CRISPR-based genetic interaction maps inform therapeutic strategies in cancer
The future of cancer precision medicine lies in the rational design of effective therapies tailored to each patient based on the genetic makeup of their tumor. While the investigation of cancer genomes has successfully revealed cancer driver genes, leading to the development of targeted therapies, drug resistance is a major challenge in the clinic. The focus of this editorial is to highlight the potential of CRISPR-based genetic interaction (GI) maps in cancer cells to elucidate therapeutic targets, biomarkers, resistance mechanisms and combination therapy targets. We introduce the concept of GIs and their relevance to cancer therapy. We discuss systematic large-scale GI maps in mammalian cells using CRISPR-based technologies such as the CRISPR-cutting, CRISPR-interference (CRISPRi) and CRISPR-activation (CRISPRa) approaches.
GIs and their relevance in cancer
Most biological and disease-related phenotypes are controlled by more than one gene. A GI between two genes, A and B, is defined as the deviation of the observed phenotype for combined perturbation of both A and B from the expected phenotype (Figure 1A). Analysis of such interactions can reveal functional relationships between genes. A GI can be “buffering” when the combinatorial perturbation of two genes result in a less severe phenotype than expected indicating the genes act in a linear pathway or encode subunits of a functional protein complex. When a combinatorial perturbation of two genes results in a toxic phenotype they exhibit “synergistic” interaction or in more severe cases, a “synthetic lethal” interaction indicating the genes act in a parallel or redundant pathways.
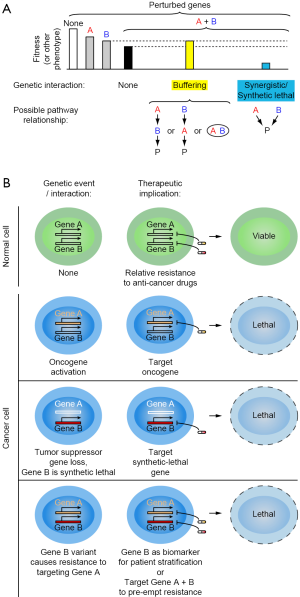
Synthetic lethality
Synthetic lethal interaction between two genes A and B can be defined as one where the loss or inhibition of either gene does not substantially affect the survival of cells, whereas combined inhibition of both genes is highly toxic. Synthetic-lethal gene pairs have an important role in cancer therapy. Cancer cell typically are dependent on genetic changes, which can be gain-of-function or loss-of-function events. A conceptually straightforward strategy to target cancers driven by gain-of-function events is the pharmacological targeting of the oncogene (Figure 1B). An early success story for such an oncogene-targeted therapy is the inhibition of Bcr-Abl fusion oncogene in Chronic Myelogenous Leukemia (1). It is conceptually much more challenging to devise targeted therapies for tumors that arose due to loss-of-function events such as the loss or mutation of a tumor suppressor gene. Since the relevant gene was lost from tumor cells, it cannot be a direct pharmacological target. In those cases, synthetic lethal interactions can provide a therapeutic strategy (Figure 1B). A first successful example was the targeting of poly (adenosine-diphosphate ribose) polymerase (PARP) in breast cancers deficient in the tumor suppressor genes BRCA1/BRCA2 (breast cancer-associated proteins). BRCA1/2-deficient cells are hypersensitive to PARP inhibition, suggesting synthetic lethality between BRCA1/2 and PARP (2). These studies led to successful use of PARP inhibitors such as Olaparib in several types of BRCA-deficient cancers, establishing a promise for synthetic lethality in cancer.
Drug response and precision medicine
Cancer patients carrying the same oncogenic driver gene or tumor suppressor gene loss can respond differently to a given drug indicating the importance of genetic background of these patients. GIs between the drug target gene and the genetic background controls drug response in patients (Figure 1B). Understanding these interactions will enable rational selection of the optimal therapeutic strategy for each patient. One way of studying such interactions is by correlating response to therapy with tumor genome in a population of patients. A potential problem of these studies is that there are several genetic alterations and considerable heterogeneity not only between patients carrying the same driver-gene but also within the individual tumor, making it challenging to identify functionally relevant genes. Experimental determination of GIs can reveal genetic modifiers of drug response. One example approach is to identify biomarker genes in patients correlating to drug response to match patients with optimal therapeutic strategy. Predictive biomarkers can be helpful in excluding patients from receiving a therapy that will not benefit. For example, presence of KRAS mutation in colorectal cancer patients has shown poor response to the epidermal growth factor receptor (EGFR) inhibitor cetuximab as opposed to patients having wild-type KRAS (3).
