A prognostic nomogram for pancreatic ductal adenocarcinoma patients’ all-cause survival in a Surveillance, Epidemiology, and End Results analysis
Introduction
Pancreatic cancer is a common malignant tumor of the digestive tract that mainly occurs in the head of the pancreas, but in rare cases can also occur in its body and tail. Most malignancies of the pancreas are adenocarcinoma. Rare pancreatic tumors include neuroendocrine tumors, which secrete hormones such as insulin or glucagon, and acinar cancers, which release digestive enzymes into the circulation. Ductal adenocarcinoma, which accounts for 80–90% of pancreatic cancers, mainly comprises glands with varying degrees of ductal structures and abundant fibrous stroma (1).
Pancreatic cancer is one of the leading causes of cancer deaths in developed countries and one of the deadliest malignancies worldwide (2). Pancreatic ductal adenocarcinoma (PDAC) accounts for more than 90% of all pancreatic tumors, and is a destructive malignant tumor with a poor prognosis. The 1-year survival rate of all disease stages is about 18%, 90% of patients die within 1 year after a diagnosis, and the 5-year survival rate is reportedly only 9% (3,4). The presence of positive resection margins, poor tumor differentiation, large tumors, and positive lymph nodes all portend a poor prognosis. Adjuvant therapy is also important in the treatment of PDAC. Clinicians worldwide are beginning to delay adjuvant radiotherapy until adequate adjuvant chemotherapy is available to prevent metastatic disease, so only those who are disease free at 4–6 months after systemic therapy will receive adjuvant radiotherapy (5).
The pathological staging system proposed by the American Joint Committee on Cancer (AJCC) is widely adopted for the clinical diagnosis, treatment, and research of pancreatic cancer both home and abroad, and has become the gold standard for malignant tumor staging. The AJCC updates the staging system every 6–8 years, and released the latest staging of the eighth edition in October 2016 (6,7). The primary tumor (T) staging and lymph node (N) staging of pancreatic cancer were both redefined and subdivided in the eighth edition (8). Although the eighth edition adds more non-anatomical factors than the sixth edition, and makes a further effort to create a more contemporary “personalized approach” (9), this version is still difficult to achieve individual predictions. In view of the high mortality rate of pancreatic cancer, new prognostic tools are needed to improve the accuracy of predicting the all-cause survival of pancreatic cancer patients.
A nomogram is based on multivariate regression analysis and predicts clinical outcomes or the probability of certain events based on multiple variables. In addition to the AJCC staging system, other factors such as age at diagnosis, sex, surgery status, and some inconclusive adjuvant therapies could also provide information that is useful for determining patient prognoses (10). The aim of the present study was to establish a comprehensive prognostic model, and to compare its predictive ability with that of the AJCC 8th edition staging system model. Our nomogram can help clinicians to predict the 1-, 2-, and 3-year all-cause survival probabilities of patients more comprehensively and on an individual basis, and hence has considerable clinical value.
Methods
Source of patients
We used SEER*Stat software (version 8.3.6, https://seer.cancer.gov/) to view patient data in the latest version of the Surveillance, Epidemiology, and End Results (SEER) database (November 2018). The SEER database includes population data categorized by age at diagnosis, sex, race, year of diagnosis, and geographic region (including SEER registries and counties). We selected demographic variables including age at diagnosis, sex, race, and marital status. We applied certain other conditions to extract the desired PDAC patients from the SEER database (11), including the “Infiltrating duct carcinoma, NOS” (8500/3) histological subtype code of the International Classification of Diseases for Oncology (ICD-O-3), while the primary site of PDAC was categorized using the following ICD-O-3 codes: “C25.0-Head of pancreas”, “C25.1-Body of pancreas”, “C25.2-Tail of pancreas”, and “other”. Moreover, the surgery status, adjuvant radiotherapy status, and adjuvant chemotherapy status were categorized into “yes” and “no/unknown”. We also selected pathology grade and regional nodes examined (RNE) as pathological indicators. The data of the eighth edition is not available in the SEER database. We chose the AJCC stage based on the sixth edition of the derived AJCC stage group because the number of cases in seventh edition is much smaller than that in sixth edition, and the staging system for both editions has not changed at all. We extracted relevant variables from the SEER database: tumor size, regional lymph node metastasis, and distant metastasis to perform the conversion from sixth edition to eighth edition. We compared these variables with TNM staging of the sixth edition of AJCC, and further summarized the eighth edition of AJCC staging system for all patients. In addition, we added the year of diagnosis variable to control the effect of time span on the results. Our study included only patients diagnosed with PDAC for the first time, and patients with other primary tumors were excluded. Patients with missing or unknown survival data were also excluded. In the end, a total of 4,229 patients were excluded, and the specific exclusion process is shown in Figure 1.
