Common and specific genes in ovarian clear cell carcinoma and serous carcinoma by gene expression analysis
Introduction
Clear cell carcinoma (CCC) and serous carcinoma (SC) are two major histological types of epithelial ovarian carcinoma, one of the deadliest gynecologic malignancies, and have different biological features and clinical behaviors. Epidemiological studies have suggested a genetic predisposition for ovarian cancer, which can run in families and also favors second primary tumors (1,2). High-grade SC is the most common subtype of ovarian cancer with approximately 70% of cases and CCC occurs at a frequency of approximately 12% (3). SC originates from the surface of the ovary or in the distal fallopian tube, whereas CCC arises from endometriosis and tends to occur in younger women, 5–6 years earlier than high-grade SC (4). Despite CCC presents with earlier-stage disease, it usually correlates with poor prognosis. For patients with CCC, median overall survival (OS) is 21.3 months (95% CI, 17.8–28.1 months) compared to a median OS of 40.8 months (95% CI, 39.7–42.2 months) for women with SC (5). The standard of epithelial ovarian cancer care remains surgery and platinum- and paclitaxel-based chemotherapies. However, CCC is reported to be resistant to standard carboplatin-paclitaxel based chemotherapy regimens (6).
High-grade SC is characterized by a high frequency of TP53 mutation with over 96% cases, and TP53 is in fact the only gene that is frequently mutated at the somatic level in high-grade SC (7). TP53 mutations occur early in tumorigenesis and thus are likely in precursor lesions of ovarian cancer, highlighting its important roles in high-grade SC. Different from SC, CCC are genetically characterized by frequent mutations of ARID1A and PIK3CA genes. The AT-rich interacting domain-containing protein 1A gene (ARID1A), a tumor suppressor, appears to be mutated in 46–57% of CCC, but not in high-grade SC (8). ARID1A mutation occurs at the early stage of cancerization from endometriosis to ovarian carcinoma, suggesting that detection of ARID1A mutation may be used for early diagnosis of endometriosis-associated ovarian carcinoma (9,10). Phosphatidylinositol-3-kinase (PI3K)/AKT pathway is reported as one of the mechanisms for the carcinogenesis of ARID1A mutation (8). The phosphatidylinositol-4,5-bisphosphate 3-kinase catalytic subunit α (PIK3CA) is a catalytic subunit of PI3K, and the frequency of PIK3CA mutations in CCC is approximately 33–43% (4). In addition, PIK3CA mutation and loss of ARID1A expression occur simultaneously (9). Except for ARID1A and PIK3CA mutations, loss of PTEN expression, over-expression of PPM1D and high levels of MTOR are also the most important molecular events that characterize ovarian CCC (8).
Gene expression profiles differences of ovarian SC and CCC have been documented, providing molecular difference in gene expression and guiding targeted therapeutic approaches among different histologic types of ovarian carcinomas. In the study by Pamula-Pilat et al., the authors find TCF2 (HNF1B) gene as a suitable marker for ovarian CCC and conclude that their gene expression profiling also shed light on the molecular mechanisms of different chemoresistance among three histologic types of ovarian carcinoma (clear-cell, endometrioid and serous) (11). Espinosa et al. report that co-expression of caspase-3 and XIAP identify two biological subtypes of high-grade SC with different prognosis by investigating the expression profile of 22 genes involved in the PI3K-AKT pathway in 19 ovarian SC and 7 ovarian CCC (12). Yanaihara et al. determine ovarian-related miRNA gene expression profiles in high-grade SC and CCC and find that miR-9 overexpression may affect CCC pathogenesis by targeting E-cadherin, inducing epithelial-mesenchymal transition (13). These studies directly compare the gene expression profiles between SC and CCC (or between CCC and SC) to identify SC biomarkers (or CCC biomarkers). However, they usually miss the specific genes changed only in SC or in CCC, which is important as the origins of the two malignancies are varying. Differ from these studies, we firstly compared the gene expression profiles between SC or CCC and normal control to identify differentially expressed genes in SC or CCC, and then performed overlap analysis to identify common genes changed both in SC and CC and specific genes independent in SC or CCC. The common genes benefit to shed light on the common part of molecular mechanisms for ovarian carcinomas pathogenesis, whereas the specific genes provide unique targeted therapy for each histological subtype.
