Construction of a gene signature associated with anoikis to evaluate the prognosis and immune infiltration in patients with colorectal cancer
Highlight box
Key findings
• This study evaluated the prognostic significance of anoikis-related genes (ARGs) in colorectal cancer, then identified two different anoikis-related clusters and assessed their respective immune microenvironments. In addition, the establishment of ARGs markers can effectively predict the prognosis of colorectal cancer, which lays a foundation for the study of ignorance in the clinical prognosis of colorectal cancer.
What is known and what is new?
• The expression level of ARGs is closely related to the prognosis of colorectal cancer patients, which is supported by a large amount of literature.
• We constructed a prognostic prediction model for colorectal cancer patients using genes associated with anoikis, which may provide a basis for post-treatment surveillance of colorectal cancer patients.
What is the implication, and what should change now?
• The analysis of our data indicates that ARGs are crucial in predicting the prognosis of colorectal cancer. It lays the foundation for further research on ARGs. In the future, researchers should focus on mining information related to these genes.
Introduction
Colorectal cancer (CRC) is the second leading cause of cancer-related death worldwide, contributing to approximately one million deaths each year (1). The current clinical approach to the treatment of CRC involves a combination of surgery, chemotherapy, radiotherapy, and the integration of molecular targeted therapies. However, the effectiveness of these treatment modalities remains suboptimal, primarily due to the challenges presented by tumor metastasis (2). The metastatic spread of CRC accounts for nearly half of all patient deaths, highlighting the critical need to identify reliable prognostic signatures associated with metastasis (3). These signatures are essential for post-intervention monitoring and prognostic prediction in CRC.
Anoikis resistance, a phenomenon characterized by the ability of cancer cells to survive detachment from the extracellular matrix (ECM), is considered a precursor to tumor metastasis (4). In normal physiological conditions, cells undergo apoptosis upon detachment, which is termed “Anoikis” (5). However, cancer cells can evade this detachment-induced apoptosis and acquire the capacity to metastasize (6). Extensive research has investigated the involvement of genes associated with anoikis in CRC. This research has demonstrated a close association between these genes and disease progression as well as patient survival (7,8). However, there has been limited exploration of prognostic models based on anoikis-related genes (ARGs) for predicting the prognosis of CRC.
The current study aimed to evaluate the prognostic significance of ARGs in CRC. Two distinct clusters associated with anoikis were identified through comprehensive analysis and examined their respective immune microenvironments. Additionally, an ARGs signature was developed that demonstrated robust predictive capabilities for assessing the prognosis of CRC patients. We present this article in accordance with the TRIPOD reporting checklist (available at https://tcr.amegroups.com/article/view/10.21037/tcr-23-1221/rc).
Methods
Data collection
The present study utilized data retrieved from The Cancer Genome Atlas (TCGA) database (https://portal.gdc.cancer.gov/) to acquire clinicopathological information including tumor-node-metastasis (TNM) stage characteristics, patient age, patient survival status, and RNA-sequencing (RNA-seq) data of patients diagnosed with colorectal adenocarcinoma (COAD). The data download was conducted on March 26, 2023. Expression profiles of 41 normal tissues and 476 COAD tissues were obtained through data collation. Furthermore, the Gene Expression Omnibus (GEO) database (https://www.ncbi.nlm.nih.gov/geo/) was utilized to obtain the GSE40967 dataset along with the associated clinical data. Somatic mutation counts and copy number variation (CNV) data were obtained from the TCGA database.
Additionally, a screening standard of relevance ≥0.4 was employed to retrieve 513 ARGs from the Genecard website (https://www.genecards.org/). The search for these genes was performed on March 26, 2023.
Analysis of differentially-expressed ARGs and CNV mutation analysis
We identified the differentially expressed genes (DEGs) in the TCGA-COAD dataset based on the 513 ARGs. To enhance the statistical power and reduce dataset heterogeneity, we integrated data from 566 tumor samples from GSE40967 and 476 tumor samples from TCGA-COAD. To identify prognostic-related genes associated with CRC patients, we conducted a univariate Cox analysis. The results of the univariate analysis were visualized using a forest plot, providing a graphical representation of the gene-gene relationships and their respective hazard ratios (HRs).