The application of these criteria identified 5,097 patients in the SEER database between 2004 and 2016. Obtaining informed patient consent is unnecessary for data obtained from the SEER database that does not include personal identifying information.
Statistical analysis
Statistical analysis was performed using IBM SPSS Statistics software (version 23.0, SPSS, Chicago, IL, USA) and R software (version 3.4.1; http://www.Rproject.org). Results were considered statistically significant with the two-sided probability value was <0.05. R software was used to randomly divided all 5,097 patients in a 7-to-3 ratio, forming a training cohort (n=3,567, 70%) for constructing a prognostic nomogram and a validation cohort (n=1,530, 30%) for evaluating the constructed nomogram. The Log-rank test was also used to determine that the differences between the groups were not statistically significant. The age at diagnosis and RNE were expressed as the median value and interquartile range, while categorical variables were expressed as percentages by SPSS software. The rest analyses were done by the R software. A multivariate Cox regression model was used to determine factors related to survival, the stepwise selection regression was used (P<0.1). The following variables were considered: age at diagnosis, marital status, primary site, pathology grade, RNE, AJCC stage, surgery status, adjuvant radiotherapy status, and adjuvant chemotherapy status. All of these variables except race and primary site were entered into the Cox regression model. All construction and verification methods were done in R 3.4.1 with several packages, such as survival, rms, foreign, survival, survival receiver operating characteristic (ROC), nricens, and decision curve packages.
Nomogram construction and evaluation
Based on the prognostic factors selected in the above analysis, we constructed a nomogram to predict the 1-, 2-, and 3-year all-cause survival probabilities of PDAC patients using the training cohort. We then verified the performance of the established nomogram using the validation cohort. We first used the concordance index (C-index) and the area under the time-dependent ROC curve (AUC) to evaluate the discrimination of the nomogram. The C-index is used to estimate the probability that predicted results are consistent with actual observed results. It is suitable for evaluating the accuracy of various prediction models, especially survival models. While the ROC curve is a comprehensive indicator of both sensitivity and specificity. The performance of the nomogram was tested by analyzing the AUC. The AUC is more suitable to verify the differentiation of models, but it does not pay attention to risk prediction, and it is more suitable for verifying the predictive power of binary regression model. The calibration plot is an index to evaluate the accuracy of disease risk model in predicting the probability of an individual’s future outcomes. We used calibration plots to evaluate the consistency between the predictions made by the model and actual outcomes. We then compared the discriminatory power of the predictive model by calculating the relative net reclassification index (NRI) and the integrated discrimination improvement (IDI), which are suitable indicators for quantitatively comparing the degree of improvement in the prediction accuracy between new and old models (12). When we want to compare whether the predictive power of the model is improved after introducing new indicators into the model, it is sometimes difficult for the newly added indicators to significantly improve the AUC, and the increment of AUC is not obvious. In this case, NRI pays more attention to the change in the number of subjects that the two models correctly classify at a given cutoff point, and is often used to compare the accuracy of the prediction power of the two models. IDI considered the situation of different tangential points, which could be used to reflect the overall improvement of the model. Although AUC also considered overall improvement, the improvement of AUC was not easy to explain in clinical practice, IDI therefore made up for the defects of AUC, which could vividly show the proportion of the research objects to be accurately re-judged. Finally, decision-curve analysis (DCA) is a simple, novel method of evaluating predictive models. It can help clinicians determine how to achieve the optimal net benefit (NB) (13), and so we used DCA to evaluate the clinical utility of the new predictive model.
