The lncRNAs MIR600HG and TSPOAP1-AS1 may potentially act as biomarkers for predicting pancreatic cancer
Introduction
Pancreatic cancer (PC) is one of the deadliest cancers; prognosis remains very poor, with a five-year net survival of less than 6% in the USA (1). Despite significant advances in molecular diagnostics and therapeutics in clinical practice, progress in understanding PC carcinogenesis remains insufficient. Lack of early detection methods and strategies leads to poor prognosis. Consequently, specific prognostic biomarkers are desperately needed in PC, and of great significance for early diagnosis and effective treatment.
Long non-coding RNAs (lncRNAs) are transcripts with more than 200 nucleotides (nt) that exhibit limited or no protein-coding capacity (2). Multiple reports revealed that lncRNAs participate in the regulation of a range of biological processes, including genomic imprinting, transcriptional regulation, and cell death, growth and differentiation, controlling apoptosis, chromatin modification and inflammatory pathologies, which play critical roles in cancer development (3). Many lncRNAs were found to be differentially expressed in various tumor types (4-10). Due to the ease of detection and high specificity of lncRNAs in tissues and body fluids, they are considered useful biomarkers for disease diagnosis and prognosis, as well as potential therapeutic targets for these types of diseases (11-16). LncRNAs have been used as biomarkers for the diagnosis and prognosis of prostate, breast, gastric, pancreatic, ovarian, and bladder cancers, as well as many other malignancies (3). The above studies demonstrated that lncRNAs could serve as non-invasive biomarkers for clinical application in PC.
It is urgent to identify a specific molecular biomarker that can determine the prognosis of PC patients and help provide adequate treatment as early as possible. This study aimed to assess differentially expressed lncRNAs (DElncRNAs) profile differences between PC and adjacent normal pancreas samples, in order to identify potential lncRNA biomarkers using The Cancer Genome Atlas (TCGA), which could help determine prognosis in PC. In addition, the functional analysis results of lncRNAs could provide insights into the effects of lncRNAs on the biological processes of PC, opening new horizons for further assessment of PC formation and development.
Methods
The dataset of PC patients
LncRNA expression and the corresponding clinical data of PC patients were obtained from the TCGA database (http://portal.gdc.cancer.gov/repository) (as of December, 2018). In the current study, a total of 177 PC patients and 182 samples were enrolled.
Identification of differentially expressed lncRNAs in PC
Gene expression profiling data of 178 cancer tissue samples and 4 normal tissue specimens were downloaded. The edgeR package (version 3.8) from Bioconductor was used to analyze DElncRNAs between normal and pancreatic tumor tissues. The criteria for screening the DElncRNAs were padj <0.05 and |log2FoldChange| >1. Volcano plots were used to visually display DElncRNAs between the upregulated and downregulated groups. Unsupervised hierarchical cluster analysis was performed based on the retrieved DElncRNAs with the pheatmap package (https://cran.r-project.org/web/packages/pheatmap/index.html) in R. All analyses were performed with R version 3.5.1.
Cox regression analysis and establishment of a prognostic risk score model
To determine associations of DElncRNAs with overall survival (OS) by univariate Cox regression analysis, lncRNAs with of P<0.001 in univariate analysis were considered candidate variables and entered into a stepwise multiple Cox regression analysis to identify covariates with independent prognostic values for patient survival. Then, a prognostic risk model was established based on the Akaike Information Criterion (AIC). A mathematical formula was computed as Risk score =−0.4768× MIR600HG expression −0.4021× TSPOAP1-AS1 expression, to predict the risk score of each patient based on multiple Cox regression analysis. Based on the median risk score, PC patients were divided into the high- and low-risk groups. Kaplan-Meier survival curves for cases predicted with low and high risk were generate respectively. Then, in order to test the sensitivity and specificity of the prognostic risk scoring model for 3-year and 5-year survival rates in pancreatic cancer patients, ROC curve analysis was performed. The area under the ROC curve (AUC) was derived as reported previously.
Univariate and multiple Cox regression analyses were used to confirm that the prognostic value of the 2-lncRNA signature is independent of clinical features
Univariate and multiple Cox regression analyses were performed with OS as the dependent variable and the lncRNA signature and other conventional clinical factors as independent variables. For clinical features with P value <0.01 in Cox regression analysis, stratification analysis was further carried out to assess whether the predictive index of the prognostic risk score for the lncRNA signature is independent of other conventional clinical factors, with P<0.05 considered statistically significant.
