A centrosome-related gene signature for predicting the overall survival of uveal melanoma
Highlight box
Key findings
• This study first investigated the role of centrosome-related genes in uveal melanoma (UVM) and constructed a centrosome-related gene signature for the prediction of patient prognosis in UVM.
What is known and what is new?
• Centrosome aberration (CA) can initiate tumorigenesis and metastasis in many cancers, including UVM.
• This study first investigated the key centrosome-related genes in UVM prognosis and constructed a centrosome-related gene signature for UVM.
What is the implication, and what should change now?
• This study can help to provide new insights into the role of CA in UVM progression and identify novel centrosome-related biomarkers for UVM.
Introduction
Uveal melanoma (UVM) is a common intraocular malignancy despite its low incidence (1). Although approximately 90% primary UVM could be controlled by radiotherapy, enucleation, or other modalities, distant metastases would ultimately occur in more than 40% of patients (2). The low tumor burden and unique molecular patterns of metastatic UVM have been shown to result in a low responsiveness to immune checkpoint therapy (3). The median overall survival (OS) of metastatic UVM is 10–13 months because of the limited therapies (2,4,5). Tebentafusp is the only validated effective therapy in the management of metastatic UVM currently, yet only patients with the HLA-A*02:01 allele respond generally to the therapy (6). Moreover, the frequency of the HLA-A*02:01 allele is approximately 30% in European and North American patients, 20–25% in Black and South Asians, and lower in South Asians (7). Hence there is an urgent need to explore the molecular mechanism underlying the development of UVM and identify novel predictors to improve the management of UVM.
The centrosome is an organelle normally localized around the nuclei, which consists of a pair of centrioles surrounded by a pericentriolar matrix (8). Centrosome homeostasis is an essential factor for regulating the cell cycle. Centrosome aberration (CA) is a common feature of human tumors which can initiate tumorigenesis and metastasis under pathophysiological conditions (9). For example, SKA1 overexpression can induce human prostate epithelial cells to undergo centrosome amplification to cause the formation of tumors in nude mice (10). Another typical example is that brain cells with centrosome amplification can form tumors in normal recipient flies (11). Targeting centrosome amplification has emerged as a promising specific anti-cancer treatment (12). More recently, CA has been reported for the first time in UVM. Sabat-Pośpiech et al. confirmed that CA existed in UVM primary tumors and cell lines (13). Moreover, a study showed that chromosome monosomy 3 (M3) and BAP1 mutant UVM had a higher level of CA, which was related to worse prognosis (13); however, this is the only study available on the role of CA in UVM and the mechanism needs further detailed study.
The role of CA in cancer is complex, involving various biological processes (BP) and signaling pathways. Transcription profile and bioinformatics tools have greatly improved the understanding of UVM molecular patterns (14,15). Our study aimed to explore the key genes and potential mechanisms related to CA in UVM by integrated bioinformatics on data from public datasets. We present this article in accordance with the TRIPOD reporting checklist (available at https://tcr.amegroups.com/article/view/10.21037/tcr-23-1486/rc).
Methods
Data source
The study was conducted in accordance with the Declaration of Helsinki (as revised in 2013). We downloaded messenger ribonucleic acid expression data and clinical information of UVM patients from The Cancer Genome Atlas (TCGA)-UVM containing 80 samples and Gene Expression Omnibus series (GSE) 22138 datasets containing 63 samples (15,16). A total of 726 centrosome-related genes were derived from MiCroKiTS (http://microkit.biocuckoo.org) (17).
Construction and evaluation of CA-related gene signature
Univariate Cox regression analysis was carried out to screen the survival-related genes. Subsequently, least absolute shrinkage and selection operator (LASSO) regression and multivariate Cox regression were conducted to construct a centrosome-related gene signature:
The Survminer package was used to determine optimal cut-off to divide patients into two groups. Kaplan-Meier (KM) survival curves were used to evaluate the survival differences between the two groups.
Differentially expressed genes and enrichment analyses
To avoid excessive false positive and false negative results, up-regulated differentially expressed genes (DEGs) between 2 groups were identified with the criteria of P<0.05 and fold change (FC) >3/2, down-regulated DEGs between two groups were identified with the criteria of P<0.05 and FC <2/3 according to the previous criteria (18-21). The ClusterProfiler package was used to conduct the Kyoto Encyclopedia of Genes and Genomes (KEGG) and Gene Ontology (GO) enrichment analyses.
