Reproducibility of radiomic features with GrowCut and GraphCut semiautomatic tumor segmentation in hepatocellular carcinoma
Introduction
Hepatocellular carcinoma (HCC), the sixth most common cancer worldwide, is the main type of cancer in liver parenchymal cells (1). Medical imaging techniques, such as computed tomography (CT), magnetic resonance imaging (MRI), positron emission CT (PET-CT), play important roles in oncology. Especially in radiotherapy, imaging dominates treatment planning and response monitoring (2). Several publications have shown that quantitative image features have potential applications in providing consistent, nonbiased descriptors to the tumor research.
For the past several years, as an emerging individualized precision medical technology, radiomics has applied advanced computational methodologies to transform the image data of the regions of interest into high dimensional feature data. Next, quantitative and high-throughput analysis of feature data is completed to probe tumor phenotype (3-6). Radiomics utilizes noninvasive imaging to provide more comprehensive information about the entire tumor and can be used in diagnosis, prognosis and prediction (6,7). For patients with colorectal liver metastases, relative differences of CT textural features occurring after treatment were better than RECIST in predicting and assessing the pathological response to chemotherapy (8). Another, more recent HCC radiomics study showed that CT-based radiomics signature was a powerful predictor for preoperative estimation of early recurrence (9).
The radiomics features must be reproducibility, non-redundancy and informative (10). Reproducibility, the most basic and essential problem in radiomics, refers to measurements of radiomic features performed using different equipment, different methods or observers, or at different sites and times (10,11). The reproducibility may be influenced by many factors, such as imaging devices (12), repeat CT scans (13-15), tumor volume definition (16-18) and feature extraction (19,20). For acquiring accurate results, the producible features should be selected in building prognostic or predictive models.
Tumor segmentation is crucial for subsequent quantitative imaging extraction. Although manual delineation by experts is a common method considered as a ‘gold standard’, it is time-consuming and suffers from inter-observer variability. Recent studies have shown that 3D Slicer semiautomatic segmentation results were almost consistent with the manual contour by expert (21-23). 3D Slicer (23) is a free and open-source software package for medical image analysis in which many extensions are available for tumor segmentation on CT images. Since liver tumors have indistinct borders, there is high variability in radiologists’ determination of tumor outlines, leading to increased variation in features extraction (17). However, to the best of our knowledge, few studies have investigated the stability of radiomic features extracted from tumor regions defined by different semiautomatic methods.
In this study, we evaluate the reproducibility of quantitative imaging features derived from tumor volume segmented using GraphCut and GrowCut interactive methods in 3D Slicer and to determine robustness of feature categories to propel clinical radiomics research of HCC patients.
Methods
CT imaging data of HCC patients
The CT imaging data set of 15 patients who have been diagnosed with primary HCC between December 2015 and May 2016 were randomly collected. All patients received abdominal enhanced CT scanning, and a Philips scanner (Holland, CT Lightspeed 16) was used with an imaging protocol of tube voltage 120 kV, cube current 300 mA, thickness 3 mm and in-plane resolution 0.97×0.97. Each patient has only one lesion (the volume range of tumor is 5–168 cm3, median 19 cm3). Arterial CT images were included in this study. This study was approved by the institutional review board (IRB) and ethics committee of Shandong Cancer Hospital Affiliated to Shandong University. The ID/number of ethics approval was 201606021.
Semiautomatic tumor segmentation
3D Slicer is an open-source, publicly available image analysis platform and was developed for segmentation, registration and three-dimensional visualization. In this study, GraphCut (24) and GrowCut (25) were implemented in 3D Slicer and tumor volume was defined twice by each of the two independent observers to determine intra-observer reproducibility. The run1 and run2 were first and second segmentations among different observers to assess inter-observer reproducibility.
GraphCut semiautomatic segmentation
The knowledge based star shape prior was incorporated in the graph cut algorithm in the 3D Slicer GraphCut extension. Star shape prior is a generic shape prior that applies to a wide class of objects to achieve more robust segmentation. Graph cut turns the image segmentation into discrete graph optimization (min cut/max flow). First, this method builds an energy function after mapping the images to undirected weighed graphs; meanwhile, voxels in the images are treated as graph nodes. Next, the similarity of nodes in graph is calculated as the weight of connections between nodes. Finally, the min cut and energy function minimized strategy is employed to obtain the optimal segmentations.
