Identification of lapatinib sensitivity-related genes by integrative functional module analysis
Introduction
Globally, gastric carcinoma (GC) is one of the most commonly encountered malignancies and is the second highest contributor to cancer mortality (1-3). Although therapy for GC has seen progressive developments in the last decade, especially with chemotherapeutic drugs, the survival rate of GC patients remains unsatisfactory (4,5). Therefore, methods to enhance the efficacy of chemotherapeutic drugs for GC patients are needed.
Lapatinib, which is orally bioavailable, is a small-molecule reversible inhibitor of both epidermal growth factor receptor (EGFR) and human epidermal growth factor receptor-2 (HER2) tyrosine kinases (6). Although lapatinib has found a purpose as a treatment of trastuzumab-resistant, HER2-positive advanced GC, many patients eventually develop resistance to or do not respond to the drugs (7,8), often as a consequence of the activation of alternative signaling pathways (9,10).
In view of this, it is critical to be able to predict which signaling pathways could be activated to mediate resistance to lapatinib treatment, which could help identify combination or secondary treatments to mitigate this resistance (11,12). However, it has been difficult to identify the key pathways and proteins involved in lapatinib resistance. Park et al. found that FOXO1, through negative crosstalk, acts as a bridge between HER2 and MET signaling pathways, and plays a core role in the regulation of acquired lapatinib resistance (13). Meanwhile, inhibition of Hes1 enhances lapatinib sensitivity in GC sphere-forming cells (14), and Kim et al. demonstrated that testican-1-mediated epithelial-mesenchymal transition signaling results in acquired resistance to lapatinib in GC (15).
However, these pathways and proteins linked to lapatinib resistance were identified by previous publications and experiments, and a comprehensive method to investigate the pathways or signaling networks involved in lapatinib resistance is needed. Fortunately, these limitations can be alleviated by the advancements that have been made with high-throughput experimental and bioinformatics databases. Exploration of the relationship between drug sensitivity and genomic data was facilitated by NCI-60 cell line panel and associated drug screens (16). Meanwhile, the furtherance of CellMiner has enabled the efficient retrieval of both genomic data and the activity reports of ~20,000 chemical compounds across the NCI-60 cell line (17).
In the this study, we sought to explore the relationship between sensitivity genes and lapatinib response in GC by integrating genomic, drug sensitivity, and tumor transcriptomic data. We constructed a lapatinib sensitivity negative network (LSN) and a lapatinib sensitivity positive network (LSP), and identified modules within each of these networks. We then evaluated these modules using the extent of enrichment of dysregulated genes in GC. BCAR1 gene, which was present in module 4 and was enriched in the Rap1 signaling pathway, was selected for further analyses. Our data indicated that knockdown of BCAR1 can reverse lapatinib resistance in SUN216/LR cells.
Methods
Network construction
Construction of a functional linkage network for lapatinib sensitivity was performed with 164 positively related genes and 117 negatively related genes, which were extracted from the CellMiner database (version 2.2) using “NCI-60 Analysis Tools”. The potential positively and negatively related genes involved in lapatinib sensitivity were obtained by calculating the Pearson’s correlation coefficient between the mRNA expression profiles of the NCI-60 cell lines and the 50% growth inhibitory concentration values. The values were determined by the developmental therapeutics program (DTP) drug screen with CellMiner, which is a platform which facilitates the efficient retrieval of gene transcript data and the activity reports for chemical compounds (17).
The protein-protein interaction (PPI) network was derived from The Human Protein Reference Database (HPRD), which contains information manually harvested by expert biologists who read, interpret, and analyze data from published literature (18). For the current study, HPRD release 9, which contains 37,039 PPIs among 9,465 proteins found in humans, was downloaded. Negatively related genes and positively related genes were projected into the HPRD PPI network and then incorporated with their first neighbors to construct the LSN and LSP networks, respectively. Visualizations were performed using Cytoscape software (19).