Results
Demographic and clinicopathological characteristics
We selected 5,097 patients with PDAC cancer from 2004 to 2016 in the SEER database, and classified 70% of them (n=3,567) into the training cohort and 30% (n=1,530) into the validation cohort using R software. In terms of demographic characteristics, the numbers of males and females were roughly equal. The median age at diagnosis was 66 years (interquartile range, 59–74 years) in the training cohort and 66 years (interquartile range, 59–74 years) in the validation cohort. Most of the patients were white (81.3% and 80.7%) and married (84.8% and 85.6%). In terms of pathology and clinical treatment, the median number of RNE was 15 (interquartile range, 9–21) in the training cohort and 15 (interquartile range, 9–22) in the validation cohort. Most patients were in pathology grade II (53.7% and 55.6%, in the training and validation cohorts, respectively), and most patients were in AJCC 8th edition stage II (44.1% and 45.6%). In most cases the primary site of PDAC was the head of the pancreas (75.0% and 75.6%), and various proportions of the patients had received surgery (99.1% and 98.7%), adjuvant radiotherapy (33.6% and 32.9%), and adjuvant chemotherapy (69.0% and 68.0%). Table 1 lists the demographic and the clinicopathological characteristics of all patients in the training and validation cohorts.
Table 1
Variable | Training cohort | Validation cohort |
---|---|---|
Number of patients, n (%) | 3,567 (70.0) | 1,530 (30.0) |
Age at diagnosis, median [IQR] | 66 [59–74] | 66 [59–74] |
Sex, n (%) | ||
Male | 1,763 (49.4) | 786 (51.4) |
Female | 1,804 (50.6) | 744 (48.6) |
Race, n (%) | ||
White | 2,899 (81.3) | 1,235 (80.7) |
Black | 339 (9.5) | 149 (9.7) |
Other | 329 (9.2) | 146 (9.5) |
Marital status, n (%) | ||
Married | 3,025 (84.8) | 1,309 (85.6) |
Unmarried | 433 (12.1) | 177 (11.6) |
Other | 109 (3.1) | 44 (2.9) |
Primary site, n (%) | ||
C25.0-Head of pancreas | 2,676 (75.0) | 1,156 (75.6) |
C25.1-Body of pancreas | 255 (7.1) | 107 (7.0) |
C25.2-Tail of pancreas | 327 (9.2) | 137 (9.0) |
Other | 309 (8.7) | 130 (8.5) |
Pathology grade, n (%) | ||
I | 364 (10.2) | 150 (9.8) |
II | 1,915 (53.7) | 850 (55.6) |
III | 1,260 (35.3) | 519 (33.9) |
IV | 28 (0.8) | 11 (0.7) |
RNE, median [IQR] | 15 [9–21] | 15 [9–22] |
AJCC, n (%) | ||
I | 846 (23.7) | 323 (21.1) |
II | 1,574 (44.1) | 697 (45.6) |
III | 974 (27.3) | 434 (28.4) |
IV | 173 (4.9) | 76 (5.0) |
Surgery, n (%) | ||
Yes | 3,536 (99.1) | 1,510 (98.7) |
No/unknown | 31 (0.9) | 20 (1.3) |
Adjuvant radiotherapy, n (%) | ||
Yes | 1,197 (33.6) | 504 (32.9) |
No/unknown | 2,370 (66.4) | 1,026 (67.1) |
Adjuvant chemotherapy, n (%) | ||
Yes | 2,461 (69.0) | 1,041 (68.0) |
No/unknown | 1,106 (31.0) | 489 (32.0) |
RNE, regional nodes examined; AJCC, American Joint Committee on Cancer; IQR, interquartile range.