Immune landscape and mutation analysis
The infiltration of the immune cells was evaluated by Cell-type Identification by Estimating Relative Subsets of RNA Transcripts (CIBERSORT) platform. The stromal score, immune score, and estimate score were evaluated by the Estimation of Stromal and Immune cells in Malignant Tumor tissues using Expression data (ESTIMATE) algorithm. Mutation analysis was conducted by the maftools R package and P value was annotated after the gene.
Statistical analysis
Statistical analysis was performed using R (version 4.0.0; The R Foundation for Statistical Computing, Vienna, Austria) and associated packages. The Survminer package was used to determine the high- and low-risk group. Gene enrichment analyses were conducted by the ClusterProfiler package. The CIBERSORT platform and ESTIMATE algorithm were used to visualize the immune landscape. Maftools R package was used to perform mutation analysis. A significant difference was indicated when P<0.05.
Results
Construction of centrosome-related gene signature
A total of 204 genes were screened out as associated with OS by univariate Cox regression analysis (P<0.05). Subsequently, LASSO regression selected 16 genes under the lambda (1,000 iterations), including MYH14, VIM, ARPC1B, PRKCD, NAXE, THPO, MCM2, CTCFL, CCND3, KIF14, CDKN2C, PNP, IGFBP4, CCDC40, MAP6, RAB6C (Figure 1). Finally, 6 genes were screened out by multivariate Cox regression to construct the signature: Risk score =−3.27071 × MAP6 −5 .03735 × CCDC40 − 2.68459 × PRKCD + 1.826349 × IGFBP4 + 11.66582 × RAB6C − 4.86899 × CCND3.
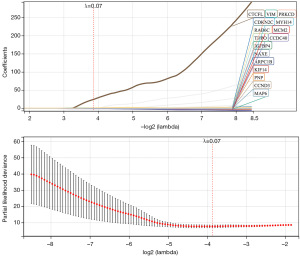
Risk score as an indicator of UVM prognosis
Patients in the TCGA-UVM cohort (80 samples) and GSE22138 cohort (63 samples) were divided into two groups by Survminer package. In the TCGA-UVM cohort, the high-risk group included 24 samples and the low-risk group included 56 samples (Figure 2A). The high-risk group had worse survival compared to that of the low-risk group, with a hazard ratio (HR) of 4,942,441,957.67 (Figure 2B). In the GSE22138 cohort, the high-risk group included 23 samples and the low-risk group included 40 samples (Figure 3A). The KM curve of GSE22138 was consistent with the TCGA-UVM cohort and the HR was 2.42 (Figure 3B).
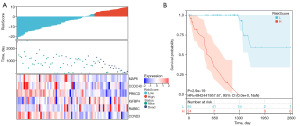
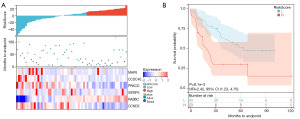
Identification of DEGs and functional enrichment associated with centrosome-related gene signature
To elucidate the relationship between gene expression profiles and CA, 1,385 up-regulated genes and 766 down-regulated genes were identified by KEGG and GO enrichment using ClusterProfiler package in the TCGA-UVM cohort (Figure 4). For KEGG, DEGs were mainly enriched in human papillomavirus infection, Epstein-Barr virus infection, and phagosome (Figure 5A). For BP, DEGs were mainly enriched in leukocyte migration, T cell activation, and regulation of leukocyte activation (Figure 5B). For cellular component (CC), DEGs were mainly enriched in extracellular matrix (ECM), collagen-containing ECM, and plasma membrane protein complex (Figure 5C). For molecular function (MF), DEGs were mainly enriched in cell adhesion molecule binding, actin binding, and amide binding (Figure 5D).