Identification of differentially expressed genes (DEGs) in gastric cancer
RNA-seq v2 datasets derived from GC were obtained from The Cancer Genome Atlas (TCGA) (http://tcga-data.nci.nih.gov/). The raw read counts were extracted for every exon from exon quantification file in the TCGA level 3 dataset. The reads per kilobase of transcript per million read mapped (RPKM) value of each gene could then be calculated by applying the formula as follows:
EC constitutes the mapped read counts of all exons of 1 gene, while SC equates to the mapped read counts of all exons in a sample. The number “l” is the total length of all exons in a gene. GENCODE (V14) was used to obtain the exon structures of genes via download. We identified the up- and down-regulated genes using the fold-change method. Genes with fold change value greater than 2 or less than one-half were considered up- or down-regulated, respectively.
Identification and evaluation of functional modules
Network modules were identified using the MCODE plug-in in Cytoscape. In the lapatinib sensitivity negatively correlated LSN and LSP networks, we identified 2 and 9 modules, respectively. Up-regulated genes in cancers tend to inhibit anti-cancer drug sensitivity while down-regulated genes show the opposite effect. To identify the functional modules of lapatinib sensitivity, we assessed the significance of the overlap between up-regulated genes in GC and each module in the LSN network using a hypergeometric test. Modules were considered significant with a p-value of less than 0.05. Similarly, we also tested the significance of the overlap between down-regulated genes in GC and each module in the LSP network. The hypergeometric test used assumes that if the entire genome has a total of m genes, in which t is part of a functional module, and up-regulated or down-regulated genes in GC are a total of n genes, in which r is part of the functional modules in LSN or LSP, then the p-value can be worked out to assess that drug’s enrichment significance using the following formula:
Using the above-described method, we calculated the enrichment significance between up- and down-regulated genes and each functional module.
Lastly, we identified 2 significant modules in the LSP: module 4 (P=0.024) and module 9 (P=0.019). To investigate the functions of these modules, we worked to enrich pathways using the Database for Annotation, Visualization, and Integrated Discovery (DAVID) tool (20).
Cell culture
The GC cell line, SUN216, was purchased from the American Type Culture Collection (Manassas, VA, USA). Culturing of the cells took place in Dulbecco’s Modified Eagle medium (DMEM, Invitrogen, USA) with 10% fetal bovine serum (FBS; Hyclone, USA), 50 U/mL penicillin, and 50 µg/mL streptomycin (Invitrogen, CA, USA). All cells were kept at 37 °C in a humidified incubator at 5% CO2.
Establishment of lapatinib-resistant SUN216 cell lines
Increasing doses of lapatinib (Shanghai Aladdin Biochemical Technology Co., Ltd., Shanghai, China) were consistently applied to the SUN216 cell line for about 12 weeks. The dosing began at 5 µM and increased to 10 µM after 4 weeks, 15 µM after another 4 weeks, and remained at 15 µM for the last 4 weeks. The resistant SUN216 cell line that we established was then maintained in DMEM medium with 10% (v/v) FBS and 10 µM lapatinib.
Cell transfection
The sequence of the BCAR1-siRNA (stQ0002578-1) was obtained from RiboBio (Guangzhou, China). SUN216 cells were seeded in 60-mm dishes 24 hours before to transfection. BCAR1-siRNA or control-siRNA was transfected into cells with Lipofectamine 3000 (Invitrogen) in serum-free media according to experiments request. Five hours following the procedure, the media was switched to complete media. Harvesting of cell lysates was carried out 48 hours after transfection.
CCK-8 cell viability assay
SUN216 cells transfected with control-siRNA or BCAR1-siRNA were seeded into 96-well plates at a density of 5×103 cells/per well and culturing took place for 4 days with varying concentrations of lapatinib (0, 5, 10, 20, 50 µg/mL). The viability of cells was determined with the Cell Counting Kit-8 (CCK-8, Promega, Kumamoto, Japan) at days 0, 2, and 4.