Drug resistance and combination therapy
Cancer patients who initially respond to a given therapy frequently relapse due to drug resistant cancer cells. Tumors are typically composed of heterogeneous cancer cells with a spectrum of mutations, including loss-of-function and gain-of-function events. Use of targeted inhibitors can kill the majority of tumor cells, but some cells may not respond to the inhibitor and/or develop resistance to the inhibitor. Cancer cells can be resistant to targeted therapy either through mutations in the direct drug target or through changes in other genes, such as activation of parallel pathways, or bypass of the cellular need for an oncogene by activation of downstream factors. Resistance mechanisms in genes other than the drug target are difficult to predict, but measurement of GIs can reveal potential resistance mechanisms. Knowledge of these mechanisms can enable the rational design of combination therapies targeting the primary oncogene and the potential resistance gene to preempt drug resistance (Figure 1B). A clinical example of a successful combination therapy is the treatment of HIV with a combination of three drugs that has led to marked improvement in patient survival and maintenance of the disease. Similarly, combination therapy in cancer has the potential to overcome drug resistance, as suggested by preclinical studies. For example, some non-small cell lung cancer (NSCLC) patients have been shown to carry mutations in the receptor tyrosine kinase, EGFR, in their tumor cells. Downstream of EGFR, both the RAS-MAPK and PI3K-AKT cell survival pathways are activated. Combined inhibition of the two parallel pathways—MAPK and PI3K pathways, has shown pre-clinical promise in synergistically killing NSCLC tumors both in vitro and in vivo (4,5).
CRISPR-based genetic approaches for mammalian cells
Tools for loss- and gain-of-function screens
Recent developments in genetic screening technologies have laid the groundwork for systematic forward genetics in mammalian cells. Until recently, the technology of choice for loss-of-function genetics in mammalian cells was RNA interference (RNAi). Although several improvements have been implemented in the RNAi system, it is still limited by its persistent off-target effects. Recently, CRISPR-based approaches were introduced for genetic screens in mammalian cells. CRISPR/Cas9 can be programmed to introduce DNA double stranded breaks at specific genomic loci by the introduction of a combination of bacterial Cas9 protein and single-guide RNA (sgRNA). In the absence of a donor DNA, this results in activation of non-homologous end joining (NHEJ) repair, which is error-prone and can result in frame shift deletions causing complete knockout of the targeted gene (Figure 2A). A variation of the traditional CRISPR-cutting system is the CRISPR-interference/-activation (CRISPRi/a) system that enables both loss-of-function and gain-of-function studies (6). The CRISPRi system utilizes a catalytically dead-Cas9 (dCas9) nuclease fused to a transcriptional repressor domain, Kruppel associated box (KRAB), resulting in transcriptional repression of specific target genes (Figure 2A). Compared to the CRISPR-cutting system, CRISPRi is inducible, reversible and non-toxic. The CRISPRi system can achieve different degrees of knockdown of genes, which may better phenocopy pharmacological inhibition of target genes with drugs, which is often partial. The CRISPRa system makes it possible to study gain-of-function events such as amplification or overexpression. The CRISPRa system utilizes the dCas9 to recruit transcriptional activator domains such as the VP64, resulting in transcriptional activation of specific target genes (Figure 2A).