Before GraphCut was activated in 3D Slicer, operators need to add four fiducials around the tumor after loading images. Two fiducials on the first slice and last slice where the tumor begins and ends to show to identify the start-end of the tumor and two fiducials on the middle slice where the tumor area is the largest at the diagonal corners of a rectangle, which can contain the tumor. Thereafter, 2D or 3D star shape constraints can be checked as needed.
GrowCut semiautomatic segmentation
GrowCut has better performance on accuracy and speed in tumor segmentation by using a competitive region growing algorithm (22,24). A set of initial labels needs to be given by users to mark foreground and background, and cellular automata automatically segments the remaining image using a weighted similarity score. The neighbor that results in the largest weight greater than the given voxel’s strength confers its label to the given voxel. If there are two or more tumors in the image to be segmented, the corresponding class of initial labels was needed.
The GrowCut is executed as follows: first, it defines the tumor and non-tumor region with different label value; next, algorithm automatically computes a region of interest. After that step, GrowCut was activated to label iteratively all of the voxels in the ROI until all the voxels are labeled or until no voxel can change its label any more.
If not satisfy with the result, the foreground tumor region can be edited manually both in GraphCut and GrowCut.
Manual tumor delineation
Five experienced radiologists manually defined the gross tumor volume (GTV) of primary HCC in MIM software (www.mimsoftware.com) twice per radiologist using standard delineation protocol (window width: 200 HU, window level: 40 HU). The radiologists were blind to one another.
Quantitative imaging feature extraction
Seventy-one quantitative imaging features were extracted from the information contained in the voxels of the tumor region segmented by the three strategies. This process was implemented in IBEX (Imaging Biomarker Explorer, MD Anderson cancer center, USA), an open-source, easy to use radiomic software (26). These features were organized into three categories: (I) intensity histogram; (II) texture; and (III) shape. Seventeen first-order statistical features derived from the tumor intensity histogram reflect distribution of values of individual voxels without concern for spatial relationships. Thirty-eight textural features describe spatial arrangement of voxels were calculated from different parent matrices, including gray-level co-occurrence matrix (GLCM), gray-level run-length matrix (GLRLM), neighbor gray-tone difference matrix (NGTDM). Sixteen shape based features provide the geometrical of tumor volume. To reduce the effect of noise on the textual features, all of the voxel intensity values within the ROI were rescaled to 8-bit images using a discrete resampling method before calculating the GLCM, GLRLM, and NGTDM features (27). In this study, GLCM features were the average of all 13 symmetric directions in 3D, GLRLM features were the average of values calculated from 2 directions in the 2D slice-by-slice, and the NGTDM was defined by neighborhood in the 3D.
Statistical analysis
In this study, intra-class correlation coefficient (ICC) as defined by McGraw and Wong (28) was employed to assess the reproducibility of radiomic features derived from tumor volume segmented by three methods. ICC is a descriptive statistic between 0 and 1, where 0 and 1 indicate null and perfect reproducibility, respectively. A variety of algorithms were provided in the literature for the ICC calculation. To assess the reproducibility of radiomic features extracted from inter-observer segmentations, we used the definition of ICC(A,1), and variance estimates were obtained from two-way mixed effect model of analysis of variance (ANOVA), given by:
Additionally, we used the definition of ICC(C, 1) to assess the reproducibility of radiomic features derived from intra-observer segmentations, variance estimates were obtained from one-way analysis of variance (ANOVA), with the following form:
where MSR = mean square for rows (observations, fixed factor), MSW = mean square for residual sources of variance, MSE = mean square error, MSC= mean square for columns (observers, random factor), k and n represent number of observers and number of subjects, respectively. In order to help readers to better understand the model, we provide a table of measurements of GLCM-Contrast from all segmentations as one example. It can be found in Table S1.
To compare the differences of feature range between manual and two semi-automatic segmentations, Z-score normalization was applied to standardize the radiomic features because different features would have various ranges. The Z-score normalization was defined as follows:
where μ and σ were the mean value and standard deviation of radiomic features, respectively.