Functional predictions of lncRNAs by bioinformatics enrichment analysis
With the Limma package in R, a lncRNA-mRNA co-expression network was established. Pearson correlation analysis was used to calculate the co-expression relationship between the two prognostic lncRNAs and each protein-coding gene. Protein-coding genes (PCGs) with |Pearson correlation coefficient| >0.40 were considered lncRNAs-related PCGs. KEGG and GO analyses of the co-expressed PCGs with the two lncRNAs were performed to predict the biological functions of lncRNAs using the DAVID Bioinformatics Tool version 6.8 (david.ncifcrf.gov/). P<0.05 was set as the cutoff value in both GO and KEGG functional analyses.
Results
Clinical characteristics of the PC patients
LncRNA expression profiles in 178 PC and 4 normal tissue samples were used to screen for DElncRNAs. A total of 177 pancreatic cancer patients were assessed for clinical data, including age, gender, grade, stage, and vital status (Table 1).
Table 1
Characteristic | Number of patients | % |
---|---|---|
Age (years) | ||
<60 | 54 | 30.50 |
≥60 | 123 | 69.49 |
Gender | ||
Male | 97 | 54.80 |
Female | 80 | 45.20 |
Grade | ||
1 | 31 | 17.51 |
2 | 92 | 51.98 |
3 | 49 | 27.68 |
4 | 2 | 1.13 |
X | 3 | 1.69 |
Stage | ||
I | 21 | 11.86 |
IIA | 28 | 19.04 |
IIB | 117 | 66.10 |
III | 3 | 1.69 |
IV | 4 | 2.26 |
Vital status | ||
Alive | 119 | 67.23 |
Dead | 58 | 32.77 |
Differentially expressed lncRNAs in PC
A total of 178 DElncRNAs were screened between pancreatic tumor and normal tissues from the TCGA database, including 9 (3.3%) upregulated and 169 (96.7%) downregulated. The DElncRNAs between the two groups were visualized with a volcanic plot (Figure 1). Unsupervised hierarchical clustering analysis was performed, and the results demonstrated that the normal tissues could be distinguished from PC samples according to DElncRNA expression levels (Figure 2).
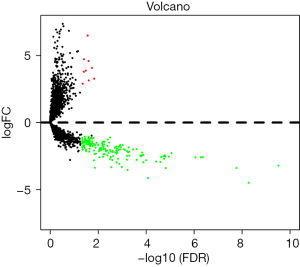
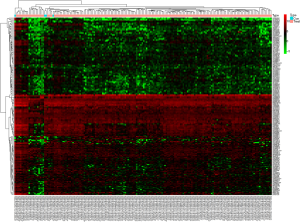
Establishment of a two-lncRNA signature associated with PC patient survival
By univariate Cox regression analysis, a set of 7 lncRNAs were considered to be associated with patient survival in PC (P<0.01). Then, the 7 lncRNAs were assessed in stepwise multiple Cox regression analysis. Finally, two-lncRNA (TSPOAP1.AS1 and MIR600HG) were identified (Table 2). We performed a risk score analysis of both lncRNAs to determine a risk score for each patient. These two lncRNAs were then used to establish a formula to assess the risk of prognosis as Risk score = (−0.4768×MIR600HG expression) + (−0.4021 × TSPOAP1-AS1 expression). This two-lncRNA signature was closely associated with low risk. In order to assess a risk score for each patient, the cases were divided into the high (88 cases) and low (89 cases) risk groups, based on the cutoff median risk score (Figures 3,4). OS was significantly prolonged in the low risk group compared with the high-risk group (P<0.0001). Kaplan-Meier survival curves are shown in Figure 5. The risk heatmap of the two-lncRNA signature’s expression profiles revealed that with increasing risk score, MIR600HG expression decreased, as well as TSPOAP1-AS1 levels; meanwhile, the risk score was negatively correlated with the levels of the two-lncRNA of the signature (Figure 6). A time-dependent ROC curve was used to assess the ability of the risk score to predict 3- and 5-year survival rates (Figures 7,8). The results showed that for 3-year survival, the AUC of the lncRNA prognostic model was 0.751; while an AUC of 0.792 was found for 5-year survival. These results indicated that the two-lncRNA signature in PC could improve the prediction of patient survival.