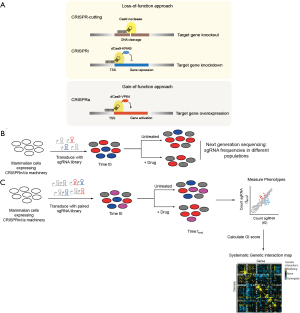
Primary screens to identify cell-intrinsic vulnerabilities and modifiers of drug response: several groups have used CRISPR-cutting and CRISPRi/a screens to identify novel intrinsic vulnerabilities in different cancer types. CRISPR-based screens can be performed in a massively-parallel format, in which a population of cells is stably transduced with a pooled lentiviral library of sgRNAs targeting different genes after which cells are selected for the specific phenotype of interest
A variation of the growth-based screen is performed in the presence of a drug of interest and can reveal genetic modifiers of drug response, or drug-gene interactions. sgRNAs affecting sensitivity to the drug can be quantified by comparing frequencies of sgRNA in the untreated and drug-treated cell populations grown in parallel. Genes knockdown of which causes the cells to sensitize to the drug indicate potential combination therapy targets. For example, we found that knockdown of PI3Kd sensitizes acute lymphoblastic leukemia cells to dexamethasone, and predicted a drug synergy between dexamethasone and the PI3Kd inhibitor CAL-101/idelalisib (10). Conversely, genes knockdown of which causes the cells to desensitize to the drug indicate potential drug resistance mechanisms. In general, the expression levels of genes found to modulate drug sensitivity can be biomarkers predictive of drug response in patients. An example from our own work is the finding that the response of multiple myeloma patients to the proteasome inhibitor carfilzomib correlates with pre-treatment expression levels of 19S proteasome subunits (11). CRISPRi and CRISPRa in combination are particularly powerful at identifying and validating relevant cellular targets of anti-cancer drugs, since the sensitivity of cells to a drug often depends on the expression level of the direct target (12-14).
Systematic large-scale GI maps
Systematic quantification of pairwise gene interactions for a large set of genes results in a GI map. Such maps reveal individual gene pairs with strong GIs, including rare synthetic lethal interaction pairs that can be excellent candidates for therapy. Depending on their GI score genes can be clustered in a GI map that reveals genes in functionally related pathways. This enables one to understand functions of previously uncharacterized genes. While specific cancers can be driven by one of a small number of characterized oncogenes and tumor suppressor genes, there is a lot of heterogeneity in cancer aggressiveness and response to therapy indicating the importance of different genetic background. Performing such GI screens in cell lines having different genetic background will reveal genes that modify the driver gene effect.
Earlier, GIs were studied in an arrayed screening format where phenotype for gene-pairs is quantified individually. Although arrayed screens allow for multi-parametric phenotype analysis, conducting such screens in large scale can be challenging to implement (15). Performing GIs using pooled double-perturbations, which are massively parallel enable large scale GI maps in mammalian cells (Figure 2C). We introduced the first systematic GI map in mammalian cells using RNAi-based pooled genetic screen (16). Our strategy first identified high-confidence hit genes using an ultracomplex shRNA library for a specific phenotype following which we generated double-shRNA libraries to construct a GI map between the gene-pairs. More recently, similar approaches have been implemented based on CRISPR technology, including CRISPR-cutting (17-20), CRISPRi (21,22) and CRISPRa (23). We recently demonstrated that combined gain-and-loss of function GI maps leveraging orthogonal CRISPR cutting and CRISPRa approaches can enable the elucidation of directional pathway information (23).
Pairwise genetic combinations from pooled screens enable highly parallel investigation of target combinations in a single experiment. In a recently published paper (19), Han and colleagues design a CRISPR-cutting based double knockout (CDKO) system to generate a large-scale mammalian GI map comprising of 21,321 drug combinations and successfully identified synthetic lethal drug target pairs in K562 chronic myeloid leukemia (CML) cells. The authors rationally designed their gene-interaction library based on the following criteria: (I) druggable genes; (II) expression level in K562 cells; (III) growth phenotype from previous screens. The authors were able to successfully identify previously reported genetic pairs and GIs that can be explained by functional redundancy such as the PIM1-PIM2; AKT1-AKT2 interactions. To validate the CRISPR-based GI map, the authors developed a GI map for AB-type protein toxin ricin, for which extensive data on functional and GIs has been published previously (24). The GI map generated for ricin toxicity clearly identified a number of functional complexes as published previously validating their system. Moreover, synthetic lethal genetic pairs identified from the GI map validated using target-specific small molecule inhibitors, demonstrating these could recapitulate GIs from the DrugTarget-CDKO map. Systematic design of drug target gene pairs in this study robustly identified a potential strategy for combination therapy using BCL2L1 and MCL1 inhibitors to prevent resistance in CML.
