Radiomics applied to lung cancer: a review
Introduction
Globally, lung cancer is the most frequent cause of death in men and the second most frequent cause amongst women in the USA (1). Past smoking patterns strongly influence actual cancer rates amongst both men and women and vary considerably due to geographical location, age, race, and socioeconomic status. There is a broad consensus that it is necessary to develop better biomarkers predicting treatment response or survival, in order to identify subgroups that would benefit from individualized treatment to improve the patient’s outcome.
Most tumours do not represent a homogenous entity; they are composed of multiple clonal sub-populations of cancer cells. This heterogeneous complex needs to be analysed in order to tailor the cancer care to the patient and their tumour. One way of characterizing the tumour is by extracting tumour tissue in a tumour biopsy, the tissue is then characterised using genomics based approaches. Although these approaches have successfully been used in clinical oncology, there are intrinsic limitations; repeated tumour biopsies increase the risk of complications for the patients, in particular in lung cancer and the results may vary depending on which part of the tumour is biopsied. These challenges can be addressed by medical imaging. Medical imaging is an important part of cancer care, it is essential to cancer staging and diagnosis. Unlike biopsies, it is noninvasive, three dimensional and provides information regarding the entire tumour.
Radiomics, first introduced by Lambin et al. (2) in 2012 and summarized in a two minute animation (http://youtu.be/Tq980GEVP0Y), is a process that converts standard of care images into minable high-dimensional data. The radiomics process can extract quantitative features from digital medical images, which can then be used to build descriptive and predictive models, linking the image features to the tumour’s gene-protein signatures or the tumour’s phenotype. As shown in recent studies, quantitative imaging features have a prognostic value and potential in predicting clinical outcomes or treatment monitoring in different cancer types (3-7). The aim of radiomics is to use these models, which can include biological or medical data, to help provide valuable diagnostic, prognostic or predictive information. radiomics aims to utilise the full potential of medical imaging by extracting and analysing large amounts of advanced quantitative imaging features, with high throughput from digital medical images, obtained with computed tomography (CT), positron emission tomography (PET) or magnetic resonance imaging (MRI). This in turn provides a more detailed quantification of tumour phenotypic characteristics describing its intensity, shape, texture, intra-tumour heterogeneity and in doing so it effectively converts medical images into a high dimensional minable feature space.
Precision oncology is the customization of cancer care where therapies and/or practices are being tailored to individual patients, with all of the information about the tumour characteristics that radiomics provides; it presents numerous new opportunities for precision medicine.
In this paper we will review the literature and discuss the potential advantages and pitfalls of radiomics in lung cancer.
Work flow of radiomics
The radiomics process consists of three steps: (I) image acquisition and volume segmentation; (II) feature extraction and storage; (III) signature development and validation on one or several datasets (Figure 1) (2,8,9). Each of these three steps poses unique challenges, which will be introduced below. Once a signature has been developed and is applied on a specific patient, the process is the same except that in step 3 the validated signature is used to determine the prognosis of the patient.
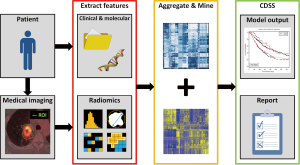
Image acquisition
Modern CT, PET and MRI scanners allow acquisition and reconstruction settings in a large range. Although this facilitates the subjective needs of the human expert, when the images are intended to be objectively characterized by a machine, these variations may create a bias that masks the true underlying biological characteristics. This phenomenon is well recognized in the field of radiomics and consequently, efforts are being made to standardize acquisition and reconstruction protocols. This advance in quantitative imaging is being led by several organizations or consortia such as the European Association of Nuclear Medicine (EANM), the QuIC-ConCePT project from the Innovative Medicine Initiative Joint Undertaking (IMIJU).
Volumes of interest
One of the central processes of radiomics is to identify one or several volumes of interest (the primary tumour, nodules, etc.) on diagnostic imaging. However, predictive value may lie in the detailed analysis of subvolumes within the tumour, also known as habitats. The heterogeneity within the tumour is due to particular combinations of blood flow, oedema, necrosis, and cell density, which creates a unique pathophysiology. Using radiomics, important additional information from these habitats as well as information from a normal healthy lung, can be extracted.
