Comprehensive analysis of the effects of the cuprotosis-associated gene SLC31A1 on patient prognosis and tumor microenvironment in human cancer
Highlight box
Key findings
• Cuprotosis-associated gene solute carrier family 31 (copper transporter), member 1 (SLC31A1) affects patient prognosis and the immune microenvironment in human cancer.
What is known, and what is new?
• SLC31A1 is responsible for copper ion transport in cuprotosis and is a key molecule in the development of cuprotosis.
• SLC31A1 is involved in regulating the prognosis of human cancer and is associated with tumor immunity.
What is the implication, and what should change now?
• SLC31A1 may be a potential tumor-associated biomarker.
Introduction
Cancer is the leading cause of death worldwide and is a major public health issue (1,2). The incidence of cancer and cancer-related mortality rates are increasing rapidly worldwide due to aging and growing populations (3). Cancer is described by the histopathological, genomic, and transcriptomic heterogeneity of the tumor, and its tissue microenvironment. Cancer heterogeneity results in changes in patient outcomes (4). Histopathology biomarkers can be used to diagnose cancer; however, most histopathology biomarkers are based solely on the morphology and location of tumor cells, and a fine-grained understanding of how the spatial organization of stromal, tumor, and immune cells in the tumor microenvironment (TME) contributes to patient risk is lacking (5-7).
In studying the process of cell death carrying copper ions, Golub’s team identified a new mode of cell death involving copper ions in cells that depends on and is regulated by copper ions, called cuprotosis (8). The mechanism of copper death involves copper ions binding directly to the lipid acylated components of the tricarboxylic acid cycle, which leads to the abnormal aggregation of fatty acylated proteins and the loss of iron-sulfur cluster proteins, which in turn leads to cell death mediated by a proteotoxic stress response (9).
Interestingly, solute carrier family 31 (copper transporter), member 1 (SLC31A1) is also a cuprotosis-associated gene. The human body contains the following two copper transporter (CTR) family proteins: SLC31A1 (CTR1) and SLC31A2 (CTR2) (10). SLC31A1 is a key residue in the highly conserved C-terminal HCH190 triplet for cell membrane cystine 189 (Cys189) and copper uptake, as in Methionine 154 (Met-154) (11-13). The main role of SLC31A1 is to transport cytosolic copper (14). SLC31A1 is engaged in the cuprum (Cu) access-dependent activation of mitogen-activated protein kinase signaling (15), which is induced by growth factors, such as the fibroblast growth factor and insulin, and the activation of Cu enzymes, including lysine oxidase (16-18). SLC31A2 is predominantly located intracellularly (19) and unlike SLC31A1, the expression level of human SLC31A1 does not result in any significant changes in cellular copper metabolism (20). SLC31A1P1 has been identified as a processing gene highly homologous to SLC31A1 (21).
In this study, we investigated the regulatory function of SLC31A1 in various cancers through a series of bioinformatics online databases. We also compared the differential expression of SLC31A1 in tumor tissues and their paracancerous tissues. In addition, patient survival and methylation levels, and their role in immune regulation were also evaluated in this study. The results suggest that SLC31A1 is closely related to tumor pathogenesis and the immune response. Finally, the results were further validated by real-time quantitative polymerase chain reaction (RT-qPCR) and western blotting (WB). The objective of this study was to identify potential targets for cancer therapy by analyzing the expression prognosis and immunity of SLC31A1 in pan-cancer, building upon previous research. The findings may provide novel insights into the molecular mechanisms of cancer and facilitate personalized treatments for pan-cancer patients. The entire study flow is shown in Figure 1. We present this article in accordance with the MDAR reporting checklist (available at https://tcr.amegroups.com/article/view/10.21037/tcr-23-1308/rc).
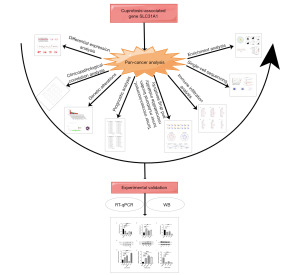
Methods
Cell culture
Cells for experiments were obtained from the Cell Resource Center of the Chinese Academy of Sciences (Shanghai, China). The cells were cultured in Roswell Park Memorial Institute-1640 (RPMI-1640), containing 10% fetal bovine serum, 100 units/mL of penicillin, and 100 µg/mL of streptomycin, and placed in a 37 °C, 5% carbon dioxide incubator.
RT-qPCR
Total RNA was extracted separately from the cells using the Trizol method, and the purified RNA was reverse-transcribed into a complementary DNA template using a reverse transcription kit, after which RT-qPCR was performed on the target genes using PCR optics, and finally the relative expression of SLC31A1 was analyzed using the 2−ΔΔCt method and normalized with reference to glyceraldehyde-3-phosphate dehydrogenase (GAPDH). The SLC31A1 primers were designed and synthesized by Bioengineering (Shanghai, China). The forward primer for SLC31A1 was: 5'-GTAAGTCACAAGTCAGCATTCG-3'; the reverse primer was: 5'-CAACAGTTTTGTGTGTCTCCAT-3'; and the GAPDH forward primer was: 5'-GGAAGCTTGTCATCAATGGAAATC-3', reverse primer 5'-TGATGACCCTTGGCTCCC-3'.
WB
The liver cancer cells, other cancer cells, and normal cells underwent protein extraction. The separation process involved the use of reduced sodium dodecyl sulfate-polyacrylamide gel electrophoresis, followed by the transfer of the separated components onto polyvinylidene fluoride membranes. Subsequently, the immunoblot technique was employed for the analysis. To detect the target proteins, rabbit-horseradish peroxidase (HRP) and mouse-HRP were employed as secondary antibodies. The gray values of the protein bands were quantitatively assessed using Image J software (*, P<0.05; **, P<0.01; ***, P<0.001; ****, P<0.0001).
