Co-expression analysis reveals key gene modules of bone metastases
Introduction
Bone metastases are very common in some advanced cancers especially in patients with lung, prostate or breast cancer (1). And it was reported that approximately 50–75% of patients suffered from bone metastases (2). Bone metastases could cause significant complications including spinal cord compression, hypercalcemia, pathologic fractures and one of the most symptoms was pain, which seriously affected the patients in their activities of daily living and decreased their quality of life (3-6). Although great improvements have been made in the systemic therapies, yet how to better understand the pathogenesis of bone metastases and find novel and effective target therapies was the top priority.
In the past few years, a number of studies were focused on the improvement of quality of life of the patients with painful bone metastases after radiotherapy (1,7). Although great improvements had been made in the systemic therapies of bone metastases and the patients obtained longer survival, yet the existing researches were lacking of gene co-expression analysis for bone metastases, which may limit our cognition of the key genes that affect the pathogenesis of bone metastases. In recent years, the co-expression gene module method has been extensively applied in the research of various diseases. While one of the most common used methods for co-expression gene module was WGCNA (weighted correlation network analysis) (8). WGCNA was usually used to find modules or clusters of highly correlated genes, or to relate one module to another and to external sample traits (by eigengene network method) (9,10). Through WGCNA, the gene screening methods based on network could be utilized to find new therapeutic targets and candidate biomarkers. WGCNA could identify the gene modules that were clinically interesting from the level of multiple of genes, and then found suitable targets through analyzing module membership (high intramodular connectivity) and other criteria including gene ontology and so on. It was reported that WGCNA had been successfully exploited in brain cancer (11), diabetes (12), chronic fatigue patients (13) and other biological contexts (14,15) to analyze their gene expression data. The data above may indicate that WGCNA could be a useful method for bone metastases study.
In the study, we aimed to construct the co-expression gene modules combining with the existing bone metastases expression data, and perform functional annotation analysis of the main modules, so as to explore the functions of the genes contained in these modules and find effective prevention and treatment methods for bone metastases.
Methods
Gene expression analysis of the bone metastases chip sample
We applied “bone metastases” as a keyword to retrieve in GEO (Gene Expression Omnibus) database (https://www.ncbi.nlm.nih.gov/geo/) in NCBI, and the probe signal values from relevant literature were extracted. The gene information was mapped to the probe using the recorded chip annotation information. The results of one probe corresponding to multiple of genes were removed, and the average gene expression of the genes corresponding to more than one probe was calculated. The gene numbers corresponding to the expression thresholds of various genes were also counted, so as to determine the appropriate threshold. WGCNA algorithm (8) was applied to evaluate gene expression, and the cluster analysis of samples under an appropriate threshold was performed by the flashClust toolkit.
Analysis of the construct of bone metastases co-expression gene modules
Firstly the power values in the process of module building were screened using the WGCNA algorithm, and the scale independent degree and average connection degree of the modules corresponding to different power values were detected by a gradient test. The appropriate power value was determined when the scale independent degree was 0.8. The module was further to be constructed and the gene information corresponding to each module was extracted using the WGCNA algorithm under this power value.
Correlation analysis between co-expression modules of bone metastases genes
The interaction relationship in R software was analyzed through R software using the WGCNA algorithm, while the strength of the relationship was presented in a heatmap draw by Heatmap toolkit in R software.
Functional annotation analysis of the genes in bone metastases co-expression module
The constructed modules were sorted according to the gene numbers, and the genes in the top five modules were chose to do functional analysis. During this process, the gene information corresponding to modules was mapped to DAVID database (https://david.ncifcrf.gov/summary.jsp) (16), and the analysis results of the biological processes in Gene Ontology (GO) (17) analysis and the functional enrichment analysis results of KEGG (18) in gene metabolic pathways analysis were extracted respectively. The analysis condition was the corrected P value (namely Benjamini value) less than 0.05. If the recorded number was more than five, then only the top five records were selected for analysis.
Results
The bone metastases chip data collection and its gene expression analysis
The latest bone metastases chip expression data (GSE74685) (19) with large amount of data was found in NCBI database using bone metastases as a keyword, from which 20 samples were found to possess the typical characteristics of bone metastases. Firstly the chip information was transformed into gene expression information according to the previously reported values of the chip probes. On the one hand the values that a single probe corresponding to multiple of genes was left out, on the other hand the mean of the values that one gene corresponding to multiple probes was calculated and regarded as the expression level of this gene. Then a total of 19,612 gene expression was obtained. The average expression distribution of these genes in 20 samples was further counted (Figure 1A). We found the lowest expression of these genes was 5.82, while the highest expression was 18.06. As shown in Figure 1A, for different gene number there was a different gene expression level. Considering the restriction of gene number to construct the module, the genes with high average expression levels were screened, and a total of 6,922 genes were screened out with 11 as the threshold of gene expression level, which accounted for about 35.3% of the total genes.