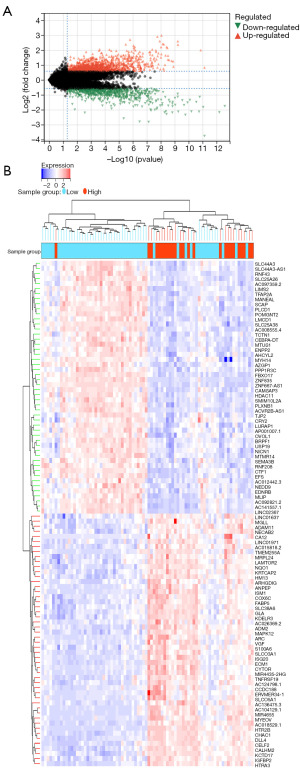
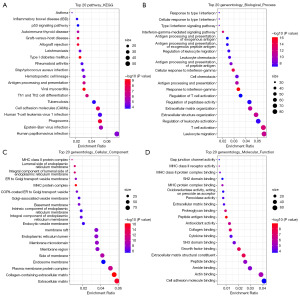
Comparison of immune infiltration
The results of CIBERSORT showed that CD4 memory resting T cells, CD4 memory activated T cells, and monocytes were highly infiltrated in the high-risk group and CD8 T cells, M0 macrophages, and M1 macrophages were highly infiltrated in the low-risk group (Figure 6A). The ESTIMATE analysis showed that the high-risk group had higher immune score and estimate score (Figure 6B). Furthermore, programmed cell death 1 (PD-1) and cytotoxic t-lymphocyte-associated protein 4 (CTLA-4) were highly expressed in the high-risk group (Figure 6C).

Assessment of mutation frequency and patterns
The top 15 frequent gene mutation frequencies and patterns were identified by the Maftools R package and are shown in Figure 7. We identified two mutation sites as significantly different between the two groups: BAP1 was associated with high risk and SF3B1 was associated with low risk. Moreover, EIF1AX only existed in the low-risk group, though the P value was 0.06.
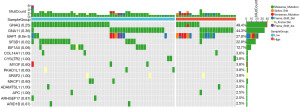
Discussion
CA is a biomarker of solid malignancies that is related to the aberrant tumor karyotypes and poor survival (22). As the most common intraocular solid malignancy, UVM is a complex heterogeneous tumor. Therefore, the study of CA in UVM may help to improve the understanding of UVM molecular characteristics. Some current advances have been made in a recent study that focused on the role of CA in UVM (13). Sabat-Pośpiech et al. found that primary UVM with M3 had higher levels of CA and M3 is one of the most important predictors of poor prognosis in UVM by many studies (3,13,15,23,24). Moreover, MP46, an UVM cell line with BAP1 mutation, showed the highest level of CA in the primary UVM cell lines and BAP1 mutation has been identified as another important predictor of poor prognosis in UVM (13,15,25). Furthermore, high levels of CA in the aggressive subtypes present a high degree of centrosome clustering to promote UVM mitosis, suggesting the potential of targeting CA in UVM therapy (13). Therefore, utilizing bioinformatics and ribonucleic acid sequencing data will provide novel insights for the role of CA in UVM (26,27).
The present study first constructed a centrosome-related gene signature and identified 6 prognostic genes: RAB6C and IGFBP4 related to high-risk, MAP6, CCDC40, CCND3, and PRKCD related to low-risk. RAB6C is involved in mitotic cell cycle and regulates centrosome duplication under normal circumstances. RAB6C has been shown to have a promoting effect on the malignant behavior of bladder cancer cells (28). However, overexpression of Rab6C results in G1 arrest and greatly hinders the proliferation of cells, which seems to be harmful to cancer development. Interestingly, the phenomenon of G1 arrest was observed in BAP1 deficient or BAP1 knockdown UVM cells (29,30). Moreover, the UVM cell lines with BAP1 mutation require far more time for doubling and more selective culture conditions. In our signature, BAP1 mutation was more frequent in the high-risk group. Therefore, we inferred that the high-risk effect of RAB6C in UVM may be related to BAP1 mutation. IGFBP4 plays an oncogenic role in glioblastoma and renal cell carcinoma (31-33). The oncogenic role of IGFBP4 is induced by the Wnt/beta-catenin pathway in renal cell carcinoma (32). Another study showed that IGFBP4 promoted the epithelial-mesenchymal transition and invasion of glioblastoma (31). MAP6, also known as STOP, encodes a microtubule-associated protein that is crucial to the assembly of spindles during cell division and its low expression may cause abnormal cell division to induce cancer development (34). A higher level of MAP6 expression has been observed in normal tissues compared with tumors and served as a biomarker for better prognosis in lung cancer (34). Moreover, a study found that MAP6 was aberrantly methylated in some oral squamous cell carcinoma cell lines, suggesting its tumor suppressor role (35). CCDC40 encodes a protein affecting motile cilia that participates in cell division; only 1 study has explored the role of CCDC40 in cancer. Ma et al. reported that CCDC40 plays a tumor suppressor role through regulating the deubiquitination process to promote the degradation of EGFR (36). Our study also supported that CCDC40 serves as a tumor suppressor, but the effect of CCDC40 in UVM remained unexplored. CCND3 is a member of cyclin family and is essential for cell cycle G1/S transition. CCND3 is related to poor prognosis in most cancers because of its promotion on the cell cycle. However, UVM is slow growing compared to other cancers and the molecular mechanism inducing cancerous transformation of normal uveal melanocytes is unique; there is even a reverse trend in the expression of many cell cycle-related proteins compared with most cancers (37-41). The prognostic value of CCND3 in UVM is quite different from other cancers and that may be attributed to the unique UVM cell cycle regulation.