Prognostic factors in the Cox regression analysis
The Cox regression used the following variables: age at diagnosis, sex, race, marital status, year of diagnosis, primary site, pathology grade, RNE, AJCC stage, surgery status, adjuvant radiotherapy status, and adjuvant chemotherapy status. Cox stepwise regression analysis showed no difference in the prognosis for PDAC in different years of diagnosis, and data on the age at diagnosis, marital status, primary site, pathology grade, RNE, AJCC stage, surgery status, adjuvant radiotherapy status, and adjuvant chemotherapy status were entered into multivariate Cox regression analyses. The Cox regression analysis revealed the following significant prognostic risk factors: age at diagnosis [hazard ratio (HR) =1.004, P=0.039], unmarried (HR =1.156 vs. married, P=0.013), primary site with the head of the pancreas had a worse prognosis than the body and tail of the pancreas, pathology grade II (HR =1.446 vs. pathology grade I, P<0.001), pathology grade III (HR =1.943 vs. pathology grade I, P<0.001), and pathology grade IV (HR =2.778 vs. pathology grade I, P<0.001), AJCC stage II (HR =1.581 vs. AJCC stage I, P<0.001), AJCC stage III (HR =2.478 vs. AJCC stage I, P<0.001), and AJCC stage IV (HR =3.442 vs. AJCC stage I, P<0.001). In particular, we found that RNE, receiving surgery, adjuvant radiotherapy, and adjuvant chemotherapy were protective factors for surviving PDAC: RNE (HR =0.986, P<0.001), no/unknown surgery status (HR =2.146, P<0.001), no/unknown adjuvant radiotherapy status (HR =1.081, P=0.083), and no/unknown adjuvant chemotherapy status (HR =1.815, P<0.001). Table 2 presents the variables selected in the multivariate Cox regression analysis.
Table 2
Variable | HR | 95% CI | P value |
---|---|---|---|
Age at diagnosis | 1.004 | 1.000–1.008 | 0.039* |
Marital | |||
Married | Reference | ||
Unmarried | 1.156 | 1.031–1.295 | 0.013* |
Unknown | 0.936 | 0.746–1.176 | 0.572 |
Primary site | |||
C25.0-Head of pancreas | Reference | ||
C25.1-Body of pancreas | 0.963 | 0.829–1.120 | 0.627 |
C25.2-Tail of pancreas | 0.877 | 0.766–1.004 | 0.058 |
Other | 1.126 | 0.988–1.283 | 0.075 |
Pathology grade | |||
I | Reference | ||
II | 1.446 | 1.259–1.660 | 0.000*** |
III | 1.943 | 1.686–2.240 | 0.000*** |
IV | 2.778 | 1.850–4.172 | 0.000*** |
RNE | 0.986 | 0.982–0.991 | 0.000*** |
AJCC | |||
I | Reference | ||
II | 1.581 | 1.428–1.750 | 0.000*** |
III | 2.478 | 2.213–2.774 | 0.000*** |
IV | 3.442 | 2.867–4.133 | 0.000*** |
Surgery | |||
Yes | Reference | ||
No/unknown | 2.146 | 1.468–3.138 | 0.000*** |
Adjuvant radiotherapy | |||
Yes | Reference | ||
No/unknown | 1.081 | 0.990–1.181 | 0.083 |
Adjuvant chemotherapy | |||
Yes | Reference | ||
No/unknown | 1.815 | 1.658–1.986 | 0.000*** |
*, P<0.05; ***, P<0.001. HR, hazard ratio; RNE, regional nodes examined; AJCC, American Joint Committee on Cancer.
Construction of the nomogram
We established a nomogram that included the important independent factors selected in the multivariate Cox regression analysis. The constructed nomogram can predict the 1-, 2-, and 3-year all-cause survival probabilities based on the values of the variables listed above. The nomogram is used by first scoring each factor according to its degree of influence, and then adding the scores to obtain the total score, which is used to determine the predicted 1-, 2-, and 3-year all-cause survival probabilities (Figure 2). The developed nomogram indicates that the AJCC stage has the greatest impact, followed by the pathology grade, RNE, surgery status, adjuvant chemotherapy status, age at diagnosis, primary site, marital status, and finally the adjuvant radiotherapy status.
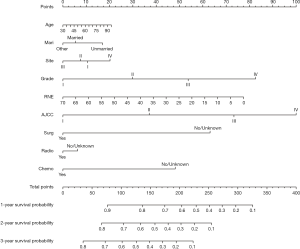
Evaluation of the nomogram
We used the C-index to evaluate the efficacy of the nomogram, and found that it was higher (0.668 for the training cohort and 0.670 for the validation cohort) than those for the AJCC staging system (0.590 and 0.578, respectively). Moreover, the ROC curves for the training cohort indicated that the AUCs were much larger for the nomogram (0.734 at 1 year, 0.699 at 2 years, and 0.703 at 3 years) than for the AJCC staging system (0.618, 0.622, and 0.646, respectively). Similarly, the ROC curves for the validation cohort indicated that the AUCs were significant larger for the nomogram (0.732 at 1 year, 0.699 at 2 years, and 0.704 at 3 years) than for the AJCC staging system (0.588, 0.621, and 0.654, respectively) (Figure 3).
