Construction of an exosome-associated miRNA-mRNA regulatory network and validation of FYCO1 and miR-17-5p as potential biomarkers associated with ovarian cancer
Highlight box
Key findings
• FYVE and coiled-coil domain containing 1 (FYCO1) was predicated and identified as the target gene of hsa-miR-17-5p.
What is known and what is new?
• A miRNA-mRNA network was constructed including 3 miRNAs (hsa-miR-17-5p, hsa-miR-20b-5p and hsa-miR-20a-5p) and 2 mRNAs [FYCO1 and purine rich element binding protein A (PURA)].
• This report added a possible mechanism of ovarian cancer (OV) related with exosomes.
What is the implication, and what should change now?
• As a potential key target gene, the expression of FYCO1 in OV exosomes may have certain diagnostic value. Experimental validation of the role of FYCO1 in OV should be conducted in the further research.
Introduction
In 2022, an estimated of 19,880 women were newly diagnosed with ovarian cancer (OV) in United States, and it caused 12,810 deaths. For the worldwide, the death toll is more than 140,000 (1,2). As one of the three major gynaecological malignancies, it has the highest mortality rate among gynaecological malignancies, with a global average incidence of nearly 10 in 100,000 women. With the widespread use of oral contraceptives, the incidence of OV has declined, but it remains the seventh most common cancer in women worldwide (1,3,4). In women over 40 years old, OV is second only to breast cancer in terms of incidence. The 5-year survival rate for patients with early-stage (stage I) OV can be as high as 92%, while the 5-year survival rate for patients with advanced OV is only 29%, and the overall 5-year survival rate for patients at all stages is 49.7% (based on the collected data between 2012 to 2018) (2,5,6). Early clinical manifestations of OV are not obvious and there are few pre-symptoms. Unfortunately, most OVs have developed to an advanced stage by the time they are diagnosed (7,8), making radical treatment difficult. Early diagnosis of OV remains a major challenge to meet public health needs (9,10). Therefore, elucidating the molecular mechanisms of OV initiation, progression and metastasis and finding effective biomarkers are essential for early diagnosis, treatment selection, follow-up schedules and prognostic assessment to prolong patient life expectancy and clinical benefit.
Exosomes are a subset of extracellular vesicles (EVs) with a diameter of 50–150 nm and have been most extensively investigated as one of the three major subgroups (exosomes, microvesicles and apoptotic vesicles) of EVs, which are released by mammalian cells in response to stimulation by normal and pathogenic factors by a variety of cell types, including tumor cells, macrophages, mast cells, mesenchymal stem cells, lymphocytes, and fibroblasts (11-15). Some specific proteins and genetic materials in exosomes reflect their cellular origin and physiological status and can be explored as biomarkers for early clinical diagnosis, treatment and prognosis of many cancers (16). In addition, exosomes secreted by some cells, including mesenchymal stem cells and cancer cells, can be used to treat various diseases. In the last decade, the study of exosomes in cancer has progressed rapidly. As one of the components of the tumor microenvironment, exosomes have been shown to have important functions in cancer development, progression, metastasis, angiogenesis, and immunity (17,18). In addition, exosomes can mediate intercellular communication by transferring mRNAs, microRNAs (miRNAs), long non-coding RNAs, proteins and DNA (18). MiRNAs are considered to be an evolutionarily conserved family of molecules, and most miRNAs in exosomes act as tumor promoters or repressors that affect other cells in the tumor microenvironment, thereby altering their biological phenotype (19). Conversely, cancer cells receive exosomes released from stromal cells, which also affect the proliferative or invasive capacity of cancer cells. There is also an increasing number of studies on OV exosomes (16,20,21). It has been shown that MMP1 (matrix metalloproteinase 1) in exosomes from OV cells may play a role in damaging the mesothelium and promoting peritoneal dissemination of cancer cells (22). MiR-21, a cancer-associated fibroblast (CAF)-derived exosome, binds to APAF1 (apoptosis protease activating factor 1) in OV cells, conferring resistance to paclitaxel (23). A comprehensive study of the interaction of miRNAs with other regulatory factors in OV exosomes is still needed.
MiRNAs are a class of small (19–25 nucleotides) non-coding RNAs that regulate gene expression primarily by targeting mRNA in a sequence-specific manner. A growing body of research indicates that miRNA dysregulation is a common feature of the tumorigenic development process, and miRNAs are closely associated with the development of a variety of cancers, including breast, lung and OVs (24-27). Mechanistic studies have shown that miRNAs are involved in a number of important cellular processes, including cell proliferation, apoptosis and vascular neogenesis (24,25,27). MiRNAs are becoming increasingly popular in the study of OV. MiR-205 promotes OV cell invasion and metastasis by suppressing the expression of the oncogene transient overexpression lysate of transcription factor 21 (TCF21), and its level in plasma exosomes has been identified as a valuable cancer biomarker that may help in the diagnosis of OV (20,28). Also, circulating exosomal miR-1290 is used as a biomarker for differential diagnosis of epithelial OV from benign ovarian neoplasm, and suppressor of cytokine signaling 4 (SOCS4) could be a potential target gene of miR-1290 (29). OV patients with high exosomal miR-1246 expression and low caveolin-1 (CAV1) expression had a significantly worse overall prognosis. Treatment with CAV1 overexpression and miR-1246 inhibition significantly increased the sensitivity of OV cells to paclitaxel stimulation. Further study showed that miR-1246 inhibits CAV1 and acts through the platelet-derived growth factor receptor β (PDGF-β) receptor on recipient cells to suppress cell proliferation (30). Exosomes with low levels of miR-543 promoted OV cell proliferation in vitro and in vivo by targeting insulin-like growth factor 2 (IGF2) (31). In addition, exosomal miR-1260a, miR-7977, miR-192-5p, miR-21-5p, miR-4732-5p and miR-429 et al. (32-35) are also promising diagnostic biomarkers or associated with chemosensitivity. Although a large number of miRNAs have been associated with OV, miRNA in serum exosomes of OV patients have not attracted enough attention. Furthermore, the function of some miRNAs carried by exosomes secreted by OV tissues is unknown. Therefore, the construction of OV-associated ceRNA regulatory network is crucial and may provide more insights into the molecular mechanisms of OV.