Table 2
Ensemble ID | LncRNA | Chromosome | Coefficient | Hazard ratio | P value |
---|---|---|---|---|---|
ENSG00000236901 | MIR600HG | Chromosome 9: 123,109,494-123,115,477 | −0.476767698 | 0.62078672 | 0.0006402 |
ENSG00000265148 | TSPOAP1-AS1 | Chromosome 17: 58,325,450-58,415,766 | −0.402126387 | 0.6688962 | 0.00099195 |
lncRNAs, long non-coding RNAs.
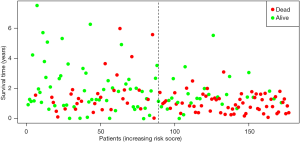
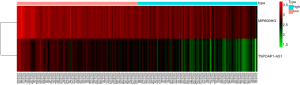
Prognostic value of the two-lncRNA signature in association with clinical factors
Univariate analysis was performed to test the prognostic value of the two-lncRNA signature in OS. The results showed that gender (HR=0.811; P=0.436) and age (HR=1.504; P=0.174) were not correlated with OS in patients with PC. Multiple Cox regression analysis indicated that risk score (HR=3.616; P=0.000) was an independent prognostic factor of PC, and the higher the risk score, the poorer the prognosis (Table 3).
Table 3
Variables | Univariate analysis | Multivariate analysis | |||
---|---|---|---|---|---|
HR (95% CI) | P value | HR (95% CI) | P value | ||
Gender (female/male) | 0.811 (0.479–1.374) | 0.436 | |||
Age (≥60/<60) | 1.504 (0.835–2.710) | 0.174 | |||
Grade (G3+G4/G1+G2) | 2.006 (1.172–3.433) | 0.011 | 1.558 (0.900–2.696) | 0.113 | |
Stage (I-IIA/IIB-IV) | 2.499 (1.287–4.854) | 0.007 | 1.691 (0.851–3.360) | 0.134 | |
Risk score (high/low) | 4.612 (2.517–8.448) | 0 | 3.616 (1.967–6.647) | 0 |
lncRNA, long non-coding RNA; HR, hazard ratio; CI, confidence interval.
Prediction of lncRNAs functions
We performed GO and KEGG functional enrichment analyses on co-expressed PCGs to reveal the potential functions of the two prognostic lncRNAs. The results indicated that co-expressed PCGs were enriched in 72 GO BP terms, which mainly focused on cell adhesion, protein binding and various nucleoside binding. KEGG functional annotation suggested that PCGs were significantly enriched in 41KEGG pathways, including multiple signal pathways, hematopoietic cell lineage, primary immunodeficiency, and cell adhesion molecules. The top 30 GO/KEGG terms are presented in Figures 9,10.
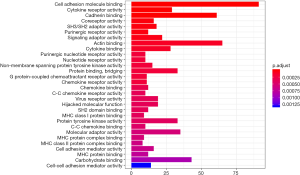
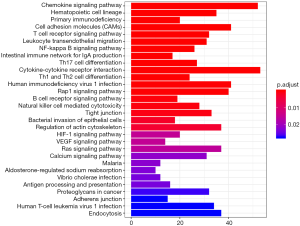
Discussion
PC is a highly lethal cancer. It caused 432,242 deaths (4.5% of all deaths caused by cancer) in 2018, ranking as the 11th among the world’s most common cancers, according to GLOBOCAN 2018 (17). PC is known to have poor prognosis. In order to improve prognosis, there is an urgent need to develop tools for diagnosing PC at an early stage.
In the past few years, increasing attention has been paid to the regulating effects of lncRNAs on the etiologies of several diseases, including cancer (18,19). Their levels are indicative of disease severity. Deregulated expression of lncRNAs is strongly linked to the development of various tumors, and can be effectively detected in body fluids and tumor tissues from patients in various cancers. It has been reported that many lncRNAs are stable in body fluids and can be detected in plasma and urine samples from cancer patients (11). Aberrant lncRNA expression and mutations in many cancers might be a major cause of tumorigenesis, with close association with metastasis and tumor stage (20,21). All these features make lncRNAs an attractive choice for application as noninvasive biomarkers of therapeutic targets in cancer. We hope to identify PC-associated lncRNAs that could be effectively used as diagnostic and prognostic biomarkers in clinical practice.
