Axillary nodal prognostic impact of two-dimensional tumor heterogeneity flux in breast cancer: evaluation via dynamic contrast-enhanced magnetic resonance imaging
Introduction
Tumor heterogeneity
The absence of uniformity in certain features characterizing a cancer is referred to as tumor heterogeneity. This property may be described at various stages ranging from molecular and genetic to phenotypic and structural (1,2). The evaluation of heterogeneity may thus include methodologies as biological assays, histological reviews, radiological imaging and theoretical models amongst others (3-6). Extensive work in these areas elucidated several clinical ramifications associated with greater degrees of heterogeneity in tumors. This is particularly valid in breast cancer, where assessment of heterogeneity yields estimations regarding prognostic factors and guides therapeutics (7,8).
Radiological modalities and features
In harboring first, second, and higher order statistics, mined radiological data may be incorporated with clinical data in formulating models to enhance diagnostic, prognostic, and predictive capacities (9). Different imaging modalities as magnetic resonance imaging (MRI), positron emission tomography (PET) and others have been studied in assessing tumor heterogeneity (10,11). Such radiological applications allowed for delineation of an array of texture analysis parameters eligible for quantifying tumor heterogeneity (12). Development of such parameters allowed systemic classification under orders pertaining to the degree of inter-voxel relationships assessed (13). First order variables generally provide composite measures of voxel intensities as a mean, median, standard deviation, etc. Second order variables, including adjacent contrast and entropy, account for the spatial configuration of a set of voxels and gauge relative conditions. Third order variables, including coarseness, busyness, and complexity, display sensitivity for finer relationships through local cluster or neighborhood analysis of grayscale variation (13).
The voxel intensity gradient (VIG) is a second-order contrast-parameter quantifying the directional variation in intensity values amongst adjacent voxels (12). Granted a population of voxels comprising a digitalized structure, this gradient may be computed for each member through consideration of its neighboring elements. The biological manifestations of voxel intensities on dynamic contrast-enhanced (DCE-) MRI include vascular components, with greater values implicating enhanced containment of Gadolinium based radiocontrast agent (14,15). Such adroit localization of contrast is specific to developed vessels in breast cancer, as confirmed in patients and animal models (16,17). Thus spatial regions encompassing the highest variation of voxel intensities, or greatest VIG, are mechanistically linked to harboring augmented angiogenesis. Special care must be implemented in the filtering and exclusion of mature vessels distinct from tumor parenchyma nesting within the structure. Breast DCE-MRI images are capable of undergoing processing via digital algorithms in detecting these vessels (18). MRI further displays exceptional resolution and tissue differentiation in breast cancer, making it suitable as the ideal modality in our study (16,19).
Divergence theorem and boundary analysis
VIG has been evaluated and correlated with clinical outcomes in various categories of cancer (20,21). Unfortunately, the majority of studies investigating these parameters and their biological representations yield results pertaining exclusively to the tumor volume in contrast to its surface. One reason is the presence of more physical voxels and data available for analysis. A second reason is the intrinsic difficulty in gauging dynamics at the tumor surface provided a temporally static image. A certain relationship generating information regarding the surface of an object given measures acquired within its volume is the divergence theorem. It is also referred to as Gauss’s theorem, when applied to a three-dimensional closed surface, and Green’s theorem. It demonstrates application to fields in mathematics, physics and engineering, including radiological analyses (22). The divergence theorem assesses the sum of a vector field’s divergence over an enclosed surface and equates it to the total flux of this field through the boundary of this space. The three-dimensional general form appears as:
Assessment of axillary nodal status
Impactful clinical trials have demonstrated momentous value in identifying breast cancer patients at high-risk for axillary nodal involvement (23,24). The burden of axillary involvement influences staging and management of patients. Previous investigations have shed light on such risk in different breast cancer cohorts utilizing non-invasive techniques as imaging or molecular assays (25-28). In evaluating measures of tumor heterogeneity flux, our project aims to enhance current predictive capabilities regarding metastatic spread to axillary nodes in early stage breast cancer patients. To this effect, our project will model the VIG as a temporally-independent spatial vector field defined in the tumor region described on DCE-MRI in early stage breast cancer patients. The focus on spatial resolution is merited since morphological architecture, which defines the respective VIG field, in addition to DCE signal intensity time course patterns were shown to be preserved given a sacrifice in temporal kinetic information (29). The field will be representative of the degree of radiological heterogeneity inherent to the tumor. Overall objectives involve computing the summated divergence of this VIG field, and employing the divergence theorem to obtain the volume normalized flux of this heterogeneity marker. Pathological records corresponding to the respective tumors will be referenced in formulating prognostic correlations of this novel heterogeneity measure to extra-tumoral axillary nodal status.