Cluster analysis
Cluster analysis was employed to ascertain the potential association between the expression of genes associated with anoikis and CRC. The findings revealed a robust intragroup correlation, while the intergroup correlation was found to be moderate when considering a value of k=2. Using the “ConsensusClusterPlus” R package (9), patients were categorized into cluster A and cluster B based on the expression of 47 ARGs. The correlation between molecular subtypes and the overall survival (OS) of CRC patients was examined using Kaplan-Meier plots. Principal component analysis (PCA) and t-distributed stochastic neighbor embedding (tSNE) are frequently employed methods for reducing the dimensionality of a dataset (10-12). This is achieved by converting a multitude of variables into a smaller set of variables that retain the majority of the information present in the original dataset (13). The differences in the expression of 47 genes related to anoikis between the two subtypes were visually represented through box plots and heatmaps. To investigate the potential mechanisms, we performed gene set enrichment analysis (GSEA) to analyze the pathways enriched in cluster B.
Construction of the prognostic signature
Integrating the information of 451 patients from TCGA database and 561 patients from GSE40967, we randomly grouped these 1,012 patients into training and validation sets (Table 1). The implementation of the least absolute shrinkage and selection operator (LASSO) regression methodology enabled the discovery of genes associated with survival (14). Subsequently, these genes mRNA expression were utilized to forecast the survival and prognosis of CRC patients within the training set. The most favorable model was subsequently chosen through the application of multivariate Cox regression analysis. A total of ten genes expression levels were used to construct a risk model. The formula for calculating individualized risk scores is: risk score = ∑(Exp(mRNA) × coef(mRNA)).
Table 1
Clinical features | Total patients (n=1,012), n (%) | TCGA (n=451), n (%) | GSE40967 (n=561), n (%) |
---|---|---|---|
Age (years) | |||
≤65 | 407 (40.22) | 185 (41.02) | 222 (39.57) |
>65 | 605 (59.78) | 266 (58.98) | 339 (60.43) |
Gender | |||
Male | 545 (53.85) | 237 (52.55) | 308 (54.90) |
Female | 467 (46.15) | 214 (47.45) | 253 (45.10) |
Fustat | |||
Alive | 725 | 355 (78.71) | 370 (65.95) |
Dead | 287 | 96 (21.29) | 191 (34.05) |
Stage T | |||
1–2 | 142 (14.37) | 80 (22.60) | 55 (10.22) |
3–4 | 846 (85.63) | 274 (77.40) | 483 (89.78) |
NA | 23 (2.27) | 0 (0.00) | 23 (4.10) |
Stage N | |||
N0 | 566 (57.40) | 230 (64.79) | 298 (55.70) |
N1–3 | 420 (42.60) | 125 (35.21) | 237 (44.30) |
NA | 26 (2.57) | 0 (0.00) | 26 (4.63) |
TCGA, The Cancer Genome Atlas; GEO, Gene Expression Omnibus; NA, not applicable.
Validation of the risk assessment model for prognosis and establishment and verification of nomograms
To gain further insights into the precision and prognostic significance of this risk score, the patients within each cluster were categorized as high-risk or low-risk based on the calculated risk score. Receiver operating characteristic (ROC) curves, area under the curve (AUC) curves, and survival curves were used to validate the accuracy and prognostic value of the model. Independent predictors identified through multivariate Cox regression analysis were incorporated to construct nomograms using the “rms” package. The consistency index was then utilized to measure the discriminatory power of the nomogram, and the observed probability was graphed in comparison to the probability predicted by the nomogram to assess the calibration curve. Decision curve analysis (DCA) was employed as a tool to evaluate the effectiveness and reliability of the nomograms.
Immune cell infiltration analysis
The ESTIMATE algorithm was utilized to analyze the immune score and tumor purity, providing insights into the extent of immune cell infiltration within the tumor tissue. The “CIBERSORT” R package was used to compare the immune microenvironment across the different clusters.
Patients and samples
To validate the reliability of the above bioinformatics analysis, we obtained clinical tissue samples from ten pairs of CRC patients from The First Affiliated Hospital of Zhejiang Chinese Medical University. After surgical resection, the tissue samples were stored in a cryogenic refrigerator at −80 ℃ and kept at low temperature during sample transfer and non-experimental processing. Biometric testing of all tissue samples was performed with the informed consent of the patients. This study was conducted in accordance with the Declaration of Helsinki (as revised in 2013). The study was approved by the Ethics Committee of The First Affiliated Hospital of Zhejiang Chinese Medical University (No. 2017-K-002-01).
Quantitative real-time polymerase chain reaction (qRT-PCR) validation
PCR primers are available in Table S1. The manufacturer’s protocol, as provided by Invitrogen (Carlsbad, USA), was followed to extract total RNA from tissue using Trizol reagent (Invitrogen, Carlsbad, USA). Subsequently, cDNA reverse transcription was performed using a reverse transcription RNA kit from Thermo Scientific (Vilnius, Lithuania). The gene of interest was then amplified using a SYBR Green PCR kit from Applied Biosystems (Invitrogen, Vilnius, Lithuania). Samples were analyzed using an ABI PCR system, and each experiment was repeated at least three times. The 2−ΔΔCt method was used to determine gene expression relative to the reference gene GAPDH.