Segmentation
Segmentation or delineation plays a crucial role within radiomics, because the features that are generated depend on the segmented volumes. However, many tumours, as well as subvolumes, have ambiguous borders. This can lead to a significant inter-reader bias and low reproducibility when these volumes are manually delineated. Unfortunately, there is no universal automatic segmentation algorithm that can work for all medical images (10,11). Consequently, the consensus that emerges out of this debate is that the optimum reproducible segmentation can be obtained via semi-automatic segmentation, which consists of automatic segmentation followed by, if necessary, manual curation (12). The different image modalities have also their own segmentation methods. For CT, the region of interest (ROI) represents for example the gross tumor volume (GTV), while for PET the metabolic target volume (MTV) is segmented as ROI. The segmentation method also depends on the endpoint of the study. Cheebsumon et al. (13) showed that CT-based delineation was overestimated compared to pathology while PET-based tumour delineation methods provided maximum diameters in closer agreement with pathology. They also showed that for example the contrast-oriented methods seem to best suited for assessing tumour size, while an adaptive 70% threshold-based method is better for response monitoring (13).
Feature extraction
The essential part of radiomics is the high throughput extraction of quantitative image features that characterize the volumes of interest. The number of features is enormous, more than 1,000, and complex, and this leads to the risk of overfitting. Overfitting exists when a model is specifically and exclusively optimized for the training dataset and consequently performs poorly on new data. To avoid overfitting, the ratio of the number of evaluated features to the number of outcome events must be kept as low as possible. To offset this risk, there is a key process of feature reduction and ranking. In order to do so, one approach consists in looking at robustness and reproducibility of features in test-retest datasets. The information extracted from two datasets of images acquired within a small period of time (few minutes to few days) from a single patient cohort is called test-retest data and is highly advantageous for ranking features thanks to their reproducibility and stability. Therefore, when coupled with robust segmentation, test-retest data should be exploited whenever possible. Another complementary approach consists of identifying features that may be redundant, if they are for example highly correlated with one another. Groups of highly correlated features can in turn be reduced to one archetypal feature.
Model development and validation
Developing a model based on the calculated radiomics features can be data-driven or hypothesis driven. The data-driven approach makes no assumption about the meaning of individual features, therefore all features are treated with equal weight during model development, whereas the hypothesis-driven approach treats cluster features according to predefined information content and a clinical context. The best models start with a well-defined end-point, such as overall survival (OS), and ideally can accommodate non-radiomics features. Covariates that need to be taken into account include clinical, treatment and genomic data [age, histology, tumour-node-metastasis (TNM) stage, serum markers, chemotherapy, radiotherapy, dose, fractionation, treatment time, gene expression, mutation status, gene polymorphisms…]. This information is occasionally missing for some patients; therefore models should accommodate sparse data. It is necessary that the models be adequately validated with an external dataset, preferably from an external institute. If data from an external institute is not available, the available data must be split into a model training dataset and a validation dataset.
Model performance is often measured in terms of calibration and discrimination. Accurate models correctly discriminate between patients. This can be measured using the c-index or the area under the curve (AUC) of receiver operating characteristic (ROC) for censored data (14). On the other hand, the calibration is the association between observed outcomes and model prediction.
Analysis of the literature
Reviewing all the literature about radiomics in lung cancer has shown that radiomics can be useful in many fields, such as in the classification of nodules, in the description of the tumour, and it has already been shown to be a tool that can assess patient prognosis (3,15). All of the articles published to date, to the best of our knowledge, about radiomics in lung cancer have been placed in Table 1.
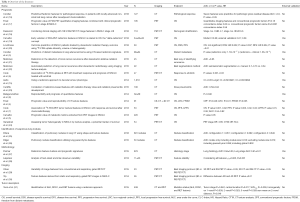
Full table
First of all, 16 articles in the table assess the prognostic value of texture analysis from standard of care CT and FDG-PET images, which can predict treatment outcome in some ways.
Certain methodological aspects of radiomics have been studied, for example, in order to find a way of reducing redundancy and comparing the prognostic characteristics of radiomics features across cancer types. Parmar et al. (27), investigated cancer-specific radiomics feature clusters in his study published in 2015. This study concluded that consensus clustering could provide robust radiomics feature clusters and therefore could reduce feature redundancy. Another study, published by Leijenaar et al. (28) analysed the test-retest and inter-observer variability of radiomics features in FDG-PET images. The study concluded that the majority of assessed features had both a high test-retest (71%) and inter-observer (91%) stability, and that overall, features that were more stable in repeated PET imaging were also found to be more robust against inter-observer variability.