Methods
Statement of ethics approval
The original data of this study was from GEO datasets and Oncomine datasets, thus, the statement of ethics approval was not required.
Derivation of gene expression data
The gene expression profiles of GSE29450 and GSE36668 were downloaded from the Gene Expression Omnibus (GEO) database (www.ncbi.nlm.nih.gov/geo), a public functional genomics data repository. The annotation platform was all the GLP201 Affymetrix Human HG-Focus Target Array platform (Affymetrix, Inc., Santa Clara, CA, USA). A total of 20 samples in GSE29450 are available, including 10 clear cell ovarian cancers and 10 normal ovarian surface epithelium. GSE36668 contains four serous ovarian carcinomas and four superficial scraping from normal ovary.
Data processing
The raw data including Series Matrix File(s) and annotation soft table were downloaded from GSE29450 and GSE36668 datasets. Probe serial numbers in the matrix were transformed into gene names by Perl (14). The R, a free software environment for statistical computing and graphics, was used to pre-process the raw data via background correction, quantile normalization, and applied “impute” package (15) to complement missing expression with its adjacent value. For genes corresponding to more than one probe, gene expression levels were determined by the average probe values. Through these processes, finally a data file was output which contains all available Entrez Gene identifiers and their corresponding expression values in all investigated samples.
Differentially expression analysis
Limma (16) package was used to screen the DEGs between CCC and normal ovarian surface epithelium in GSE29450 and that between SC and superficial scraping from normal ovary in GSE36668 with |log2(fold change)| >0.45 and adjusted P value <0.05. The adjusted P value was obtained through applying Benjamini and Hochberg’s (BH) false discovery rate correction on the original P value. Cluster analysis and classifications were based on the DEGs of each histologic subtype.
Functional enrichment analysis
To functionally annotate DEGs identified between the ovarian carcinoma group and the normal ovarian surface epithelium group, R packages including GOstats and clusterProfiler (17) were used to analyze the Gene Ontology (GO) categories and Kyoto Encyclopedia of Genes and Genomes (KEGG) pathways with significant over representation in DEGs compared with the whole genome (P value <0.05).
Protein-protein interaction (PPI) network construction
Cytoscape is an open source software platform for visualizing complex networks and integrating these with any type of attribute data (18). PPI databases from HPRD (19), BIOGRID (20), and PIP (21) databases were downloaded, and 562252 pair interactions were extracted. Cytoscape 3.2.1 (22) was used to construct interaction network, and the interacted gene pairs in our curated PPI database were imported as stored network.
Oncomine analysis
The expression levels of ESPL1 and CDC25C gene in ovarian carcinoma were identified from Oncomine database (www.oncomine.org), which is an online cancer microarray database to facilitate discovery from genome-wide expression analyses. The mRNA expression fold in ovarian cancer tissue compared to the normal tissue was obtained. We used a Students’ t-test to generate a P value which was set up at 0.01.
Results
Differentially expressed genes between each ovarian cancer histologic subtype and normal ovarian epithelium
To identify genes involved in the development of ovarian CCC and SC, separate comparisons of each histologic subtype to normal ovary brushings were completed. Gene expression data from 10 CCC samples and 10 normal ovarian surface epithelium samples were obtained from GSE29450 dataset. With adjusted P value <0.05 and |log2(fold change)| >0.45, 4,483 DEGs between the CCC group and the normal group were obtained. Nearly half of these DEGs [2,204] were upregulated. The volcano plot depicting DEGs (red and green points) was shown in Figure 1A. A hierarchical clustering analysis of the expression values was shown in Figure 1B.