Differential expression analysis
The Tumor Immune Estimation Resource 2.0 (TIMER2.0) (http://timer.cistrome.org/) database was used to analyze the differential expression of SLC31A1 in different cancer tissues and normal tissues. Additionally, the Gene Expression Profiling Interactive Analysis (GEPIA) (http://gepia.cancer-pku.cn) and GEPIA2.0 (http://gepia2.cancer-pku.cn/#index) databases were used to assess the differential expression of SLC31A1 in 33 cancers with corresponding pan-cancer tissues, and pan-cancer correlation with SLC31A1. The University of Alabama at Birmingham CANcer (UALCAN) (http://ualcan.path.uab.edu/index.html) database was used to assess the methylation levels and protein expression levels of SLC31A1 in pan-cancer based on The Cancer Genome Atlas (TCGA) database samples. The Human Protein Atlas (HPA) (https://www.proteinatlas.org) database was used to present staining visualization to reflect the protein levels of SLC31A1 in normal tissues and corresponding cancer tissues (the screening criteria were moderate or high staining intensity, and a cell count ≥25–75%). The full names and abbreviations of the pan-cancers are shown in Table 1.
Table 1
Abbreviations | Full names |
---|---|
ACC | Adrenocortical carcinoma |
BLCA | Bladder urothelial carcinoma |
BRCA | Breast invasive carcinoma |
CESC | Cervical squamous cell carcinoma and endocervical adenocarcinoma |
CHOL | Cholangiocarcinoma |
COAD | Colon adenocarcinoma |
DLBC | Lymphoid neoplasm diffuse large B-cell lymphoma |
ESCA | Esophageal carcinoma |
GBM | Glioblastoma multiforme |
HNSC | Head and neck squamous cell carcinoma |
KICH | Kidney chromophobe |
KIRC | Kidney renal clear cell carcinoma |
KIRP | Kidney renal papillary cell carcinoma |
LAML | Acute myeloid leukemia |
LGG | Lower grade glioma |
LIHC | Liver hepatocellular carcinoma |
LUAD | Lung adenocarcinoma |
LUSC | Lung squamous cell carcinoma |
MESO | Mesothelioma |
OV | Ovarian serous cystadenocarcinoma |
PAAD | Pancreatic adenocarcinoma |
PCPG | Pheochromocytoma and paraganglioma |
PRAD | Prostate adenocarcinoma |
READ | Rectum adenocarcinoma |
SARC | Sarcoma |
SKCM | Skin cutaneous melanoma |
STAD | Stomach adenocarcinoma |
TGCT | Testicular germ cell tumor |
THCA | Thyroid carcinoma |
THYM | Thymoma |
UCEC | Uterine corpus endometrial carcinoma |
UCS | Uterine carcinosarcoma |
UVM | Uveal melanoma |
TCGA, The Cancer Genome Atlas.
Clinicopathological correlation analysis
The R packages “limma” and “Nagpur” were used for the clinicopathological related studies. TCGA and the genotype-tissue expression (GTEx) RNA-sequencing data were analyzed and visualized using the R packages “pROC” and “ggplot2”. Xiantao Academic (https://www.xiantao.love/) was used to obtain the area under the receiver operating characteristic (ROC) curve (AUC) to determine diagnosis and prognosis.
Genetic alterations and prognostic analysis
We assessed the genetic alterations of the SLC31A1 gene, including missense mutations, deletions, and splicing, in different cancers using the cBioPortal (https://www.cbioportal.org/) database. Survival information data for each sample were retrieved and downloaded from the TCGA database. The OS, disease-specific survival (DSS), disease-free survival (DFS), and progression-free survival (PFS) of the cancer patients were analyzed using a Cox regression analysis. In addition, the GEPIA2.0 database was used to analyze the prognosis of SLC31A1 gene expression in different cancers, including OS and recurrence-free survival (RFS).
TME, tumor mutational burden (TMB), microsatellite instability (MSI), and drug sensitivity
The R packages “ggplot2”, “ggpubr”, and “ggExtra” were used to analyze the correlation between SLC31A1 expression and the TME (P<0.001 was set as the cut-off value). Correlations between the TMB and MSI and SLC31A1 expression were calculated using the Spearman method. The R package “fmsb” was used for image visualization. NCI-60 compound activity data and RNA-sequencing expression profiles were downloaded from CellMinerTM to analyze the drug sensitivity of SLC31A1 in pan-cancer (https://ngdc.cncb.ac.cn/databasecommons/database/id/5025). Food and drug administration (FDA)-approved drugs or drugs in clinical trials were selected for the analysis. The visualization was performed using the R packages “impute”, “limma”, “ggplot2”, and “ggpubr” (*, P<0.05; **, P<0.01; ***, P<0.001).
Immune infiltration analysis
We used the TIMER2.0 database to explore the relationship between SLC31A1 gene expression and the immune infiltrating cells.
Single-cell sequencing data analysis
The different biological functions of cancer cells in multiple cancers were analyzed at the single-cell level using the single-cell sequencing platform CancerSEA (http://biocc.hrbmu.edu.cn/CancerSEA/) database. Data on the correlation between SLC31A1 expression and different tumor functional states were downloaded from the CancerSEA database, and correlation heat maps were created using the Xiantao Academic Online website. t-distributed stochastic neighbor embedding (t-SNE) plots from the CancerSEA database were used to identify SLC31A1 expression in individual cancers.
Enrichment analysis
The SLC31A1 protein co-expression network was analyzed using the BioGRID database (https://thebiogrid.org/). The top 100 SLC31A1-related genes in pan-cancer were obtained using the GEPIA2.0 database. The association heat map between SLC31A1 and its related genes in pan-cancer was generated using the TIMER2.0 database. In addition, a Kyoto Encyclopedia of Genes and Genomes (KEGG) enrichment analysis of SLC31A1-related genes was conducted using Xiantao Academic.
Statistical analysis
The differences between SLC31A1 expression and prognosis (OS and RFS) in different cancer patients were obtained from the GEPIA 2.0 database. In addition, a t-test, Cox regression analysis, and linear regression analysis were used to compare the differences between different groups, and the data are expressed as the mean ± standard deviation. A P value <0.05 was considered statistically significant.