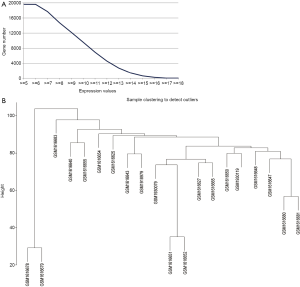
The expression levels of these 6,922 genes in the 20 bone metastases samples were further extracted, and the sample cluster analysis was performed through flashClust toolkit by the WGCNA algorithm. The results showed the 20 samples were mainly divided into two clusters, among which GSM1616678 and GSM1616679 were clustered into a cluster, while the other 18 samples were clustered into another one (Figure 1B).
Construction of bone metastases gene co-expression module
The bone metastases gene co-expression module was constructed based on the expression levels of these 6,922 genes in 20 samples by WGCNA toolkit. The power value was a very key parameter in this process, which mainly affected the scale independent degree and average connection degree of gene co-expression module. First of all, we screened the power value. The scale independent degree and average connection degree were relatively balanced when the single power value was 6 (Figure 2).
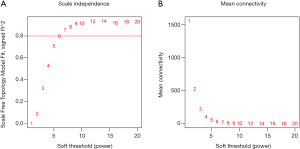
The co-expression modules (Figure 3) of these 6,922 genes in 20 bone metastases samples were further constructed using the selected power value (6), finally 15 gene co-expression modules were successfully constructed, and they were showed in different colors. The modules were numbered after sorting by gene number, the module with the largest number of genes contained 1,336 genes (turquoise), while the module with the least number of genes contained only 8 genes (gray).
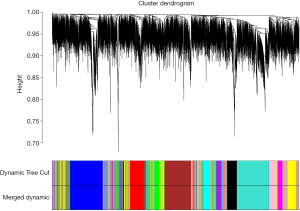
Analysis of the relations between the gene co-expression modules
We further analyzed the relations between these 15 gene co-expression modules (Figure 4), from which, we could see there were significant differences between different modules.
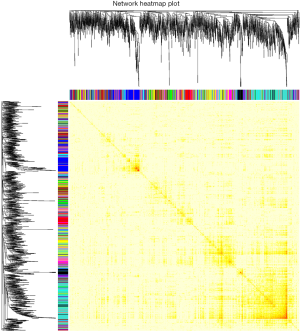
The interaction relationships between modules were further subjected to do clustering analysis and connection degree analysis of the key genes (Figure 5), so as to understand the relationship between the gene co-expression modules constructed in this study. From Figure 5A, these 15 modules could be divided into two large clusters, and one included ten modules, which were gray, black, red, turquoise and pink clusters; the other ten modules was another cluster, this cluster could be further divided into two sub-clusters. Similarly, the heat map (Figure 5B) of the connection degrees of module key genes also confirmed the results.

Functional annotation enrichment analysis of the genes in key modules
The top five modules with the largest number of genes were chose to do gene function analysis within the module. And it achieved mainly through the detection of gene GO enrichment and KEGG enrichment results. Significant differences were existed between GO enrichment analysis of genes in the module (Table 1), the genes were mainly enriched in nucleosome and chromatin assembly in module 1 while the genes in module 2 were mainly enriched in bone vessel development, cell adhesion biological processes and so on; the genes in module 3 were mainly enriched in mRNA cut and process and the genes in module 4 were mainly enriched in large molecules catabolism; finally the genes in module 5 were mainly enriched in translation and ribonucleoprotein synthesis process.
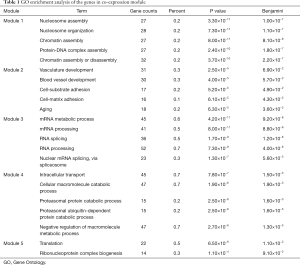
Full table
The KEGG enrichment results of the genes in each top five module were further tested (Table 2), which showed that the genes in module 1 were enriched in processes of amino acid metabolism, sugar metabolism and RNA degradation; the genes in module 2 were enriched in metabolic pathways of cell adhesion, ECM receptor response and cell adhesion molecules and others, these pathways had been widely reported in previous disease study; the genes in module 3 were enriched in spliceosome reaction pathway; the genes in module 4 were enriched in proteasome reaction pathway; and module 5 was also enriched in spliceosome reaction pathway.
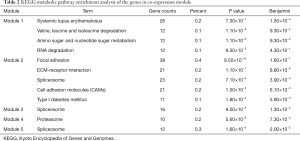
Full table
Based on the GO enrichment and KEGG enrichment results, we could speculate that module 2 was the most critical module during the process of bone metastases.