Conclusions
The complex genetic heterogeneity of a tumor makes it essential to understand gene interactions and their therapeutic implications in cancer. Systematic GI maps are powerful tools for understanding gene functions within pathways or networks and identifying synthetic lethal gene interaction pairs revealing potential combination therapy targets. Previously combination therapies in cancer have been researched by array-based functional chemical approaches that test for combination of small-molecule inhibitors resulting in synergistic cytotoxicity (25). One major challenge with such approaches includes scalability of such approaches, and the fact that many drugs inhibit more than one cellular target. Current advances in genomic approaches such as the CRISPR-based technology have enabled systematic large-scale GI maps. Important future applications will need to address the interactions between tumor cells and their microenvironment, and investigate genetic determinants controlling response to promising new immunotherapies.
Acknowledgments
Funding: Cancer research in the Kampmann lab is supported by K99/R00 CA181494 (M Kampmann), a Stand Up to Cancer Innovative Research Grant (M Kampmann), and the Grand Multiple Myeloma Translational Initiative.
Footnote
Provenance and Peer Review: This article was commissioned and reviewed by the Section Editor Chen Qian (Center for Inflammation & Epigenetics, Houston Methodist Hospital Research Institute, Houston, TX, USA).
Conflicts of Interest: Both authors have completed the ICMJE uniform disclosure form (available at http://dx.doi.org/10.21037/tcr.2018.01.02). The authors have no conflicts of interest to declare.
Ethical Statement: The authors are accountable for all aspects of the work in ensuring that questions related to the accuracy or integrity of any part of the work are appropriately investigated and resolved.
Open Access Statement: This is an Open Access article distributed in accordance with the Creative Commons Attribution-NonCommercial-NoDerivs 4.0 International License (CC BY-NC-ND 4.0), which permits the non-commercial replication and distribution of the article with the strict proviso that no changes or edits are made and the original work is properly cited (including links to both the formal publication through the relevant DOI and the license). See: https://creativecommons.org/licenses/by-nc-nd/4.0/.
References
- Savage DG, Antman KH. Imatinib mesylate--a new oral targeted therapy. N Engl J Med 2002;346:683-93. [Crossref] [PubMed]
- Ashworth A. A synthetic lethal therapeutic approach: poly(ADP) ribose polymerase inhibitors for the treatment of cancers deficient in DNA double-strand break repair. J Clin Oncol 2008;26:3785-90. [Crossref] [PubMed]
- Karapetis CS, Khambata-Ford S, Jonker DJ, et al. K-ras mutations and benefit from cetuximab in advanced colorectal cancer. N Engl J Med 2008;359:1757-65. [Crossref] [PubMed]
- Chan BA, Hughes BGM. Targeted therapy for non-small cell lung cancer: current standards and the promise of the future. Transl Lung Cancer Res 2015;4:36-54. [PubMed]
- Meng J, Dai B, Fang B, et al. Combination treatment with MEK and AKT inhibitors is more effective than each drug alone in human non-small cell lung cancer in vitro and in vivo. PLoS One 2010;5:e14124 [Crossref] [PubMed]
- Kampmann M. CRISPRi and CRISPRa Screens in Mammalian Cells for Precision Biology and Medicine. ACS Chem Biol 2017; [Epub ahead of print]. [Crossref] [PubMed]