Results
The expression levels of SLC31A1 in pan-cancer
The expression profile of SLC31A1 was explored using the TIMER2.0, GEPIA2.0, and UALCAN platforms. First, we used the TIMER2.0 platform to evaluate the expression profile of SLC31A1 in tumor tissues and normal tissues. We found that SLC31A1 expression was up-regulated in a portion of cancers, such as bladder urothelial carcinoma (BLCA), BRCA, cervical squamous cell carcinoma and endocervical adenocarcinoma (CESC), esophageal carcinoma (ESCA), glioblastoma multiforme (GBM), head and neck squamous cell carcinoma (HNSC), pheochromocytoma and paraganglioma (PCPG), stomach adenocarcinoma (STAD), and uterine corpus endometrial carcinoma (UCEC). Conversely, SLC31A1 expression was down-regulated in another fraction of cancers, such as cholangiocarcinoma (CHOL), KIRC, kidney renal papillary cell carcinoma (KIRP), liver hepatocellular carcinoma (LIHC), lung adenocarcinoma (LUAD), lung squamous cell carcinoma (LUSC), prostate adenocarcinoma (PRAD), and thyroid carcinoma (THCA) (Figure 2A).
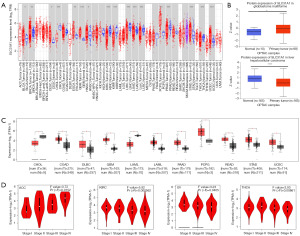
We also analyzed the protein expression of SLC31A1 using the UALCAN platform. The results showed that the SLC31A1 protein was highly expressed in GBM and lowly expressed in LIHC (Figure 2B). Since the TIMER2.0 database does not contain the paraneoplastic tissues data of several cancers, we also used GEPIA2.0 to explore the SLC31A1 expression levels between these tumors and the corresponding normal tissues. The results showed that SLC31A1 was highly expressed in colon adenocarcinoma (COAD), lymphoid neoplasm diffuse large B-cell lymphoma (DLBC), GBM, LGG, pancreatic adenocarcinoma (PAAD), PCPG, rectum adenocarcinoma (READ), STAD, and UCEC, and lowly expressed only in CHOL and acute myeloid leukemia (LAML) (Figure 2C).
Additionally, we analyzed the correlation between SLC31A1 expression and pathological stages using the GEPIA 2.0 database. The results showed that SLC31A1 expression was closely correlated with the stage of patients with ACC, KIRC, ovarian serous cystadenocarcinoma (OV), and THCA. In ACC, SLC31A1 had the highest expression in stage IV and the lowest expression in stage I. In KIRC, SLC31A1 had the highest expression in stage I and the lowest expression in stage IV. In OV and THCA, SLC31A1 had the highest expression in stage II and the lowest expression in stage IV (Figure 2D).
We then selected cancer types with differential expression of SLC31A1 in the UALCAN database and visualized their protein expression levels using the HPA database. The results showed that SLC31A1 expression was up-regulated in BRCA, STAD, and UCEC, and down-regulated in LIHC. SLC31A1 showed moderate or high staining in BRCA, STAD, and UCEC tumor tissues with ≥25–75% stained cells, while its corresponding normal tissues were moderately stained or unstained. Conversely, the LIHC tumor tissues were moderately stained or unstained, while its corresponding normal tissues were moderately or highly stained. The differential expression and protein levels differential expression of the above results were consistent (Figure 3). The differential expression results for the other cancers with opposite protein-level expression are shown in Figure S1.
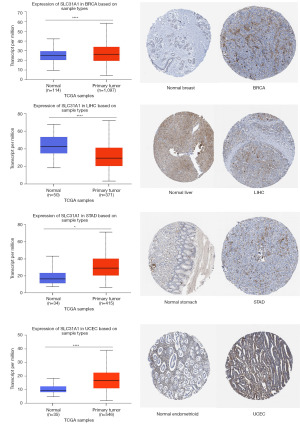
The survival analysis of SLC31A1 in cancer
The GEPIA2.0 database was used to study the prognostic value of SLC31A1 expression in cancer. We divided the patients into a high expression group and a low expression group. In KIRC, high SLC31A1 expression was associated with good OS, and in ACC, BRCA, LGG, MESO, and SKCM, low SLC31A1 expression was associated with good OS (Figure 4A). Further, we found that high expression levels of SLC31A1 were associated with good DFS in KIRC and STAD, and low expression levels of SLC31A1 were associated with good DFS in ACC, LGG and MESO (Figure 4B).
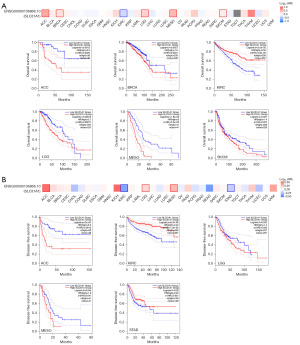
Correlation of SLC31A1 expression with clinicopathology
As Figure 5A shows, SLC31A1 was highly expressed in ACC stage III–IV patients and lowly expressed in stage I–II patients. SLC31A1 was highly expressed in testicular germ cell tumors (TGCTs) stage II–III patients and lowly expressed in stage I patients. Conversely, SLC31A1 was highly expressed in KIRC and THCA stage I–II patients and lowly expressed in stage III–IV patients. In addition, SLC31A1 expression was strongly correlated with the age of ESCA, OV, sarcoma (SARC), STAD, and UCEC patients. Of these, SLC31A1 was highly expressed in OV and UCEC patients up to and including 65 years of age, while it was highly expressed in ESCA, SARC and STAD patients over 65 years of age. Finally, we found that SLC31A1 was highly expressed in female patients with ACC, BRCA, kidney chromophobe (KICH), KIRC, and KIRP. Next, ROC curves were used to verify the diagnostic value of SLC31A1 for different cancers. As Figure 5B shows, SLC31A1 had more than moderate diagnostic accuracy (AUCs above 0.69 and even 0.8) for a variety of tumors including BRCA, CESC, and CHOL. In conclusion, the ROC curve analysis showed that SLC31A1 is a valuable diagnostic biomarker.
