Radiomics in glioblastoma: current status, challenges and potential opportunities
Introduction
Grade IV glioblastoma multiforme (GBM) are the most aggressive brain tumors, with an extremely poor prognosis, dismal median overall survival rate of approximately 12–15 months with standard treatment, and a relative 2-year survival of only 30% (1). Lower-grade gliomas (grades I, II and III) comprise the remainder of primary malignant gliomas, and have a relative 2-year survival rate of 70–90% (2). Approximately 17,000 new cases of malignant gliomas are diagnosed each year in children, adolescents and adults, at an occurrence rate of about 5 in 100,000. Of these cases, 60–70% are GBM, which result in a mortality rate of over 10,000 deaths each year (3). Some GBM tumors manifest as primary tumors, and others show signs of progression from a lower-grade glioma (4). The aggressiveness of GBM has driven development of new surgical techniques, anti-angiogenic therapies, immunotherapies, and improved radiotherapies. The response to treatment has been correlated with molecular classification and subtyping based on genetics and expression data (5-7). Despite these efforts to better understand the underlying biology of GBM and advancements in the clinical treatment of this disease, it remains one of the most recalcitrant tumor types.
Like other solid tumor types, GBM develops a heterogeneous pattern of mutations (5). GBM does not feature sequential characteristic driver mutations, with multiple alterations occurring early in tumor pathogenesis, making the development of targeted therapies particularly challenging (8). Individual mutations or chromosomal alterations have not been linked with stages in tumor progression, unlike colorectal (9) and prostate cancers (10). In contrast, progression of colorectal cancer (CRC) has been associated with step-wise mutations and chromosomal alterations (9). Similarly, evidence from mouse models of prostate cancer as well as clinical observations indicate that sequential alterations in p27, NKX3.1, PTEN, and androgen receptor drive the pathogenesis of the disease (10). Women are more likely to have mutations in TP53, since these mutations are more prevalent in secondary GBM, which is more common in women (11), and IDH mutations are more common in adult secondary GBM than in children (12,13). Recent efforts have shown the predictive and prognostic utility of genetic characterization of GBM (14,15). Mutation, copy number, and expression data have been used to segregate GBM into four genomically-defined subtypes: classic, mesenchymal, neural, and proneural (7). Mutation and expression data can predict patient response to therapy (16), and correlations have been drawn between response to therapy and MGMT promoter methylation (17,18). The molecular heterogeneity represents a major challenge to the development of novel targeted therapies for GBM and evidence-based clinical decision-making (19). High-resolution genetic, epigenetic, and molecular descriptions of the range of GBM phenotypes will likely provide the basis for future improvements in treatment and the development of novel therapies.
Radiomics workflow
As discussed above, solid tumors have heterogeneous mutations, copy number alterations, and chromosomal aberrations across the tumor volume (20). This intrinsic property has made characterization of tumor phenotypes and the development of targeted therapies particularly challenging (21). Given the heterogeneity of GBM and other cancer types, numerous imaging approaches have been taken to comprehensively characterize tumors (22). In the last decade, radiomics has emerged as the concept of extracting quantitative radiologic features and drawing associations with clinical outcomes in tumors of the breast (23), brain (24), head and neck (25). Imaging data is acquired through the application of a variety of techniques and variants of X-ray computed tomography (CT), positron emission tomography (PET), and magnetic resonance imaging (MRI) (Figure 1A,B). The goal of this approach is to inform clinical decision-making by providing semi-automatically and automatically extracting radiologic features (Figure 1C,D) and associating these factors with outcomes like progression and survival. Clinical and biological associations are made through data mining, hypothesis generation, and biomarker discovery (Figure 1E). Given the complex intra-tumoral and inter-patient heterogeneity characteristic of GBM, and the difficulty in obtaining representative biopsies from which detailed molecular information can be extracted (26), sophisticated imaging approaches have the potential to address the tumor heterogeneity problem (27). It is hypothesized that it is possible to extract detailed phenotypic information by processing radiological imaging data (22). Determining tumor genetics and expression patterns from radiologic features, and developing these features as prognostic and predictive markers is an exciting possibility (28). Additionally, radiomics analysis has the potential to distinguish those low-grade gliomas which will progress to GBM from those which will not by determining the underlying genetic and molecular indicators of progression (4). Thus radiomics provides an avenue to tackle the formidable challenges of cancer treatment based on image-derived appearance, especially in the case of GBM.