In this study, lncRNA expression data and related clinical characteristics were collected from the TCGA database, and 178 DElncRNAs between pancreatic cancer and normal tissues were initially confirmed. By univariate and multiple Cox regression analyses, we established a two-lncRNA (TSPOAP1.AS1 and MIR600HG) model to predict the clinical results of PC. Using this predictive model, a significant gap was observed between the high-risk and low-risk patient groups in survival. As shown in the risk heat map of the two-lncRNA signature’s expression profiles, with increasing risk score, MIR600HG and TSPOAP1-AS1 amounts decreased, and the risk score was negatively correlated with expression levels of these the two-lncRNA. An AUC of 0.751 for 3-year survival and AUC of 0.792 for 5-year survival were obtained by ROC analysis, indicating that the lncRNA signature model possesses high sensitivity and specificity. Meanwhile, univariate Cox regression analysis showed that the characteristics of the two-lncRNA signature were not associated with clinical characteristics that affect overall survival, including gender, age, and tumor stage. Further stratified analysis of the remaining clinicopathological factors showed that risk score was an independent prognostic factor of PC, and the higher the risk score, the poorer the prognosis. This may provide additional references for clinicians in selecting improved personalized and effective treatments for patients with different survival risks. Finally, by constructing a lncRNA-mRNA co-expression network, we screened PCGs associated with lncRNAs for functional enrichment analysis by GO and KEGG. In GO enrichment analysis, we found that lncRNA functions were mainly focused on cell adhesion, protein binding and various receptor activities. KEGG analysis revealed associations with chemokine signaling pathways, hematopoietic lineage, and primary immunodeficiency.
Since our work is mainly based on the TCGA database, the limitation of this study is that the number of normal pancreatic tissues is relatively small. In our future work, we will integrate data from multiple databases for further analysis and subgroup analysis. In addition, we will collect patient samples and further verify our conclusions through experiments.
In conclusion, this study assessed the expression profiles of lncRNAs from the TCGA database, and identified a two-lncRNA (TSPOAP1.AS1, MIR600HG) signature as a cancer biomarker predicting 3-/5-year survival in PC patients. These two-lncRNA signature may become a new prognostic indicator for predicting clinical outcome. However, further experimental studies are required to assess the expression levels of the two-lncRNA signature in PC tissues and cells. In addition, the prognostic value of this two-lncRNA signature should be assessed and validated with lncRNA expression profiles from other databases. Few studies have explored the underlying mechanisms of lncRNAs, by using the small interfering RNA transfection technology to observe tumor growth and cell apoptosis, further confirming the function of lncRNAs in the development and progression of PC.
Acknowledgments
Funding: None.
Footnote
Conflicts of Interest: Both authors have completed the ICMJE uniform disclosure form (available at http://dx.doi.org/10.21037/tcr.2019.12.09). The authors have no conflicts of interest to declare.
Ethical Statement: The authors are accountable for all aspects of the work in ensuring that questions related to the accuracy or integrity of any part of the work are appropriately investigated and resolved. The study was conducted in accordance with the Declaration of Helsinki (as revised in 2013).
Open Access Statement: This is an Open Access article distributed in accordance with the Creative Commons Attribution-NonCommercial-NoDerivs 4.0 International License (CC BY-NC-ND 4.0), which permits the non-commercial replication and distribution of the article with the strict proviso that no changes or edits are made and the original work is properly cited (including links to both the formal publication through the relevant DOI and the license). See: https://creativecommons.org/licenses/by-nc-nd/4.0/.