Statistical analysis
Statistical analysis and graph plotting were performed using R (version 4.2.1) and GraphPad Prism (version 9), respectively. The t-test was used to compare the data between the two groups. Statistical significance was determined at P values of <0.05 (*), <0.01 (**), and <0.001 (***) for differences observed within and between the groups.
Results
Identification of prognostic-related differential ARGs in CRC and normal tissues
The study’s workflow is depicted in Figure 1. The TCGA dataset was utilized to investigate the expression levels of 513 ARGs in both normal and COAD specimens. Through differential analysis, a total of 160 DEGs associated with anoikis were identified, with 113 genes upregulated and 47 genes downregulated in COAD tissues compared to normal tissues (Figure 2A, Figure S1). Among these DEGs, 47 ARGs exhibited a strong correlation with the prognosis of CRC patients through univariate regression analysis. A forest plot, generated from the results of the univariate regression analysis, demonstrated that approximately 30 gene expression levels showed a significant association with the OS of CRC patients (Figure 2B).
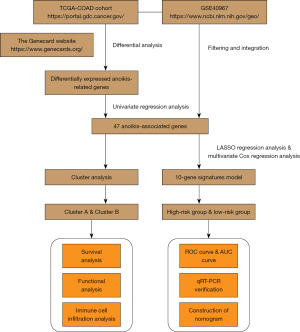
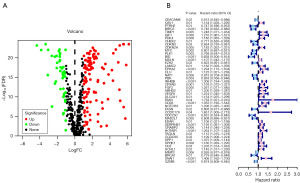
Genetic variations of ARGs in COAD
A network diagram was employed to depict the intricate interactions between genes associated with anoikis and their prognostic significance in CRC (Figure 3A). Genetic mutations play a crucial role in tumor development and contribute to characteristics like tumor metastasis. The CNV mutation data for 47 genes located on various chromosomes was presented (Figure 3B). Analysis of these 47 DEGs associated with anoikis revealed a high prevalence of CNV-related mutations (Figure 3C). Notably, several genes, including MYC, SNAI1, FASN, BIRC5, BRCA1, and SLC2A1, exhibited widespread CNV amplification (Figure 3C).
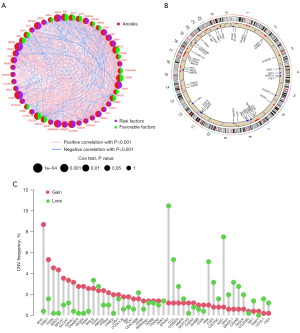
Cluster analysis with 47 ARGs
A consensus clustering approach was used to categorize patients with CRC into two distinct clusters based on the expression patterns of 47 ARGs. The data revealed that classifying patients into cluster A and cluster B proved to be a viable option (Figure 4A). Furthermore, the results from the survival analysis indicated that patients classified in cluster A had a more favorable prognosis compared to those in cluster B (Figure 4B). To validate the accuracy of the clustering, PCA and t-SNE analysis were conducted, confirming substantial differences in the transcriptional expression of ARGs-associated genes between cluster A and cluster B (Figure 4C,4D). Almost all of the 47 DEGs exhibited significant differences in expression between the two clusters (Figure 4E).
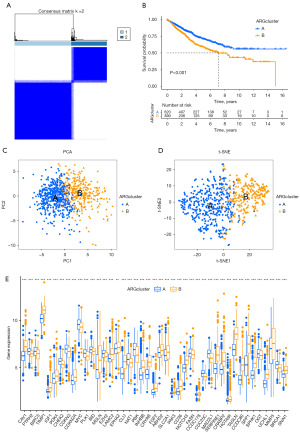
Immunoassay and functional analysis with two clusters
The heatmap visualization depicted the detailed expression patterns of the 47 ARGs in cluster A and cluster B (Figure 5A). When comparing the tumor microenvironments (TMEs) of the two clusters, it was observed that cluster B exhibited a significantly higher prevalence of immune cell infiltrates (Figure 5B). To further elucidate the potential mechanisms underlying the prognostic impact of ARGs, GSVA enrichment analysis was performed on the two clusters. The results revealed 20 Kyoto Encyclopedia of Genes and Genomes (KEGG) pathways that exhibited differential enrichment between the two clusters (Figure 6A). Cluster A showed significant enrichment in pathways such as peroxisome and aminoacyl tRNA biosynthesis, while cluster B exhibited enrichment in pathways such as glycosaminoglycan biosynthesis (chondroitin sulfate), complement and coagulation cascades, as well as other pathways. Notably, cluster B displayed higher activity in pathways such as ECM receptor interaction, hematopoietic cell lineage, and cell adhesion molecules (CAMs) (Figure 6A,6B).