In this paper, we speculate that miRNAs carried by exosomes secreted from OV tissues may regulate the expression of other mRNAs in OV tissues and thus participate in the development of OV. First, we investigated the potential signaling regulatory pathway of differentially expressed miRNAs (DEMs) and genes (DEGs) from exosomes using bioinformatics strategies. Second, a miRNA-mRNA interaction network was constructed. Our study elucidated the regulatory roles and relationships of exosome-derived miRNAs in the development of OV. This study provides a new reference for the early diagnosis, subsequent treatment and prognosis of OV. We present this article in accordance with the TRIPOD reporting checklist (available at https://tcr.amegroups.com/article/view/10.21037/tcr-23-940/rc).
Methods
Data resources and preprocessing
First, we searched the public high-throughput gene expression database—Gene Expression Omnibus (GEO; https://www.ncbi.nlm.nih.gov/geoprofiles) (36) for datasets related to OV using the keywords ‘ovarian cancer’ and ‘exosomes’. Microarray data ‘MINiML formatted family file(s)’ of GSE103708, GSE76449, GSE54388, GSE17308, GSE12470 and GSE6008 were downloaded and used for analysis. Biological fluid samples were not included in this study. The data source and attributes of the datasets are summarized in Table 1. For miRNA expression profiling associated with exosomes in OV, a total of 26 exosome samples from OV cell lines and 6 exosome samples from normal human ovarian surface epithelial cell lines were included in GSE103708 (platform: GPL18402 Agilent-046064 Unrestricted_Human_miRNA_V19. 0_Microarray), while 24 exosome samples from OV cell lines and 4 exosome samples from normal ovarian cell lines were included in GSE76449 (platform: GPL19117 Affymetrix Multispecies miRNA-4 Array). For gene/mRNA expression profiling in OV, 16 OV surface epithelium samples and 6 ovarian surface epithelium samples were included in GSE54388 (platform: GPL570 Affymetrix Human Genome U133 Plus 2.0 Array), while 35 OV surface epithelium samples and 11 non-OV surface epithelium samples (4 normal and 7 benign) were included in GSE17308 (platform: GPL8926 PC Human Operon 21k v2). Forty-three OV tissues and 10 normal peritoneal samples were enrolled in GSE12470 (platform: GPL887 Agilent-012097 Human 1A Microarray), while 41 individual ovarian tumors and 4 individual normal ovarian samples were enrolled in GSE6008 (platform: GPL96 Affymetrix Human Genome U133A Array). The study was conducted in accordance with the Declaration of Helsinki (as revised in 2013).
Table 1
GEO No. | Sample number | Platform | Type of experiment | |
---|---|---|---|---|
Normal sample | OV sample | |||
GSE103708 | 6 | 26 | GPL18402 Agilent-046064 Unrestricted_Human_miRNA_V19.0_Microarray (miRNA ID version) | Non-coding RNA profiling by array |
GSE76449 | 4 | 24 | GPL19117 [miRNA-4] Affymetrix Multispecies miRNA-4 Array | Non-coding RNA profiling by array |
GSE54388 | 6 | 16 | GPL570 [HG-U133_Plus_2] Affymetrix Human Genome U133 Plus 2.0 Array | Expression profiling by array |
GSE17308 | 11 | 35 | GPL8926 PC Human Operon 21k v2 | Expression profiling by array |
GSE12470 | 10 | 43 | GPL887 Agilent-012097 Human 1A Microarray (V2) G4110B (Feature Number version) | Expression profiling by array |
GSE6008 | 4 | 41 | GPL96 [HG-U133A] Affymetrix Human Genome U133A Array | Expression profiling by array |
GEO, Gene Expression Omnibus; OV, ovarian cancer.
Screening of DEMs and DEGs
First, all data were normalized using the DESeq2 R package to ensure accuracy. They were then analysed separately. The interactive online tool GEO2R (www.ncbi.nlm.nih.gov/geo/geo2r) (37) was used to screen the DEMs between non-OV exosome samples and OV exosome samples. P value <0.05, adjusted P value <0.01 and |logFC| >1 were selected as the cut-off standard. For DEMs related to exosomes in OV, the overlapping miRNAs in GSE103708 and GSE76449 were selected as DEMs. Next, the DEGs between non-OV samples and OV samples were also screened by GEO2R. P value <0.05, adjusted P value <0.01 and |logFC| >1 were considered as cut-off criteria. For DEGs in OV, the overlapping mRNAs in GSE54388, GSE17308, GSE12470 and GSE6008 were selected as DEGs. The Venn diagram was generated using an online website (http://bioinformatics.psb.ugent.be/webtools/Venn/).
Gene ontology (GO) and Kyoto Encyclopedia of Genes and Genomes (KEGG) enrichment analysis
GO and KEGG pathway analyses were performed to predict the possible function of these DEGs using the “clusprofiler” R package (38). GO enrichment analysis is a common approach for functional studies of genomic or transcriptomic data (39). It has been classified into three distinct aspects of biological content: biological process (BP), cellular component (CC) and molecular function (MF). GO and KEGG analyses were performed using the DAVID (distributed access view integrated database) database (40). For GO analysis, q-value <0.05 was considered statistically significant. For KEGG analysis, P value <0.05 was set as the threshold.
Construction of exosomal miRNA-mRNA network
Here, the target genes of these miRNA signatures were obtained used the miRDB (41,42), miRTarBase (43) and TargetScan databases (44). The genes screened from the above databases were considered to be the target genes of these miRNAs. The predicted target genes were then compared with the DEGs. Construction of exosomal miRNA-mRNA networks using only the remaining overlapping genes and their interacting pairs. In our study, the mRNAs in the network were considered as hub mRNAs. Consequently, the miRNA-mRNA network was inextricably linked to exosomes in OV.
Validation of the miRNA-mRNA regulatory network
Gene Expression Profiling Interactive Analysis (GEPIA2; http://gepia2.cancer-pku.cn/) was used to verify the expression level of mRNAs in the miRNA-mRNA network based on The Cancer Genome Atlas (TCGA; https://portal.gdc.cancer.gov/). GEPIA2 is a public, open website that analyses cancer types and large amounts of genetic data. It provides free access to cancer type and gene data and allows users to validate biomarkers or target potential genes.
Validation of hub-genes on prognostic survival of OV
The Kaplan-Meier Plotter (http://kmplot.com/analysis/) (45) and GEPIA2 tools (46) were used to validate overall survival (OS) and progression-free survival (PFS) or recurrence-free survival (RFS) of hub genes in the miRNA-mRNA network. The Kaplan-Meier Plotter is a freely accessible website that provides a search service for correlations between the expression of all genes and survival in various tumor types, including breast, ovarian, lung and gastric cancer. Sources for these databases include GEO, EGA and TCGA.
