveillance of Liver-Resident and Conventional NK Cells by Disrupting PI3K Signaling. Cancer Res 2020;80:1130-42. [Crossref] [PubMed]
- Russell BL, Sooklal SA, Malindisa ST, et al. The Tumor Microenvironment Factors That Promote Resistance to Immune Checkpoint Blockade Therapy. Front Oncol 2021;11:641428. [Crossref] [PubMed]
- Hamad RS, Al Abdulsalam NK, Elrefaiy MA, et al. Interplay between TGF-b1 and miRNA-122 biomarkers in hepatocellular carcinoma progression in patients with chronic hepatitis C. Trop Biomed 2022;39:559-68. [Crossref] [PubMed]
- Meng G, Fei Z, Fang M, et al. Fludarabine as an Adjuvant Improves Newcastle Disease Virus-Mediated Antitumor Immunity in Hepatocellular Carcinoma. Mol Ther Oncolytics 2019;13:22-34. [Crossref] [PubMed]
- Song M, Ma L, Shen C, et al. FGD5-AS1/miR-5590-3p/PINK1 induces Lenvatinib resistance in hepatocellular carcinoma. Cell Signal 2023;111:110828. [Crossref] [PubMed]