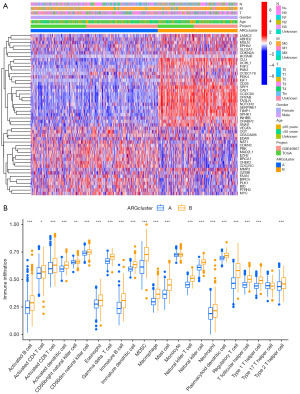
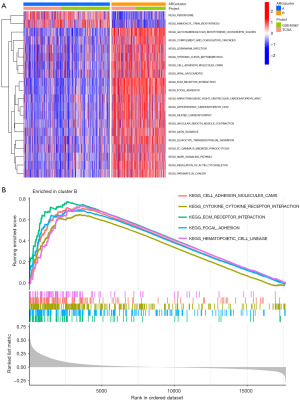
Construction of an anoikis-related signature and a nomogram based on the signature
In order to avoid overfitting, the data dimensionality was reduced using LASSO regression analysis and multivariate Cox regression analysis, resulting in the identification of ten prognostic ARGs for COAD patients (Figure 7A,7B). Among these ARGs, LAMC2, inhibin subunit beta B gene (INHBB), CD36, EDAR, HOX transcript antisense RNA (HOTAIR), and SNAI1 were identified as risk factors associated with a higher risk (HR >1) for COAD patients. On the other hand, NAT1, FASN, CCDC80, and GZMB were identified as protective factors associated with improved prognosis for COAD patients. A risk score calculation formula was established based on the expression levels of these ten ARGs. The model’s reliability was confirmed in both the training and test sets (Figures 7,8). The training and test sets were divided into high-risk and low-risk groups using the median value of the risk score. Kaplan-Meier analysis demonstrated that patients with high-risk scores had a significantly higher probability of mortality in the training set, test set, and the entire cohort (Figure 7C). ROC curve analysis showed that the risk score signature had AUC values of 0.666 (1 year), 0.710 (3 years), and 0.661 (5 years) for the entire cohort (Figure 7D). The model displayed good predictive ability and accuracy in identifying the prognosis of CRC patients. The relationship between the prognostic model of ARGs and clinicopathological characteristics was analyzed using a heatmap, which revealed the expression differences of ARGs between the high-risk and low-risk groups (Figure 8A,8B). A Sankey diagram demonstrated the relationship between the two clusters, risk scores, and the prognostic status of patients (Figure 8C). To enhance the predictive capacity of the model, a nomogram was developed by integrating clinical factors and risk scores, enabling the prediction of OS at 1, 3, and 5 years (Figure 9A). The calibration plot indicated a satisfactory level of concordance between the nomogram and the model (Figure 9B). This approach allowed us to conduct a more comprehensive analysis of the risk model’s predictive potential. The cumulative risk curve demonstrates that patients classified in the high-risk group exhibit a higher risk magnitude compared to those in the low-risk group as time progresses (Figure 9C). The nomogram exhibited advanced prognostic abilities compared to other clinical characteristics, as demonstrated by the DCA (Figure 9D-9F).
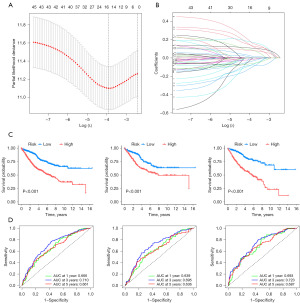
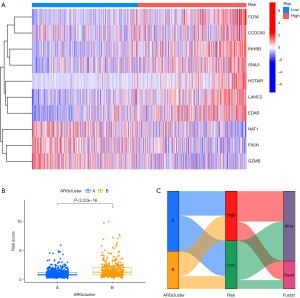
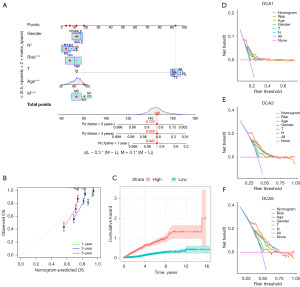
Overall, this comprehensive analysis of the risk model’s predictive potential provided valuable insights into the prognosis and survival prediction of COAD patients.