Methods
Project framework
Information derived from patient records was incorporated into a secure database established using the institutionally provided online project manager Research Electronic Data Capture REDCap™ (Vanderbilt, Nashville, TN). Consistent compliance in accordance with standards set by HIPAA was enforced through regulation of access rights via the departmental IT branch. Design and implementation of the project was subject to departmental board review and approval. Participants included in the study provided prior informed consent with respect to anonymous data collection and utilization.
Pathological review
A retrospective extraction of pertinent patient data was performed using the University of Miami Electronic Medical Record system. A filtered list of breast cancer patients at the Sylvester Comprehensive Cancer Center allowed for consequential patient accrual. Such patients underwent adjuvant radiation therapy between 2006 and 2015 typically with additional adjuvant chemotherapy or hormonal therapy. Study inclusion criteria necessitate a diagnosis of early stage breast cancer, defined as either T1 or T2 staging through clinical or pathological assessment with clinical nodal-negativity in accordance with the American Joint Committee on Cancer 7th edition classification (30). Inclusion criteria involved patients harboring pathologic N0, N1, and N2 disease. Patients receiving neoadjuvant systemic therapy or surgery prior to their breast MRI were excluded from inclusion. The study group was comprised of 47 women who met the criteria of early stage breast cancer with pre-treatment evaluation via breast MRI. Among the 47 patients analyzed, 33 had T1 tumors and 14 had T2 tumors. Pathologically measured tumor size (mean ± standard deviation) was 1.02±0.48 cm and 3.10±0.86 cm for T1 and T2, respectively. Median age was 54 years ranging from 40 to 85 years. Post-menopausal state was determined on 27 patients (57.4%) and pre-menopausal state on 8 patients (17.0%). Surgical pathology interpretations were acquired by reviewing official reports issued by board-certified pathologists (with 15–20 years of experience in breast pathology interpretation) at the University of Miami. In particular, relevant data identified included the ratio of positive axillary lymph nodes to total number sampled, in addition to tumor size, grade, receptor status, lymphovascular space invasion, and extracapsular extension.
Image acquisition
MRI radiological sequences were obtained at the University of Miami for 41/47 (87.2%) patients while 6/47 patients (12.8%) attained outside imaging. Every patient was uniformly scanned at a 1.5 Tesla field: institutionally, thirty-eight patients were scanned at Magnetom Sonata™ (Siemens, Erlangen, Germany) and outside studies were carried out on Siemens Espree™ (n=2), Siemens Avanto™ (n=2) and Signa HDxt™ (n=2) (GE, Waukesha, USA). The acquisition protocol criteria required a systematic generation of DCE-MRI sequences on a 1.5 Tesla magnet using dedicated breast coils with a mean TR time of 4.3 ms and TE time of 1.51 ms. Axial T1, T2 and inversion recovery sequences were performed. Subsequently, T1, 3-D volume acquisition was obtained using multiple frames in dynamic fashion before and after Gadoteridol (ProHance®, Bracco Imaging, Milan, Italy) administration intravenously at a dose of 0.1 mmol/kg at a rate of 10–60 cc/min. DynaCAD was used for further evaluation of images and performance of specialized curves and analysis of contrast uptake. Maximum intensity projections, subtracted image sets, and 3-D reconstructions were also obtained. Mean voxel resolutions were 0.417×0.417×1.35 mm (0.235 mm3), with mean image data matrices of size 768×768×112 pixels. The imaging protocol used was in accordance with American College of Radiology guidelines.