Interestingly, our signature identified PRKCD as a low-risk-related gene, whereas some previous studies had supported that PRKCD was a potential therapeutic target in UVM (42-44). PRKCD is a member of Protein kinase C, playing various cellular functions, including proliferation, migration, apoptosis, and autography. Berardi et al. confirmed that PRKCD could decrease the proliferative capacity of breast cancer (45). Furthermore, PRKCD also has been known as a tumor suppressor due to its importance in apoptotic pathways (46). Interestingly, some studies have asserted that PRKCD served as an oncogene, including a UVM study (47); however, that study used UVM cell lines with SF3B1 or EIF1AX mutation without BAP1 mutation and SF3B1 or EIF1AX was related to low risk. Furthermore, although great efforts have been devoted to PKC inhibitors therapy in UVM, limited effects have been achieved to date, only 3% and 9% of metastatic UVM cases achieved a partial response in 2 PKC inhibitor clinical trials, respectively (42-44,48). More importantly, most available studies supporting PKC inhibitors as a promising treatment for UVM have been based on cell lines without BAP1 mutation. A recent study showed that PKC inhibitors were not sufficient to induce robust cell death and only have limited inhibitive effects because UVM cells, particularly MP38, a BAP1 mutation cell line, showed strong regulative capacity and resistance to the inhibitor (49). The BAP1 mutation UVM may have less dependence on the PKC-MAPK pathway compared with SF3B1 or EIF1AX mutation and have a lower expression of PRKCD because of its better regulative capacity. The study indicated that PRKCD was related to low-risk and the reasons may be various. It may be attributed to the fact that UVM with SF3B1 or EIF1AX mutation is more reliant on PRKCD expression to activate the MAPK pathway to develop and UVM with BAP1 mutation is relatively less reliant, so high expression of PRKCD suggests an SF3B1 or EIF1AX mutation, which are related to low-risk. Another hypothesis is that PRKCD not only acts as a mediator of MAPK activation, but also has some tumor suppressive functions, including promoting apoptosis via tyrosine phosphorylation or decreasing proliferative capacity via increasing autophagy flux and cell cycle arrest, as previously reported (45,50). The inhibition of PRKCD not only suppresses its oncogenic role, but also limits its tumor suppressive functions. Considering that limited benefit had been achieved by PKC inhibitors and the role of PRKCD had mainly been explored in SF3B1 or EIF1AX mutation UVM previously, it is necessary to evaluate the role of PRKCD in BAP1 mutation UVM.
We assessed the DEGs between 2 groups and found that DEGs were mainly implicated in cancer progression, such as ECM and cell adhesion molecule binding. These pathways are closely concerned with tumor migration, invasion, and spread (51). Moreover, DEGs were also implicated in inflammation, including virus infection, leukocyte migration, and T cell activation. In fact, inflammation is also a hallmark of disease progression and poor prognosis in UVM (52,53). Furthermore, according to immune infiltration analysis, the high-risk group had higher immune score and more immune infiltration. Unexpectedly, CD8+ T cells were more infiltrated in the low-risk group and CD8+ T cells usually suggest a poor outcome. However, the high-risk group showed a higher level of immune resistance, including higher abundance of CD4+ memory resting T cells, higher expression of PD-1 and CTLA-4. Thus, the immune resistance might limit the CD8+ T cells infiltration in the high-risk group.
Conclusions
In summary, our research first studied the association of centrosome-related genes with UVM OS. Moreover, we constructed a centrosome-related gene signature for UVM. The signature provides new insights into the role of CA in UVM progression and identifies novel centrosome-related biomarkers for UVM.