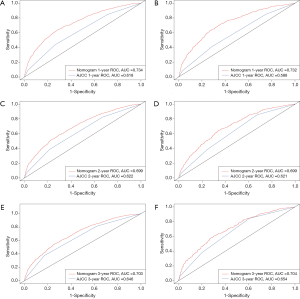
The NRI and IDI are more sensitive than the C-index. The NRI values at 1, 2, and 3 years of follow-up were 0.547 (95% CI: 0.481–0.610), 0.411 (95% CI: 0.365–0.485), and 0.375 (95% CI: 0.320–0.462), respectively, in the training cohort, and 0.607 (95% CI: 0.491–0.703), 0.443 (95% CI: 0.344–0.550), and 0.354 (95% CI: 0.208–0.475) in the validation cohort. These values indicate that the nomogram provided greatly improved predictive performance. Similarly, the IDI values at 1, 2, and 3 years were 0.057, 0.068, and 0.063, respectively, in the training cohort (P<0.001), and 0.065, 0.082, and 0.076 in the validation cohort (P<0.001). These findings also suggest that the predictive power of the new model is superior to that of the old model.
The calibration curves of the nomogram for 1-, 2-, and 3-year all-cause survival probabilities were close to the dotted lines in both the training and validation cohorts, indicating that the model was well calibrated (Figure 4).
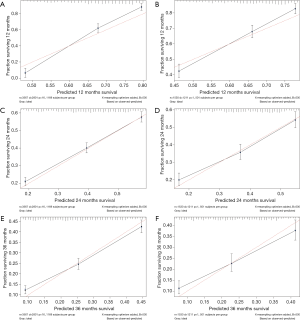
In the DCA, the curves for the two models were above the extreme curves; moreover, the DCA curves for the nomogram were significantly higher than those of the AJCC staging system in both the training and validation cohorts (Figure 5). This indicates that the NB and clinical effectiveness were greater for the nomogram than for the AJCC 8th edition staging system in terms of the 1-, 2-, and 3-year all-cause survival probabilities.
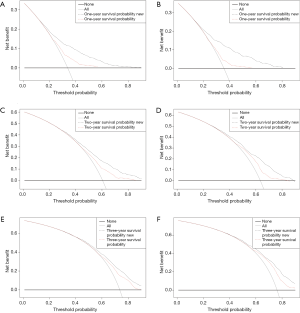
Discussion
Pancreatic cancer is one of the leading causes of cancer deaths and one of the deadliest malignancies worldwide. The changing demographics and morbidity and mortality of the average annual percentage changes mean that the significance of pancreatic cancer is predicted to increase relative to breast cancer, prostate cancer, and colorectal cancer by becoming the second leading cause of cancer-related deaths by 2030 (14). Pancreatic cancer has the lowest 5-year relative survival rate among cancers reported by the American Cancer Society, at 9%. Surgery is currently thought to the only useful treatment option for PDAC, but fewer than 20% of patients are eligible for surgical tumor removal (4,15). Some studies have found evidence of a survival benefit for adjuvant chemotherapy and adjuvant radiotherapy (16,17), which therefore warrants further attention.
PDAC is associated with a median survival of 6 months after the diagnosis, which represents the worst prognosis of all solid tumors (18). This situation prompted the present search for a comprehensive prognosis model that combines population statistics variables such as age at diagnosis, sex, and marital status, as well as the surgery status, adjuvant radiotherapy status, and adjuvant chemotherapy status. The existing AJCC 8th edition staging system can be used to predict the prognosis of pancreatic cancer patients, but greater progress may require incorporating complex methods such as new biomarkers (19). It also does not include demographic characteristics, treatments, or other important factors. A nomogram is a useful risk assessment tool based on multivariate regression analysis to predict certain clinical outcomes or the probability of certain events based on the values of multiple variables. A nomogram has the particular advantage of being able to personalize the survival probability of patients with specific tumors, which furnishes it with great value in clinical practice (20,21).