Discussion
In our study, we constructed the bone metastases gene co-expression modules and identified the key modules and the contained genes through functional analysis of the genes in these modules using a systematic gene approach WGCNA.
WGCNA could avoid the fussy testing problems in different microarray data analysis as it mainly focused on modules analysis rather than individual gene expression. And it focused on the interactions between various modules or traits. As modules were corresponding to different biological pathways, so WGCNA may screen the modules that were related to a certain disease (15). Comparing to other network analysis tools such as gene network enrichment analysis (20), functional analysis of gene co-expression networks (21) or general network structures in Bioconductor (22), WGCNA complemented their deficiencies as most of them pay attention to unweighted networks while WGCNA could be used for both unweighted and weighted correlation networks. Furthermore, WGCNA may be considered as a gene screening tool or a data exploratory method.
From Figure 4 we found that the interactions between these constructed gene co-expression modules were significantly different. In order to illustrate the differences, we then performed clustering analysis and connection degree analysis of the key genes in these modules. The results showed that these modules were different in their functions. Among which, the GO enrichment analysis showed the top five modules were associated with nucleosome and chromatin assembly; bone vessel development, cell adhesion biological processes and so on; mRNA cut and process; large molecules catabolism and translation and ribonucleoprotein synthesis process respectively. And KEGG enrichment analysis further affirmed why the gene co-expression modules were different with each other. Among them module 2 was mainly enriched in metabolic pathways of cell adhesion, ECM receptor response and cell adhesion molecules and others, which had been widely reported in previous studies. Therefore we speculated that module 2 may play key roles during the pathogenesis of bone metastases.
As we know, the key process for any metastatic invasion like bone metastases was the process that could induce a vascular network. While cell adhesion molecules were the core to the invasive process, as cell adhesion molecules had ability to function as a molecular link between cells and cells or extracellular matrix (ECM) (23). In addition, the previous studies affirmed that cell adhesion molecule receptors could affect cell migration and other signal transduction pathways which regulated cell functions including proliferation, differentiation, receptor activation and so on (24). Besides, ECM (consisting of non-cellular component of tissues and structural and functional proteins) (25) was also crucial to the metastatic process, for it was essential for the cancer cells to degrade the ECM molecules (26). Degradation of ECM could facilitate tumor cells to spread and may also contribute to the biological effects of tumor cells. Recently, a number of studies were focused on the study of ECM and ECM receptor in order to explore potential targets for the therapy of various diseases. And many ECM degrading enzymes were repeatedly stressed in tumor invasion and metastasis. From the GO and KEGG enrichment analysis results, we knew that the key genes in module 2 were all enriched in cell adhesion biological processes and ECM receptor response, considering the important roles of cell adhesion and ECM, we may better understand the mechanisms of bone metastases.
To conclude, the bone metastases co-expression gene modules were constructed in the study in order to explore the functions of the genes, finally we identified module 2 as the key module and from which we may further find potential biomarkers or targets for the prevention and treatment of bone metastases.
Acknowledgments
Funding: None.
Footnote
Conflicts of Interest: All authors have completed the ICMJE uniform disclosure form (available at http://dx.doi.org/10.21037/tcr.2017.05.07). The authors have no conflicts of interest to declare.
Ethical Statement: The authors are accountable for all aspects of the work in ensuring that questions related to the accuracy or integrity of any part of the work are appropriately investigated and resolved. The study was conducted in accordance with the Declaration of Helsinki (as revised in 2013). Institutional ethical approval and informed consent were waived.
Open Access Statement: This is an Open Access article distributed in accordance with the Creative Commons Attribution-NonCommercial-NoDerivs 4.0 International License (CC BY-NC-ND 4.0), which permits the non-commercial replication and distribution of the article with the strict proviso that no changes or edits are made and the original work is properly cited (including links to both the formal publication through the relevant DOI and the license). See: https://creativecommons.org/licenses/by-nc-nd/4.0/.