Radiological algorithm
Analysis of MRI studies was performed utilizing commercially available software MIM™ (MIM Vista Corp, Cleveland, OH). All imaging DCE-MRI sequences were uploaded in MIM and viewed in three orientations (Figure 1A). The tumor was outlined by a board certified, fellowship trained breast radiologist with 14 years of experience and/or radiation oncologist on the DCE-MRI series. The images were exported using MIM as a series of 2-dimensional (2D) slices in .TIFF format. This format was elected to standardize analysis of all axial slices between patients to ensure consistency and accuracy in the 2D computations. Further, a lack of compression allows .TIFF images to forego original data loss and retain compatibility with processing software. This series was processed in the GNU Image Manipulation Program (GIMP) to filter out image components not relevant to displaying the tumor and equalize dimensions of each file (Figure 1B). GIMP was used to superimpose a pure blue background (hexadecimal code #0000ff) saved upon each slice as a copy for tumor delineation and identification by other software (Figure 1C). Mature vessels not pertaining to tumor parenchyma were detected using a RGB intensity threshold of 0.2 relative to unity and excluded. Code scripts pertaining to image series importation, tumor recognition (Figure 1D), mature vessel filtration (Figure 1E) and relevant computations were composed and compiled using commercially available Mathematica™ (Wolfram Research, Champaign, IL, USA).
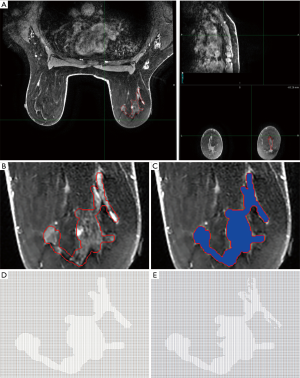
The VIG () was modeled as the vector field of interest in our analysis. It was approximated in 2-dimensions for each pixel (i) with coordinates (xi,yi) encompassed by our tumor perimeter on every 2D axial slice. This was performed by averaging the grayscale values of the two adjacent pixels (xi±∆x,yi) in one dimension and summing it with the result from repeating this operation in the orthogonal direction (xi,yi±∆y). In particular, the pixels one column and row behind the element of interest were subtracted from those one column and row ahead. Grayscale values for each pixel were obtained via the mean of the three red, green, and blue (RGB) components characterizing the pixel. Pixels with adjacent elements falling on or exterior to the border were excluded. A 2D matrix spanning the image length and width was populated with values corresponding to the VIG at each tumor pixel [I'(xi,yi)]. Non-tumor pixels carried a null placeholder. This operation was again applied to the VIG in ascertaining the divergence of the gradient at each pixel []. A new 2D matrix was constructed harboring the divergence values at the respective element locations for each transverse slice. Granted each pixel is identical in length and width (∆x=∆y), its area is equal to a side squared (∆A=∆x2). Since each divergence term carries an inversed squared relationship to a pixel side [], this cancels the element of area incorporated into the sum. Thus, a summation was performed over all non-null values contained in the divergence matrix disregarding dimensional factors. Further computing the series across all slices is representative of the integration of divergence over the tumor’s volume in the divergence theorem. This output was normalized to the total volume of the tumor (V), calculated from the primary contour. This quantity thus approximates the surface flux of the VIG heterogeneity () normalized per unit tumor volume ().
Statistical analysis and validation
Analyses for correlations between the radiologically derived quantities, as well as axillary nodal markers were conducted using Pearson and Spearman correlation coefficients. Cross-correlations amongst the radiologic and remaining pathological data were computed in excluding potential confounders. Multivariable linear regression was analyzed in both step-wise and forced inclusion fashions for known prognostic determinants: age, body mass index (BMI), tumor size, and tumor grade. Similarly, partial correlation coefficients controlling for the effects of these prognostic determinants were derived. A two-tailed P-value of 0.05 was applied as a standard of significance. All analyses were performed using SPSS Statistics® software (version 22; IBM Corporation, Armonk, NY, USA) and plots generated using Excel™ software (version 14.0; Microsoft Corporation, Redmond, WA, USA).