Colony formation assay
Approximately 8×102 SUN216 cells transfected with control-siRNA or BCAR1-siRNA were placed in 60 mm dishes. After 10 days had passed, colonies were stained with 0.1% crystal violet in 20% methanol for 15 min, before photographs were taken and the number of visible colonies was counted.
Acridine orange/ethidium bromide (AO/EB) staining
Acridine orange (20 µL) and ethidium bromide (20 µL) mixing solution were placed for 5 minutes (Solarbio of Biotechnology, Beijing, China) in 1 well with 2 mL PBS at room temperature. Fluorescence microscopy (×200) allowed cellular morphological changes to be studied. The following formula was used to figure out the percentage of apoptotic cells: apoptotic rate (%) = apoptotic cells/all cells counted.
Transwell assay
Transwell chambers were used to test the migration (Cat No. 3422, Corning Costar, Cambridge, MA, USA) and invasion (Cat No. 356234, BD Biosciences, San Jose, CA, USA). All procedures were conducted, as described by Zhang et al. (21).
Western blotting
As described previously, Western blotting was implemented (22). Protein (60–80 µg) was separated by 10% SDS-PAGE and then transfected to membranes for 1 hour. Membrane blocking was achieved with 5% dry nonfat milk for 2 hours and subsequent incubation with BCAR1 (Cat: ab3183, Abcom, USA) overnight at 4 °C. An electrochemiluminescence detection system (Thermo Fisher Scientific, CA, USA) was employed to detect signals.
Data analysis
Data are expressed as mean ± SD. Student's non-paired t-test or a one-way analysis of variance (ANOVA), followed by Tukey’s test for multiple group comparisons, was applied for statistical analysis. P<0.05 signified a significant difference. Graph Pad Prism 5.0 and SPSS 19.0 were used to analyze data.
Results
Construction of lapatinib sensitivity-related functional linkage networks
To identify the positively and negatively related genes involved in lapatinib sensitivity, we obtained lapatinib sensitivity-related genes from the CellMiner database by using the default cutoff. A total of 164 positively related genes and 117 negatively related genes were obtained and considered as core genes. LSP and LSN networks were constructed with the proteins encoded by these genes. Linkages between proteins were extracted from the HPRD database. Finally, the LSP and LSN networks were constructed using core genes and their first neighbors. There were 581 nodes and 574 edges in the LSP (Figure 1A), and 438 nodes and 414 edges in the LSN (Figure 1B).
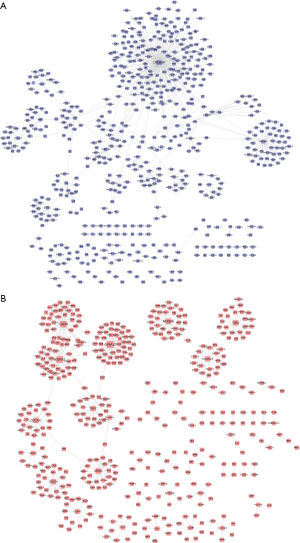
Evaluation of functional modules in the LSP and LSN networks
Genes in similar biological processes, such as signaling pathways, tend to carry out similar functions, and therefore interact with each other as groups with high density in interaction networks (22). These entities are classed as functional modules, in which members are functionally linked to each other. In the LSP and LSN networks, we identified 2 and 9 of these modules, respectively. We then identified the significant modules in the LSP and LSN networks and evaluated these modules by the extent of enrichment of dysregulated genes in GC. We finally identified 2 significant modules in the LSN: module 4 (P=0.024) and module 8 (P=0.019) (Figure 2). There were no significant modules in the LSP network. Pathway enrichment analysis revealed the involvement of module 4 genes in the neuroactive ligand-receptor interaction network (hsa04080), the cAMP signaling pathway (hsa04024), and the Rap1 signaling pathway (hsa04015) (Figure 3A). Module 8 was involved in the TNF signaling pathway (hsa04668) and the MAPK signaling pathway (hsa04010) (Figure 3B). Among these, we chose BCAR1 in the Rap1 signaling pathway in module 4 for further investigation, because of its higher degree in module 4 (Figure 2A).