Acknowledgments
Funding: This work was supported by
Footnote
Reporting Checklist: The authors have completed the TRIPOD reporting checklist. Available at https://tcr.amegroups.com/article/view/10.21037/tcr-23-1486/rc
Peer Review File: Available at https://tcr.amegroups.com/article/view/10.21037/tcr-23-1486/prf
Conflicts of Interest: All authors have completed the ICMJE uniform disclosure form (available at https://tcr.amegroups.com/article/view/10.21037/tcr-23-1486/coif). The authors have no conflicts of interest to declare.
Ethical Statement: The authors are accountable for all aspects of the work in ensuring that questions related to the accuracy or integrity of any part of the work are appropriately investigated and resolved. The study was conducted in accordance with the Declaration of Helsinki (as revised in 2013).
Open Access Statement: This is an Open Access article distributed in accordance with the Creative Commons Attribution-NonCommercial-NoDerivs 4.0 International License (CC BY-NC-ND 4.0), which permits the non-commercial replication and distribution of the article with the strict proviso that no changes or edits are made and the original work is properly cited (including links to both the formal publication through the relevant DOI and the license). See: https://creativecommons.org/licenses/by-nc-nd/4.0/.
References
- Jager MJ, Shields CL, Cebulla CM, et al. Uveal melanoma. Nat Rev Dis Primers 2020;6:24. [Crossref] [PubMed]
- Carvajal RD, Sacco JJ, Jager MJ, et al. Advances in the clinical management of uveal melanoma. Nat Rev Clin Oncol 2023;20:99-115. [Crossref] [PubMed]
- Smit KN, Jager MJ, de Klein A, et al. Uveal melanoma: Towards a molecular understanding. Prog Retin Eye Res 2020;75:100800. [Crossref] [PubMed]
- Lane AM, Kim IK, Gragoudas ES. Survival Rates in Patients After Treatment for Metastasis From Uveal Melanoma. JAMA Ophthalmol 2018;136:981-6. [Crossref] [PubMed]
- Seedor RS, Eschelman DJ, Gonsalves CF, et al. An Outcome Assessment of a Single Institution's Longitudinal Experience with Uveal Melanoma Patients with Liver Metastasis. Cancers (Basel) 2020;12:117. [Crossref] [PubMed]
- Nathan P, Hassel JC, Rutkowski P, et al. Overall Survival Benefit with Tebentafusp in Metastatic Uveal Melanoma. N Engl J Med 2021;385:1196-206. [Crossref] [PubMed]
- Howlett S, Carter TJ, Shaw HM, et al. Tebentafusp: a first-in-class treatment for metastatic uveal melanoma. Ther Adv Med Oncol 2023;15:17588359231160140. [Crossref] [PubMed]
- Loncarek J, Bettencourt-Dias M. Building the right centriole for each cell type. J Cell Biol 2018;217:823-35. [Crossref] [PubMed]
- Wu Q, Yu X, Liu L, et al. Centrosome-phagy: implications for human diseases. Cell Biosci 2021;11:49. [Crossref] [PubMed]
- Li J, Xuan JW, Khatamianfar V, et al. SKA1 over-expression promotes centriole over-duplication, centrosome amplification and prostate tumourigenesis. J Pathol 2014;234:178-89. [Crossref] [PubMed]
- Basto R, Brunk K, Vinadogrova T, et al. Centrosome amplification can initiate tumorigenesis in flies. Cell 2008;133:1032-42. [Crossref] [PubMed]
- Godinho SA, Pellman D. Causes and consequences of centrosome abnormalities in cancer. Philos Trans R Soc Lond B Biol Sci 2014;369:20130467. [Crossref] [PubMed]
- Sabat-Pośpiech D, Fabian-Kolpanowicz K, Kalirai H, et al. Aggressive uveal melanoma displays a high degree of centrosome amplification, opening the door to therapeutic intervention. J Pathol Clin Res 2022;8:383-94. [Crossref] [PubMed]
- Jager MJ, Brouwer NJ, Esmaeli B. The Cancer Genome Atlas Project: An Integrated Molecular View of Uveal Melanoma. Ophthalmology 2018;125:1139-42. [Crossref] [PubMed]
- Robertson AG, Shih J, Yau C, et al. Integrative Analysis Identifies Four Molecular and Clinical Subsets in Uveal Melanoma. Cancer Cell 2017;32:204-220.e15. [Crossref] [PubMed]
- Laurent C, Valet F, Planque N, et al. High PTP4A3 phosphatase expression correlates with metastatic risk in uveal melanoma patients. Cancer Res 2011;71:666-74. [Crossref] [PubMed]
- Huang Z, Ma L, Wang Y, et al. MiCroKiTS 4.0: a database of midbody, centrosome, kinetochore, telomere and spindle. Nucleic Acids Res 2015;43:D328-34. [Crossref] [PubMed]