Statistical validation was procured through cross-validation partitioning of the data into training and test sets respectively. Leave-one-out (LOO) techniques were utilized in completely permuting a single member test set through training sets constructed via a linear regression of the withheld members. Predictive capabilities were quantitated using mean squared error and interval normalized root mean square deviation (NRMSD). The code script was composed and compiled using Mathematica™ software.
Results
Patient tumor pathological status
All analyzed patients surgically underwent either lumpectomy or mastectomy of the affected breast followed by radiation therapy. Histologically, 42 patients (89.4%) were diagnosed with invasive ductal carcinoma, 4 patients (8.5%) with invasive lobular carcinoma, and 1 patient (2.1%) with tubular carcinoma. Nottingham histological grading of the breast tumors provided 10 patients (21.3%) with low grade 1, 17 patients (36.2%) with intermediate grade 2, and 20 patients (42.6%) with high grade 3. Lymphovascular invasion was confirmed in specimens from 10 patients (21.3%), and absent or unnoticed in 37 patients (78.7%). Extracapsular extension of the tumor was noted in 5 patients (10.6%). Thirty-six (76.6%) patients displayed positivity for estrogen receptor, 29 (61.7%) for progesterone receptor, and 9 (19.1%) for human epidermal growth factor receptor 2 (HER2). Overall, 10 (21.3%) patients were triple negative for these receptors (Table 1). Receptor status was unavailable for analysis in one patient.
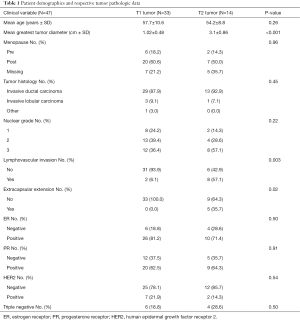
Full table
Patient nodal pathological status
All patients were subject to lymph node assessment through either sentinel node biopsy, axillary node dissection, or both (16 patients with both). Thirty-four patients underwent sentinel lymph node dissections (18 alone without further axillary node dissection) with a median of three nodes collected. These were positive in 14 patients ranging from 1 to 4 nodes. Axillary lymph nodes were completely dissected in a total of 29 patients (13 alone without prior sentinel node biopsy) with a median of 15 nodes collected. Twelve patients returned dissections pathologically negative for metastatic spread (Table 2).
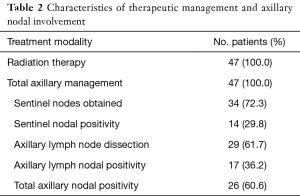
Full table
The degree of total axillary nodes positive to number dissected (TN Ratio) exhibited a median of 0.04 in all patients; range 0 to 1.00, and a median of 0.14 in nodal-positive patients; range 0.03 to 1.00. The degree of non-sentinel axillary nodes positive to number dissected (AN Ratio) exhibited a median of 0.06 in all patients; range 0 to 1.00, and a median of 0.14 in nodal-positive patients; range 0.03 to 1.00. The degree of sentinel nodes positive to number dissected exhibited a median of 0 in all patients; range 0 to 1.00, and a median of 0.50 in nodal-positive patients; range 0.20 to 1.00.
Radiological analysis
MRI sequence analysis and calculations demonstrated a mean contoured tumor volume of 6.32 cm3; range 0.26 to 32.34 cm3. The sum of VIG divergence exhibited a mean value of 84.06 in all patients; range −8.89 to 966.49. The normalized values, representative of the 2D heterogeneity flux, displayed a mean of 9.39 in all patients; range −13.89 to 51.01. In nodal-positive patients, this normalized 2D heterogeneity flux displayed a mean of 11.40 with an identical range. In patients having undergone axillary dissection, this mean is 10.36 and 11.38 for all patients and nodal-positive patients respectively with ranges identical to the cohort.