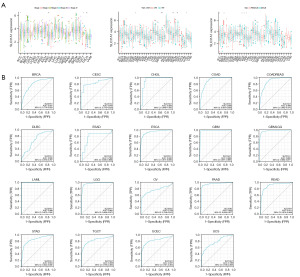
Genetic alterations of SLC31A1 in pan-cancer
We used the cBioPortal tool to study SLC31A1 gene alterations in pan-cancer. As Figure 6A shows, SLC31A1 had the highest amplification frequency in ACC, the highest mutation frequency in UCEC, the highest deep deletion frequency in THCA, and the highest multiple alteration frequency in BRCA. In addition, we explored the mutation types and mutation sites in the SLC31A1 sequence. The mutation types of SLC31A1 mainly included missense mutations, truncation mutations, splice mutations, and synaptic vesicle (SV)/fusion. In addition, missense mutations accounted for a portion of the mutation types. The truncating mutation, S105Y, may be a putative cancer driver (Figure 6B). We further derived the three-dimensional (3D) structure of S105Y (Figure 6C).
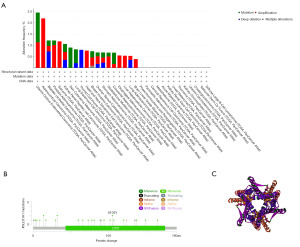
Next, we used forest plots to show the association between SLC31A1 genetic alterations and patient prognosis. SLC31A1 genetic alterations were associated with OS in patients with ACC, BLCA, BRCA, KIRC, LGG, MESO, and SKCM. Among them, SLC31A1 genetic alterations were positively correlated with OS in ACC, BLCA, BRCA, LGG, MESO, and SKCM patients, and SLC31A1 genetic alterations were negatively correlated with OS in KIRC patients (Figure 7A). SLC31A1 genetic alterations were associated with PFS in ACC, BLCA, BRCA, CESC, KIRC, LGG, MESO, READ, and uveal melanoma (UVM) patients. Among them, SLC31A1 genetic alterations were positively correlated with PFS in ACC, BLCA, BRCA, CESC, LGG, MESO, and UVM patients, and SLC31A1 genetic alterations were negatively correlated with PFS in KIRC and READ patients (Figure 7B). SLC31A1 genetic alterations were associated with DSS in ACC, KIRC, LGG, MESO, and SKCM patients. Among them, SLC31A1 genetic alterations were positively correlated with DSS in ACC, LGG, MESO, and SKCM patients, and SLC31A1 genetic alterations was negatively correlated with DSS in KIRC patients (Figure 7C). Figure 7D shows the relationship between cancer and DFS. Taken together, these results suggest that genetic alterations in SLC31A1 are closely associated with the prognosis of patients with the above-mentioned cancers.
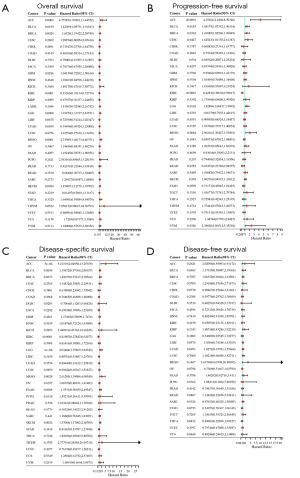
TME, TMB, MSI, and drug sensitivity
Our study showed that SLC31A1 has a prognostic role in pan-cancer; thus, we further explored the expression of TME and SLC31A1 in different tumors. The results showed that the expression of SLC31A1 was positively correlated with the scores of stromal cells and immune cells in DLBC, LAML, LUAD, MESO, and SARC (Figure 8A), which suggest that the content of stromal cells or immune cells increases as the SLC31A1 expression level increases. However, the opposite results were found in ACC and thymoma (THYM). TMB refers to the rate of the base mutation in 1 million bases. MSI refers to the phenomenon of the emergence of new microsatellite alleles at the microsatellite site of a tumor due to gene insertion or deletion compared to normal tissue. This study found a clear association between SLC31A1 and TMB in ACC, BLCA, BRCA, COAD, LGG, LUAD, OV, READ, SARC, STAD, THYM, UCEC, and uterine carcinosarcoma (UCS) (Figure 8B). This study also found a clear association between SLC31A1 and MSI in CESC, COAD, DLBC, HNSC, LUSC, PRAD, SARC, STAD, THCA, and UCEC (Figure 8C). Finally, we used the CellMinerTM database to explore the potential correlation between drug sensitivity and SLC31A1 expression. The results showed that SLC31A1 expression was negatively correlated with the drug sensitivity of denileukin diftitox, entinostat, and alectinib, but positively correlated with (+)-BET bromodomain inhibitor (JQ1) (Figure 8D). In summary, SLC31A1 may be associated with chemotherapy resistance to certain chemotherapy drugs.
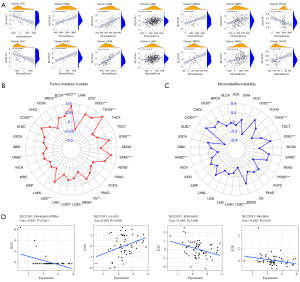
Methylation levels of SLC31A1 in pan-cancer
We explored the methylation levels of SLC31A1 in various cancers using the UALCAN database. The results showed that SLC31A1 methylation levels were highly expressed in LUSC and READ. Conversely, SLC31A1 methylation levels were lowly expressed in HNSC, KIRP, LIHC, PRAD, and UCEC (Figure 9).