- Feng C, Xu Y, Liu Y, et al. Gene Expression Subtyping Reveals Immune alterations:TCGA Database for Prognosis in Ovarian Serous Cystadenocarcinoma. Front Mol Biosci 2021;8:619027. [Crossref] [PubMed]
- Zhang J, Huang X, Wang X, et al. Identification of potential crucial genes in atrial fibrillation: a bioinformatic analysis. BMC Med Genomics 2020;13:104. [Crossref] [PubMed]
- Yu X, Li W, Feng Y, et al. The prognostic value of hedgehog signaling in bladder cancer by integrated bioinformatics. Sci Rep 2023;13:6241. [Crossref] [PubMed]
- Li W, Guo X, Chen C, et al. The prognostic value of arachidonic acid metabolism in breast cancer by integrated bioinformatics. Lipids Health Dis 2022;21:103. [Crossref] [PubMed]
- Mittal K, Kaur J, Jaczko M, et al. Centrosome amplification: a quantifiable cancer cell trait with prognostic value in solid malignancies. Cancer Metastasis Rev 2021;40:319-39. [Crossref] [PubMed]
- Prescher G, Bornfeld N, Hirche H, et al. Prognostic implications of monosomy 3 in uveal melanoma. Lancet 1996;347:1222-5. [Crossref] [PubMed]
- Damato B, Eleuteri A, Taktak AF, et al. Estimating prognosis for survival after treatment of choroidal melanoma. Prog Retin Eye Res 2011;30:285-95. [Crossref] [PubMed]
- Kalirai H, Dodson A, Faqir S, et al. Lack of BAP1 protein expression in uveal melanoma is associated with increased metastatic risk and has utility in routine prognostic testing. Br J Cancer 2014;111:1373-80. [Crossref] [PubMed]
- Guo X, Yu X, Li F, et al. Identification of Survival-Related Metabolic Genes and a Novel Gene Signature Predicting the Overall Survival for Patients with Uveal Melanoma. Ophthalmic Res 2022;65:516-28. [Crossref] [PubMed]
- Guo X, Yu X, Zhang Y, et al. A Novel Glycolysis-Related Signature for Predicting the Prognosis and Immune Infiltration of Uveal Melanoma. Ophthalmic Res 2023;66:692-705. [Crossref] [PubMed]
- He L, Pan X, Wang X, et al. Rab6c is a new target of miR 218 that can promote the progression of bladder cancer. Mol Med Rep 2021;24:792. [Crossref] [PubMed]
- Pan H, Jia R, Zhang L, et al. BAP1 regulates cell cycle progression through E2F1 target genes and mediates transcriptional silencing via H2A monoubiquitination in uveal melanoma cells. Int J Biochem Cell Biol 2015;60:176-84. [Crossref] [PubMed]
- Yu L, Zhou D, Zhang G, et al. Co-occurrence of BAP1 and SF3B1 mutations in uveal melanoma induces cellular senescence. Mol Oncol 2022;16:607-29. [Crossref] [PubMed]
- Praveen Kumar VR, Sehgal P, Thota B, et al. Insulin like growth factor binding protein 4 promotes GBM progression and regulates key factors involved in EMT and invasion. J Neurooncol 2014;116:455-64. [Crossref] [PubMed]
- Ueno K, Hirata H, Majid S, et al. IGFBP-4 activates the Wnt/beta-catenin signaling pathway and induces M-CAM expression in human renal cell carcinoma. Int J Cancer 2011;129:2360-9. [Crossref] [PubMed]
- Zhong Z, Xu X, Han S, et al. Comprehensive Analysis of Prognostic Value and Immune Infiltration of IGFBP Family Members in Glioblastoma. J Healthc Eng 2022;2022:2929695. [Crossref] [PubMed]
- Luo J, Hu Q, Gou M, et al. Expression of Microtubule-Associated Proteins in Relation to Prognosis and Efficacy of Immunotherapy in Non-Small Cell Lung Cancer. Front Oncol 2021;11:680402. [Crossref] [PubMed]
- Abe M, Yamashita S, Mori Y, et al. High-risk oral leukoplakia is associated with aberrant promoter methylation of multiple genes. BMC Cancer 2016;16:350. [Crossref] [PubMed]
- Ma J, Ma S, Zhang Y, et al. Kinectin1 depletion promotes EGFR degradation via the ubiquitin-proteosome system in cutaneous squamous cell carcinoma. Cell Death Dis 2021;12:995. [Crossref] [PubMed]
- LaFave LM, Béguelin W, Koche R, et al. Reply to "Uveal melanoma cells are resistant to EZH2 inhibition regardless of BAP1 status". Nat Med 2016;22:578-9. [Crossref] [PubMed]
- Amirouchene-Angelozzi N, Nemati F, Gentien D, et al. Establishment of novel cell lines recapitulating the genetic landscape of uveal melanoma and preclinical validation of mTOR as a therapeutic target. Mol Oncol 2014;8:1508-20. [Crossref] [PubMed]
- Jager MJ, Magner JA, Ksander BR, et al. Uveal Melanoma Cell Lines: Where do they come from? (An American Ophthalmological Society Thesis). Trans Am Ophthalmol Soc 2016;114:T5.