One of the first studies showing the potential of CT texture analysis as independent marker of survival for patients with non-small cell lung cancer (NSCLC) is the study of Ganeshan et al. from 2012 (24). They showed that patients with heterogeneous tumours with low uniformity values demonstrated poorer survival and that CT texture and PET stage were significant independent predictors of survival. Another study of Balagurunathan et al. (8) showed that a large value of run length calculated on CT images (one of the reproducible features) indicated a more homogeneous tumour, and that this was related to a longer survival. The largest, the most comprehensive and rigorous study is from Aerts et al., (15) who worked on radiomics to decode the tumour phenotype, found that a large number of radiomics features have prognostic power in independent datasets of lung and head and neck cancer patients, many of which were previously not identified as significant. After a very strict features reduction process, using test-retest datasets and multiple delineations datasets, they created a signature consisting of “only” four features quantifying tumour heterogeneity, consistently with their initial hypothesis. Combining this radiomics signature with TNM staging showed a significant improvement in all datasets, compared with TNM staging alone, considered as gold standard. Remarkably, the same signature was working on lung and head and neck cancer suggesting that radiomics identifies a general prognostic phenotype existing in both lung and head and neck cancer. They also showed in a series of operated patients, having had standardized CT imaging and gene expression array, the so-called “radiogenomic approach”, that the four feature prognostic radiomics signature, capturing intratumour heterogeneity, is associated with underlying gene-expression patterns linked to tumour proliferation (32). Overall, this study convincingly validate the signature on three external datasets and recently in a fourth one (33).
Next to the studies describing radiomics alone to stratify patients, there are also some studies describing the value of adding radiomics features to conventional prognostic factors alone. A study of Desseroit et al. (18) described the development of a nomogram combining clinical staging with PET/CT image features stage I-III NSCLC patients. The nomogram had a higher stratification power than the clinical staging alone. Patients with stage III disease, with a large tumour volume, low CT heterogeneity although a high PET heterogeneity had the poorest prognosis. A study of Fried et al. (4) also showed that models using textural features and conventional prognostic factors improved stratification power compared to models using conventional prognostic factors alone.
In addition, it was found that radiomics features can reflect different biologic mechanisms, such as gene-expression patterns or cell cycling pathways. A study of Yoon et al. (31) also made the link with gene-expression and showed that quantitative imaging using radiomics can capture distinct phenotypic differences between tumours. They showed that ALK/ROS1/RET fusion positive lung adenocarcinomas possesses certain imaging features next to clinical features that enable good discrimination between fusion positive and fusion negative tumours.
Three articles studied the imaging texture analysis of lung cancer in patients treated by stereotactic radiotherapy (20-22). Two studies by Mattonen et al. (21,22) investigated the use of imaging texture analysis in a decision support system in order to support earlier salvage for patients with recurrence and fewer investigations of benign radiation-induced lung injury. They found that radiomic features, extracted from CT images using an automated method, are able to predict recurrence with better performance than physicians. The study of Lovinfosse et al. (20) used radiomics along with clinical features, using univariate and multivariate analysis for OS, disease-specific survival (DSS) and disease-free survival (DFS) in order to assess the predictive value of the radiomics features. They concluded that the textural feature ‘dissimilarity’ measured on the baseline 18F-FDG PET/CT appears to be a strong independent predictor of the outcome in patients with NSCLC treated by stereotactic body radiation therapy.
Prognostic value of metabolic metrics extracted from baseline PET images in NSCLC is studied in three separate articles using OS to assess the prognostic value (5,7,17). The study from 2013 by Carvalho et al. (5) examined the prognostic value of metabolic PET descriptors on OS for NSCLC patients and they showed that only one metric, relative volume above 80% SUV, was significantly related to OS (P=0.05). The study from 2013 Cook et al. (7) investigated the relation of radiomics features to response and survival after chemoradiotherapy for 53 NSCLC patients. This study concluded that three textural features were able to differentiate between responders and non-responders to chemoradiotherapy (determined using RECIST) and were independent predictors of OS.