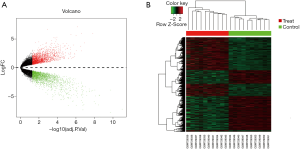
To screen DEGs between the SC group and the normal group, we analyzed GSE36668 dataset including 4 CCC samples and 4 surface epithelium scrapings from normal ovaries, and identified 5,693 DEGs. A total of 2,719 genes have increased expression in ovarian SC compared with normal ovarian epithelium, whereas 2,974 have decreased expression in SC. The volcano plot and heat map were shown in Figure 2.
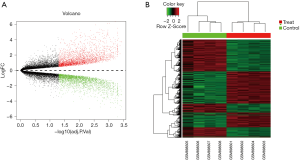
Common DEGs and their biological meaning in both histologic subtypes
To identify the common DEGs deregulated in both ovarian CCC and SC, we performed overlap analysis. Overlapping the DEGs of the two subtypes of ovarian carcinoma, 1,265 common genes were identified. To identify the functions of these DEGs, all of the up- and downregulated genes were mapped to terms of the GO categories, and KEGG was used to further identify the altered biological functions arising from these common DEGs. Accordingly, 1,103 GO terms and ten KEGG pathways, such as “chromosome segregation”, “cell cycle”, “cell division” and “nuclear division” were extracted, which are important factors for identifying key genes.
In addition, we constructed a biological network by 1,126 pair interactions (Figure 3). To screen the hub node proteins with a large connection degree, the constructed network was divided into relative independent sub-modules. Three critical modules were obtained by Cytocluster, and key nodes were identified according to the connection degree in critical modules, including CDC6, CDK1, CCNB1, ESPL1, CDC25C and CCNB2. CCNB1, CDC6 and ESPL1 were involved in “chromosome segregation”, whereas CDK1, CCNB1 and CCNB2 participated in “p53 signaling pathway”. These key nodes were all cell cycle genes, and CDC6, CDK1, CCNB1 and CCNB2 have been reported to be correlated with ovarian cancer progression or prognosis (23-26). However, this is the first time to report that ESPL1 and CDC25C were associated with ovarian carcinoma. Thus, we further performed Oncomine analysis on these two novel genes. As is shown in Figure 4A,B, CDC25C is highly expressed in grade 3 of ovarian serous adenocarcinoma compared with grade 2, and elevated level of CDC25C is observed in ovarian serous adenocarcinoma compared with normal peritoneum. From Figure 4C, ESPL1 expression is significantly increased in grade 2 of ovarian mucinous adenocarcinoma compared with grade 1 with over 103 fold change. Similar to CDC25C, ESPL1 is also up-regulated in ovarian serous adenocarcinoma compared with normal peritoneum (Figure 4D).
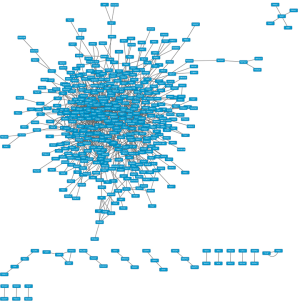
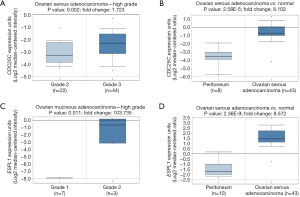
Specific DEGs and their biological meaning in each histologic subtype
To determine the specific DEGs deregulated only in one histologic subtype, we performed overlap analysis. Total 2,971 specific DEGs were identified in the development of ovarian CCC, and these DEGs were enriched in 857 GO terms and 10 KEGG pathways, including “tissue morphogenesis” and “vascular smooth muscle contraction”. The PPI network containing 3,404 pair interactions were constructed (Figure 5). Then, we performed Cytocluster analysis and obtained 15 critical modules with key nodes. Among the key nodes, several members of ribosomes family were found (Table 1), including RPL11, RPL15, RPL23A, RPL27A, RPS25, RPS5, RPS6 and RPS7, suggesting their important roles in ovarian CCC.