Estimation of tumor immune cell infiltration according to the signature
The CIBERSORT algorithm was used to analyze a total of 22 immune cell types, allowing for the investigation of the ability of ARGs models to accurately reflect the immune micro-environment in CRC (Figure 10A). Heatmaps were created to illustrate the close association between the ten signatures and immune cells (Figure 10B). Moreover, the high-risk group exhibited increased numbers of macrophages M0, macrophages M2, eosinophils, and neutrophils cells compared to the low-risk group (Figure 10C). Conversely, the high-risk group displayed significantly reduced numbers of T cell CD8 and T cell CD4 memory activated (Figure 10C). These findings suggest that the model is associated with immune cell infiltration in COAD specimens.
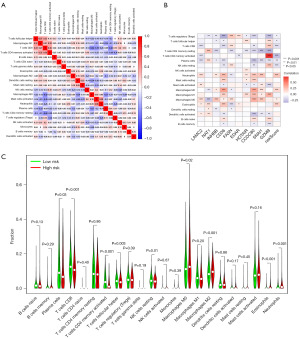
The expression of ARGs in CRC
To validate the results obtained from our data analysis, we collected total RNA from both tumor tissue and adjacent normal tissue. Subsequently, we performed qRT-PCR assays to assess the mRNA expression levels of CD36, CCDC80, INHBB, SNAI1, HOTAIR, LAMC2, EDAR, NAT1, FASN, and GZMB. The qRT-PCR results demonstrated that the mRNA expression levels of CD36, CCDC80, HOTAIR, EDAR, NAT1, INHBB, and GZMB were significantly lower in colon cancer tissue compared to adjacent normal tissue. Conversely, the mRNA levels of SNAI1, FASN, and LAMC2 were significantly elevated in colon cancer tissue (Figure 11). This validation experiment supports the findings of our data analysis and further confirms the differential expression of these ARGs in CRC.
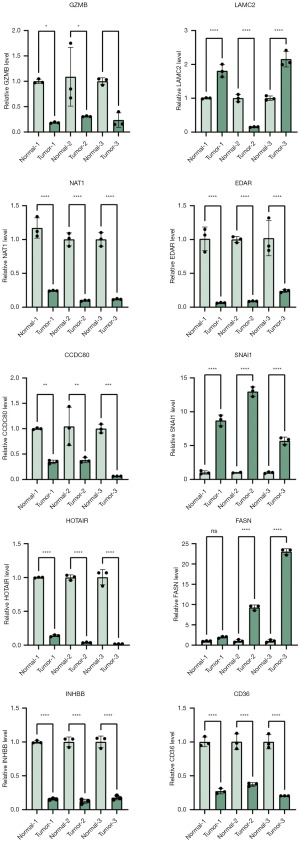
Discussion
Anoikis resistance is a crucial process that allows tumor cells to evade apoptosis and regain attachment capacity, facilitating dissemination, metastasis, and invasion (15). It plays a significant role in tumor metastasis, and various molecules, including SNORA42, have been implicated in the development of anoikis resistance in CRC (16). Additionally, certain drugs like Src kinase inhibitor AZD0530 have been found to reverse an anoikis-resistant and spheroidogenic intermediate mesenchymal state phenotype of SKOV3 ovarian cancer cells (17). Previous research has highlighted the potential of genes associated with anoikis as prognostic markers for CRC, but limited studies have explored their prognostic impact.
Our investigation aimed to examine the correlation between genes involved in anoikis and the prognosis of individuals with CRC. Our study revealed mutations in these genes at both the genetic and transcriptional levels. Consistent with previous studies, we found that genes with abnormal CNVs may serve as potential candidate markers for CRC (18). Understanding the differential expression of ARGs in CRC can contribute to the development of personalized and precise treatment strategies. Tumor molecular classification refers to the categorization of tumors using molecular analysis techniques, with varying types necessitating distinct treatment approaches, thereby facilitating tailored disease management (19). Therefore, an unsupervised consensus clustering algorithm was used to classify patients with CRC and divided all patients into two clusters. We further analyzed and evaluated these two subtypes, and the survival curve showed cluster B had a shortened survival time than cluster A. The role of the immune microenvironment in the development of CRC and evasion of primary corresponding treatment is very critical (20). In order to examine the potential correlation between the observed difference and the immune microenvironment, an investigation was conducted on the immune infiltration of patients belonging to two distinct clusters. A number of scholarly articles have previously reported that patients diagnosed with CRC and with a poor prognosis exhibit a greater degree of immune infiltration (21). The findings of our study indicate that cluster B patients’ tumor tissue exhibited a higher degree of immune cell infiltration. This suggests a robust association between the TME and the regulation of anoikis in CRC, a relationship that has been substantiated by earlier investigations (22).