A study by Cook et al. (6) showed that the response to erlotinib is associated with reduced heterogeneity at 18F-FDG PET and that changes in first-order entropy are independently associated with OS and treatment response. And the 2016 study by Fried et al. (17) showed that pretreatment PET features were associated with OS in 195 patients with stage III NSCLC. In this study, predictors of OS generated with both quantitative imaging features and conventional prognostic factors demonstrated improved risk stratification compared with those generated with conventional prognostic factors.
Another study investigating the prognostic value of PET features by van Gómez López et al. (34) showed that textural analyses of 18F-FDG PET images to assess tumour heterogeneity were related to global metabolic parameters (e.g., SUVmax, SUVmean, MTV and TLG) and pathologic staging.
In addition, the study by Coroller et al. (16) identified predictive radiomic features for pathological response in 127 patients with locally advanced NSCLC after neoadjuvant chemoradiation. This study demonstrated that radiomics can provide valuable clinical information, and perform better than conventional imaging.
Furthermore, one study published in 2015 used radiomics to predict tumour distant metastasis, and concluded that radiomics features capture detailed information of the tumour phenotype and therefore can be used as a prognostic biomarker for clinically-relevant factors such as distant metastasis (3). However, distant metastasis is not the only clinically-relevant factor with which radiomics features can be correlated. A different study assessed the relationship between radiation dose and change of texture-based features of lung tissue in order to determine the ability of texture analysis to identify patients who develop radiation pneumonitis (RP) (23). A relationship between dose and change in a set of image-based features was observed. For 12 features, feature values were significantly related to RP development. This study demonstrated the ability of radiomics to provide a quantitative, individualized measurement of patient lung tissue reaction to radiation therapy and assess RP development.
In the field of diagnostics, radiomics can be used for the classification of nodules. A study by Dhara et al. (25) showed that using support vector machine nodules can be classified as benign or malignant. In addition the study by Dilger et al. (26) showed that this classification can be improved by including features quantified from the surrounding lung tissue.
Radiomics is usually performed using textural information derived from the primary tumor. However, textural information derived from the lymph nodes may contain complementary information. An ongoing study on 18F-FDG-PET images of 262 NSCLC patients is performing radiomics on both primary tumour and lymph nodes and is investigating the predictive performance to OS (35).
The above-mentioned studies performed radiomics on pre-treatment scans to advance the stratification of patients for therapy leading to improvements in health outcomes. Some other studies focused more on radiomics for response assessment by comparing radiomics features of a baseline scan withradiomics features of a second scan performed during treatment. Information about early changes in radiomics features can potentially increase the prognostic value of a model. Currently, percentage variations of radiomics features derived from PET images at baseline and the second week of treatment are calculated and related to OS for NSCLC patients (19). The next challenge will be to use radiomics approaches for PET biomarkers different then fluorodeoxyglucose in an attempt to extract more information (36-39).
Challenges and future prospects
Tumour motion
Standardization of image acquisition and reconstruction is one of the major challenges of radiomics (40-42). Reproducibility and stability of radiomics features is important for potential clinical utility (12,28,43). In lung cancer, breathing-induced tumour motion is one of the factors causing variability in image features, and different methods exist to reduce the influence of breathing motion on image characteristics (44). Due to the scan duration of a 3D (free-breathing) PET scan, images are averaged over multiple breathing cycles and introduce some noise. During a respiratory correlated 4D PET acquisition, counts are binned into typically 5 to 10 phases. This gating based on respiratory motion reduces blurring, but due to the reduced acquisition time per phase an increased noise is observed. However, there are also motion correction techniques available (45-47). For CT images of lung cancer patients, the mid-ventilation phase of a respiratory-gated (4D) acquisition is commonly used, in the motion management option, for target delineation. This phase is typically used for treatment planning purposes and the GTV or MTV can also be used as the ROI for tumour quantification with radiomics. Variations in radiomics feature values may occur when a different phase or a static (3D) CT is used instead. In Figure 2, variations in SUVmax are shown using different acquisition methods.
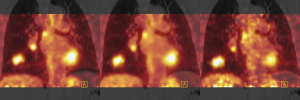
For generalizability and robust extraction of radiomics for lung cancer, it is important that the impact of tumour motion on the radiomics feature values is minimized, using gated acquisitions or breath-hold methods.