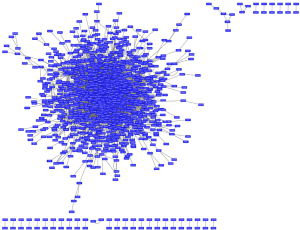
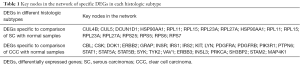
Full table
Whereas in ovarian SC, a list of 4,181 DEGs were specifically identified. And these specific DEGs were enriched in 911 GO terms and 34 KEGG pathways, which included “positive regulation of biological process” and “cell adhesion molecules (CAMs)”. With these specific DEGs, we constructed a PPI network by using 8,341 pair interactions and some key nodes were identified in the top 15 significant modules according to the connection degree, such as KIT and SYK (Figure 6 and Table 1).
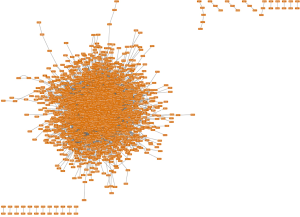
Discussion
Gene expression profiling studies have demonstrated an essential role of genes in ovarian carcinogenesis. Direct comparison of ovarian histological subtypes allows for comparisons and observations and has clinical implications, however, Zorn et al. failed to identified a gene set that distinguish SC from CCC by using this way, suggesting the two ovarian histological subtypes have different gene expression (30). Thus, in this study, we make two comparisons between each ovarian carcinoma subtype and normal ovarian surface epithelium. With adjusted P value<0.05 and |log2(fold change)| >0.45, a group of 4,483 genes appeared on ovarian CCC comparison with normal samples, whereas 5,693 DEGs were identified in ovarian SC comparing with normal samples, nearly 27% more than that in CCC.
Overlap analysis only revealed 1,265 common DEGs with consistent up- or down-regulation in both SC and CCC. This suggests that at least part of the cell processes in carcinogenesis is shared between SC and CCC. We identified the functions of these common DEGs by performing functional annotation and pathway enrichment analysis, and “chromosome segregation” “cell cycle”, “cell division” and “nuclear division” were significant abnormal in both subtypes. Then, PPI network and oncomine analysis showed ESPL1 and CDC25C as novel genes associated with ovarian carcinoma. And Oncomine analysis validated the expression of ESPL1 and CDC25C in ovarian cancer. ESPL1/separase, an enzyme that cleaves the chromosomal cohesion during mitosis, is highly expressed in tumors and overexpression of ESPL1 in animal models results in aneuploidy and tumorigenesis (31). In luminal tumors, overexpression of ESPL1 causes complex genomic profiles and molecular features of chromosomal instability and loss of tumor suppressor genes (P53 and Rb) (32). The importance of chromosomal cohesion and separation in tumorigenesis has become increasingly evident (31), thus, the combination of chromosomal instability and loss of P53 function further results in tumorigenesis and disease progression. In our study, ESPL1 was highly expressed in both SC and CCC, and involved in “chromosome segregation”, “cell cycle”, “cell division” and “nuclear division” pathways, which were the top significant pathways in ovarian carcinoma. It is also reported that separase activity is critical to the smooth progression to cytokinesis (31). Accordingly, the role of ESPL1 is of critical importance in ovarian SC and CCC, future studies present the possibility of using this protein as a diagnostic marker for the disease. The cell division cycle protein 25 homolog C (CDC25C) is a dual specificity phosphatase playing a crucial role in the cell cycle in the G2-M phase transition. In prostate cancer, CDC25C up-regulation promotes tumor development and progression by regulating androgen receptor activation (33). In addition, CDC25C is reported to be a potential biomarker of radio-resistance. Li et al. find that the total level of CDC25C increases only after irradiation and its activity remains stable, suggesting that it may be the increased presence of the protein itself that modifies the radio-sensitivity of lung cancer cells (34). Moreover, in our study, we determined that CDC25C mRNA expression was also increased in primary tumors of previously untreated ovarian carcinoma patients, revealing its potential role in ovarian cancer.