Wilcoxon rank-sum tests were used to compare the differences of ICC between manual delineation and two semiautomatic methods. P<0.05 was considered to be significant. All data were expressed as the mean ± SD. SPSS version 22.0 (SPSS, Chicago, IL, USA) was used for ICC and Wilcoxon rank-sum test computation.
Results
The ICC values of the 71 quantitative imaging features across tumors segmented by three methods was presented in Figure 1. Noticeably, radiomic features derived from GrowCut-based segmentation (ICC =0.87±0.19) had significantly higher ICC values compared to features extracted from GraphCut-based segmentation (ICC =0.82±0.24, P<0.001) and manual delineation (ICC=0.80±0.21, P<0.001). The statistically significant difference was observed in ICC values for features-based GraphCut and manual segmentation (P=0.036). For ICCs of manual, GraphCut and GrowCut methods, the confidence intervals were (0.608, 0.954), (0.774, 0.938) and (0.752, 0.967), respectively. Overall, 53 of the radiomic feature showed higher ICC for GrowCut, and 47 showed higher ICC values for GraphCut segmentation sets compared to the manual method (P<0.001). Also, comparing GrowCut to GraphCut, 52 features with higher ICC values were extracted from GrowCut segmentations.
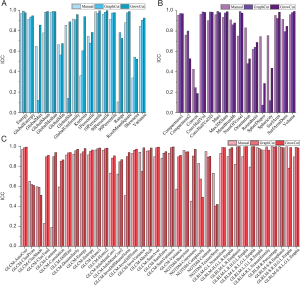
For tumor intensity histogram features, no statistically significant change was observed in GraphCut segmentation sets (ICC =0.77±0.29) compared to the manual method (ICC =0.76±0.26) (P=0.332), but GrowCut (ICC =0.90±0.14) showed significantly higher reproducibility (P<0.001). For GLCM features in the textural category, GraphCut (ICC =0.89±0.11) and GrowCut (ICC =0.91±0.12) has significantly higher reproducibility than the manual method (ICC =0.77±0.23) (P=0.010, P=0.004, respectively). For GLRLM, NGTDM features in textural category and shape-based features, no statistically significant difference was observed between the manual and the two semiautomatic methods. All of the features were divided into four groups according to their ICC values: excellent (0.73≤ ICC ≤1), good (0.6≤ ICC <0.75), fair (0.4≤ ICC <0.6) and poor (ICC <0.4) reproducibility (29). The number of features in each group is presented in Table 1. The excellent reproducibility of radiomic features for manual, GraphCut, and GrowCut segmentations were 73% [52], 77% [55], and 81% [58] of total, respectively. These features can be found in Figure S1.
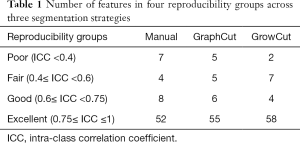
Full table
To evaluate the effect on robustness with multiple algorithmic initializations, we analyzed the ICC of features extracted from inter-observer and intra-observer segmentations. In Figure 2A, we observed higher ICC values in GrowCut inter-observer segmentation groups (average ICC =0.87±0.18). In Figure 2B, higher ICC values were also observed in GrowCut intra-observer segmentations (average ICC =0.90±0.11). There are distinct differences of ICC in GraphCut for both inter- and intra-observer segmentation (P<0.001, P=0.008, respectively). Figure 3 depicts the Z-score normalized feature range of all of the 18 segmentation sets (10 manual, 4 GraphCut and 4 GrowCut). Overall, the range of feature based GrowCut segmentations was smaller than that of GraphCut (P<0.001) and manual (P<0.001). GraphCut showed no significant difference compared to manual method (P=0.062). These data are available in Figure S2.
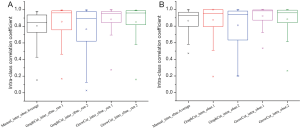
Discussion
Medical imaging is now routinely used and is playing an essential role in clinical oncology. As an emerging field in precision medicine, radiomics utilizes quantitative imaging features to assess the characteristics of tumor phenotype and has potential applicability in treatment planning and monitoring. For example, the changes of radiomic features extracted from post-treatment CT images can serve as early indicators of progression to local recurrence within six months after SABR in early-stage lung cancer (30). In another study, 440 imaging features extracted from CT data of 1,019 patients with lung or head-and-neck cancer can capture intratumor heterogeneity and are associated with gene expression patterns, TNM staging and prognosis of patients (6).