Luciferase reporter assay
HEK-293T cells (5×104/well) were seeded in a 24-well plate maintained in a humidified atmosphere containing 5% CO2 at 37 ℃. After a 12 h-culture, when the cell confluency reached approximately 80%, cells were co-transfected with re-constructed plasmids (luciferase reporter containing wild type or mutant target site of miR-17-5p constructed based on Con245 plasmid vector), Renilla luciferase vector and miR-17-5p mimics (150 nM, Genecopoeia, Guangzhou, China) or mimics negative control (NC) using lipofectamine 3000 (Invitrogen, Waltham, MA, USA) for 48 h as previously described (47,48). The relative luciferase activity assay was conducted using a luciferase reporter gene assay system (Genecopoeia) equipped with a Glomax plate reader (Promega, Madison, WI, USA). The relative firefly luciferase activity was normalized with Renilla luciferase activity.
Cell culture and quantitative reverse transcription-polymerase chain reaction (qRT-PCR)
The SKOV3 cell line was obtained from the American Type Culture Collection (ATCC; Manassas, VA, USA). Cells were incubated in Dulbecco’s modified Eagle’s medium (DMEM; Gibco, Grand Island, NY, USA) containing 10% fetal bovine serum (Gibco), 100 IU/mL penicillin/streptomycin at 37 ℃ in a humidified atmosphere containing 5% CO2. Total cell RNA was extracted with TRIzol according to the manufacturer’s protocol (Sigma-Aldrich, St. Louis, MO, USA). RNA (0.5 µg) was reverse transcribed into cDNA using PrimeScript RT Master Mix (TaKaRa, Otsu, Japan). The qRT-PCR was performed using SYBR Green qPCR Super Mixture (Takara, Tokyo, Japan) and a StepOnePlus Real-Time PCR System (Thermo Fisher Scientific, Inc., Waltham, MA, USA). The following primer sequences were used: GAPDH (forward: TGGTCGTATTGGGCGCCTGGT; reverse: TCGCTCCTGGAAGATGGTGA) and FYVE and coiled-coil domain containing 1 (FYCO1) (forward: GTTCGCCTCACTTGCTTGGTAG; reverse GTGTGGTAGTCCTCCTCCTTCTC). FYCO1 mRNA expression level fold change was calculated using 2−ΔΔCt.
Statistical analysis
The differences between groups were analysed by paired Student’s t-test, and differences in multiple groups were analysed by one-way analysis of variance (ANOVA). All statistical analyses were performed using GraphPad Prism 7.0 software (GraphPad Prism 7 Software, San Diego, CA, USA). P<0.05 was considered statistically significant.
Results
Identification of DEMs and DEGs
The whole workflow of our study is shown in Figure 1. For DEMs, A total of 192 DEMs (32 up-regulated and 160 down-regulated) were screened from the GSE103708 dataset, while 201 DEMs (72 up-regulated and 129 down-regulated) were screened from GSE76449 (Figure 2A). As shown in Figure 2B, 14 overlapping miRNAs in GSE103708 and GSE76449 were selected as DEMs (3 up-regulated and 11 down-regulated). For DEGs, a total of 8,729 DEGs (5,452 up-regulated and 3,277 down-regulated), 1,526 DEGs (760 up-regulated and 766 down-regulated), 5,765 DEGs (4,324 up-regulated and 1,441 down-regulated) and 6,494 DEGs (3,328 up-regulated and 3,166 down-regulated) were screened from GSE54388, GSE17308, GSE12470 and GSE6008, respectively. The overlapping mRNAs in GSE54388, GSE17308, GSE12470 and GSE6008 were selected as DEGs. Finally, 101 DEGs (67 up-regulated and 34 down-regulated) were screened out (Figure 3A,3B). The DEMs and DEGs were then used for the following analysis.
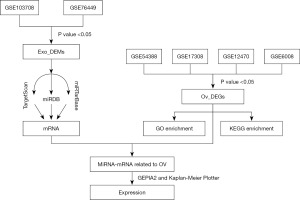
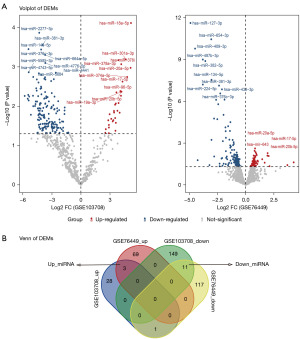
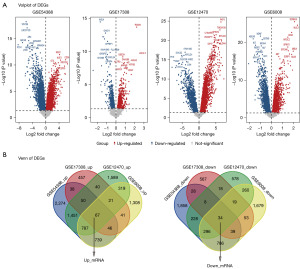
Functional enrichment analysis of DEGs by GO and KEGG
The GO analysis resulted in a total of 101 DEGs mapped to the GO terms. Using false discovery rate (FDR)-corrected P value <0.05 and enrichment score >1.5 as a threshold, significantly enriched functional clusters were identified in the BP, CC and MF terms (as shown in Figure 4A). In the BP group, DEGs were mainly enriched in cell cycle-related processes and nutrient metabolism, such as ‘DNA replication’, ‘DNA-dependent DNA replication’, ‘response to amino acid’. In the CC group, DEGs were mainly enriched in ‘focal adhesion’, ‘cell-substrate junction’. In the MF group, DEGs were mainly enriched in ‘glycosaminoglycan binding’, ‘cadherin binding’. For KEGG enrichment analysis, the DEGs were mainly enriched in ‘base excision repair’, ‘amino acid biosynthesis’, ‘carbon metabolism’, ‘DNA replication’ and also enriched in several signaling pathways such as ‘ras signaling pathway’ and ‘PI3K-Akt signaling pathway’ (Figure 4B).
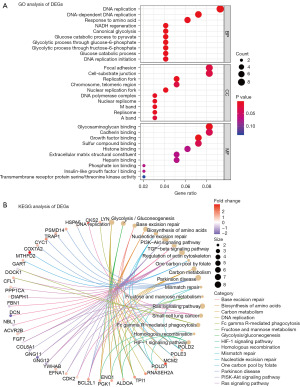
Construction of miRNA-mRNA regulatory network
As the presented miRNA-mRNA network in Figure 5A, the two overlapping genes, FYCO1 and purine rich element binding protein A (PURA) were regulated by three DEMs (hsa-miR-17-5p, hsa-miR-20b-5p and hsa-miR-20a-5p). Interestingly, the three miRNAs were up-regulated while the two mRNAs were down-regulated in exosome tissues of OV disease. These results suggest that the miRNA carried by exosomes from OV tissues may regulate the expression of some crucial mRNA in OV.