The specific genes in each histologic subtype revealed huge differences in gene expression so that 2,971 (66% of the whole DEGs) specific DEGs were identified in the development of ovarian CCC, whereas in ovarian SC, a list of 4,181 (73% of the whole DEGs) DEGs were specifically identified. Moreover, these specific DEGs constructed a huge and complicated PPI network. These findings reveal that these two ovarian histological types have huge differences in gene expression, which may be correlated with their different response to regular treatment and makes it less likely that they can be clinically managed in an identical fashion. In the PPI network constructed by specific DEGs in CCC, we further identified several key nodes belonging to ribosome family, including RPL11, RPL15, RPL23A, RPL27A, RPS25, RPS5, RPS6 and RPS7. Ribosomes are cellular machines essential for protein synthesis, and ribosomal protein genes are reported to play important roles in cancer. RPL15 is reported as a prognostic marker in pancreatic ductal adenocarcinoma, decreased expression of which is significantly associated with poor overall survival (35). RPL23 as well as RPS13 may suppress drug-induced apoptosis of gastric cancer cells (36). High expression of RPS6 is associated with high grade renal cell carcinoma and poor clinical outcome (37). RPS7 suppresses ovarian tumorigenesis and metastasis through PI3K/AKT and MAPK signal pathways (38). In our study, these ribosomal protein genes function as key nodes in ovarian CCC, suggesting that they may be used as potential marker for ovarian CCC.
Common genes identified in our study may benefit to shed light on the common part of molecular mechanisms for ovarian carcinomas pathogenesis, whereas the specific genes provide unique targeted therapy for each histological subtype. CCC is associated with poor prognosis after the regular treatment for ovarian carcinoma, and the failure of current chemotherapy for CCC is recognized. Thus, new therapies predominating to cure CCC may be developed and the specific genes differentially expressed in CCC only, such as ribosomal protein genes, may be designed as one of the interventions.
In conclusion, the present study analyzed the gene expression profiles between ovarian carcinoma samples (CCC and SC) and normal ovarian surface epithelium, and overlap analysis identified the common and specific DEGs in ovarian CCC and SC. Functional annotation of the DEGs into GO terms and KEGG pathways was performed, and a PPI network was constructed, followed by module analysis. Common genes including ESPL1 and CDC25C may have important roles in ovarian carcinoma development, and specific genes including ribosomal protein genes may be associated with CCC progression and unique targeted therapy.
Acknowledgments
Funding: None.
Footnote
Conflicts of Interest: Both authors have completed the ICMJE uniform disclosure form (available at http://dx.doi.org/10.21037/tcr.2018.11.12). The authors have no conflicts of interest to declare.
Ethical Statement: The authors are accountable for all aspects of the work in ensuring that questions related to the accuracy or integrity of any part of the work are appropriately investigated and resolved. The study was conducted in accordance with the Declaration of Helsinki (as revised in 2013). The original data of this study was from GEO datasets and Oncomine datasets, thus, the statement of ethics approval was not required. Informed consent was waived.
Open Access Statement: This is an Open Access article distributed in accordance with the Creative Commons Attribution-NonCommercial-NoDerivs 4.0 International License (CC BY-NC-ND 4.0), which permits the non-commercial replication and distribution of the article with the strict proviso that no changes or edits are made and the original work is properly cited (including links to both the formal publication through the relevant DOI and the license). See: https://creativecommons.org/licenses/by-nc-nd/4.0/.