Normalized 2D VIG divergence correlations
In all patients, the normalized 2D heterogeneity flux (hereby labeled “flux”) was observed to significantly correlate with the TN Ratio and AN Ratio. The Pearson correlation coefficient between flux and TN ratio (Figure 2A) is 0.50 (P<0.001) and AN Ratio (Figure 2B) is 0.57 (P=0.001). After adjusting for age, BMI, tumor size, and grade, Pearson correlation coefficient (i.e., partial correlation) is 0.45 (P=0.003) and 0.51 (P=0.009) for TN Ratio and AN Ratio respectively. These relationships were substantially augmented in nodal-positive patients (AN/TN Ratio ≠0). In such patients, Pearson correlation coefficient between flux and TN ratio (Figure 2C) is 0.58 (P=0.002) and AN Ratio (Figure 2D) is 0.72 (P=0.001). The adjusted partial coefficients are 0.60 (P=0.003) and 0.60 (P=0.032) for nodal-positive TN Ratio and AN Ratio respectively.
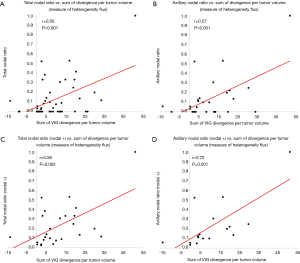
Statistical validation
These significant results were further justified through LOO cross validation studies. Mean squared error for all AN Ratio and TN Ratio was 0.048 (NRMSD =0.22) and 0.036 (NRMSD =0.19) respectively. For nodal-positive patients, these mean squared errors were 0.049 (NRMSD =0.23) and 0.045 (NRMSD =0.22) respectively.
Multivariable analysis
The normalized 2D heterogeneity flux was only further detected to significantly correlate with patient age via Pearson coefficient of 0.32 (P=0.027). Patient age also displayed significant correlation with AN Ratio in nodal-positive patients with coefficient of 0.51 (P=0.036). However, forced inclusion multivariable linear regression in this subgroup yielded no significant relationship between age and AN Ratio with a partial coefficient of 0.23 (P=0.45) in light of including flux. In fact, step-wise multivariable linear regression exclusively incorporated flux as the sole significant independent determinant of all nodal ratios. The standardized beta regression coefficient for flux was 0.469 (P=0.003) and 0.562 (P=0.009) in the case of TN Ratio and AN Ratio respectively. In nodal-positive patients, this regression coefficient for flux was 0.711 (P=0.003) and 0.602 (P=0.032) for TN Ratio and AN Ratio respectively. The flux was not found to significantly cross-correlate with other clinically relevant markers. Similarly, each clinical marker including the AN Ratio and TN Ratio demonstrated no significant cross-correlation with the remaining pathological features analyzed.
Discussion
Heterogeneity flux pertinence and mechanism
To our knowledge, our study is the first in radiologically investigating 2D breast tumor heterogeneity flux through the divergence theorem via summation of VIG divergence. In particular, our analysis focused on ascertaining potential correlations to pathologically acquired axillary prognostic markers. Axillary nodal measures demonstrated several statistically significant correlations. Both the TN Ratio and AN Ratio with significantly linked with 2D heterogeneity flux across all patients evaluated. Further assessment of such correlations in nodal-positive subgroups of patients yielded emphatically improved Pearson coefficient results.
The observed outcomes of our methodology lend a degree of justification to its underlying premise. In formulating an algorithm for calculating the total VIG divergence encompassed in a tumor, we intended on deriving information regarding the normalized VIG flux at its surface. Such flux quantifies an intrinsic emanation portrayed by tumor components as cells and vessels. In light of this fundamental principle, the involvement of extra-tumor features as distant nodal status is mechanistically merited by elevated values of surface flux. It is interesting to contrast intra-tumor pathological markers as size, grade, receptor status and lymphovascular invasion which were not found to significantly correlate with flux.