No previous studies have established a comprehensive nomogram of PDAC including demographics, clinicopathological features, or treatment approaches, and compared it with the latest AJCC 8th edition staging system. The nomogram model constructed in this study included the surgery status, adjuvant chemotherapy status, and adjuvant radiotherapy status, and we have demonstrated that it can reliably predict the effect of various types of treatment on the prognosis of PDAC. Improving adjuvant therapies in PDAC patients is expected to yield greater improvements in their prognosis (22-24), and we hope that our new nomogram will help clinicians to implement personalized predictions to facilitate personalized prognosis assessment and make more-effective clinical decisions.
The multivariate Cox regression analysis performed in this study revealed that age at diagnosis, marriage status, primary site, pathology grade, RNE, AJCC stage, surgery status, adjuvant radiotherapy status, and adjuvant chemotherapy status significantly impact the survival of PDAC patients, and we have used this information to establish and validate a nomogram for predicting the 1, 2, and 3 years all-cause survival probabilities. To determine whether our prognostic model is superior to the traditional AJCC 8th edition staging system, we used the C-index, AUC, NRI, IDI, calibration plots, and DCA to evaluate the performance of the nomogram. These parameters were analyzed from the three aspects of differentiation ability, clinical effectiveness, and calibration in order to comprehensively evaluate the practical significance of the nomogram.
The differentiation ability of the model was assessed using four indexes: C-index, AUC, NRI, and IDI (25-27). The results showed that our model has good discrimination ability, with C-index values were much higher than those of the AJCC staging system. As shown in Figure 2, the ROC curves for 1-, 2-, and 3-year all-cause survival indicated that the nomogram model had a better AUC and discrimination ability than the AJCC 8th edition staging system in both the training and validation cohorts.
The NRI and IDI were calculated to quantify the improvement achieved by utilizing the nomogram in a more comprehensive and multilevel way. The NRI can be used to compare the predictive ability of the nomogram model with that of the AJCC 8th edition staging system model after adding other indicators. The NRI showed that the predictive ability of the new model was improved after the addition of the new index, and the proportions of correct classifications increased by 50.7%, 42.3%, and 34.8% for 1, 2, and 3 years, respectively (all P<0.001) in the validation cohort. The IDI analyzed the overall improvement of the nomogram, and showed that the nomogram provides improvements in predictive ability compared with the old model of 4.2%, 4.8%, and 4.2% for 1, 2, and 3 years, respectively (all P<0.001). These positive results for the nomogram further demonstrated its superior performance and good discrimination.
The calibration performance of the model was assessed by analyzing calibration plots. The dotted lines in Figure 3 represent the actual situation. For the 1-, 2-, and 3-year predictions, the line for the model is very close to the dotted line and there is a uniform distribution of prediction points, which indicates that the nomogram was well calibrated and had a good discrimination ability in both the training and verification cohorts.
We used DCA to assess the clinical effectiveness of the model. Some researchers have suggested that DCA is a suitable method for evaluating alternative diagnostic and prognostic strategies, and has advantages over other commonly used measures and techniques (28-30). The horizontal coordinate of the DCA graph is the threshold probability (Pt), while the vertical coordinate is the NB after the benefit is subtracted from the disadvantage. The present results show that over a large range of Pt values, the NB of the new predictive model was higher than that of the AJCC staging system, indicating that the Pt range of the new model is relatively large and relatively safe. In the validation cohort, the 1-year DCA curve of the new model was superior to the AJCC staging system over a large range. In the presence of a high prediction probability, the 2-year DCA curve was also superior; however, the 3-year DCA curve showed that while the NB of the new model was large in cases of extremely high prediction probabilities, there was no difference between the two models in cases of low probability. This also demonstrates the inadequacy of the model in predicting long-term survival, but this may be largely attributable to the extremely low long-term survival rate of PDAC. In short, the current results show that the 1-, 2-, and 3-year DCA curves of our model provide greater NBs than the traditional AJCC 8th edition staging system (Figure 4).
In conclusion, our newly developed nomogram as a predictive model for PDAC patients provides significantly improved predictive performance and is more comprehensive than the latest AJCC 8th edition staging system. These findings support the use of our nomogram as a tool to help clinicians predict the all-cause survival time of individual patients.