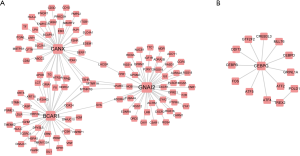
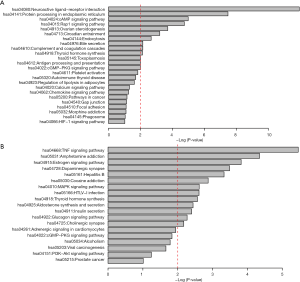
Knockdown of BCAR1 sensitizes GC cells to lapatinib treatment
We investigated whether knockdown of BCAR1 could sensitize GC cells to lapatinib treatment. We first established lapatinib-resistant SUN216 cells (SUN216/LR) after 4 months of culturing with lapatinib. SUN216/LR cells had a similar cell morphology as SUN216 cells (Figure 4A). Using the CCK-8 assay, we determined the IC50 value of SUN216 cells to be 0.24 nM. However, a much higher IC50 value (>10 nM) was determined for SUN216/LR cells (Figure 4B). We examined the levels of BCAR1 in SUN216/LR cells. Compared with normal SUN216 cells, SUN216/LR cells had significantly higher BCAR1 levels (Figure 4C). To investigate whether BCAR1 regulates lapatinib resistance, we first tested the expression after transfection with BCAR1-siRNA by Western blotting. Our data showed that the expression of BCAR1 was down-regulated after the transfection with BCAR1-siRNA (Figure 4D). BCAR1 was knocked down in SUN216 cells using BCAR1-siRNA. Using the CCK-8 assay, we demonstrated that the IC50 value of lapatinib in SUN216/LR cells was markedly reduced upon BCAR1 knockdown (Figure 4E). A clonogenic assay exhibited fewer colonies of SUN216/LR cells after BCAR1 knockdown and lapatinib treatment (Figure 4F). Next, using acridine orange/ethidium bromide (AO/EB) staining, we tested the cell apoptosis after transfection with BCAR1-siRNA and found the cell apoptosis rate to be more up-regulated in the BCAR1-si group than in the control group (Figure 4G). We also tested migration and invasion (Figure S1), and our data showed that the silencing of BCAR1 could inhibit cell migration and invasion. These data indicate that knockdown of BCAR1 reverses lapatinib resistance in SUN216/LR cells.
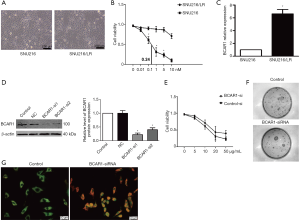
Discussion
Acquired resistance is a major cause of poor clinical outcomes in cancer patients undergoing chemotherapy (23). Identification of genes of sensitivity to anti-cancer drugs would help to optimize the anti-tumor efficacy and lower toxicity of chemotherapeutic drugs like (24). Herein, we present a computational procedure for identifying sensitivity genes for lapatinib treatment based on multiple functional gene modules, which we verified using an in vitro model.
We first constructed 2 functional linkage networks, LSN and LSP, and mined the functional modules from the 2 networks. We then investigated the significance of the modules in the 2 networks. Finally, 2 modules in the LSP network, module 4 and module 8 were identified. A Kyoto Encyclopedia of Genes and Genomes (KEGG) pathway analysis showed the involvement of genes in module 4 in pathways, including the neuroactive ligand-receptor interaction network (hsa04080) and protein processing in the endoplasmic reticulum (hsa04141). Some tyrosine kinase inhibitors, such as lapatinib, produce reactive oxygen species-dependent endoplasmic reticulum stress and rapid cytosolic calcium mobilization from endoplasmic reticulum stores (25-27). Genes in module 8 were involved in the TNF signaling pathway (hsa04668), the estrogen signaling pathway (hsa04915), and the MAP kinase signaling pathway (hsa04010).