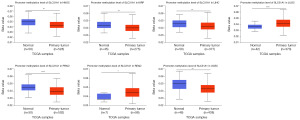
Correlation between SLC31A1 expression and the immune response
We used the TIMER2.0 database to explore the correlation between immune infiltrating cells and SLC31A1 expression using TCGA database. We found that SLC31A1 expression was positively correlated with the immune infiltration of B cells in PAAD and PCPG. Conversely, it was negatively correlated with the immune infiltration of B cells in BRCA-Basal, DLBC, MESO, and TGCT (Figure 10A). Meanwhile, SLC31A1 expression was positively correlated with the immune infiltration of cluster of differentiation (CD)4+ T cells in COAD and DLBC. Conversely, it was negatively correlated with the immune infiltration of CD4+ T cells in CHOL and GBM (Figure 10B). Further, SLC31A1 expression was positively correlated with the immune infiltration of CD8+ T cells in DLBC, LGG, PAAD, and UVM. Conversely, it was negatively correlated with the immune infiltration of CD8+ T cells in ACC and ESCA (Figure 10C). Further, SLC31A1 expression was positively correlated with the immune infiltration of natural killer (NK) cell in BLCA, COAD, DLBC, LIHC, and TGCT. Conversely, it was negatively correlated with the immune infiltration of NK cells in KIRC, KIRP, LGG, MESO, SKCM, and THCA (Figure 10D). In addition, SLC31A1 expression was positively correlated with the immune infiltration of dendritic cells (DCs) in BRCA, COAD, DLBC, HNSC-human papillomavirus (HPV)+, KIRC, KIRP, LGG, LUAD, PAAD, PRAD, SKCM, and TGCT. Conversely, it negatively correlated with the immune infiltration of DCs in ESCA and LIHC (Figure 10E). Finally, SLC31A1 expression was positively correlated with the immune infiltration of regulatory T cells (Tregs) in BLCA, CESC, ESCA, LGG, LUSC, PAAD, SKCM, TGCT, and UVM. Conversely, it was negatively correlated with the immune infiltration of Tregs cells in ACC, DLBC, KIRC, and PCPG (Figure 10F). Further, Figure S2 sets out the correlations between SLC31A1 expression and neutrophils, monocytes, macrophages, mast cells, cancer-associated fibroblasts, common lymphoid progenitor cells, endothelial cells, common myeloid progenitor cells, the immune infiltration of eosinophils, granulocyte-monocyte progenitor cells, hematopoietic stem cells, follicular helper T cells, gamma delta T cells, NK T cells, and myeloid-derived suppressor cells. The above study indicates the potential significance of SLC31A1 in the immune infiltration of the TME.
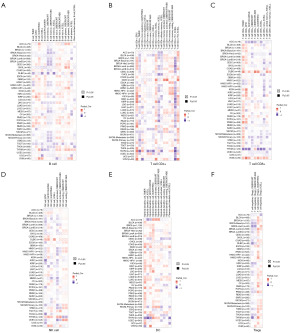
Expression of SLC31A1 at the single-cell level
The CancerSEA database was used to study the expression levels of SLC31A1 at the single-cell level to further explore the role of SLC31A1 in bio-functional states. SLC31A1 expression in UVM was negatively correlated with DNA damage, DNA repair, and apoptosis. The expression level of SLC31A1 in retinoblastoma (RB) was positively correlated with angiogenesis and differentiation. The expression of SLC31A1 in OV was positively correlated with quiescence (Figure 11A). Further, Figure 11B shows the correlations between SLC31A1 expression and DNA repair in UVM, angiogenesis in RB, and quiescence in OV. Finally, Figure 11C shows the distribution of SLC31A1 expression at the single-cell level in UVM, RB, and OV. Taken together, it appears that SLC31A1 could potentially be critical in the regulation of biological functions in cancer.
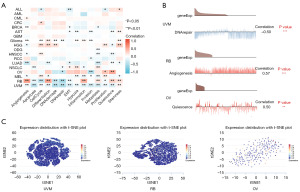
Enrichment analysis of SLC31A1-related genes
We used bioGRID to investigate the SLC31A1-interacting biomarkers (Figure 12A). Meanwhile, the top 100 genes associated with SLC31A1 were downloaded from the GEPIA 2.0 database (Table S1). The expression levels of SLC31A1 were correlated with CHGB, CYB561, DBH, EML5, PHOX2B, and TBX20 in pan-cancer (Figure 12B). Further, as the heat map in Figure 12C shows, SLC31A1 was positively correlated with the above genes in most cancers. Finally, the Gene Ontology (GO) and KEGG enrichment analysis indicated that SLC31A1-related genes were mainly enriched in the mitochondrial matrix and coated vesicles (Figure 12D).
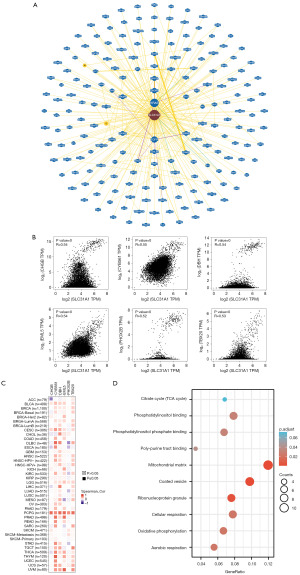
Validation of SLC31A1 expression
To verify the expression of SLC31A1 in pan-cancer, we explored it at the messenger RNA (mRNA) and protein levels in liver cancer cells (L-O2, SMMC-7721, HUh7, H-97, and HepG2), gastric cancer cells (GES-1, HGC-27, AGS, and MKN-54,) and colon cancer cells (NCM460, RKO, LoVo, and DLD-1) using both RT-qPCR and WB. We found that at the mRNA level, the liver, gastric, and colon cancer cells were lowly, highly, and lowly expressed, respectively, compared with their corresponding normal cells (Figure 13A-13C). Next, our results showed that hepatocellular carcinoma and gastric carcinoma cells were highly expressed at the protein level (except SMMC-7721) compared with their corresponding normal cells. Conversely, the colon cancer cells were lowly expressed at the protein level (Figure 13D-13F). Figure 13G-13I provides a quantitative graph of protein expression.
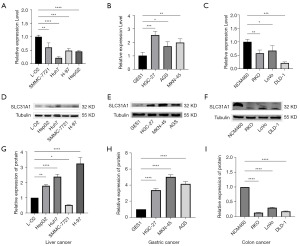
Discussion
In this study, we explored SLC31A1 expression, prognosis, mutation, methylation, and the immune response, and conducted a single-cell assessment, and an enrichment analysis in cancer using a series of bioinformatics online database approaches. Finally, RT-qPCR and WB were used to validate the differentially expressed significant hepatocellular carcinomas, gastric cancer, and colon cancer. The results showed that as a CTR, SLC31A1 has an important effect on the human body.
Copper is an essential trace element for life. All living things require copper to function properly and to maintain homeostasis; thus, maintaining the proper level of copper is crucial. Copper deficiency impairs the activity of copper-binding enzymes, and copper buildup causes cell death (22). Tsvetkov et al. recently demonstrated that copper alone, as opposed to copper ion clusters, is harmful to cells (8). Unlike other known types of death, such as apoptosis, ferroptosis, and necroptosis, cuproptosis is a completely new type of cell death (8). Instead of making adenosine triphosphate (ATP), it depends on mitochondrial respiration ATP (8). One of the key elements in maintaining intracellular copper concentration is the copper importer (SCL31A1). SCL31A1 encodes CTR1, which is essential for the uptake of high-affinity copper (23).