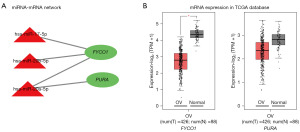
Validation of the miRNAs and mRNAs in miRNA-mRNA regulatory network
Validation of FYCO1 and PURA expression level in normal tissues and OV samples was conducted through the GEPIA2 online database. However, although the expression of the two mRNAs were down-regulated in OV tissues (as shown in Figure 5B) on the basis of gene expression profiles from TCGA and the genotype-tissue expression (GTEx) projects, only the downregulation of FYCO1 expression was statistically significant. At last, the expression trends of FYCO1 and PURA in cancer tissues from OV patients and normal tissues from healthy people based on the GEPIA2 database were in accordance with that based on the GEO database.
Validation of hub-gene FYCO1 and PURA on survival of OV
The Kaplan-Meier Plotter analysis showed that high FYCO1 expression was significantly associated with decreased OS (P=0.001) (Figure 6A) and PFS (P<0.001) (Figure 6B) in OV when using a probe with affymetrix id of 1555523_a_at. However, when a different probe with id 218204_s_at was used, FYCO1 expression levels did not significantly correlate with OS (P=0.33) (Figure 6C) and PFS (P=0.071) (Figure 6D) in OV. Regardless of the probe (204020_at, 204021_s_at, 213806_at or 229167_at) selected, PURA expression level was not associated with OV survival. GEPIA2 analysis showed that both FYCO1 (P=0.33) and PURA (P=0.26) expression were not associated with OS in OV (as shown in Figure S1).
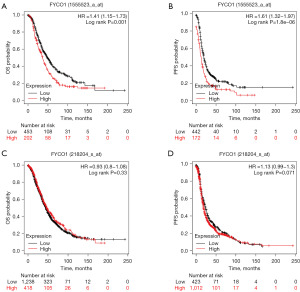
Verification of the mRNA expression and miRNA targets
Luciferase reporter assay was conducted to confirm the potential binding relationship. Wild-type and mutant FYCO1 3'UTR were inserted into the luciferase reporter vector Con245 (Figure 7A,7B), and co-transfected with miR-17-5p mimics in HEK-293A cells. The group with wild-type FYCO1 3'UTR transfection showed a significant decrease in luciferase activity compared to the mimic NC group after transfection with miR-17-5p mimics, whereas no significant difference was observed in the mutant FYCO1 3'UTR transfection group when transfected with miR-17-5p mimics or mimics NC (Figure 7C). To further investigate whether miR-17-5p inhibits endogenous FYCO1 expression, miR-17-5p mimics were transfected into SKOV-3 cells, which resulted in significant inhibition of FYCO1 transcripts (Figure 7D). These results confirmed that miR-17-5p targets FYCO1 in OV cells.
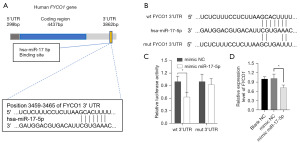
Discussion
OV is more prevalent in middle-aged and older women. It poses a significant threat to the health of this demographic and is the leading cause of death among gynecological tumors (3,5,49). The inconspicuous early symptoms often result in late-stage diagnoses (49). Early diagnosis and treatment can lead to a better prognosis and longer survival. Gene-based diagnosis and treatment, a frontier in biomedical advancement, has exhibited promising results in clinical trials (49). Over the past decade, extensive research has unveiled the release of exosomes by diverse cell types, such as mesenchymal stem cells, macrophages, and tumor cells (12,50,51). Exosomes carry a multitude of nucleic acids, metabolites, proteins, and other bioactive substances. Among these, miRNAs released from cancer cell-derived exosomes play a central role in mediating intercellular communication with normal cells, thus fueling tumor progression, metastasis, and angiogenesis (17,18). Our study aims to explore the miRNA-mRNA interactions in the progression of OV, offering valuable insights for possible early diagnosis and prognosis.
In the present study, we screened a total of 14 DEMs (3 up-regulated, 11 down-regulated) and 101 DEGs (67 up-regulated, 34 down-regulated) from 6 datasets. Both GO and KEGG gene enrichment were used to analyse these DEGs. Meanwhile, miRDB (41,42), miRTarBase (43) and TargetScan databases (44) were used to predict DEMs, and then the predicted mRNAs and previously identified DEGs were intersected, resulting in a miRNA-mRNA interaction network containing three miRNAs and two mRNAs. We further confirmed that FYCO1 was the target of hsa-miR-17-5p. Interestingly, we observed that high expression of the FYCO1 gene may be associated with a lower OS in OV. This observation appears to be contradictory to the findings in Figure 5A,5B, as the expression of FYCO1 was significantly lower in OV samples compared to normal samples. However, it should be noted that the choice of different FYCO1 probes, such as 218204_s_at (as demonstrated in Figure 6C,6D), can also influence the assessment of the relationship between the expression of FYCO1 and the prognosis of OV. Therefore, FYCO1 shows potential as a diagnostic gene for predicting ovarian carcinogenesis, while its role in prognostic survival of requires further clinical investigation.
Only 14 DEMs were available in this study, and it was not possible to see from the GO and KEGG analysis in which pathways they were more significantly enriched. Therefore, we only show here the enrichment of 101 DEGs on GO and KEGG pathways. Uncontrolled proliferation, infiltration and metastasis, as well as energy wastage or metabolic reprogramming (Warburg effect) are common features of all cancer cells (52,53). GO analysis revealed that these DEGs were mainly enriched in these aspects of cell proliferation, metastasis and amino acid metabolism. In the present study, we used the serous OV samples, which showed that this type of OV is more prominent in proliferation and metastasis (54). Similar to GO analysis, KEGG analysis revealed that DEGs were mainly enriched in DNA replication-related regulatory pathways, in addition to amino acid and carbon metabolism. Géraldine G demonstrated for the first-time metabolic heterogeneity in high-grade serous ovarian cancer (HGSOC) by proteomics and metabolomics, where low-oxidative phosphorylation is predominantly glycolytic and high-oxidative phosphorylation is dependent on glutamine and fatty acid-supported oxidative phosphorylation (55). High levels of extracellular glutamine promote OV cell proliferation, and its important metabolic enzyme glutamine synthetase (GS) is positively correlated with poor prognosis (56). Furthermore, KEGG enrichment analysis of downregulated DEGs was also associated with renin-angiotensin system (Ras) and phosphatidylinositol 3-kinase/protein kinase B (PI3K/Akt) signaling pathways. It has been shown that PI3K/Akt is highly mutated and/or over-activated in most OV patients, it plays an important role in OV tumorigenesis, proliferation and is associated with advanced grade and poor prognosis (57,58). Itamochi et al. (59) found that 3-year OS was significantly higher in patients with activated PI3K/Akt and RTK/Ras signaling pathways, implying that both pathways are associated with OV progression. Our enrichment results suggest that the DEGs screened in the above datasets are trustworthy and that the pathways enriched by these DEGs are highly correlated with important features of selected serous OVs.