As is the case with any clinical study, our methodologies and results may be subject to biases and flaws precluding perfect generalization. The number of patients analyzed in retrospective fashion is modest. Although all patients displayed axillary nodal evaluation, which significantly correlated with the flux in terms of positivity, the route of assessment was not uniform. 3-dimensional volumetric analyses were reduced to a series of 2D slice analyses secondary to a pre-determined sampling rate constraint adhered to by the MRI modality. Thus, solely flux exclusively tangential to the acquired transverse slides was subject to investigation using our 2D application of the divergence theorem. Although accurately capable of ascertaining the flux emanating through transverse surface boundaries, our methodology is susceptible to improvement in detecting flux possessing orthogonal components to axial planes (z-directional). Correlation statistics utilized assume a degree of linearity to potential relationships. The true format of such relationships is not logically precluded from partial non-linear behavior and may not be perfectly represented via our chosen models.
Clinical translation
Throughout history, a consistent de-escalation to the surgical treatment of breast cancer is witnessed (31). Extensive national phase III trials have linked less aggressive operations to improved patient outcomes (32-34). Most currently, the ACOSOG Z0011 trial showed no survival difference in excluding certain groups of patients with positive sentinel nodes from undergoing an axillary lymph node dissection (35). As such, a lack of information pertaining to patients’ nodal disease may frequently be experienced in clinical cases. A potential conflict arises in light of the recently published results of the NCIC MA20 and EORTC 22922/10925 trials. These trials convergently demonstrated significant benefit in terms of decreased breast cancer recurrence rates, albeit sustaining the cost of additional adverse effects, with regional nodal irradiation (RNI) (23,24). This RNI was comprehensively administered to axillary, internal mammary, and supraclavicular lymph nodes. The MA20 study criteria accrued patients with confirmed nodal positivity or high nodal risk and mandated axillary lymph node dissection in all recruits (24). This generates an innate incongruence between the requirements and outcomes of these trails. A patient with inadequate lymph node sampling per Z11 may carry ambiguity in determining whether he/she would benefit from RNI per MA20. Complementary information provided via non-invasive radiogenomic methodologies may aid in optimally stratifying nodal-risk in lieu of pathological assessment. Personalized clinical decisions for administering RNI would thus be enhanced in accounting for results pertaining to axillary nodal status utilizing the 2D heterogeneity flux in early stage breast cancers.
Conclusions
In conclusion, our work introduces the quantity of tumor heterogeneity flux, a novel radiological variable capable of characterizing tumor environment. Being a static measure of intensity gradient emanating from the structural surface, such flux may correlate with a worse prognosis as manifested by axillary nodal involvement in early stage breast cancer. This correlation is optimally displayed in patients with nodal positivity. Our approach can be incorporated into future prognostic indices (nomograms) aimed at helping clinicians with axillary risk assessment.
Acknowledgments
Funding: This work was supported by the Radiological Society of North American Medical Student Grant #RMS1628.
Footnote
Conflicts of Interest: All authors have completed the ICMJE uniform disclosure form (available at http://dx.doi.org/10.21037/tcr.2017.01.02). RS serves as an unpaid editorial board member of Translational Cancer Research from Sep 2016 to Aug 2018. The other authors have no conflicts of interest to declare.
Ethical Statement: The authors are accountable for all aspects of the work in ensuring that questions related to the accuracy or integrity of any part of the work are appropriately investigated and resolved. The authors declare that the methodology used in the ascertainment and analysis of data in this manuscript complies with the current laws in the United States. All procedures performed in studies involving human participants were in accordance with the ethical standards of the institutional and/or nation research committee and with the 1964 Helsinki declaration and its later amendments or comparable ethical standards. For this type of study formal consent is not required. Informed consent was obtained from all individual participants included in the study.
Open Access Statement: This is an Open Access article distributed in accordance with the Creative Commons Attribution-NonCommercial-NoDerivs 4.0 International License (CC BY-NC-ND 4.0), which permits the non-commercial replication and distribution of the article with the strict proviso that no changes or edits are made and the original work is properly cited (including links to both the formal publication through the relevant DOI and the license). See: https://creativecommons.org/licenses/by-nc-nd/4.0/.