Our study showed that SLC31A1 is expressed in most cancers. Among them, SLC31A1 was highly expressed in BLCA, BRCA, CESC, COAD, DLBC, ESCA, GBM, LGG, HNSC, PAAD, PCPG, READ, STAD, and UCEC. Conversely, SLC31A1 was lowly expressed in CHOL, KIRC, KIRP, LAML, LIHC, LUAD, LUSC, PRAD, and THCA. Barresi et al. reported high expressions of SLC31A1 in COAD (24). Li et al. showed that SLC31A1 was highly expressed in BRCA, which validates our findings (25). Jiang et al. showed that SLC31A1 was highly expressed in HNSC epithelial cells, which also validates our findings (26). Song et al. showed that the suppression of the SLC31A1 gene, which is responsible for encoding the primary transmembrane CTR1, effectively diminishes the malignancy of PAAD through the down-regulation of intracellular copper levels. These findings align with our own research outcomes (27). In summary, SLC31A1 is expressed in most cancers.
Our study found that high SLC31A1 expression was associated with good OS in KIRC, while the opposite was true in ACC, BRCA, LGG, MESO, and SKCM. High expression levels of SLC31A1 were associated with good DFS in KIRC and STAD, but the opposite was true in ACC, LGG, and MESO. Lv et al. showed that SLC31A1 up-regulation has value in predicting the prognosis of SKCM patients. This is consistent with our findings (28). Li et al. found that SLC31A1 may be a promising diagnostic/prognostic biomarker and predictor of the drug response in breast cancer patients (25).
Further, we evaluated the genetic alterations of SLC31A1 in pan-cancer. The results showed that the amplification frequency of SLC31A1 was the highest in ACC, and its mutation types mainly included missense mutations, truncating mutations, splicing mutations, and fusion. The truncating mutation, S105Y, has the potential to be a putative cancer driver. We also presented the 3D structure of S105Y.
DNA methylation is known to be abnormal in all forms of cancer (29). Normal cells may be transformed by the onset of driver mutations and then consequently undergo de novo and demethylation processes, thereby initiating a series of programmed changes in gene expression. Alternatively, a subpopulation of normal cells that may have undergone methylation changes, possibly due to senescence, may be preferred targets for oncogenic transformation (30,31). Our study found that the methylation level of SLC31A1 was highly expressed in LUSC and READ; however, it was lowly expressed in HNSC, KIRP, LIHC, PRAD, and UCEC.
Adaptive immune responses may be triggered by innate immune cells, and research into and the development of immunotherapy will be aided by knowledge of the internal workings of cancer (32,33). According to Schalper et al. and Bremnes et al. (34,35), type I immune responses are typically associated with CD8+ T cells and T cells triggered by CD4+ type 1 T helper cells, and they are associated with a positive prognosis for lung cancer patients. According to Marshall et al. (36), type 2 T helper cells, type 17 T helper cells, and Foxp3+ Tregs are frequently linked to poor tumor growth and prognosis. Sautès-Fridman et al. (37) showed that tumor-infiltrating B lymphocytes elicit a strong and advantageous immune response in the majority of solid tumors. According to Petitprez et al. (38), the B-cell count is the best indicator of long-term survival in soft tissue sarcomata. Our study found that SLC31A1 expression was positively correlated with the immune infiltration of B cells in PAAD and PCPG. However, the immune infiltration of B cells was negatively correlated with BRCA-basal, DLBC, MESO, and TGCT. SLC31A1 expression was positively correlated with the CD4+ immune infiltration of T cells in COAD and DLBC. However, it was negatively correlated with the immune infiltration of CD4+ in T cells in CHOL and GBM. SLC31A1 expression was positively correlated with the CD8+ immune infiltration of T cells in DLBC, LGG, PAAD, and UVM. However, it was negatively correlated with the T cell CD8+ immune infiltration in ACC and ESCA. SLC31A1 expression was positively correlated with the immune infiltration of NK cells in BLCA, COAD, DLBC, LIHC, and TGCT. Conversely, it was negatively correlated with the immune infiltration of NK cells in KIRC, KIRP, LGG, MESO, SKCM, and THCA. SLC31A1 expression was positively correlated with the immune infiltration of DCs in BRCA, COAD, DLBC, HNSC-HPV+, KIRC, KIRP, LGG, LUAD, PAAD, PRAD, SKCM, and TGCT. Conversely, it was negatively correlated with the immune infiltration of DCs in ESCA and LIHC. Finally, SLC31A1 expression was positively correlated with the immune infiltration of Tregs in BLCA, CESC, ESCA, LGG, LUSC, PAAD, SKCM, TGCT, and UVM. Conversely, it was negatively correlated with the immune infiltration of Tregs cells in ACC, DLBC, KIRC, and PCPG. In summary, our study further elucidated the TME of pan-cancer.
Further, we investigated the single-cell level expression of SLC31A1 and conducted an enrichment analysis of the SLC31A1-related genes. We found that SLC31A1 plays an important role in UVM, RB, and OV, etc., which helps us explore further the role of SLC31A1 in tumors. The SLC31A1 enrichment analysis revealed that the SLC31A1-related genes were mainly enriched in the mitochondrial matrix and coated vesicles, which gives us a better understanding of its mechanism. It has been demonstrated that SLC31A1 affects intracellular (Cuprum) Cu2+ levels by acting as a copper importer. High cell membrane trace elements and a higher threshold level of mitochondrial membrane potential (ΔΨm) are required for trace element entrance into the mitochondrial matrix. Due to the use of all ATP, the increase of trace elements in the mitochondrial matrix causes a decrease in ATP levels. Ca2+ inflow into the mitochondrial matrix is mostly regulated by the (Natrium) Na+/Ca2+ exchanger (NCX) and the mitochondrial calcium monomer (MCU).