The miRNA-mRNA regulatory network was constructed by interacting the DEGs obtained from the target genes predicted by the DEMs with the other four datasets. The only three miRNAs in this network, all of which are upregulated, have been extensively reported in the literature (about 270 articles in the last 5 years), however, except for miR-17-5p, the remaining two miRNAs have not been reported in ovarian-related studies. The combined studies suggest that miR-17-5p may play a role as a pro-oncogenic factor in a variety of cancers (60-62). By targeting heparan sulfate proteoglycan 2 (HSP2) and cell adhesion molecule 2 (CADM2), two tumor suppressor genes, it inhibits apoptosis and promotes proliferation, migration and invasion of colorectal cancer cells (63,64). Another study showed that hsa-miR-17-5p acts on multiple targets of the transforming growth factor-β (TGF-β) signaling pathway, and maintains long-term downregulation before and after conventional or combination chemotherapy, making hsa-miR-17-5p a potential biomarker for effective combination chemotherapy in colorectal cancer (65). Similarly, miR-17-5p was expressed 2-fold higher in peripheral lymphocytes of OV patients compared to healthy controls. Also, miR-17-5p expression levels were higher in OV patients with a history of smoking compared to non-smoking patients (66). The remaining two miRNAs belonging to the miR-20-5p family have not been studied in OV and their effect on tumor progression is controversial. In the studies of bladder cancer and prostate cancer, miR-20a-5p was considered as a negative factor for cancer progression (67,68). By promoting epithelial-mesenchymal transition (EMT), miR-20a-5p overexpression promotes the proliferation, invasion and migration of bladder cancer cells (67). In contrast, miR-20a-5p has been implicated a tumor suppressor in endometrial cancer, inhibiting cancer cell proliferation, invasion and adhesion of cancer cells by decreasing janus kinase 1 (JAK1) expression (69). Like hsa-miR-20a-5p, hsa-miR-20b-5p expression is upregulated in prostate cancer (70). High expression of hsa-miR-20b-5p in leukemic cells reduces apoptosis and promotes proliferation. This may be related to the fact that hsa-miR-20b-5p inhibits the expression of phosphatase and tensin homolog deleted on chromosome ten (PTEN) and Bcl-2 interacting mediator (BIM) of cell death, two important tumor suppressor genes (71). However, this miRNA is a tumor suppressor in colon cancer, and through the cyclin D1 (CCND1)/cyclin-dependent kinase 4 (CDK4)/forkhead box protein M1 (FOXM1) axis it achieves regulation of cell cycle, migration and invasion (72). We tried to explore the differences in the expression of these miRNAs in normal and OV samples using a web-based database (http://bioinfo.jialab-ucr.org/CancerMIRNome), which unexpectedly contains only cancer samples and lacks corresponding normal samples. Due to the limited clinical resources, we could not collect enough clinical OV samples to verify whether the above miRNAs are associated with tumorigenesis, progression and prognosis, and we will explore the relationship between these miRNAs and OV in more detail in future studies.
Among the elements of the regulatory networks we constructed, two down-regulated mRNAs, FYCO1 (73,74) and PURA (47,75), correspond to miRNAs. Interestingly, these genes have rarely been reported in tumor studies. Nevertheless, FYCO1 has been increasingly studied in recent years. There are 21 articles on FYCO1 research in the last 10 years, including 16 in the last 5 years and 12 in 2021 to date. FYCO1 is an adaptor protein that binds to autophagy-associated proteins, such as microtubule-associated protein 1 light chain3 (LC3), and functionally assists in the transport of autophagic vesicles (76,77). Mutations in the FYCO1 gene can affect lens development and transparency, ultimately leading to cataract development (78). During starvation or stress overload stage, FYCO1 rescues impaired cardiac function by inducing autophagy (79). Importantly, FYCO1 reduces post-mitotic midbody (MB) accumulation by regulating MB degradation, thereby reducing anchorage-independent growth and invasiveness of HeLa and squamous cell carcinoma cells (80). In our previous enrichment analysis, we also found that OV exosomal DEGs were mainly enriched in pathways associated with cancer cell proliferation and invasion; therefore, our study is consistent with literature reports. Most of the research on the functions of PURA is related to developmental disorders of the central nervous system (81). However, one article reported that PURA is a potential downstream target of miR-144. Overexpression of miR-144 promotes esophageal cancer cell proliferation and migration accompanied with significantly decreased PURA mRNA expression (47). Although the expression level of PURA between normal sample and OV sample was not significant, as a regulatory network member, PURA showed the common effects among the different types of cancer.
Unexpectedly, both FYCO1 and PURA were found to have no significant relationship with prognostic survival of OV using GEPIA2 survival analysis (Figure S1). Interestingly, the higher but not lower expression of FYCO1 was associated with lower OS and PFS as verified by Kaplan-Meier Plotter analysis (probe: 1555523_a_at) (45), other probe of FYCO1 showed no significant relation with survival of OV. In fact, the lower expression level of FYCO1 was a negative factor for survival in most cancers (82), such as breast cancer (OS, P<0.01, probe: 218204_s_at; RFS, P<0.001, all probes, Kaplan-Meier Plotter), gastric cancer (OS, P<0.001, 218204_s_at, Kaplan-Meier Plotter) and lung cancer (OS, P<0.001, probe: 218204_s_at, Kaplan-Meier Plotter; OS, P<0.05, GEPIA2) (Figure S2). Although no study has been reported on the relationship between FYCO1 expression and tumor survival. Due to the limited number of OV samples collected and the observation time, a statistically significant survival analysis could not be performed.