References
- Shah SP, Roth A, Goya R, et al. The clonal and mutational evolution spectrum of primary triple-negative breast cancers. Nature 2012;486:395-9. [PubMed]
- Tabassum DP, Polyak K. Tumorigenesis: it takes a village. Nat Rev Cancer 2015;15:473-83. [Crossref] [PubMed]
- Ding L, Ellis MJ, Li S, et al. Genome remodelling in a basal-like breast cancer metastasis and xenograft. Nature 2010;464:999-1005. [Crossref] [PubMed]
- Fujii H, Szumel R, Marsh C, et al. Genetic progression, histological grade, and allelic loss in ductal carcinoma in situ of the breast. Cancer Res 1996;56:5260-5. [PubMed]
- Lambin P, Rios-Velazquez E, Leijenaar R, et al. Radiomics: extracting more information from medical images using advanced feature analysis. Eur J Cancer 2012;48:441-6. [Crossref] [PubMed]
- Lloyd MC, Cunningham JJ, Bui MM, et al. Darwinian Dynamics of Intratumoral Heterogeneity: Not Solely Random Mutations but Also Variable Environmental Selection Forces. Cancer Res 2016;76:3136-44. [Crossref] [PubMed]
- Koren S, Bentires-Alj M. Breast Tumor Heterogeneity: Source of Fitness, Hurdle for Therapy. Mol Cell 2015;60:537-46. [Crossref] [PubMed]
- Lynch HT, Lynch JF. Breast cancer genetics: family history, heterogeneity, molecular genetic diagnosis, and genetic counselling. Curr Probl Cancer 1996;20:329-65. [Crossref] [PubMed]
- Gillies RJ, Kinahan PE, Hricak H. Radiomics: Images Are More than Pictures, They Are Data. Radiology 2016;278:563-77. [Crossref] [PubMed]
- Leijenaar RT, Nalbantov G, Carvalho S, et al. The effect of SUV discretization in quantitative FDG-PET Radiomics: the need for standardized methodology in tumor texture analysis. Sci Rep 2015;5:11075. [Crossref] [PubMed]
- Wang J, Kato F, Oyama-Manabe N, et al. Identifying Triple-Negative Breast Cancer Using Background Parenchymal Enhancement Heterogeneity on Dynamic Contrast-Enhanced MRI: A Pilot Radiomics Study. PLoS One 2015;10:e0143308 [Crossref] [PubMed]
- Castellano G, Bonilha L, Li LM, et al. Texture analysis of medical images. Clin Radiol 2004;59:1061-9. [Crossref] [PubMed]
- Chicklore S, Goh V, Siddique M, et al. Quantifying tumour heterogeneity in 18F-FDG PET/CT imaging by texture analysis. Eur J Nucl Med Mol Imaging 2013;40:133-40. [Crossref] [PubMed]
- Barrett T, Brechbiel M, Bernardo M, et al. MRI of tumor angiogenesis. J Magn Reson Imaging 2007;26:235-49. [Crossref] [PubMed]
- Ocak I, Baluk P, Barrett T, et al. The biologic basis of in vivo angiogenesis imaging. Front Biosci 2007;12:3601-16. [Crossref] [PubMed]
- Consolino L, Longo DL, Dastrù W, et al. Functional imaging of the angiogenic switch in a transgenic mouse model of human breast cancer by dynamic contrast enhanced magnetic resonance imaging. Int J Cancer 2016;139:404-13. [Crossref] [PubMed]
- Li L, Wang K, Sun X, et al. Parameters of dynamic contrast-enhanced MRI as imaging markers for angiogenesis and proliferation in human breast cancer. Med Sci Monit 2015;21:376-82. [Crossref] [PubMed]
- Vignati A, Giannini V, Bert A, et al. A fully automatic multiscale 3-dimensional Hessian-based algorithm for vessel detection in breast DCE-MRI. Invest Radiol 2012;47:705-10. [Crossref] [PubMed]
- Tafreshi NK, Gillies RJ, Morse DL. Molecular imaging of breast cancer lymph node metastasis. Eur J Radiol 2012;81:S160-1. [Crossref] [PubMed]