Among these pathways, the role the Rap1 signaling pathway takes on in cancer was recently noted (28,29). Rap1 signaling is a major player in the control of cell-cell and cell-matrix interactions through its regulation in the function of integrins and other adhesion molecules in a variety of cell types (30,31). Rap1 is also a regulator of MAP kinase activity in a fashion which is highly conditional on cell type context (32). In this pathway, we found that one of the annotated genes, BCAR1, encodes a scaffold protein that acts as a central pivot in cellular signaling. It paves the way for multi-protein complexes that regulate diverse cellular processes, such as migration, invasion, proliferation, and survival, to assemble. BCAR1 takes part in the signal transduction of major oncogenic kinases such as Abl, FAK, and Src. Consequently, the overexpression of BCAR1 in a diverse range of malignancies, including cancer of the breast, lung, liver, and brain, has been demonstrated, and a link between BCAR1 overexpression and adverse features in these organs had been made (33,34). Earlier evidence also suggested that BCAR1 is a factor in prostate cancer progression (35), as its overexpression was linked to an adverse tumor phenotype and biochemical relapse in 3 studies analyzing 110, 130, and 242 prostate cancer specimens (36). However, there are no studies concerning the relationship between BCAR1 and lapatinib in GC. Therefore, we assessed the role of BCAR1 in lapatinib sensitivity by using lapatinib-resistant SUN216 cells in vitro. We found that BCAR1 was up-regulated in SUN216/LR cells compared to SUN216 cells. Interestingly, knockdown of BCAR1 dramatically reduced the IC50 of lapatinib in SUN216/LR cells. Thus, we discovered that BCAR1 might contribute to lapatinib resistance in GC.
In this study, we efficiently identified the crucial modules that were highly related to lapatinib sensitivity in GC by using a topological network method. BCAR1 was identified as a potentially critical gene playing a role in lapatinib sensitivity, and experiments confirmed that BCAR1 might contribute to lapatinib resistance in GC. These results provide further insight into the molecular basis of lapatinib sensitivity and could offer novel strategies for the future treatment of GC. However, different GC cell lines, as well as in vivo experiments, are required to confirm the function of BCAR1 in the lapatinib resistance in GC.
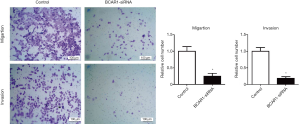
Acknowledgments
Funding: None.
Footnote
Conflicts of Interest: All authors have completed the ICMJE uniform disclosure form (available at http://dx.doi.org/10.21037/tcr.2020.01.30). The authors have no conflicts of interest to declare.
Ethical Statement: The authors are accountable for all aspects of the work in ensuring that questions related to the accuracy or integrity of any part of the work are appropriately investigated and resolved. The data comes from databases, so ethical reviews and informed consent are exempt. The study was conducted in accordance with the Declaration of Helsinki (as revised in 2013).
Open Access Statement: This is an Open Access article distributed in accordance with the Creative Commons Attribution-NonCommercial-NoDerivs 4.0 International License (CC BY-NC-ND 4.0), which permits the non-commercial replication and distribution of the article with the strict proviso that no changes or edits are made and the original work is properly cited (including links to both the formal publication through the relevant DOI and the license). See: https://creativecommons.org/licenses/by-nc-nd/4.0/.