Two recent studies investigated the influence of motion on radiomics feature values derived from PET and CT. Relative differences in features values were calculated between 3D and 4D acquisitions. A study by Yip et al. (30) investigated five texture features derived from 3D PET and 4D PET for 26 lung cancer patients. In this study, 4D PET counts were binned into five phases and the differences in features values between all five phase bins were assessed. They reported significant differences between 3D and all bins of 4D PET for three features and significant differences between 3D and 4D for four out of five bins for one feature. The textural feature ‘Contrast’ was not significantly different between 3D and 4D PET. Between different breathing phases, none of the five features was significantly different. A second study evaluated the influence of 56 radiomics features between 3D PET/CT and 4D PET/CT acquisitions for lung cancer patients (n=23) (29). The acquired data from the PET scan were binned into ten phases. Features were extracted from the first phase (inhale) and the fifth phase (exhale) of the respiratory-gated scan. They found that the differences in features between a 3D and 4D acquisitions varied between 0% and 193% for PET and 0% and 176% for CT, with the feature kurtosis being an outlier for both modalities. Substantial differences between radiomics features derived from 3D and 4D acquisitions were observed for both CT and PET. Respiratory-gated imaging reduces effects of motion, including blurring, rotation and deformation at the expense of a somewhat higher noise level. It is important to choose the respiratory phase-bin with robust features for quantitative tumour characterization using 4D imaging. To improve standardization in radiomics, acquisition and reconstruction protocols used to acquire quantitative image features should always be described in detail for both PET and CT.
Methodological issues
There is one major risk in radiomics studies: the selection of significant features by chance and overfitting. This issue is very similar to that in the field of genomics. Authors should clearly state in their publication, in what way the study has advanced the field of radiomics and how it has specifically identified and met an unmet need. It is also important that authors avoid making overly optimistic claims concerning robustness and generalizability as they diminish scientific and clinical impact. Furthermore, only 5 of the 22 articles were externally validated, this highlights another methodological issue. Study design, protocols, detailed quality assurance processes and standard operating procedures should be exhaustively reported in the publications. Rigorous reporting guidelines and requirements are necessary for the maturation of radiomics. While the minute technical details of radiomics and finding external validation datasets can be tedious, they potentially have a great influence on the robustness, generalizability and confound meta-analyses.
The following points are crucial in radiomics studies:
- Standardized imaging protocols allow for appropriate meta-analysis;
- The effects of inter-scanner differences should considered and minimized;
- Robust segmentation, preferably automated, is advantageous for ranking features on the basis of their spatial reproducibility/stability;
- Test-retest is useful for ranking features on the basis of their temporal reproducibility/stability (28);
- Independent validation datasets, preferably from another centre and multiple datasets can provide crucial information with regard to overfitting, clinical performance and generalizability.
Conclusions
There are two main applications of radiomics in lung cancer, classification of lung nodules (diagnostic) or prognostication of established lung cancer (theragnostic). Overall, it is clear that radiomics has great potential to improve diagnosis and patients stratification in lung cancer. It may also have a clinical impact as imaging is routinely used in clinical practice, providing an unprecedented opportunity to improve decision support in lung cancer for diagnosis or treatment at low cost (48-51).
Acknowledgments
Funding: Authors acknowledge financial support from the QuIC-ConCePT project, which is partly funded by EFPI Acompanies and the Innovative Medicine Initiative Joint Undertaking (IMIJU) under Grant Agreement No. 115151. This research is also supported by the Dutch technology Foundation STW (grant n 10696 DuCAT; n P14-19 radiomics STRaTegy), which is the applied science division of NWO. Authors also acknowledge financial support from EU 7th framework program (ARTFORCE – n 257144, REQUITE - n 601826), SME Phase 2 (EU proposal 673780 - RAIL). Authors thank the contribution of Simone Moorman for editing.
Footnote
Provenance and Peer Review: This article was commissioned by the Guest Editors (Laurence E. Court, Arvind Rao and Sunil Krishnan) for the series “Radiomics in Radiation Oncology” published in Translational Cancer Research. The article has undergone external peer review.
Conflicts of Interest: All authors have completed the ICMJE uniform disclosure form (available at http://dx.doi.org/10.21037/tcr.2016.06.18). The series “Radiomics in Radiation Oncology” was commissioned by the editorial office without any funding or sponsorship. The authors have no other conflicts of interest to declare.