Finally, we used RT-qPCR and WB to verify the expression of SLC31A1 in the pan-cancer. The RT-qPCR results showed that the expression of SLC31A1 in liver and gastric cancers was consistent with our predicted results, but in colon cancer the expression was opposite to our predicted results. This could be caused by the heterogeneity between cells, as our prediction results came from tissue samples in a largely open database, and could also be caused by differences in tissue sample volume or tissue and cellular levels. Based on the above issues, we further validated the protein expression levels in liver, gastric, and colon cancer cells using the WB technique. Contrary to our RT-qPCR results and bioinformatics predictions, the results showed high SLC31A1 expression in liver cancer cells (except SMMC-7721), which may be due to cell-to-cell differences; thus, further validation of its expression in tissues will be necessary in future studies.
The present study observed contrasting outcomes pertaining to SLC31A1 expression at both the mRNA and protein levels in hepatocellular carcinoma cells, which might be due to the following factors: (I) given that this study serves as a fundamental validation of a bioinformatics analysis, it is plausible that incongruity exists in the expression of this gene at both the transcript and protein levels; (II) intriguingly, this discrepancy could potentially be attributed to a multitude of transcriptome or protein-level modifications, which will subsequently be explored as the next avenue of investigation in our research; (III) in light of the absence of evidence regarding the expression of the SLC31A1 gene in hepatocellular carcinoma in the previous relevant literature, we intend to delve deeper into this finding by conducting further investigations; (IV) despite the low mRNA level, the protein level adequately reflects the clinical significance of SLC31A1, and its elevated expression is strongly associated with clinical prognosis. The expression of gastric cancer cells at the protein level was in full agreement with our RT-qPCR results and bioinformatics predictions, which further improved the reliability of our study. The expression of colon cancer cells at the protein level was consistent with our RT-qPCR results, but contrary to the bioinformatics predictions. This may be because our predictions were based on tissue samples from the database, which may have some differences among the cells. Therefore, we should have further investigated the expression of SLC31A1 at the tissue level, but we lacked the conditions to validate it in tissues, which is the biggest limitation of our study.
Conclusions
In summary, this study analyzed the expression level, methylation level, gene mutation, patient survival prognosis, and immune cell infiltration of the cuprotosis-related gene SLC31A1 in pan-cancer. We also analyzed SLC31A1 at the single-cell transcriptional sequencing level and explored its different biological functions. SLC31A1 may mediate the prognosis of tumor patients by regulating tumor energy metabolism processes, coating vesicles, and affecting the immune microenvironment, and may be a potential genetic, immune, and energy-metabolic-dependent predictive target.
Acknowledgments
We would like to thank the staff at the Key Laboratory of Molecular Diagnostics and Precision Medicine for Surgical Oncology in Gansu Province, and the General Surgery, Clinical Medical Center of Gansu Provincial Hospital for their contributions.
Funding: This work was supported by grants from
Footnote
Reporting Checklist: The authors have completed the MDAR reporting checklist. Available at https://tcr.amegroups.com/article/view/10.21037/tcr-23-1308/rc
Peer Review File: Available at https://tcr.amegroups.com/article/view/10.21037/tcr-23-1308/prf
Conflicts of Interest: All authors have completed the ICMJE uniform disclosure form (available at https://tcr.amegroups.com/article/view/10.21037/tcr-23-1308/coif). The authors have no conflicts of interest to declare.
Ethical Statement: The authors are accountable for all aspects of the work in ensuring that questions related to the accuracy or integrity of any part of the work are appropriately investigated and resolved.
Open Access Statement: This is an Open Access article distributed in accordance with the Creative Commons Attribution-NonCommercial-NoDerivs 4.0 International License (CC BY-NC-ND 4.0), which permits the non-commercial replication and distribution of the article with the strict proviso that no changes or edits are made and the original work is properly cited (including links to both the formal publication through the relevant DOI and the license). See: https://creativecommons.org/licenses/by-nc-nd/4.0/.
References
- The global burden of adolescent and young adult cancer in 2019: a systematic analysis for the Global Burden of Disease Study 2019. Lancet Oncol 2022;23:27-52. [Crossref] [PubMed]
- Sung H, Ferlay J, Siegel RL, et al. Global Cancer Statistics 2020: GLOBOCAN Estimates of Incidence and Mortality Worldwide for 36 Cancers in 185 Countries. CA Cancer J Clin 2021;71:209-49. [Crossref] [PubMed]
- Gersten O, Barbieri M. Evaluation of the Cancer Transition Theory in the US, Select European Nations, and Japan by Investigating Mortality of Infectious- and Noninfectious-Related Cancers, 1950-2018. JAMA Netw Open 2021;4:e215322. [Crossref] [PubMed]
- Marusyk A, Almendro V, Polyak K. Intra-tumour heterogeneity: a looking glass for cancer? Nat Rev Cancer 2012;12:323-34. [Crossref] [PubMed]
- Kather JN, Suarez-Carmona M, Charoentong P, et al. Topography of cancer-associated immune cells in human solid tumors. Elife 2018;7:e36967. [Crossref] [PubMed]
- Tarantino P, Mazzarella L, Marra A, et al. The evolving paradigm of biomarker actionability: Histology-agnosticism as a spectrum, rather than a binary quality. Cancer Treat Rev 2021;94:102169. [Crossref] [PubMed]