Tumor segmentation is an essential step in the workflow of radiomics. Many semi-automatic and automatic segmentation algorithms have been developed for tumor delineation. Therefore, the GraphCut and GrowCut semiautomatic methods were used in liver tumor segmentation in this study. The detailed workflow of these two semiautomatic segmentation tools can be found in Figure S3. These methods yield more stable segmentation and need less time compared with manual delineation, as manual delineation is time-consuming and prone to higher inter-observer variability. Parmar et al. concluded that the quantitative imaging feature extracted from semiautomatic tumor segmentations showed significantly higher reproducibility than manual delineations (18). However, there were few reports in the literature about the effect on the stability of radiomic features derived from tumors segmented using different semiautomatic algorithms.
In this study, 71 commonly used quantitative imaging features were selected and organized into three categories (17 tumor intensity histogram based features, 38 textural features and 16 shape based features). We analyzed the robustness of these features when they were extracted from tumor regions segmented using three methods. In all 71 radiomic features, GrowCut segmentations showed significantly higher ICC values than GraphCut segmentations and manual delineations (P<0.001). While GraphCut is not as significant as GrowCut, GraphCut had slightly better robustness than manual delineations (P=0.036), indicating that 3D Slicer tumor segmentation tools can extract more reproducible quantitative imaging features. These results can be explained by the fact that semiautomatic tumor segmentation algorithms require no more manual intervention since algorithm initialization was performed, then the tumor was segmented by an efficient algorithm. There is too much underlying uncertainty in the manual tumor delineation because of the inter-observer variability may be accumulated through slice-by-slice manual delineation.
We observed that GLCMs-based features were more robust to semiautomatic tumor segmentations, GrowCut and GraphCut has significantly higher reproducibility compared to manual delineations (P=0.001, P<0.001, respectively). Additionally, the tumor intensity histogram features were more reproducible when they were extracted from GrowCut segmentations. However, there was no significant difference in reproducibility of other feature categories. These results indicate that CT textural features derived from semiautomatic segmentations are highly reproducible for HCC patients.
To evaluate the performance of three segmentation strategies, we analyzed inter- and intra-observer reproducibility. We found that features derived from GrowCut had higher ICC values in both inter- and intra-observer, indicating that it was able to extract more reproducible features against the different algorithm initializations. We also observed that reproducible features extracted from GraphCut-based segmentations were unstable for inter-observer. The feature range that we observed was significantly smaller in GrowCut compared with other two segmentation methods.
Our findings demonstrate that stable and reproducible radiomic features can be extracted from semiautomatic tumor segmentations and that textural features extracted using these segmentation tools are more suitable. However, inter-observer initialization differences result in various segmentations due to different principles of semiautomatic methods. We can apply these methods that were evaluated in radiomics studies to yield reproducible results. In this study, tumor segmentation based on GrowCut presented great performance on the feature extraction both inter- and intra-observer.
A limitation of this study is that although clinical data for these patients are available, the small patient cohort prevented prediction/prognosis models from being devised. The conclusions drawn from this study should be applicable to predict outcomes in HCC patients. This work will be done when we have collected large prospective patient cohorts. Another limitation is that tumor size could also be an important factor related to feature reproducibility and prognostic value. These volume-dependent features will be involved in future research. Furthermore, many software packages are available for use in radiomics research (31). The increased usage of these computational resources will bridge the gaps between radiomics and clinical oncology.
Conclusions
Our study reveals that variations exist in the reproducibility of quantitative imaging features extracted from tumor region segmented using different methods. For HCC radiomics studies, tumor intensity histogram-based features and textural features were more reproducible when they were extracted from GrowCut semiautomatic segmentations. Therefore, 3D Slicer can serve as a better alternative to the manual delineation method, and care must be taken when selecting segmentation tools to draw tumor regions.