References
- Kanninen TT, Nasioudis D, Sisti G, et al. Epidemiology of Second Primary Tumors in Women With Ovarian Cancer. Int J Gynecol Cancer 2017;27:659-67. [Crossref] [PubMed]
- Nasioudis D, Ramer I, Sisti G, et al. Risk of Second Primary Tumors After Childhood and Adolescent Ovarian Malignancies: A SEER Analysis (1973-2011). J Pediatr Adolesc Gynecol 2015;28:522-5. [Crossref] [PubMed]
- Wiegand KC, Hennessy BT, Leung S, et al. A functional proteogenomic analysis of endometrioid and clear cell carcinomas using reverse phase protein array and mutation analysis: protein expression is histotype-specific and loss of ARID1A/BAF250a is associated with AKT phosphorylation. BMC Cancer 2014;14:120. [Crossref] [PubMed]
- Ku FC, Wu RC, Yang LY, et al. Clear cell carcinomas of the ovary have poorer outcomes compared with serous carcinomas: Results from a single-center Taiwanese study. J Formos Med Assoc 2018;117:117-25. [Crossref] [PubMed]
- Boelling J, Iversen V. Recommendations of the 2005 Ovarian Cancer State of the Science Meeting. Gynecol Oncol 2006;103:26. [Crossref]
- del Carmen MG, Birrer M, Schorge JO. Clear cell carcinoma of the ovary: a review of the literature. Gynecol Oncol 2012;126:481-90. [Crossref] [PubMed]
- Cole AJ, Dwight T, Gill AJ, et al. Assessing mutant p53 in primary high-grade serous ovarian cancer using immunohistochemistry and massively parallel sequencing. Sci Rep 2016;6:26191. [Crossref] [PubMed]
- Takeda T, Banno K, Okawa R, et al. ARID1A gene mutation in ovarian and endometrial cancers Oncol Rep 2016;35:607-13. (Review). [Crossref] [PubMed]
- Yamamoto S, Tsuda H, Takano M, et al. Loss of ARID1A protein expression occurs as an early event in ovarian clear-cell carcinoma development and frequently coexists with PIK3CA mutations. Mod Pathol 2012;25:615-24. [Crossref] [PubMed]
- Ayhan A, Mao TL, Seckin T, et al. Loss of ARID1A expression is an early molecular event in tumor progression from ovarian endometriotic cyst to clear cell and endometrioid carcinoma. Int J Gynecol Cancer 2012;22:1310-5. [Crossref] [PubMed]
- Pamula-Pilat J, Rubel T, Rzepecka IK, et al. Gene expression profiles in three histologic types, clear-cell, endometrioid and serous ovarian carcinomas. J Biol Regul Homeost Agents 2014;28:659-74. [PubMed]
- Espinosa I, Catasus L, Canet B, et al. Gene expression analysis identifies two groups of ovarian high-grade serous carcinomas with different prognosis. Mod Pathol 2011;24:846-54. [Crossref] [PubMed]
- Yanaihara N, Noguchi Y, Saito M, et al. MicroRNA Gene Expression Signature Driven by miR-9 Overexpression in Ovarian Clear Cell Carcinoma. PLoS One 2016;11:e0162584 [Crossref] [PubMed]
- Stajich JE, Block D, Boulez K, et al. The Bioperl toolkit: Perl modules for the life sciences. Genome Res 2002;12:1611-8. [Crossref] [PubMed]
- Hastie T, Tibshirani R, Narasimhan B, et al. Impute: Imputation for microarray data. Oral History Review 2011;128-30.
- Smyth GK. limma: Linear Models for Microarray Data. In: Gentleman R, Carey VJ, Huber W, et al. editors. Bioinformatics & Computational Biology Solutions Using R & Bioconductor. New York, NY: Springer, 2005:397-420.
- Yu G, Wang LG, Han Y, et al. clusterProfiler: an R package for comparing biological themes among gene clusters. OMICS 2012;16:284-7. [Crossref] [PubMed]
- Kalinka AT. The generation, visualization, and analysis of link communities in arbitrary networks with the R package linkcomm. 2011.