Conclusions
In summary, we constructed a miRNA-mRNA regulatory network. By target prediction and interaction, we identified three miRNAs (hsa-miR-17-5p, hsa-miR-20b-5p and hsa-miR-20a-5p) and two mRNAs (FYCO1 and PURA) that were associated with ovarian carcinogenesis. By functional validation, our study reported for the first time that FYCO1 was significantly downregulated in OV, and this result was validated in clinical samples tested by qPCR. However, the impact of FYCO1 expression level on the prognostic survival of OV patients remains controversial, which may be mainly related to our use of cancer cell exosomes as the study subject. The present study provides new ideas and new opportunities for OV diagnosis and prognostic survival. As more standardized OV exosome data are developed in the future, the relationship between FYCO1 and OV prognosis will become clearer.
Acknowledgments
Funding: This research was supported by
Footnote
Reporting Checklist: The authors have completed the TRIPOD reporting checklist. Available at https://tcr.amegroups.com/article/view/10.21037/tcr-23-940/rc
Peer Review File: Available at https://tcr.amegroups.com/article/view/10.21037/tcr-23-940/prf
Conflicts of Interest: All authors have completed the ICMJE uniform disclosure form (available at https://tcr.amegroups.com/article/view/10.21037/tcr-23-940/coif). The authors have no conflicts of interest to declare.
Ethical Statement: The authors are accountable for all aspects of the work in ensuring that questions related to the accuracy or integrity of any part of the work are appropriately investigated and resolved. The study was conducted in accordance with the Declaration of Helsinki (as revised in 2013).
Open Access Statement: This is an Open Access article distributed in accordance with the Creative Commons Attribution-NonCommercial-NoDerivs 4.0 International License (CC BY-NC-ND 4.0), which permits the non-commercial replication and distribution of the article with the strict proviso that no changes or edits are made and the original work is properly cited (including links to both the formal publication through the relevant DOI and the license). See: https://creativecommons.org/licenses/by-nc-nd/4.0/.
References
- Penny SM. Ovarian Cancer: An Overview. Radiol Technol 2020;91:561-75.
- National Cancer Institute. Surveillance, Epidemiology, and End Results (SEER) Program. Cancer stat facts: ovarian cancer; 1973 Jan 1 [cited 2023 May 30]. Available online: https://seer.cancer.gov/statfacts/html/ovary.html
- Arora T, Mullangi S, Lekkala MR. Ovarian Cancer. Treasure Island, FL, USA: StatPearls Publishing, 2023.
- Webb PM, Jordan SJ. Epidemiology of epithelial ovarian cancer. Best Pract Res Clin Obstet Gynaecol 2017;41:3-14. [Crossref] [PubMed]
- Stewart C, Ralyea C, Lockwood S. Ovarian Cancer: An Integrated Review. Semin Oncol Nurs 2019;35:151-6. [Crossref] [PubMed]
- Jessmon P, Boulanger T, Zhou W, et al. Epidemiology and treatment patterns of epithelial ovarian cancer. Expert Rev Anticancer Ther 2017;17:427-37. [Crossref] [PubMed]
- Jayson GC, Kohn EC, Kitchener HC, et al. Ovarian cancer. Lancet 2014;384:1376-88. [Crossref] [PubMed]
- Bray F, Ferlay J, Soerjomataram I, et al. Global cancer statistics 2018: GLOBOCAN estimates of incidence and mortality worldwide for 36 cancers in 185 countries. CA Cancer J Clin 2018;68:394-424. [Crossref] [PubMed]
- Rampes S, Choy SP. Early diagnosis of symptomatic ovarian cancer in primary care in the UK: opportunities and challenges. Prim Health Care Res Dev 2022;23:e52. [Crossref] [PubMed]
- Lawson-Michod KA, Watt MH, Grieshober L, et al. Pathways to ovarian cancer diagnosis: a qualitative study. BMC Womens Health 2022;22:430. [Crossref] [PubMed]
- Kalluri R, LeBleu VS. The biology, function, and biomedical applications of exosomes. Science 2020;367:eaau6977. [Crossref] [PubMed]
- Pegtel DM, Gould SJ. Exosomes. Annu Rev Biochem 2019;88:487-514. [Crossref] [PubMed]
- Mashouri L, Yousefi H, Aref AR, et al. Exosomes: composition, biogenesis, and mechanisms in cancer metastasis and drug resistance. Mol Cancer 2019;18:75. [Crossref] [PubMed]
- Liu J, Ren L, Li S, et al. The biology, function, and applications of exosomes in cancer. Acta Pharm Sin B 2021;11:2783-97. [Crossref] [PubMed]
- Kalluri R. The biology and function of exosomes in cancer. J Clin Invest 2016;126:1208-15. [Crossref] [PubMed]
- Zhou Y, Zhang Y, Gong H, et al. The Role of Exosomes and Their Applications in Cancer. Int J Mol Sci 2021;22:12204. [Crossref] [PubMed]
- Wang S, Wang J, Wei W, et al. Exosomes: The Indispensable Messenger in Tumor Pathogenesis and the Rising Star in Antitumor Applications. Adv Biosyst 2019;3:e1900008. [Crossref] [PubMed]
- Zhang L, Yu D. Exosomes in cancer development, metastasis, and immunity. Biochim Biophys Acta Rev Cancer 2019;1871:455-68. [Crossref] [PubMed]
- Zhao L, Liang X, Wang L, et al. The Role of miRNA in Ovarian Cancer: an Overview. Reprod Sci 2022;29:2760-7. [Crossref] [PubMed]
- He L, Zhu W, Chen Q, et al. Ovarian cancer cell-secreted exosomal miR-205 promotes metastasis by inducing angiogenesis. Theranostics 2019;9:8206-20. [Crossref] [PubMed]
- Li Z, Yan-Qing W, Xiao Y, et al. Exosomes secreted by chemoresistant ovarian cancer cells promote angiogenesis. J Ovarian Res 2021;14:7. [Crossref] [PubMed]