References
- McDonald R, Chow E, Rowbottom L, et al. Quality of life after palliative radiotherapy in bone metastases: A literature review. J Bone Oncol 2014;4:24-31. [Crossref] [PubMed]
- Nguyen J, Chow E, Zeng L, et al. Palliative response and functional interference outcomes using the Brief Pain Inventory for spinal bony metastases treated with conventional radiotherapy. Clin Oncol (R Coll Radiol) 2011;23:485-91. [Crossref] [PubMed]
- Chow E, James J, Barsevick A, et al. Functional interference clusters in cancer patients with bone metastases: a secondary analysis of RTOG 9714. Int J Radiat Oncol Biol Phys 2010;76:1507-11. [Crossref] [PubMed]
- Caissie A, Zeng L, Nguyen J, et al. Assessment of health-related quality of Life with the European Organization for Research and Treatment of Cancer QLQ-C15-PAL after palliative radiotherapy of bone metastases. Clin Oncol (R Coll Radiol) 2012;24:125-33. [Crossref] [PubMed]
- Mantyh P. Bone cancer pain: causes, consequences, and therapeutic opportunities. Pain 2013;154:S54-62. [Crossref] [PubMed]
- Pituskin E, Fairchild A, Dutka J, et al. Multidisciplinary team contributions within a dedicated outpatient palliative radiotherapy clinic: a prospective descriptive study. Int J Radiat Oncol Biol Phys 2010;78:527-32. [Crossref] [PubMed]
- Raman S, Ding K, Chow E, et al. A prospective study validating the EORTC QLQ-BM22 bone metastases module in patients with painful bone metastases undergoing palliative radiotherapy. Radiother Oncol 2016;119:208-12. [Crossref] [PubMed]
- Langfelder P, Horvath S. WGCNA: an R package for weighted correlation network analysis. BMC Bioinformatics 2008;9:559. [Crossref] [PubMed]
- Stuart JM, Segal E, Koller D, et al. A gene-coexpression network for global discovery of conserved genetic modules. Science 2003;302:249-55. [Crossref] [PubMed]
- Zhang B, Horvath S. A general framework for weighted gene co-expression network analysis. Stat Appl Genet Mol Biol 2005;4:Article17.
- Horvath S, Zhang B, Carlson M, et al. Analysis of oncogenic signaling networks in glioblastoma identifies ASPM as a molecular target. Proc Natl Acad Sci U S A 2006;103:17402-7. [Crossref] [PubMed]
- Keller MP, Choi Y, Wang P, et al. A gene expression network model of type 2 diabetes links cell cycle regulation in islets with diabetes susceptibility. Genome Res 2008;18:706-16. [Crossref] [PubMed]
- Presson AP, Sobel EM, Papp JC, et al. Integrated weighted gene co-expression network analysis with an application to chronic fatigue syndrome. BMC Syst Biol 2008;2:95. [Crossref] [PubMed]
- Oldham MC, Horvath S, Geschwind DH. Conservation and evolution of gene coexpression networks in human and chimpanzee brains. Proc Natl Acad Sci U S A 2006;103:17973-8. [Crossref] [PubMed]
- Fuller TF, Ghazalpour A, Aten JE, et al. Weighted gene coexpression network analysis strategies applied to mouse weight. Mamm Genome 2007;18:463-72. [Crossref] [PubMed]
- Delker DA, Geter DR, Roop BC, et al. Oncogene expression profiles in K6/ODC mouse skin and papillomas following a chronic exposure to monomethylarsonous acid. J Biochem Mol Toxicol 2009;23:406-18. [Crossref] [PubMed]
- Ashburner M, Ball CA, Blake JA, et al. Gene ontology: tool for the unification of biology. Nat Genet 2000;25:25-9. [Crossref] [PubMed]
- Du J, Yuan Z, Ma Z, et al. KEGG-PATH: kyoto encyclopedia of genes and genomes-based pathway analysis using a path analysis model. Mol Biosyst 2014;10:2441-7. [Crossref] [PubMed]
- Haider M, Zhang X, Coleman I, et al. Epithelial mesenchymal-like transition occurs in a subset of cells in castration resistant prostate cancer bone metastases. Clin Exp Metastasis 2016;33:239-48. [Crossref] [PubMed]
- Liu M, Liberzon A, Kong SW, et al. Network-based analysis of affected biological processes in type 2 diabetes models. PLoS Genet 2007;3:e96 [Crossref] [PubMed]
- Henegar C, Clément K, Zucker JD. Unsupervised Multiple-Instance Learning for Functional Profiling of Genomic Data. In Machine Learning: Ecml 2006, European Conference on Machine Learning, Berlin, Germany, September 18-22, 2006, Proceedings, 2006.
- Carey VJ, Gentry J, Whalen E, et al. Network structures and algorithms in Bioconductor. Bioinformatics 2005;21:135-6. [Crossref] [PubMed]
- Anderson AR. A hybrid mathematical model of solid tumour invasion: the importance of cell adhesion. Math Med Biol 2005;22:163-86. [Crossref] [PubMed]
- Clark EA, Brugge JS. Integrins and signal transduction pathways: the road taken. Science 1995;268:233-9. [Crossref] [PubMed]
- Ravindran S, George A. Multifunctional ECM proteins in bone and teeth. Exp Cell Res 2014;325:148-54. [Crossref] [PubMed]
- Lawrence JA, Steeg PS. Mechanisms of tumor invasion and metastasis. World J Urol 1996;14:124-30. [Crossref] [PubMed]