This study was subject to some limitations. First, race was not included in the constructed nomogram, which may be due to most of the patients included in SEER database being white. Therefore, the number of patients of other races should be increased in future analyses. Second, data were not available on several factors such as genetic mutations or deletions, perineural invasion, lymphovascular invasion, obesity, and eating habits. More extensive investigations are therefore required to clarify the relationship between these factors and prognoses, and thereby establish an even better predictive model. Third, the SEER database contains retrospective data, and so various types of bias such as selection bias and information bias are inevitable. Then, some variables such as surgery, adjuvant radiotherapy, and adjuvant chemotherapy were dichotomized into “Yes” and “No/Unknown” in the SEER database. The unknown data may affect the authenticity of the results and generate information bias. Finally, this study only adopted internal validation, potentially leading to overfitting of the model. Further external validation is needed to provide more accurate assessments of the effectiveness of the model.
Conclusions
Nomograms are receiving greater attention and being applied more in medical research and clinical practice. We have developed and validated a prognosis nomogram for PDAC that is superior to the conventional AJCC 8th edition staging system. Our very realistic predictive model can help clinicians to better predict the 1-, 2-, and 3-year all-cause survival probabilities of PDAC patients.
Acknowledgments
Funding: The study was supported by
Footnote
Conflict of Interest: All authors have completed the ICMJE uniform disclosure form (available at http://dx.doi.org/10.21037/tcr-19-2962). The authors have no conflicts of interest to declare.
Ethical Statement: The authors are accountable for all aspects of the work in ensuring that questions related to the accuracy or integrity of any part of the work are appropriately investigated and resolved.
Availability of Data and Materials: SEER collects cancer incidence data from population-based cancer registries covering approximately 34.6 percent of the U.S. population. SEER releases a standard set of research data every spring based on the previous November’s submission of data from the registries. The data we used is based on the November 2018 submission. We accessed these through the SEER*Stat software with additional approvals.
Open Access Statement: This is an Open Access article distributed in accordance with the Creative Commons Attribution-NonCommercial-NoDerivs 4.0 International License (CC BY-NC-ND 4.0), which permits the non-commercial replication and distribution of the article with the strict proviso that no changes or edits are made and the original work is properly cited (including links to both the formal publication through the relevant DOI and the license). See: https://creativecommons.org/licenses/by-nc-nd/4.0/.
References
- Kleeff J, Korc M, Apte M, et al. Pancreatic cancer. Nat Rev Dis Primers 2016;2:16022. [Crossref] [PubMed]
- Ferlay J, Soerjomataram I, Dikshit R, et al. Cancer incidence and mortality worldwide: sources, methods and major patterns in GLOBOCAN 2012. Int J Cancer 2015;136:E359-86. [Crossref] [PubMed]
- GBD 2015 Mortality and Causes of Death Collaborators (2016). Global, regional, and national life expectancy, all-cause mortality, and cause-specific mortality for 249 causes of death, 1980-2015: a systematic analysis for the Global Burden of Disease Study 2015. Lancet 2016;388:1459-544. [Crossref] [PubMed]
- Siegel RL, Miller KD, Jemal A. Cancer statistics, 2020. CA Cancer J Clin 2020;70:7-30. [Crossref] [PubMed]
- Vincent A, Herman J, Schulick R, et al. Pancreatic cancer. Lancet 2011;378:607-20. [Crossref] [PubMed]
- Amin MB, Edge SB, Greene FL, et al, eds. AJCC Cancer Staging Manual. 8th edition. New York: Springer, 2017.
- O'Sullivan B, Brierley J, Byrd D, et al. The TNM classification of malignant tumours-towards common understanding and reasonable expectations. Lancet Oncol 2017;18:849-51. [Crossref] [PubMed]
- Kamarajah SK, Burns WR, Frankel TL, et al. Validation of the American Joint Commission on Cancer (AJCC) 8th Edition Staging System for Patients with Pancreatic Adenocarcinoma: A Surveillance, Epidemiology and End Results (SEER) Analysis. Ann Surg Oncol 2017;24:2023-30.
- Amin MB, Greene FL, Edge SB, et al. The Eighth Edition AJCC Cancer Staging Manual: Continuing to build a bridge from a population-based to a more "personalized" approach to cancer staging. CA Cancer J Clin 2017;67:93-9.
- Hidalgo M. Pancreatic cancer. N Engl J Med 2010;362:1605-17. [Crossref] [PubMed]
- Yang J, Liu QQ, Geng H, et al. SEER database application and data extraction methods and processes. Chinese Journal of Evidence-Based Cardiovascular Medicine 2018;10:781-4.