To further predict the prognosis of CRC patients, we performed LASSO regression analysis and multi-univariate analysis. This allowed us to construct a risk model based on the expression of ten genes related to anoikis. The signature included CD36, CCDC80, INHBB, SNAI1, HOTAIR, LAMC2, EDAR, NAT1, FASN, and GZMB. These genes have been closely associated with tumor development and progression. For example, CD36, a membrane glycoprotein involved in various cellular functions including fatty acid metabolism and apoptotic cell clearance, has been shown to inhibit tumor cell proliferation and promote apoptosis in CRC cells when activated by the long non-coding RNA TINCR (23,24). Similarly, CCDC80 has been found to attenuate the proliferative impact of nonylphenol on CRC cells by inhibiting nonylphenol-mediated ERK1/2 activation (25). These findings suggest that CCDC80 may function as a tumor suppressor in CRC cells, exerting an anti-cancer effect. These studies are consistent with our results, suggesting that CD36 and CCDC80 are meaningful as prognostic monitoring factors. The model’s applicability was confirmed through the utilization of survival and ROC curves, which demonstrated its superior ability to predict patient prognostic risk. Notably, the risk score for the previous classification exhibited a significant divergence between the two clusters, indicating the meaningfulness of ARG expression detection in the prognosis prediction of CRC. Then, a nomogram was built to accurately quantify personalized predictive scores and survival probabilities. The calibration curve and DCA showed that the nomogram had a good prediction effect. Through the nomogram, an accurate digital survival or risk probability can be provided for each patient, which can assist clinicians in making decisions. An immune cell infiltration analysis was conducted on both risk groups, revealing notable differences in the majority of immune cells. This finding provides additional evidence of the strong correlation between ARGs and the TME in individuals with CRC. However, further investigation is required to establish the precise nature of this relationship.
To enhance the credibility of our findings, we conducted a measurement of the expression levels of ten genes in both tumor and adjacent tissues of tumor patients. While the majority of gene expression levels aligned with database projections, a few exhibited different trends. This discrepancy may be attributed to the limited sample size of three pairs. Consequently, we conducted a literature search to investigate the expression patterns of these four genes. INHBB encodes a pre-protein, which subsequently is processed to inhibin and activin. In many cancers, including CRC, INHBB shows a tendency to be overexpressed (26). In CRC, INHBB overexpression is often closely related to tumor metastasis and CRC staging (27). HOTAIR expression in cancerous tissues was significantly higher than in paracancerous tissues and high-expression HOTAIR was strongly associated with poor prognosis (28,29). This may be due to the induction of genome-wide retargeting of polycomb-repressive complex 2 (PRC2) in CRC by HOTAIR expression (29). HOTAIR trimethylates histone H3 lysine-27 (H3K27me3) of the HOXD locus with the PRC2 (30), which is composed of EZH2, SUZ12, and EED, and inhibits HOXD gene expression. The ectodysplasin a receptor (EDAR) may be a novel component of the Wnt/β-catenin signaling cascade, which plays a key role in regulating the proliferative ability of CRC. The elevated expression of EDAR was significantly related to the pathogenesis and progression of CRC (31) and is a potential indicator of CRC diagnosis and prognosis. Granzyme B (GZMB) is a member of the serine protease family and is a potent cytotoxic molecule. GZMB synthesized with toxic T lymphocytes and natural killer cells can enter tumor cells through the channel formed by perforin, targeting and destroying cancer cells (32,33).
Our study provides the differential expression of ARGs in CRC tumor tissue and normal groups and their effects on the immune microenvironment. Despite the robust prognostic capabilities exhibited by the ARGs signatures developed, this study is not without its constraints. A lack of clinical specimens can cause validation to be inaccurate. Additionally, additional experiments like immunohistochemistry (IHC) and immune blotting may be necessary for joint validation, and qRT-PCR alone may be insufficient to validate the predictions. It is also required to validate our prognostic model using the patient’s prognostic data. Drawing upon the amalgamation of findings from this investigation and antecedent research, it is our contention that the ARGs hold considerable importance in the monitoring of prognoses for CRC. Furthermore, we posit that the potential mechanism of ARGs and the immune microenvironment in CRC presents expansive opportunities for exploration and warrants further investigation.
Conclusions
This study evaluated the prognostic value of ARGs in CRC. Two distinct clusters were identified based on their association with anoikis, and the corresponding immune microenvironments were examined. An ARGs signature was developed, which demonstrated strong predictive capabilities for determining the prognosis of CRC. These findings provide a strong basis for further investigation into the role of anoikis in the clinical prognosis of CRC.