References
- Yuasa Y. Control of gut differentiation and intestinal-type gastric carcinogenesis. Nat Rev Cancer 2003;3:592-600. [Crossref] [PubMed]
- Panani AD. Cytogenetic and molecular aspects of gastric cancer: clinical implications. Cancer Lett 2008;266:99-115. [Crossref] [PubMed]
- Mali AV, Padhye SB, Anant S, et al. Anticancer and antimetastatic potential of enterolactone: Clinical, preclinical and mechanistic perspectives. Eur J Pharmacol 2019;852:107-24. [Crossref] [PubMed]
- Song Y, Ye M, Zhou J, et al. Targeting E-cadherin expression with small molecules for digestive cancer treatment. Am J Transl Res 2019;11:3932-44. [PubMed]
- Neuzillet C, Rousseau B, Kocher H, et al. Unravelling the pharmacologic opportunities and future directions for targeted therapies in gastro-intestinal cancers Part 1: GI carcinomas. Pharmacol Ther 2017;174:145-72. [Crossref] [PubMed]
- Voigtlaender M, Schneider-Merck T, Trepel M. Lapatinib. Recent Results Cancer Res 2018;211:19-44. [Crossref] [PubMed]
- Xuhong JC, Qi XW, Zhang Y, et al. Mechanism, safety and efficacy of three tyrosine kinase inhibitors lapatinib, neratinib and pyrotinib in HER2-positive breast cancer. Am J Cancer Res 2019;9:2103-19. [PubMed]
- Menderes G, Lopez S, Han C, et al. Mechanisms of resistance to HER2-targeted therapies in HER2-amplified uterine serous carcinoma, and strategies to overcome it. Discov Med 2018;26:39-50. [PubMed]
- Engelman JA, Zejnullahu K, Mitsudomi T, et al. MET amplification leads to gefitinib resistance in lung cancer by activating ERBB3 signaling. Science 2007;316:1039-43. [Crossref] [PubMed]
- Vernieri C, Milano M, Brambilla M, et al. Resistance mechanisms to anti-HER2 therapies in HER2-positive breast cancer: Current knowledge, new research directions and therapeutic perspectives. Crit Rev Oncol Hematol 2019;139:53-66. [Crossref] [PubMed]
- Corso S, Ghiso E, Cepero V, et al. Activation of HER family members in gastric carcinoma cells mediates resistance to MET inhibition. Mol Cancer 2010;9:121. [Crossref] [PubMed]
- Simiczyjew A, Pietraszek-Gremplewicz K, Dratkiewicz E, et al. Combination of Selected MET and EGFR Inhibitors Decreases Melanoma Cells' Invasive Abilities. Front Pharmacol 2019;10:1116. [Crossref] [PubMed]
- Park J, Choi Y, Ko YS, et al. FOXO1 Suppression is a Determinant of Acquired Lapatinib-Resistance in HER2-Positive Gastric Cancer Cells Through MET Upregulation. Cancer Res Treat 2018;50:239-54. [Crossref] [PubMed]
- Li L, Li Y, Wang L, et al. Inhibition of Hes1 enhances lapatinib sensitivity in gastric cancer sphere-forming cells. Oncol Lett 2017;14:3989-96. [Crossref] [PubMed]
- Kim HP, Han SW, Song SH, et al. Testican-1-mediated epithelial-mesenchymal transition signaling confers acquired resistance to lapatinib in HER2-positive gastric cancer. Oncogene 2014;33:3334-41. [Crossref] [PubMed]
- Liu H, D'Andrade P, Fulmer-Smentek S, et al. mRNA and microRNA expression profiles of the NCI-60 integrated with drug activities. Mol Cancer Ther 2010;9:1080-91. [Crossref] [PubMed]
- Reinhold WC, Sunshine M, Liu H, et al. CellMiner: a web-based suite of genomic and pharmacologic tools to explore transcript and drug patterns in the NCI-60 cell line set. Cancer Res 2012;72:3499-511. [Crossref] [PubMed]
- Keshava Prasad TS, Goel R, Kandasamy K, et al. Human Protein Reference Database--2009 update. Nucleic Acids Res 2009;37:D767-72. [Crossref] [PubMed]