- Orlhac F, Soussan M, Maisonobe JA, et al. Tumor texture analysis in 18F-FDG PET: relationships between texture parameters, histogram indices, standardized uptake values, metabolic volumes, and total lesion glycolysis. J Nucl Med 2014;55:414-22. [Crossref] [PubMed]
- Tixier F, Le Rest CC, Hatt M, et al. Intratumor heterogeneity characterized by textural features on baseline 18F-FDG PET images predicts response to concomitant radiochemotherapy in esophageal cancer. J Nucl Med 2011;52:369-78. [Crossref] [PubMed]
- Hughes SW, D’Arcy TJ, Maxwell DJ, et al. Application of a new discreet form of Gauss' theorem for measuring volume. Phys Med Biol 1996;41:1809-21. [Crossref] [PubMed]
- Poortmans PM, Collette S, Kirkove C, et al. Internal Mammary and Medial Supraclavicular Irradiation in Breast Cancer. N Engl J Med 2015;373:317-27. [Crossref] [PubMed]
- Whelan TJ, Olivotto IA, Parulekar WR, et al. Regional Nodal Irradiation in Early-Stage Breast Cancer. N Engl J Med 2015;373:307-16. [Crossref] [PubMed]
- Abramson RG, Lambert KF, Jones-Jackson LB, et al. Prone Versus Supine Breast FDG-PET/CT for Assessing Locoregional Disease Distribution in Locally Advanced Breast Cancer. Acad Radiol 2015;22:853-9. [Crossref] [PubMed]
- Banerjee SM, Williams NR, Davidson TI, et al. The use of onestep nucleic acid amplification (OSNA) and tumour related factors in the treatment of axillary breast cancer: A predictive model. Eur J Surg Oncol 2016;42:641-9. [Crossref] [PubMed]
- Roy PG, Chan SM, Ng V, et al. Risk stratification of patients with early breast cancer. Clin Breast Cancer 2014;14:68-73. [Crossref] [PubMed]
- Obeid JP, Stoyanova R, Kwon D, et al. Multiparametric evaluation of preoperative MRI in early stage breast cancer: prognostic impact of peri-tumoral fat. Clin Transl Oncol 2017;19:211-8. [Crossref] [PubMed]
- Kuhl CK, Schild HH, Morakkabati N. Dynamic bilateral contrast-enhanced MR imaging of the breast: trade-off between spatial and temporal resolution. Radiology 2005;236:789-800. [Crossref] [PubMed]
- Edge SB, Compton CC. The American Joint Committee on Cancer: the 7th edition of the AJCC cancer staging manual and the future of TNM. Ann Surg Oncol 2010;17:1471-4.
- Franceschini G, Martin Sanchez A, Di Leone A, et al. New trends in breast cancer surgery: a therapeutic approach increasingly efficacy and respectful of the patient. G Chir 2015;36:145-52. [PubMed]
- Ashikaga T, Krag DN, Land SR, et al. Morbidity results from the NSABP B-32 trial comparing sentinel lymph node dissection versus axillary dissection. J Surg Oncol 2010;102:111-8. [Crossref] [PubMed]
- Fisher B, Wolmark N, Redmond C, et al. Findings from NSABP Protocol No. B-04: comparison of radical mastectomy with alternative treatments. II. The clinical and biologic significance of medial-central breast cancers. Cancer 1981;48:1863-72. [Crossref] [PubMed]
- Krag DN, Anderson SJ, Julian TB, et al. Sentinel-lymph-node resection compared with conventional axillary-lymph-node dissection in clinically node-negative patients with breast cancer: overall survival findings from the NSABP B-32 randomised phase 3 trial. Lancet Oncol 2010;11:927-33. [Crossref] [PubMed]
- Giuliano AE, McCall L, Beitsch P, et al. Locoregional recurrence after sentinel lymph node dissection with or without axillary dissection in patients with sentinel lymph node metastases: the American College of Surgeons Oncology Group Z0011 randomized trial. Ann Surg 2010;252:426-32; discussion 432-3. [PubMed]