- Ghafouri-Fard S, Shoorei H, Taheri M. miRNA profile in ovarian cancer. Exp Mol Pathol 2020;113:104381. [Crossref] [PubMed]
- Wang Y, Chen G, Dai F, et al. miR-21 Induces Chemoresistance in Ovarian Cancer Cells via Mediating the Expression and Interaction of CD44v6 and P-gp. Onco Targets Ther 2021;14:325-36. [Crossref] [PubMed]
- Hill M, Tran N. miRNA interplay: mechanisms and consequences in cancer. Dis Model Mech 2021;14:dmm047662. [Crossref] [PubMed]
- Zhang Y, Yang P, Wang XF. Microenvironmental regulation of cancer metastasis by miRNAs. Trends Cell Biol 2014;24:153-60. [Crossref] [PubMed]
- Schmeier S, Schaefer U, Essack M, et al. Network analysis of microRNAs and their regulation in human ovarian cancer. BMC Syst Biol 2011;5:183. [Crossref] [PubMed]
- Lu TX, Rothenberg ME. MicroRNA. J Allergy Clin Immunol 2018;141:1202-7. [Crossref] [PubMed]
- Zhu Z, Chen Z, Wang M, et al. Detection of plasma exosomal miRNA-205 as a biomarker for early diagnosis and an adjuvant indicator of ovarian cancer staging. J Ovarian Res 2022;15:27. [Crossref] [PubMed]
- Jeon H, Seo SM, Kim TW, et al. Circulating Exosomal miR-1290 for Diagnosis of Epithelial Ovarian Cancer. Curr Issues Mol Biol 2022;44:288-300. [Crossref] [PubMed]
- Kanlikilicer P, Bayraktar R, Denizli M, et al. Exosomal miRNA confers chemo resistance via targeting Cav1/p-gp/M2-type macrophage axis in ovarian cancer. EBioMedicine 2018;38:100-12. [Crossref] [PubMed]
- Zhang S, Pan D, Zhang S, et al. Exosomal miR-543 Inhibits the Proliferation of Ovarian Cancer by Targeting IGF2. J Immunol Res 2022;2022:2003739. [Crossref] [PubMed]
- Chen L, Wang K, Li L, et al. Plasma exosomal miR-1260a, miR-7977 and miR-192-5p as diagnostic biomarkers in epithelial ovarian cancer. Future Oncol 2022;18:2919-31. [Crossref] [PubMed]
- Zhuang L, Zhang B, Liu X, et al. Exosomal miR-21-5p derived from cisplatin-resistant SKOV3 ovarian cancer cells promotes glycolysis and inhibits chemosensitivity of its progenitor SKOV3 cells by targeting PDHA1. Cell Biol Int 2021;45:2140-9. [Crossref] [PubMed]
- Liu J, Yoo J, Ho JY, et al. Plasma-derived exosomal miR-4732-5p is a promising noninvasive diagnostic biomarker for epithelial ovarian cancer. J Ovarian Res 2021;14:59. [Crossref] [PubMed]
- Li T, Lin L, Liu Q, et al. Exosomal transfer of miR-429 confers chemoresistance in epithelial ovarian cancer. Am J Cancer Res 2021;11:2124-41.
- Clough E, Barrett T. The Gene Expression Omnibus Database. Methods Mol Biol 2016;1418:93-110. [Crossref] [PubMed]
- Barrett T, Wilhite SE, Ledoux P, et al. NCBI GEO: archive for functional genomics data sets--update. Nucleic Acids Res 2013;41:D991-5. [Crossref] [PubMed]
- Wu T, Hu E, Xu S, et al. clusterProfiler 4.0: A universal enrichment tool for interpreting omics data. Innovation (Camb) 2021;2:100141. [Crossref] [PubMed]
- Hulsegge I, Kommadath A, Smits MA. Globaltest and GOEAST: two different approaches for Gene Ontology analysis. BMC Proc 2009;3:S10. [Crossref] [PubMed]
- Ashburner M, Ball CA, Blake JA, et al. Gene ontology: tool for the unification of biology. The Gene Ontology Consortium. Nat Genet 2000;25:25-9. [Crossref] [PubMed]
- Chen Y, Wang X. miRDB: an online database for prediction of functional microRNA targets. Nucleic Acids Res 2020;48:D127-31. [Crossref] [PubMed]
- Liu W, Wang X. Prediction of functional microRNA targets by integrative modeling of microRNA binding and target expression data. Genome Biol 2019;20:18. [Crossref] [PubMed]
- Huang HY, Lin YC, Cui S, et al. miRTarBase update 2022: an informative resource for experimentally validated miRNA-target interactions. Nucleic Acids Res 2022;50:D222-30. [Crossref] [PubMed]
- Agarwal V, Bell GW, Nam JW, et al. Predicting effective microRNA target sites in mammalian mRNAs. Elife 2015;4:e05005. [Crossref] [PubMed]
- Győrffy B. Discovery and ranking of the most robust prognostic biomarkers in serous ovarian cancer. Geroscience 2023;45:1889-98. [Crossref] [PubMed]
- Tang Z, Kang B, Li C, et al. GEPIA2: an enhanced web server for large-scale expression profiling and interactive analysis. Nucleic Acids Res 2019;47:W556-60. [Crossref] [PubMed]
- Sharma P, Sharma R. miR-144 functions as an oncomiR in KYSE-410 human esophageal carcinoma cell line in vitro and targets PURA. Neoplasma 2018;65:542-51. [Crossref] [PubMed]
- Zhang H, Li T, Cai X, et al. MicroRNA-203a-3p regulates CoCl(2)-induced apoptosis in human retinal pigment epithelial cells by targeting suppressor of cytokine signaling 3. J Diabetes Complications 2020;34:107668. [Crossref] [PubMed]
- La Vecchia C. Ovarian cancer: epidemiology and risk factors. Eur J Cancer Prev 2017;26:55-62. [Crossref] [PubMed]
- Familtseva A, Jeremic N, Tyagi SC. Exosomes: cell-created drug delivery systems. Mol Cell Biochem 2019;459:1-6. [Crossref] [PubMed]
- Rudraprasad D, Rawat A, Joseph J. Exosomes, extracellular vesicles and the eye. Exp Eye Res 2022;214:108892. [Crossref] [PubMed]
- Hanahan D. Hallmarks of Cancer: New Dimensions. Cancer Discov 2022;12:31-46. [Crossref] [PubMed]
- Hanahan D, Weinberg RA. Hallmarks of cancer: the next generation. Cell 2011;144:646-74. [Crossref] [PubMed]