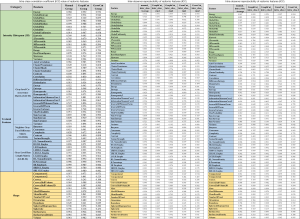
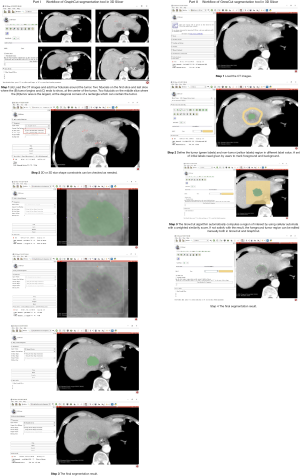
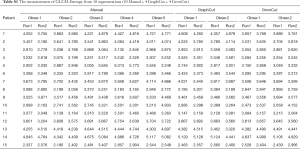
Full table
Acknowledgments
The authors would like to thank Dr. Lifei Zhang (MD Anderson Cancer Center) for her guidance.
Funding: This research was supported by the National Natural Science Foundation of China (No. 81472811 and No. 81272699), the Science and Technology Planning Project of Shandong Province (2014GSF118011) and the Shandong Natural Science Foundation (ZR2017PH071).
Footnote
Conflicts of Interest: All authors have completed the ICMJE uniform disclosure form (available at http://dx.doi.org/10.21037/tcr.2017.09.47). The authors have no conflicts of interest to declare.
Ethical Statement: The authors are accountable for all aspects of the work in ensuring that questions related to the accuracy or integrity of any part of the work are appropriately investigated and resolved. The study was conducted in accordance with the Declaration of Helsinki (as revised in 2013). This study was approved by Institutional Review Board (IRB) and ethics committee of Shandong Cancer Hospital Affiliated to Shandong University and the ID/number of ethics approval was 201606021. Informed consent was waived.
Open Access Statement: This is an Open Access article distributed in accordance with the Creative Commons Attribution-NonCommercial-NoDerivs 4.0 International License (CC BY-NC-ND 4.0), which permits the non-commercial replication and distribution of the article with the strict proviso that no changes or edits are made and the original work is properly cited (including links to both the formal publication through the relevant DOI and the license). See: https://creativecommons.org/licenses/by-nc-nd/4.0/.
References
- Singh S, Singh PP, Roberts LR, et al. Chemopreventive strategies in hepatocellular carcinoma. Nat Rev Gastroenterol Hepatol 2014;11:45-54. [Crossref] [PubMed]
- Dutta R, Mahato RI. Recent advances in hepatocellular carcinoma therapy. Pharmacol Ther 2017;173:106-17. [Crossref] [PubMed]
- Lambin P, Rios-Velazquez E, Leijenaar R, et al. Radiomics: extracting more information from medical images using advanced feature analysis. Eur J Cancer 2012;48:441-6. [Crossref] [PubMed]
- Gillies RJ, Kinahan PE, Hricak H. Radiomics: Images Are More than Pictures, They Are Data. Radiology 2016;278:563-77. [Crossref] [PubMed]
- Yip SS, Aerts HJ. Applications and limitations of radiomics. Phys Med Biol 2016;61:R150-66. [Crossref] [PubMed]
- Aerts HJ, Velazquez ER, Leijenaar RT, et al. Decoding tumour phenotype by noninvasive imaging using a quantitative radiomics approach. Nat Commun 2014;5:4006. [PubMed]
- Bashir U, Siddique MM, Mclean E, et al. Imaging Heterogeneity in Lung Cancer: Techniques, Applications, and Challenges. AJR Am J Roentgenol 2016;207:534-43. [Crossref] [PubMed]
- Rao SX, Lambregts DM, Schnerr RS, et al. CT texture analysis in colorectal liver metastases: A better way than size and volume measurements to assess response to chemotherapy? United European Gastroenterol J 2016;4:257-63. [Crossref] [PubMed]
- Zhou Y, He L, Huang Y, et al. CT-based radiomics signature: a potential biomarker for preoperative prediction of early recurrence in hepatocellular carcinoma. Abdom Radiol (NY) 2017;42:1695-704. [Crossref] [PubMed]
- O'Connor JP, Aboagye EO, Adams JE, et al. Imaging biomarker roadmap for cancer studies. Nat Rev Clin Oncol 2017;14:169-86. [Crossref] [PubMed]
- Kumar V, Gu Y, Basu S, et al. Radiomics: the process and the challenges. Magn Reson Imaging 2012;30:1234-48. [Crossref] [PubMed]