- Shannon P, Markiel A, Ozier O, et al. Cytoscape: a software environment for integrated models of biomolecular interaction networks. Genome Res 2003;13:2498-504. [Crossref] [PubMed]
- Huang da W. Sherman BT, Lempicki RA. Systematic and integrative analysis of large gene lists using DAVID bioinformatics resources. Nat Protoc 2009;4:44-57. [Crossref] [PubMed]
- Zhang P, Li S, Lv C, et al. BPI-9016M, a c-Met inhibitor, suppresses tumor cell growth, migration and invasion of lung adenocarcinoma via miR203-DKK1. Theranostics 2018;8:5890-902. [Crossref] [PubMed]
- Oulas A, Minadakis G, Zachariou M, et al. Systems Bioinformatics: increasing precision of computational diagnostics and therapeutics through network-based approaches. Brief Bioinform 2019;20:806-24. [Crossref] [PubMed]
- Wongsirisin P, Limpakan Yamada S, Yodkeeree S, et al. Association of DNA Repair and Drug Transporter in Relation to Chemosensitivity in Primary Culture of Thai Gastric Cancer Patients. Biol Pharm Bull 2018;41:360-7. [Crossref] [PubMed]
- Shan K, Liu C, Liu BH, et al. Circular Noncoding RNA HIPK3 Mediates Retinal Vascular Dysfunction in Diabetes Mellitus. Circulation 2017;136:1629-42. [Crossref] [PubMed]
- Cruickshanks N, Tang Y, Booth L, et al. Lapatinib and obatoclax kill breast cancer cells through reactive oxygen species-dependent endoplasmic reticulum stress. Mol Pharmacol 2012;82:1217-29. [Crossref] [PubMed]
- Carloni S, Fabbri F, Brigliadori G, et al. Tyrosine kinase inhibitors gefitinib, lapatinib and sorafenib induce rapid functional alterations in breast cancer cells. Curr Cancer Drug Targets 2010;10:422-31. [Crossref] [PubMed]
- Mahmud Z, Gomes AR, Lee HJ, et al. EP300 and SIRT1/6 Co-Regulate Lapatinib Sensitivity Via Modulating FOXO3-Acetylation and Activity in Breast Cancer. Cancers (Basel) 2019; [Crossref] [PubMed]
- Chen YL, Ge GJ, Qi C, et al. A five-gene signature may predict sunitinib sensitivity and serve as prognostic biomarkers for renal cell carcinoma. J Cell Physiol 2018;233:6649-60. [Crossref] [PubMed]
- Li Y, Liu X, Tang H, et al. RNA Sequencing Uncovers Molecular Mechanisms Underlying Pathological Complete Response to Chemotherapy in Patients with Operable Breast Cancer. Med Sci Monit 2017;23:4321-7. [Crossref] [PubMed]
- Kooistra MR, Dube N, Bos JL. Rap1: a key regulator in cell-cell junction formation. J Cell Sci 2007;120:17-22. [Crossref] [PubMed]
- Shah S, Brock EJ, Ji K, et al. Ras and Rap1: A tale of two GTPases. Semin Cancer Biol 2019;54:29-39. [Crossref] [PubMed]
- Breckler M, Berthouze M, Laurent AC, et al. Rap-linked cAMP signaling Epac proteins: compartmentation, functioning and disease implications. Cell Signal 2011;23:1257-66. [Crossref] [PubMed]
- Huang W, Deng B, Wang RW, et al. BCAR1 protein plays important roles in carcinogenesis and predicts poor prognosis in non-small-cell lung cancer. PLoS One 2012;7:e36124. [Crossref] [PubMed]
- Wallez Y, Riedl SJ, Pasquale EB. Association of the breast cancer antiestrogen resistance protein 1 (BCAR1) and BCAR3 scaffolding proteins in cell signaling and antiestrogen resistance. J Biol Chem 2014;289:10431-44. [Crossref] [PubMed]
- Fromont G, Vallancien G, Validire P, et al. BCAR1 expression in prostate cancer: association with 16q23 LOH status, tumor progression and EGFR/KAI1 staining. Prostate 2007;67:268-73. [Crossref] [PubMed]
- Heumann A, Heinemann N, Hube-Magg C, et al. High BCAR1 expression is associated with early PSA recurrence in ERG negative prostate cancer. BMC Cancer 2018;18:37. [Crossref] [PubMed]