- Lisio MA, Fu L, Goyeneche A, et al. High-Grade Serous Ovarian Cancer: Basic Sciences, Clinical and Therapeutic Standpoints. Int J Mol Sci 2019;20:952. [Crossref] [PubMed]
- Gentric G, Mieulet V, Mechta-Grigoriou F. Heterogeneity in Cancer Metabolism: New Concepts in an Old Field. Antioxid Redox Signal 2017;26:462-85. [Crossref] [PubMed]
- Furusawa A, Miyamoto M, Takano M, et al. Ovarian cancer therapeutic potential of glutamine depletion based on GS expression. Carcinogenesis 2018;39:758-66. [Crossref] [PubMed]
- Ghoneum A, Said N. PI3K-AKT-mTOR and NFκB Pathways in Ovarian Cancer: Implications for Targeted Therapeutics. Cancers (Basel) 2019;11:949. [Crossref] [PubMed]
- Ediriweera MK, Tennekoon KH, Samarakoon SR. Role of the PI3K/AKT/mTOR signaling pathway in ovarian cancer: Biological and therapeutic significance. Semin Cancer Biol 2019;59:147-60. [Crossref] [PubMed]
- Itamochi H, Oishi T, Oumi N, et al. Whole-genome sequencing revealed novel prognostic biomarkers and promising targets for therapy of ovarian clear cell carcinoma. Br J Cancer 2017;117:717-24. [Crossref] [PubMed]
- Zou M, Zhang Q. miR-17-5p accelerates cervical cancer cells migration and invasion via the TIMP2/MMPs signaling cascade. Cytotechnology 2021;73:619-27. [Crossref] [PubMed]
- Wang M, Zhao M, Guo Q, et al. Non-small cell lung cancer cell-derived exosomal miR-17-5p promotes osteoclast differentiation by targeting PTEN. Exp Cell Res 2021;408:112834. [Crossref] [PubMed]
- Stoen MJ, Andersen S, Rakaee M, et al. High expression of miR-17-5p in tumor epithelium is a predictor for poor prognosis for prostate cancer patients. Sci Rep 2021;11:13864. [Crossref] [PubMed]
- Yu W, Wang J, Li C, et al. miR-17-5p promotes the invasion and migration of colorectal cancer by regulating HSPB2. J Cancer 2022;13:918-31. [Crossref] [PubMed]
- Wang Y, Zhao J, Wang Y, et al. MiR-17-5p Targets and Downregulates CADM2, Activating the Malignant Phenotypes of Colon Cancer Cells. Mol Biotechnol 2022;64:1388-400. [Crossref] [PubMed]
- Despotovic J, Dragicevic S, Nikolic A. Effects of Chemotherapy for Metastatic Colorectal Cancer on the TGF-β Signaling and Related miRNAs hsa-miR-17-5p, hsa-miR-21-5p and hsa-miR-93-5p. Cell Biochem Biophys 2021;79:757-67.
- Saral MA, Tuncer SB, Odemis DA, et al. New biomarkers in peripheral blood of patients with ovarian cancer: high expression levels of miR-16-5p, miR-17-5p, and miR-638. Arch Gynecol Obstet 2022;305:193-201. [Crossref] [PubMed]
- Yang H, Chen Z, Liu Z. MiR-20a-5p Negatively Regulates NR4A3 to Promote Metastasis in Bladder Cancer. J Oncol 2021;2021:1377989. [Crossref] [PubMed]
- Stoen MJ, Andersen S, Rakaee M, et al. Overexpression of miR-20a-5p in Tumor Epithelium Is an Independent Negative Prognostic Indicator in Prostate Cancer-A Multi-Institutional Study. Cancers (Basel) 2021;13:4096. [Crossref] [PubMed]
- He Y, Ma H, Wang J, et al. miR-20a-5p inhibits endometrial cancer progression by targeting janus kinase 1. Oncol Lett 2021;21:427. [Crossref] [PubMed]
- Zhai TY, Dou M, Ma YB, et al. miR-20b-5p is a novel biomarker for detecting prostate cancer. Oncol Lett 2022;24:426. [Crossref] [PubMed]
- Drobna M, Szarzyńska B, Jaksik R, et al. hsa-miR-20b-5p and hsa-miR-363-3p Affect Expression of PTEN and BIM Tumor Suppressor Genes and Modulate Survival of T-ALL Cells In Vitro. Cells 2020;9:1137. [Crossref] [PubMed]
- Yang H, Lin J, Jiang J, et al. miR-20b-5p functions as tumor suppressor microRNA by targeting cyclinD1 in colon cancer. Cell Cycle 2020;19:2939-54. [Crossref] [PubMed]
- Sun X, Zhou L, Wang X, et al. FYCO1 regulates migration, invasion, and invadopodia formation in HeLa cells through CDC42/N-WASP/Arp2/3 signaling pathway. Biochem Cell Biol 2022;100:458-72. [Crossref] [PubMed]
- Ma C, Zhao J, Wu Y, et al. Diagnostic value of abnormal chromosome 3p genes in small-cell lung cancer. Oncol Lett 2022;24:209. [Crossref] [PubMed]
- Lezon-Geyda K, Najfeld V, Johnson EM. Deletions of PURA, at 5q31, and PURB, at 7p13, in myelodysplastic syndrome and progression to acute myelogenous leukemia. Leukemia 2001;15:954-62. [Crossref] [PubMed]
- Xiao W, Yeerken D, Li J, et al. Nlp promotes autophagy through facilitating the interaction of Rab7 and FYCO1. Signal Transduct Target Ther 2021;6:152. [Crossref] [PubMed]
- Khan SY, Ali M, Kabir F, et al. The role of FYCO1-dependent autophagy in lens fiber cell differentiation. Autophagy 2022;18:2198-215. [Crossref] [PubMed]
- Shirzadeh E, Piryaei F, Naddaf H, et al. Two New Variants in FYCO1 Are Responsible for Autosomal Recessive Congenital Cataract in Iranian Population. Cell J 2022;24:546-51. [Crossref] [PubMed]
- Kuhn C, Menke M, Senger F, et al. FYCO1 Regulates Cardiomyocyte Autophagy and Prevents Heart Failure Due to Pressure Overload In Vivo. JACC Basic Transl Sci 2021;6:365-80. [Crossref] [PubMed]
- Dionne LK, Peterman E, Schiel J, et al. FYCO1 regulates accumulation of post-mitotic midbodies by mediating LC3-dependent midbody degradation. J Cell Sci 2017;130:4051-62. [Crossref] [PubMed]
- Spangenberg L, Guecaimburú R, Tapié A, et al. Novel frameshift mutation in PURA gene causes severe encephalopathy of unclear cause. Mol Genet Genomic Med 2021;9:e1622. [Crossref] [PubMed]
- Dufresne J, Bowden P, Thavarajah T, et al. The plasma peptides of breast versus ovarian cancer. Clin Proteomics 2019;16:43. [Crossref] [PubMed]