- Cree IA. Cell cycle and melanoma--two different tumours from the same cell type. J Pathol 2000;191:112-4. [Crossref] [PubMed]
- Pardo M, Piñeiro A, de la Fuente M, et al. Abnormal cell cycle regulation in primary human uveal melanoma cultures. J Cell Biochem 2004;93:708-20. [Crossref] [PubMed]
- Piperno-Neumann S, Larkin J, Carvajal RD, et al. Genomic Profiling of Metastatic Uveal Melanoma and Clinical Results of a Phase I Study of the Protein Kinase C Inhibitor AEB071. Mol Cancer Ther 2020;19:1031-9. [Crossref] [PubMed]
- Shoushtari AN, Khan S, Komatsubara K, et al. A Phase Ib Study of Sotrastaurin, a PKC Inhibitor, and Alpelisib, a PI3Kα Inhibitor, in Patients with Metastatic Uveal Melanoma. Cancers (Basel) 2021;13:5504. [Crossref] [PubMed]
- Kapiteijn E, Carlino M, Boni V, et al. Abstract CT068: a phase I trial of LXS196, a novel PKC inhibitor for metastatic uveal melanoma. Cancer Res 2019;79:CT068. [Crossref] [PubMed]
- Berardi DE, Flumian C, Rodriguez CE, et al. PKCδ Inhibition Impairs Mammary Cancer Proliferative Capacity But Selects Cancer Stem Cells, Involving Autophagy. J Cell Biochem 2016;117:730-40. [Crossref] [PubMed]
- Dashzeveg N, Yoshida K. Crosstalk between tumor suppressors p53 and PKCδ: Execution of the intrinsic apoptotic pathways. Cancer Lett 2016;377:158-63. [Crossref] [PubMed]
- Chen X, Wu Q, Depeille P, et al. RasGRP3 Mediates MAPK Pathway Activation in GNAQ Mutant Uveal Melanoma. Cancer Cell 2017;31:685-696.e6. [Crossref] [PubMed]
- Croce M, Ferrini S, Pfeffer U, et al. Targeted Therapy of Uveal Melanoma: Recent Failures and New Perspectives. Cancers (Basel) 2019;11:846. [Crossref] [PubMed]
- Park JJ, Stewart A, Irvine M, et al. Protein kinase inhibitor responses in uveal melanoma reflects a diminished dependency on PKC-MAPK signaling. Cancer Gene Ther 2022;29:1384-93. [Crossref] [PubMed]
- Baek JH, Yun HS, Kwon GT, et al. PLOD3 suppression exerts an anti-tumor effect on human lung cancer cells by modulating the PKC-delta signaling pathway. Cell Death Dis 2019;10:156. [Crossref] [PubMed]
- Theocharis AD, Manou D, Karamanos NK. The extracellular matrix as a multitasking player in disease. FEBS J 2019;286:2830-69. [Crossref] [PubMed]
- Bronkhorst IH, Jager MJ. Uveal melanoma: the inflammatory microenvironment. J Innate Immun 2012;4:454-62. [Crossref] [PubMed]
- Bronkhorst IH, Jager MJ. Inflammation in uveal melanoma. Eye (Lond) 2013;27:217-23. [Crossref] [PubMed]