References
- Kamisawa T, Wood LD, Itoi T, et al. Pancreatic cancer. Lancet 2016;388:73-85. [Crossref] [PubMed]
- Silva A, Bullock M, Calin G. The Clinical Relevance of Long Non-Coding RNAs in Cancer. Cancers (Basel) 2015;7:2169-82. [Crossref] [PubMed]
- Bolha L, Ravnik-Glavac M, Glavač D. Long Noncoding RNAs as Biomarkers in Cancer. Dis Markers 2017;2017:7243968. [Crossref] [PubMed]
- Yu DJ, Zhong M, Wang WL. Long noncoding RNA CASC15 is upregulated in non-small cell lung cancer and facilitates cell proliferation and metastasis via targeting miR-130b-3p. Eur Rev Med Pharmacol Sci 2019;23:7943-9. [PubMed]
- Wang LL, Zhang L, Cui XF. Downregulation of long noncoding RNA LINC01419 inhibits cell migration, invasion, and tumor growth and promotes autophagy via inactivation of the PI3K/Akt1/mTOR pathway in gastric cancer. Ther Adv Med Oncol 2019;11:1758835919874651. [Crossref] [PubMed]
- Li J, Xu Q, Wang W, et al. MIR100HG: a credible prognostic biomarker and an oncogenic lncRNA in gastric cancer. Biosci Rep 2019;39:BSR20190171. [Crossref] [PubMed]
- Lee YJ, Oh H, Kim E, et al. Long noncoding RNA HOTTIP overexpression: A potential prognostic biomarker in prostate cancer. Pathol Res Pract 2019;215:152649. [Crossref] [PubMed]
- Kumar P, Khadirnaikar S, Shukla SK. PILAR1, a novel prognostic LncRNA, reveals the presence of a unique subtype of lung adenocarcinoma patients with KEAP1 mutations. Gene 2019;691:167-75. [Crossref] [PubMed]
- Gao H, Yin Y, Qian A, et al. LncRNA LINC00974 Upregulates CDK6 to Promote Cell Cycle Progression in Gastric Carcinoma. Cancer Biother Radiopharm 2019; [Crossref] [PubMed]
- Thomas PB, Seim I, Jeffery PL, et al. The long non-coding RNA GHSROS facilitates breast cancer cell migration and orthotopic xenograft tumour growth. Int J Oncol 2019;55:1223-36. [PubMed]
- Shi T, Gao G, Cao Y. Long Noncoding RNAs as Novel Biomarkers Have a Promising Future in Cancer Diagnostics. Dis Markers 2016;2016:9085195. [Crossref] [PubMed]
- Barth DA, Slaby O, Klec C, et al. Current Concepts of Non-Coding RNAs in the Pathogenesis of Non-Clear Cell Renal Cell Carcinoma. Cancers(Basel) 2019;11:1580. [Crossref] [PubMed]
- Tamang S, Acharya V, Roy D, et al. SNHG12: An LncRNA as a Potential Therapeutic Target and Biomarker for Human Cancer. Front Oncol 2019;9:901. [PubMed]
- Wu C, Tang ZY, Chen HY, et al. High-expression of lncRNA CEBPA-AS1 promotes liver cancer progression. Eur Rev Med Pharmacol Sci 2019;23:8295-302. [PubMed]
- Yan PH, Wang L, Chen H, et al. LncRNA RUNX1-IT1 inhibits proliferation and promotes apoptosis of hepatocellular carcinoma by regulating MAPK pathways. Eur Rev Med Pharmacol Sci 2019;23:8287-94. [PubMed]
- Yin Q, Shen X, Cui X, et al. Elevated serum lncRNA TUG1 levels are a potential diagnostic biomarker of multiple myeloma. Exp Hematol 2019; [Crossref] [PubMed]
- Bray F, Ferlay J, Soerjomataram I, et al. Global cancer statistics 2018: GLOBOCAN estimates of incidence and mortality worldwide for 36 cancers in 185 countries. CA Cancer J Clin 2018;68:394-424. [Crossref] [PubMed]
- Bhan A, Soleimani M, Mandal SS. Long Noncoding RNA and Cancer: A New Paradigm. Cancer Res 2017;77:3965-81. [Crossref] [PubMed]
- Akhade VS, Pal D, Kanduri C. Long Noncoding RNA: Genome Organization and Mechanism of Action. Adv Exp Med Biol 2017;1008:47-74. [Crossref] [PubMed]
- Bartonicek N, Maag JL, Dinger ME. Long noncoding RNAs in cancer: mechanisms of action and technological advancements. Mol Cancer 2016;15:43. [Crossref] [PubMed]
- Vitiello M, Tuccoli A, Poliseno L. Long non-coding RNAs in cancer: implications for personalized therapy. Cell Oncol (Dordr) 2015;38:17-28. [Crossref] [PubMed]