- Wang T, Yu H, Hughes NW, et al. Gene Essentiality Profiling Reveals Gene Networks and Synthetic Lethal Interactions with Oncogenic Ras. Cell 2017;168:890-903.e15. [Crossref] [PubMed]
- Hart T, Chandrashekhar M, Aregger M, et al. High-Resolution CRISPR Screens Reveal Fitness Genes and Genotype-Specific Cancer Liabilities. Cell 2015;163:1515-26. [Crossref] [PubMed]
- Martin TD, Cook DR, Choi MY, et al. A Role for Mitochondrial Translation in Promotion of Viability in K-Ras Mutant Cells. Cell Rep 2017;20:427-38. [Crossref] [PubMed]
- Kruth KA, Fang M, Shelton DN, et al. Suppression of B-cell development genes is key to glucocorticoid efficacy in treatment of acute lymphoblastic leukemia. Blood 2017;129:3000-8. [Crossref] [PubMed]
- Acosta-Alvear D, Cho MY, Wild T, et al. Paradoxical resistance of multiple myeloma to proteasome inhibitors by decreased levels of 19S proteasomal subunits. Elife 2015;4:e08153 [Crossref] [PubMed]
- Anderson Daniel J, Le Moigne R, Djakovic S, et al. Targeting the AAA ATPase p97 as an Approach to Treat Cancer through Disruption of Protein Homeostasis. Cancer Cell 2015;28:653-65. [Crossref] [PubMed]
- Jost M, Chen Y, Gilbert LA, et al. Combined CRISPRi/a-Based Chemical Genetic Screens Reveal that Rigosertib Is a Microtubule-Destabilizing Agent. Mol Cell 2017;68:210-223.e6. [Crossref] [PubMed]
- Kampmann M. Elucidating drug targets and mechanisms of action by genetic screens in mammalian cells. Chem Commun (Camb) 2017;53:7162-7. [Crossref] [PubMed]
- Laufer C, Fischer B, Billmann M, et al. Mapping genetic interactions in human cancer cells with RNAi and multiparametric phenotyping. Nat Methods 2013;10:427-31. [Crossref] [PubMed]
- Kampmann M, Bassik MC, Weissman JS. Integrated platform for genome-wide screening and construction of high-density genetic interaction maps in mammalian cells. Proc Natl Acad Sci U S A 2013;110:E2317-26. [Crossref] [PubMed]
- Shen JP, Zhao D, Sasik R, et al. Combinatorial CRISPR-Cas9 screens for de novo mapping of genetic interactions. Nat Methods 2017;14:573-6. [Crossref] [PubMed]
- Wong AS, Choi GC, Cui CH, et al. Multiplexed barcoded CRISPR-Cas9 screening enabled by CombiGEM. Proc Natl Acad Sci U S A 2016;113:2544-9. [Crossref] [PubMed]
- Han K, Jeng EE, Hess GT, et al. Synergistic drug combinations for cancer identified in a CRISPR screen for pairwise genetic interactions. Nat Biotechnol 2017;35:463-74. [Crossref] [PubMed]
- Rosenbluh J, Mercer J, Shrestha Y, et al. Genetic and Proteomic Interrogation of Lower Confidence Candidate Genes Reveals Signaling Networks in beta-Catenin-Active Cancers. Cell Syst 2016;3:302-16.e4. [Crossref] [PubMed]
- Roguev A, Talbot D, Negri GL, et al. Quantitative genetic-interaction mapping in mammalian cells. Nat Methods 2013;10:432-7. [Crossref] [PubMed]
- Du D, Roguev A, Gordon DE, et al. Genetic interaction mapping in mammalian cells using CRISPR interference. Nat Methods 2017;14:577-80. [Crossref] [PubMed]
- Boettcher M, Tian R, Blau J, et al. Decoding directional genetic dependencies through orthogonal CRISPR/Cas screens. Nat Biotech 2018 (In Press). bioRxiv 2017;120170. Available online: https://doi.org/
10.1101/120170 - Bassik MC, Kampmann M, Lebbink RJ, et al. A systematic mammalian genetic interaction map reveals pathways underlying ricin susceptibility. Cell 2013;152:909-22. [Crossref] [PubMed]
- Zimmermann GR, Lehár J, Keith CT. Multi-target therapeutics: when the whole is greater than the sum of the parts. Drug Discov Today 2007;12:34-42. [Crossref] [PubMed]