- Mackin D, Fave X, Zhang L, et al. Measuring Computed Tomography Scanner Variability of Radiomics Features. Invest Radiol 2015;50:757-65. [Crossref] [PubMed]
- Balagurunathan Y, Gu Y, Wang H, et al. Reproducibility and Prognosis of Quantitative Features Extracted from CT Images. Transl Oncol 2014;7:72-87. [Crossref] [PubMed]
- Balagurunathan Y, Kumar V, Gu Y, et al. Test-retest reproducibility analysis of lung CT image features. J Digit Imaging 2014;27:805-23. [Crossref] [PubMed]
- Hunter LA, Krafft S, Stingo F, et al. High quality machine-robust image features: identification in nonsmall cell lung cancer computed tomography images. Med Phys 2013;40:121916 [Crossref] [PubMed]
- Leijenaar RT, Carvalho S, Velazquez ER, et al. Stability of FDG-PET Radiomics features: an integrated analysis of test-retest and inter-observer variability. Acta Oncol 2013;52:1391-7. [Crossref] [PubMed]
- Echegaray S, Gevaert O, Shah R, et al. Core samples for radiomics features that are insensitive to tumor segmentation: method and pilot study using CT images of hepatocellular carcinoma. J Med Imaging (Bellingham) 2015;2:041011 [Crossref] [PubMed]
- Parmar C, Rios Velazquez E, Leijenaar R, et al. Robust Radiomics feature quantification using semiautomatic volumetric segmentation. PLoS One 2014;9:e102107 [Crossref] [PubMed]
- Fave X, Zhang L, Yang J, et al. Impact of image preprocessing on the volume dependence and prognostic potential of radiomics features in non-small cell lung cancer. Transl Cancer Res 2016;5:349-63. [Crossref]
- Hu P, Wang J, Zhong H, et al. Reproducibility with repeat CT in radiomics study for rectal cancer. Oncotarget 2016;7:71440-6. [PubMed]
- Velazquez ER, Aerts HJ, Gu Y, et al. A semiautomatic CT-based ensemble segmentation of lung tumors: Comparison with oncologists’ delineations and with the surgical specimen. Radiother Oncol 2012;105:167-73. [Crossref] [PubMed]
- Velazquez ER, Parmar C, Jermoumi M, et al. Volumetric CT-based segmentation of NSCLC using 3D-Slicer. Sci Rep 2013;3:3529. [Crossref] [PubMed]
- Fedorov A, Beichel R, Kalpathy-Cramer J, et al. 3D Slicer as an image computing platform for the Quantitative Imaging Network. Magn Reson Imaging 2012;30:1323-41. [Crossref] [PubMed]
- Vezhnevets V, Konouchine V. GrowCut: Interactive multi-label N-D image segmentation by cellular automata. Proc Graphicon 2005;1:150-6.
- Freedman D, Zhang T. Interactive graph cut based segmentation with shape priors. Computer Vision and Pattern Recognition, 2005. CVPR 2005. IEEE Computer Society Conference on. IEEE 2005;1:755-62.
- Zhang L, Fried DV, Fave XJ, et al. IBEX: an open infrastructure software platform to facilitate collaborative work in radiomics. Med Phys 2015;42:1341-53. [Crossref] [PubMed]
- Fave X, Mackin D, Yang J, et al. Can radiomics features be reproducibly measured from CBCT images for patients with non-small cell lung cancer? Med Phys 2015;42:6784-97. [Crossref] [PubMed]
- McGraw KO, Wong SP. Forming inferences about some intraclass correlation coefficients. Psychol Methods 1996;1:30-46. [Crossref]
- Cicchetti DV. Guidelines, criteria, and rules of thumb for evaluating normed and standardized assessment instruments in psychology. Psychol Assess 1994;6:284-290. [Crossref]
- Mattonen SA, Palma DA, Johnson C, et al. Detection of Local Cancer Recurrence After Stereotactic Ablative Radiation Therapy for Lung Cancer: Physician Performance Versus Radiomic Assessment. Int J Radiat Oncol Biol Phys 2016;94:1121-8. [Crossref] [PubMed]
- Court LE, Fave X, Mackin D, et al. Computational resources for radiomics. Transl Cancer Res 2016;5:340-8. [Crossref]