- Steyerberg EW, Vickers AJ, Cook NR, et al. Assessing the Performance of Prediction Models. Epidemiology 2010;21:128-38. [Crossref] [PubMed]
- Vickers AJ, Elkin EB. Decision curve analysis: a novel method for evaluating prediction models. Med Decis Making 2006;26:565-74. [Crossref] [PubMed]
- Rahib L, Smith BD, Aizenberg R, et al. Projecting cancer incidence and deaths to 2030: the unexpected burden of thyroid, liver, and pancreas cancers in the United States. Cancer Res 2014;74:2913-21. [Crossref] [PubMed]
- Spanknebel K, Conlon KC. Advances in the surgical management of pancreatic cancer. Cancer J 2001;7:312-23. [PubMed]
- Neoptolemos JP, Dunn JA, Stocken DD, et al. Adjuvant chemoradiotherapy and chemotherapy in resectable pancreatic cancer: a randomised controlled trial. Lancet 2001;358:1576-85. [Crossref] [PubMed]
- Gillen S, Schuster T, Meyer Zum Buschenfelde C, et al. Preoperative/neoadjuvant therapy in pancreatic cancer: a systematic review and meta-analysis of response and resection percentages. PLoS Med 2010;7:e1000267. [Crossref] [PubMed]
- Jemal A, Bray F, Center MM, et al. Global cancer statistics. CA Cancer J Clin 2011;61:69-90. [Crossref] [PubMed]
- van Roessel S, Kasumova GG, Verheij J, et al. International Validation of the Eighth Edition of the American Joint Committee on Cancer (AJCC) TNM Staging System in Patients With Resected Pancreatic Cancer. JAMA Surg 2018;153:e183617.
- Bevilacqua JL, Kattan MW, Fey JV, et al. Doctor, what are my chances of having a positive sentinel node? A validated nomogram for risk estimation. J Clin Oncol 2007;25:3670-9. [Crossref] [PubMed]
- Grimes DA. The nomogram epidemic: resurgence of a medical relic. Ann Intern Med 2008;149:273-5. [Crossref] [PubMed]
- Neoptolemos JP, Kleeff J, Michl P, et al. Therapeutic developments in pancreatic cancer: current and future perspectives. Nat Rev Gastroenterol Hepatol 2018;15:333-48. [Crossref] [PubMed]
- Burris HA 3rd, Moore MJ, Andersen J, et al. Improvements in survival and clinical benefit with gemcitabine as first-line therapy for patients with advanced pancreas cancer: a randomized trial. J Clin Oncol 1997;15:2403-13. [Crossref] [PubMed]
- Mancuso A, Calabro F, Sternberg CN. Current therapies and advances in the treatment of pancreatic cancer. Crit Rev Oncol Hematol 2006;58:231-41. [Crossref] [PubMed]
- Loupy A, Aubert O, Orandi BJ, et al. Prediction system for risk of allograft loss in patients receiving kidney transplants: international derivation and validation study. BMJ 2019;366:l4923. [Crossref] [PubMed]
- Pencina MJ, D'Agostino RB Sr, D'Agostino RB Jr, et al. Evaluating the added predictive ability of a new marker: from area under the ROC curve to reclassification and beyond. Stat Med 2008;27:157-72; discussion 207-12. [Crossref] [PubMed]
- Leslie WD, Schousboe JT, Lix LM. Towards better use of the net reclassification improvement (NRI) index. Osteoporos Int 2016;27:411-2. [Crossref] [PubMed]
- Vickers AJ, Cronin AM. Traditional statistical methods for evaluating prediction models are uninformative as to clinical value: towards a decision analytic framework. Semin Oncol 2010;37:31-8. [Crossref] [PubMed]
- Kerr KF, Brown MD, Zhu K, et al. Assessing the Clinical Impact of Risk Prediction Models With Decision Curves: Guidance for Correct Interpretation and Appropriate Use. J Clin Oncol 2016;34:2534-40. [Crossref] [PubMed]
- Van Calster B, Vickers AJ. Calibration of risk prediction models: impact on decision-analytic performance. Med Decis Making 2015;35:162-9. [Crossref] [PubMed]