Ethical Statement: The authors are accountable for all aspects of the work in ensuring that questions related to the accuracy or integrity of any part of the work are appropriately investigated and resolved.
Open Access Statement: This is an Open Access article distributed in accordance with the Creative Commons Attribution-NonCommercial-NoDerivs 4.0 International License (CC BY-NC-ND 4.0), which permits the non-commercial replication and distribution of the article with the strict proviso that no changes or edits are made and the original work is properly cited (including links to both the formal publication through the relevant DOI and the license). See: https://creativecommons.org/licenses/by-nc-nd/4.0/.
References
- Available online: https://cancerstatisticscenter.cancer.org
- Lambin P, Rios-Velazquez E, Leijenaar R, et al. Radiomics: extracting more information from medical images using advanced feature analysis. Eur J Cancer 2012;48:441-6. [Crossref] [PubMed]
- Coroller TP, Grossmann P, Hou Y, et al. CT-based radiomic signature predicts distant metastasis in lung adenocarcinoma. Radiother Oncol 2015;114:345-50. [Crossref] [PubMed]
- Fried DV, Tucker SL, Zhou S, et al. Prognostic value and reproducibility of pretreatment CT texture features in stage III non-small cell lung cancer. Int J Radiat Oncol Biol Phys 2014;90:834-42. [Crossref] [PubMed]
- Carvalho S, Leijenaar RT, Velazquez ER, et al. Prognostic value of metabolic metrics extracted from baseline positron emission tomography images in non-small cell lung cancer. Acta Oncol 2013;52:1398-404. [Crossref] [PubMed]
- Cook GJ, O'Brien ME, Siddique M, et al. Non-Small Cell Lung Cancer Treated with Erlotinib: Heterogeneity of (18)F-FDG Uptake at PET-Association with Treatment Response and Prognosis. Radiology 2015;276:883-93. [Crossref] [PubMed]
- Cook GJ, Yip C, Siddique M, et al. Are pretreatment 18F-FDG PET tumor textural features in non-small cell lung cancer associated with response and survival after chemoradiotherapy? J Nucl Med 2013;54:19-26. [Crossref] [PubMed]
- Balagurunathan Y, Gu Y, Wang H, et al. Reproducibility and Prognosis of Quantitative Features Extracted from CT Images. Transl Oncol 2014;7:72-87. [Crossref] [PubMed]
- Kumar V, Gu Y, Basu S, et al. Radiomics: the process and the challenges. Magn Reson Imaging 2012;30:1234-48. [Crossref] [PubMed]
- Velazquez ER, Parmar C, Jermoumi M, et al. Volumetric CT-based segmentation of NSCLC using 3D-Slicer. Sci Rep 2013;3:3529. [Crossref] [PubMed]
- Hatt M, Cheze-le Rest C, van Baardwijk A, et al. Impact of tumor size and tracer uptake heterogeneity in (18)F-FDG PET and CT non-small cell lung cancer tumor delineation. J Nucl Med 2011;52:1690-7. [Crossref] [PubMed]
- Parmar C, Rios Velazquez E, Leijenaar R, et al. Robust Radiomics feature quantification using semiautomatic volumetric segmentation. PLoS One 2014;9:e102107 [Crossref] [PubMed]
- Cheebsumon P, Boellaard R, deRuysscher D, et al. Assessment of tumour size in PET/CT lung cancer studies: PET- and CT-based methods compared to pathology. EJNMMI Res 2012;2:56. [Crossref] [PubMed]
- Steyerberg EW, Vickers AJ, Cook NR, et al. Assessing the Performance of Prediction Models. Epidemiology 2010;21:128-38. [Crossref] [PubMed]
- Aerts HJ, Velazquez ER, Leijenaar RT, et al. Decoding tumour phenotype by noninvasive imaging using a quantitative radiomics approach. Nat Commun 2014;5:4006. [PubMed]
- Coroller TP, Agrawal V, Narayan V, et al. Radiomic phenotype features predict pathological response in non-small cell lung cancer. Radiother Oncol 2016;119:480-6. [Crossref] [PubMed]