- Ahmed R, Augustine R, Valera E, et al. Spatial mapping of cancer tissues by OMICS technologies. Biochim Biophys Acta Rev Cancer 2022;1877:188663. [Crossref] [PubMed]
- Tsvetkov P, Coy S, Petrova B, et al. Copper induces cell death by targeting lipoylated TCA cycle proteins. Science 2022;375:1254-61. [Crossref] [PubMed]
- Jiang Y, Huo Z, Qi X, et al. Copper-induced tumor cell death mechanisms and antitumor theragnostic applications of copper complexes. Nanomedicine (Lond) 2022;17:303-24. [Crossref] [PubMed]
- Kim H, Wu X, Lee J. SLC31 (CTR) family of copper transporters in health and disease. Mol Aspects Med 2013;34:561-70. [Crossref] [PubMed]
- Eisses JF, Kaplan JH. The mechanism of copper uptake mediated by human CTR1: a mutational analysis. J Biol Chem 2005;280:37159-68. [Crossref] [PubMed]
- Maryon EB, Molloy SA, Ivy K, et al. Rate and regulation of copper transport by human copper transporter 1 (hCTR1). J Biol Chem 2013;288:18035-46. [Crossref] [PubMed]
- Aupič J, Lapenta F, Janoš P, et al. Intrinsically disordered ectodomain modulates ion permeation through a metal transporter. Proc Natl Acad Sci U S A 2022;119:e2214602119. [Crossref] [PubMed]
- Wei J, Wang S, Zhu H, et al. Hepatic depletion of nucleolar protein mDEF causes excessive mitochondrial copper accumulation associated with p53 and NRF1 activation. iScience 2023;26:107220. [Crossref] [PubMed]
- Das A, Ash D, Fouda AY, et al. Cysteine oxidation of copper transporter CTR1 drives VEGFR2 signalling and angiogenesis. Nat Cell Biol 2022;24:35-50. [Crossref] [PubMed]
- Brady DC, Crowe MS, Turski ML, et al. Copper is required for oncogenic BRAF signalling and tumorigenesis. Nature 2014;509:492-6. [Crossref] [PubMed]
- Chen GF, Sudhahar V, Youn SW, et al. Copper Transport Protein Antioxidant-1 Promotes Inflammatory Neovascularization via Chaperone and Transcription Factor Function. Sci Rep 2015;5:14780. [Crossref] [PubMed]
- Grasso M, Bond GJ, Kim YJ, et al. The copper chaperone CCS facilitates copper binding to MEK1/2 to promote kinase activation. J Biol Chem 2021;297:101314. [Crossref] [PubMed]
- Logeman BL, Wood LK, Lee J, et al. Gene duplication and neo-functionalization in the evolutionary and functional divergence of the metazoan copper transporters Ctr1 and Ctr2. J Biol Chem 2017;292:11531-46. [Crossref] [PubMed]
- Wezynfeld NE, Vileno B, Faller P. Cu(II) Binding to the N-Terminal Model Peptide of the Human Ctr2 Transporter at Lysosomal and Extracellular pH. Inorg Chem 2019;58:7488-98. [Crossref] [PubMed]
- Møller LB, Petersen C, Lund C, et al. Characterization of the hCTR1 gene: genomic organization, functional expression, and identification of a highly homologous processed gene. Gene 2000;257:13-22. [Crossref] [PubMed]
- Kahlson MA, Dixon SJ. Copper-induced cell death. Science 2022;375:1231-2. [Crossref] [PubMed]
- Lutsenko S. Human copper homeostasis: a network of interconnected pathways. Curr Opin Chem Biol 2010;14:211-7. [Crossref] [PubMed]
- Barresi V, Trovato-Salinaro A, Spampinato G, et al. Transcriptome analysis of copper homeostasis genes reveals coordinated upregulation of SLC31A1,SCO1, and COX11 in colorectal cancer. FEBS Open Bio 2016;6:794-806. [Crossref] [PubMed]
- Li X, Ma Z, Mei L. Cuproptosis-related gene SLC31A1 is a potential predictor for diagnosis, prognosis and therapeutic response of breast cancer. Am J Cancer Res 2022;12:3561-80.
- Jiang X, Ke J, Jia L, et al. A novel cuproptosis-related gene signature of prognosis and immune microenvironment in head and neck squamous cell carcinoma cancer. J Cancer Res Clin Oncol 2023;149:203-18. [Crossref] [PubMed]
- Song G, Dong H, Ma D, et al. Tetrahedral Framework Nucleic Acid Delivered RNA Therapeutics Significantly Attenuate Pancreatic Cancer Progression via Inhibition of CTR1-Dependent Copper Absorption. ACS Appl Mater Interfaces 2021;13:46334-42. [Crossref] [PubMed]
- Lv H, Liu X, Zeng X, et al. Comprehensive Analysis of Cuproptosis-Related Genes in Immune Infiltration and Prognosis in Melanoma. Front Pharmacol 2022;13:930041. [Crossref] [PubMed]
- Klutstein M, Nejman D, Greenfield R, et al. DNA Methylation in Cancer and Aging. Cancer Res 2016;76:3446-50. [Crossref] [PubMed]
- Issa JP. Aging and epigenetic drift: a vicious cycle. J Clin Invest 2014;124:24-9. [Crossref] [PubMed]
- Easwaran H, Tsai HC, Baylin SB. Cancer epigenetics: tumor heterogeneity, plasticity of stem-like states, and drug resistance. Mol Cell 2014;54:716-27. [Crossref] [PubMed]
- Kishi M, Asgarova A, Desterke C, et al. Evidence of Antitumor and Antimetastatic Potential of Induced Pluripotent Stem Cell-Based Vaccines in Cancer Immunotherapy. Front Med (Lausanne) 2021;8:729018. [Crossref] [PubMed]
- Shen P, Deng X, Hu Z, et al. Rheumatic Manifestations and Diseases From Immune Checkpoint Inhibitors in Cancer Immunotherapy. Front Med (Lausanne) 2021;8:762247. [Crossref] [PubMed]
- Schalper KA, Brown J, Carvajal-Hausdorf D, et al. Objective measurement and clinical significance of TILs in non-small cell lung cancer. J Natl Cancer Inst 2015;107:dju435. [Crossref] [PubMed]
- Bremnes RM, Busund LT, Kilvær TL, et al. The Role of Tumor-Infiltrating Lymphocytes in Development, Progression, and Prognosis of Non-Small Cell Lung Cancer. J Thorac Oncol 2016;11:789-800. [Crossref] [PubMed]
- Marshall EA, Ng KW, Kung SH, et al. Emerging roles of T helper 17 and regulatory T cells in lung cancer progression and metastasis. Mol Cancer 2016;15:67. [Crossref] [PubMed]
- Sautès-Fridman C, Lawand M, Giraldo NA, et al. Tertiary Lymphoid Structures in Cancers: Prognostic Value, Regulation, and Manipulation for Therapeutic Intervention. Front Immunol 2016;7:407. [Crossref] [PubMed]
- Petitprez F, de Reyniès A, Keung EZ, et al. B cells are associated with survival and immunotherapy response in sarcoma. Nature 2020;577:556-60. [Crossref] [PubMed]