Acknowledgments
Funding: This study was supported by grants from
Footnote
Reporting Checklist: The authors have completed the TRIPOD reporting checklist. Available at https://tcr.amegroups.com/article/view/10.21037/tcr-23-1221/rc
Data Sharing Statement: Available at https://tcr.amegroups.com/article/view/10.21037/tcr-23-1221/dss
Peer Review File: Available at https://tcr.amegroups.com/article/view/10.21037/tcr-23-1221/prf
Conflicts of Interest: All authors have completed the ICMJE uniform disclosure form (available at https://tcr.amegroups.com/article/view/10.21037/tcr-23-1221/coif). The authors have no conflicts of interest to declare.
Ethical Statement: The authors are accountable for all aspects of the work in ensuring that questions related to the accuracy or integrity of any part of the work are appropriately investigated and resolved. This study was conducted in accordance with the Declaration of Helsinki (as revised in 2013). The study obtained approval from the Ethics Committee of The First Affiliated Hospital of Zhejiang Chinese Medical University on February 13, 2017 (Approval No. 2017-K-002-01). Informed consent was obtained from all individual participants.
Open Access Statement: This is an Open Access article distributed in accordance with the Creative Commons Attribution-NonCommercial-NoDerivs 4.0 International License (CC BY-NC-ND 4.0), which permits the non-commercial replication and distribution of the article with the strict proviso that no changes or edits are made and the original work is properly cited (including links to both the formal publication through the relevant DOI and the license). See: https://creativecommons.org/licenses/by-nc-nd/4.0/.
References
- Sung H, Ferlay J, Siegel RL, et al. Global Cancer Statistics 2020: GLOBOCAN Estimates of Incidence and Mortality Worldwide for 36 Cancers in 185 Countries. CA Cancer J Clin 2021;71:209-49. [Crossref] [PubMed]
- Kim KJ, Yu JW, Hwang HS, et al. CIIA is a novel regulator of detachment-induced cell death. Cancer Res 2010;70:6352-8. [Crossref] [PubMed]
- De Angelis ML, Francescangeli F, Nicolazzo C, et al. An Orthotopic Patient-Derived Xenograft (PDX) Model Allows the Analysis of Metastasis-Associated Features in Colorectal Cancer. Front Oncol 2022;12:869485. [Crossref] [PubMed]
- Zhang J, Li X, Lu Y, et al. Anoikis-Related Gene Signature for Prognostication of Pancreatic Adenocarcinoma: A Multi-Omics Exploration and Verification Study. Cancers (Basel) 2023;15:3146. [Crossref] [PubMed]
- Frisch SM, Francis H. Disruption of epithelial cell-matrix interactions induces apoptosis. J Cell Biol 1994;124:619-26. [Crossref] [PubMed]
- Zhou J, Yang S, Zhu D, et al. The crosstalk between anoikis and epithelial-mesenchymal transition and their synergistic roles in predicting prognosis in colon adenocarcinoma. Front Oncol 2023;13:1184215. [Crossref] [PubMed]
- Derouet M, Wu X, May L, et al. Acquisition of anoikis resistance promotes the emergence of oncogenic K-ras mutations in colorectal cancer cells and stimulates their tumorigenicity in vivo. Neoplasia 2007;9:536-45. [Crossref] [PubMed]
- Zou G, Ren B, Liu Y, et al. Inhibin B suppresses anoikis resistance and migration through the transforming growth factor-β signaling pathway in nasopharyngeal carcinoma. Cancer Sci 2018;109:3416-27. [Crossref] [PubMed]
- Wilkerson MD, Hayes DN. ConsensusClusterPlus: a class discovery tool with confidence assessments and item tracking. Bioinformatics 2010;26:1572-3. [Crossref] [PubMed]
- Adcock SA, McCammon JA. Molecular dynamics: survey of methods for simulating the activity of proteins. Chem Rev 2006;106:1589-615. [Crossref] [PubMed]
- Fu GB, Huang WJ, Zeng M, et al. Expansion and differentiation of human hepatocyte-derived liver progenitor-like cells and their use for the study of hepatotropic pathogens. Cell Res 2019;29:8-22. [Crossref] [PubMed]
- Li H, Horns F, Wu B, et al. Classifying Drosophila Olfactory Projection Neuron Subtypes by Single-Cell RNA Sequencing. Cell 2017;171:1206-1220.e22. [Crossref] [PubMed]