- Fried DV, Mawlawi O, Zhang L, et al. Stage III Non–Small Cell Lung Cancer: Prognostic Value of FDG PET Quantitative Imaging Features Combined with Clinical Prognostic Factors. Radiology 2016;278:214-22. [Crossref] [PubMed]
- Desseroit MC, Visvikis D, Tixier F, et al. Development of a nomogram combining clinical staging with F-FDG PET/CT image features in non-small-cell lung cancer stage I-III. Eur J Nucl Med Mol Imaging 2016;43:1477-85. [Crossref] [PubMed]
- Carvalho S, Leijenaar RT, Troost EG, et al. Early variation of FDG-PET radiomics features in NSCLC is related to overall survival - the “delta radiomics” concept. Radiother Oncol 2016;118:S20-S21. [Crossref]
- Lovinfosse P, Janvary ZL, Coucke P, et al. FDG PET/CT texture analysis for predicting the outcome of lung cancer treated by stereotactic body radiation therapy. Eur J Nucl Med Mol Imaging 2016;43:1453-60. [Crossref] [PubMed]
- Mattonen SA, Palma DA, Johnson C, et al. Detection of Local Cancer Recurrence After Stereotactic Ablative Radiation Therapy for Lung Cancer: Physician Performance Versus Radiomic Assessment. Int J Radiat Oncol Biol Phys 2016;94:1121-8. [Crossref] [PubMed]
- Mattonen SA, Tetar S, Palma DA, et al. Imaging texture analysis for automated prediction of lung cancer recurrence after stereotactic radiotherapy. J Med Imaging (Bellingham) 2015;2:041010 [Crossref] [PubMed]
- Cunliffe A, Armato SG 3rd, Castillo R, et al. Lung texture in serial thoracic computed tomography scans: correlation of radiomics-based features with radiation therapy dose and radiation pneumonitis development. Int J Radiat Oncol Biol Phys 2015;91:1048-56. [Crossref] [PubMed]
- Ganeshan B, Panayiotou E, Burnand K, et al. Tumour heterogeneity in non-small cell lung carcinoma assessed by CT texture analysis: a potential marker of survival. Eur Radiol 2012;22:796-802. [Crossref] [PubMed]
- Dhara AK, Mukhopadhyay S, Dutta A, et al. A Combination of Shape and Texture Features for Classification of Pulmonary Nodules in Lung CT Images. J Digit Imaging 2016;29:466-75. [Crossref] [PubMed]
- Dilger SK, Uthoff J, Judisch A, et al. Improved pulmonary nodule classification utilizing quantitative lung parenchyma features. J Med Imaging (Bellingham) 2015;2:041004 [Crossref] [PubMed]
- Parmar C, Leijenaar RT, Grossmann P, et al. Radiomic feature clusters and prognostic signatures specific for Lung and Head & Neck cancer. Sci Rep 2015;5:11044. [Crossref] [PubMed]
- Leijenaar RT, Carvalho S, Velazquez ER, et al. Stability of FDG-PET Radiomics features: an integrated analysis of test-retest and inter-observer variability. Acta Oncol 2013;52:1391-7. [Crossref] [PubMed]
- Oliver JA, Budzevich M, Zhang GG, et al. Variability of Image Features Computed from Conventional and Respiratory-Gated PET/CT Images of Lung Cancer. Transl Oncol 2015;8:524-34. [Crossref] [PubMed]
- Yip S, McCall K, Aristophanous M, et al. Comparison of Texture Features Derived from Static and Respiratory-Gated PET Images in Non-Small Cell Lung Cancer. PloS One 2014;9:e115510 [Crossref] [PubMed]
- Yoon HJ, Sohn I, Cho JH, et al. Decoding Tumor Phenotypes for ALK, ROS1, and RET Fusions in Lung Adenocarcinoma Using a Radiomics Approach. Medicine (Baltimore) 2015;94:e1753 [Crossref] [PubMed]
- Panth KM, Leijenaar RT, Carvalho S, et al. Is there a causal relationship between genetic changes and radiomics-based image features? An in vivo preclinical experiment with doxycycline inducible GADD34 tumor cells. Radiother Oncol 2015;116:462-6. [Crossref] [PubMed]
- Leijenaar RT, Carvalho S, Hoebers FJ, et al. External validation of a prognostic CT-based radiomic signature in oropharyngeal squamous cell carcinoma. Acta Oncol 2015;54:1423-9. [Crossref] [PubMed]