- Keshava Prasad TS, Goel R, Kandasamy K, et al. Human Protein Reference Database--2009 update. Nucleic Acids Research 2009;37:767-72. [Crossref] [PubMed]
- Chatraryamontri A, Breitkreutz BJ, Heinicke S, et al. The BioGRID interaction database: 2013 update. Nucleic Acids Research 2013;41:816-23. [Crossref] [PubMed]
- McDowall MD, Scott MS, Barton GJ. PIPs: human protein-protein interaction prediction database. Nucleic Acids Res 2009;37:D651-6. [PubMed]
- Smoot ME, Ono K, Ruscheinski J, et al. Cytoscape 2.8: new features for data integration and network visualization. Bioinformatics 2011;27:431-2. [Crossref] [PubMed]
- Deng Y, Jiang L, Wang Y, et al. High expression of CDC6 is associated with accelerated cell proliferation and poor prognosis of epithelial ovarian cancer. Pathol Res Pract 2016;212:239-46. [Crossref] [PubMed]
- Zhang R, Shi H, Ren F, et al. The aberrant upstream pathway regulations of CDK1 protein were implicated in the proliferation and apoptosis of ovarian cancer cells. J Ovarian Res 2017;10:60. [Crossref] [PubMed]
- Wei S, Liu J, Shi Y, et al. Exploration of the sequential gene changes in epithelial ovarian cancer induced by carboplatin via microarray analysis. Mol Med Rep 2017;16:3155-60. [Crossref] [PubMed]
- Cunningham JM, Vierkant RA, Sellers TA, et al. Cell cycle genes and ovarian cancer susceptibility: a tagSNP analysis. Br J Cancer 2009;101:1461-8. [Crossref] [PubMed]
- Denkert C, Budczies J, Darb-Esfahani S, et al. A prognostic gene expression index in ovarian cancer - validation across different independent data sets. J Pathol 2009;218:273-80. [Crossref] [PubMed]
- Yoshihara K, Tajima A, Komata D, et al. Gene expression profiling of advanced-stage serous ovarian cancers distinguishes novel subclasses and implicates ZEB2 in tumor progression and prognosis. Cancer Sci 2009;100:1421-8. [Crossref] [PubMed]
- Schwartz DR, Kardia SL, Shedden KA, et al. Gene expression in ovarian cancer reflects both morphology and biological behavior, distinguishing clear cell from other poor-prognosis ovarian carcinomas. Cancer Res 2002;62:4722-9. [PubMed]
- Zorn KK, Bonome T, Gangi L, et al. Gene expression profiles of serous, endometrioid, and clear cell subtypes of ovarian and endometrial cancer. Clin Cancer Res 2005;11:6422-30. [Crossref] [PubMed]
- Mukherjee M, Byrd T, Brawley VS, et al. Overexpression and constitutive nuclear localization of cohesin protease Separase protein correlates with high incidence of relapse and reduced overall survival in glioblastoma multiforme. J Neurooncol 2014;119:27-35. [Crossref] [PubMed]
- Finetti P, Guille A, Adelaide J, et al. ESPL1 is a candidate oncogene of luminal B breast cancers. Breast Cancer Res Treat 2014;147:51-9. [Crossref] [PubMed]
- Dedobbeleer M, Willems E, Freeman S, et al. Phosphatases and solid tumors: focus on glioblastoma initiation, progression and recurrences. Biochem J 2017;474:2903-24. [Crossref] [PubMed]
- Li J, Yang CX, Mei ZJ, et al. Involvement of cdc25c in cell cycle alteration of a radioresistant lung cancer cell line established with fractionated ionizing radiation. Asian Pac J Cancer Prev 2013;14:5725-30. [Crossref] [PubMed]
- Yan TT, Fu XL, Li J, et al. Downregulation of RPL15 may predict poor survival and associate with tumor progression in pancreatic ductal adenocarcinoma. Oncotarget 2015;6:37028-42. [Crossref] [PubMed]
- Shi Y, Zhai H, Wang X, et al. Ribosomal proteins S13 and L23 promote multidrug resistance in gastric cancer cells by suppressing drug-induced apoptosis. Exp Cell Res 2004;296:337-46. [Crossref] [PubMed]
- Knoll M, Macher-Goeppinger S, Kopitz J, et al. The ribosomal protein S6 in renal cell carcinoma: functional relevance and potential as biomarker. Oncotarget 2016;7:418-32. [Crossref] [PubMed]
- Wang Z, Hou J, Lu L, et al. Small ribosomal protein subunit S7 suppresses ovarian tumorigenesis through regulation of the PI3K/AKT and MAPK pathways. PLoS One 2013;8:e79117 [Crossref] [PubMed]