- Lim WX, Chen Z, Ahmed A. The adoption of deep learning interpretability techniques on diabetic retinopathy analysis: a review. Med Biol Eng Comput 2022;60:633-42. [Crossref] [PubMed]
- Ding R, Wang Y, Fan J, et al. Identification of immunosuppressive signature subtypes and prognostic risk signatures in triple-negative breast cancer. Front Oncol 2023;13:1108472. [Crossref] [PubMed]
- Adeshakin FO, Adeshakin AO, Afolabi LO, et al. Mechanisms for Modulating Anoikis Resistance in Cancer and the Relevance of Metabolic Reprogramming. Front Oncol 2021;11:626577. [Crossref] [PubMed]
- Okugawa Y, Toiyama Y, Toden S, et al. Clinical significance of SNORA42 as an oncogene and a prognostic biomarker in colorectal cancer. Gut 2017;66:107-17. [Crossref] [PubMed]
- Cao Z, Livas T, Kyprianou N. Anoikis and EMT: Lethal "Liaisons" during Cancer Progression. Crit Rev Oncog 2016;21:155-68. [Crossref] [PubMed]
- Kim HC, Chang J, Lee HS, et al. Mitochondrial UQCRB as a new molecular prognostic biomarker of human colorectal cancer. Exp Mol Med 2017;49:e391. [Crossref] [PubMed]
- Sandhu V, Bowitz Lothe IM, Labori KJ, et al. Molecular signatures of mRNAs and miRNAs as prognostic biomarkers in pancreatobiliary and intestinal types of periampullary adenocarcinomas. Mol Oncol 2015;9:758-71. [Crossref] [PubMed]
- Jiang SS, Xie YL, Xiao XY, et al. Fusobacterium nucleatum-derived succinic acid induces tumor resistance to immunotherapy in colorectal cancer. Cell Host Microbe 2023;31:781-797.e9. [Crossref] [PubMed]
- de Andrea CE, Schalper KA, Sanmamed MF, et al. Immunodivergence in Metastatic Colorectal Cancer. Cancer Cell 2018;34:876-8. [Crossref] [PubMed]
- Du L, Han XG, Tu B, et al. CXCR1/Akt signaling activation induced by mesenchymal stem cell-derived IL-8 promotes osteosarcoma cell anoikis resistance and pulmonary metastasis. Cell Death Dis 2018;9:714. [Crossref] [PubMed]
- Febbraio M, Hajjar DP, Silverstein RL. CD36: a class B scavenger receptor involved in angiogenesis, atherosclerosis, inflammation, and lipid metabolism. J Clin Invest 2001;108:785-91. [Crossref] [PubMed]
- Zhang X, Yao J, Shi H, et al. LncRNA TINCR/microRNA-107/CD36 regulates cell proliferation and apoptosis in colorectal cancer via PPAR signaling pathway based on bioinformatics analysis. Biol Chem 2019;400:663-75. [Crossref] [PubMed]
- Wang J, Zhang YW, Zhang NJ, et al. Coiled-Coil Domain Containing 80 Suppresses Nonylphenol-Induced Colorectal Cancer Cell Proliferation by Inhibiting the Activation of ERK1/2. Front Cell Dev Biol 2021;9:759820. [Crossref] [PubMed]
- Yuan J, Xie A, Cao Q, et al. INHBB Is a Novel Prognostic Biomarker Associated with Cancer-Promoting Pathways in Colorectal Cancer. Biomed Res Int 2020;2020:6909672. [Crossref] [PubMed]
- Xu Z, Li Y, Cui Y, et al. Identifications of Candidate Genes Significantly Associated With Rectal Cancer by Integrated Bioinformatics Analysis. Technol Cancer Res Treat 2020;19:1533033820973270. [Crossref] [PubMed]
- Kogo R, Shimamura T, Mimori K, et al. Long noncoding RNA HOTAIR regulates polycomb-dependent chromatin modification and is associated with poor prognosis in colorectal cancers. Cancer Res 2011;71:6320-6. [Crossref] [PubMed]
- Liu B, Liu Q, Pan S, et al. The HOTAIR/miR-214/ST6GAL1 crosstalk modulates colorectal cancer procession through mediating sialylated c-Met via JAK2/STAT3 cascade. J Exp Clin Cancer Res 2019;38:455. [Crossref] [PubMed]
- Rinn JL, Kertesz M, Wang JK, et al. Functional demarcation of active and silent chromatin domains in human HOX loci by noncoding RNAs. Cell 2007;129:1311-23. [Crossref] [PubMed]
- Wang B, Liang Y, Chai X, et al. Ectodysplasin A receptor (EDAR) promotes colorectal cancer cell proliferation via regulation of the Wnt/β-catenin signaling pathway. Exp Cell Res 2020;395:112170. [Crossref] [PubMed]
- Hiebert PR, Wu D, Granville DJ. Granzyme B degrades extracellular matrix and contributes to delayed wound closure in apolipoprotein E knockout mice. Cell Death Differ 2013;20:1404-14. [Crossref] [PubMed]
- Tibbs E, Cao X. Emerging Canonical and Non-Canonical Roles of Granzyme B in Health and Disease. Cancers (Basel) 2022;14:1436. [Crossref] [PubMed]