- van Gómez López O, García Vicente AM. Heterogeneity in [18F]fluorodeoxyglucose positron emission tomography/computed tomography of non-small cell lung carcinoma and its relationship to metabolic parameters and pathologic staging. Mol Imaging 2014;13. [PubMed]
- Carvalho S, Leijenaar RT, Troost EG, et al. OC-0205: Prognostic value of pre-RT PET metrics of lymph nodes vs. primary tumor in NSCLC: which holds more information? Radiother Oncol 2015;115:S103-S104. [Crossref]
- Zegers CM, van Elmpt W, Reymen B, et al. In Vivo Quantification of Hypoxic and Metabolic Status of NSCLC Tumors Using [18F]HX4 and [18F]FDG-PET/CT Imaging. Clin Cancer Res 2014;20:6389-97. [Crossref] [PubMed]
- Zegers CM, van Elmpt W, Wierts R, et al. Hypoxia imaging with [18F]HX4 PET in NSCLC patients: defining optimal imaging parameters. Radiother Oncol 2013;109:58-64. [Crossref] [PubMed]
- Dubois LJ, Lieuwes NG, Janssen MH, et al. Preclinical evaluation and validation of [18F]HX4, a promising hypoxia marker for PET imaging. Proc Natl Acad Sci U S A 2011;108:14620-5. [Crossref] [PubMed]
- van Loon J, Janssen MH, Ollers M, et al. PET imaging of hypoxia using [18F]HX4: a phase I trial. Eur J Nucl Med Mol Imaging 2010;37:1663-8. [Crossref] [PubMed]
- Galavis PE, Hollensen C, Jallow N, et al. Variability of textural features in FDG PET images due to different acquisition modes and reconstruction parameters. Acta Oncol 2010;49:1012-6. [Crossref] [PubMed]
- Fave X, Mackin D, Yang J, et al. Can radiomics features be reproducibly measured from CBCT images for patients with non-small cell lung cancer? Med Phys 2015;42:6784-97. [Crossref] [PubMed]
- Leijenaar RT, Nalbantov G, Carvalho S, et al. The effect of SUV discretization in quantitative FDG-PET Radiomics: the need for standardized methodology in tumor texture analysis. Sci Rep 2015;5:11075. [Crossref] [PubMed]
- Balagurunathan Y, Kumar V, Gu Y, et al. Test-retest reproducibility analysis of lung CT image features. J Digit Imaging 2014;27:805-23. [Crossref] [PubMed]
- Nagel CC, Bosmans G, Dekker AL, et al. Phased attenuation correction in respiration correlated computed tomography/positron emitted tomography. Med Phys 2006;33:1840-7. [Crossref] [PubMed]
- van Elmpt W, Hamill J, Jones J, et al. Optimal gating compared to 3D and 4D PET reconstruction for characterization of lung tumours. Eur J Nucl Med Mol Imaging 2011;38:843-55. [Crossref] [PubMed]
- Liu C, Alessio A, Pierce L, et al. Quiescent period respiratory gating for PET/CT. Med Phys 2010;37:5037-43. [Crossref] [PubMed]
- Dawood M, Buther F, Jiang X, et al. Respiratory motion correction in 3-D PET data with advanced optical flow algorithms. IEEE Trans Med Imaging 2008;27:1164-75. [Crossref] [PubMed]
- Lambin P, van Stiphout RG, Starmans MH, et al. Predicting outcomes in radiation oncology--multifactorial decision support systems. Nat Rev Clin Oncol 2013;10:27-40. [Crossref] [PubMed]
- Lambin P, Roelofs E, Reymen B, et al. 'Rapid Learning health care in oncology' - an approach towards decision support systems enabling customised radiotherapy'. Radiother Oncol 2013;109:159-64. [Crossref] [PubMed]
- Lambin P, Petit SF, Aerts HJ, et al. The ESTRO Breur Lecture 2009. From population to voxel-based radiotherapy: exploiting intra-tumour and intra-organ heterogeneity for advanced treatment of non-small cell lung cancer. Radiother Oncol 2010;96:145-52. [Crossref] [PubMed]
- Lambin P, Zindler J, Vanneste B, et al. Modern clinical research: How rapid learning health care and cohort multiple randomised clinical trials complement traditional evidence based medicine. Acta Oncol 2015;54:1289-300. [Crossref] [PubMed]