In the past, radiology was analyzed qualitatively, following clinical algorithms to determine patient response to therapy and disease progression (29). Standardized measures of tumor volume by MRI, CT and ultrasound were incorporated into the RECIST criteria for tumor response (30), and advances in the use of PET modalities have been used in the more recently published PERCIST tumor response criteria (31). Developments in image analysis have allowed quantitative information to be derived from medical imaging. One-dimensional histogram-based and two-dimensional co-occurrence texture analyses (32) were developed to study and compare MRI (33) and other diagnostic images (34). Texture analysis has been used to predict clinical responses in non-small cell lung cancer patients (35) and was shown to be capable of discriminating between prostate tumor Gleason scores (36). This approach has been taken further by making associations between GBM tumor morphology as seen in MRI and underlying genetics (37) and expression data (38). Analysis of GBM tumor MRI imaging revealed novel imaging biomarkers capable of predicting clinical outcomes (39). These studies illustrate the potential of imaging data to overcome the limitations of traditional biomarkers.
Strategies—qualitative and quantitative measurement methods
Two main approaches have been taken to develop features for radiomic studies in GBM. The first has been to create standardized semantic features (Figure 2) which can be reproducibly scored by radiologists. This data is generated manually or by semi-automated methods. The second approach has been to derive fully computational features using imaging and statistical techniques. Both semantic and computational radiomic features are derived from multiple imaging techniques and modalities, including MR, PET, and CT. Semantic and computational features must take consideration of their dependence on scanning and acquisition protocols, signal-to-noise ratio and image resolution variations, properties unique to each modality and technique.
Several studies have correlated semantic features with clinical outcomes. Necrosis and tumor enhancement were identified as prognostic indicators (40). Non-contrast-enhancing tumor, multifocality, necrosis, satellites, and edema correlated with prognosis and survival (41). Iterative scatter search combined with an induction learning algorithm correlated imaging features with clinical data to predict survival in high-grade gliomas (42). The Visually Accessible Rembrandt Images (VASARI) feature set (Figure 2), comprising thirty semantic features developed to standardize radiological assessment of GBM, predicted survival and molecular subtype (29). These studies indicate the feasibility of developing standardized semantic feature sets and the efficacy of associating semantic features with prognostics and molecular descriptions in GBM.
While the studies outlined above focused on associating semantic features with clinical outcomes and tumor genetics, other studies have derived computational features computed from 2D/3D tumor regions (Figure 3) and related them to the molecular characteristics of GBM. Haralick features are first-order statistics which discriminate images based on texture are calculated from a co-occurrence matrix of pixel intensities within a region-of-interest (32). The original set of Haralick texture features was two-dimensional, and the approach has been adapted for segmenting 3D CT data by mathematically re-defining each feature in three dimensions (43). This method was used to accurately segment structures within the abdominal cavity (44). Spatial habitats are tumor-subregions computed across multi-modality imaging sequences [e.g., T1-post contrast, T2 and fluid attenuated inversion recovery (FLAIR) sequences], where each habitat represents a region with a unique combination of “high” and “low” pixel intensities in each imaging sequence, and have been associated with gene expression status of epidermal growth factor receptor and 12-month overall survival status (24) in GBM. Imaging habitats are correlated with GBM molecular subtype status (neural, pro-neural, mesenchymal and classical) and survival status (45). Imaging habitats quantify the grey-level heterogeneity in GBM MR scans (46) and track tumor evolution driven by detecting variations in tumor blood supply (47).
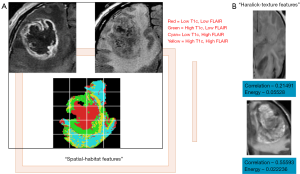
The majority of radiomics studies in GBM have focused on MRI images (27) derived from T1-weighted acquisitions, T2-weighted, and FLAIR (Figure 4). Gadolinium contrast agent is often administered following T1-weighted acquisition. Following this acquisition sequence, GBM generally appears as a non-enhancing region ringed with enhancing signal, with the area surrounding the tumor (peritumoral) being bright in the T2 and FLAIR scans (48,49). The signal acquired by each modality indicates the presence of vasogenic edema, tumor infiltration, peritumoral tissue, etc. (50). T1-T2-T1c-FLAIR modalities were used to develop computational features which correlated strongly with the VASARI semantic feature set (37), and in multiple radiogenomics studies (51) which correlated VASARI features with mRNA expression (38), mutational status (29), dysfunctional metabolism (52), molecular subtype and survival (53). The mesenchymal GBM subtype was identified using T1c-FLAIR images (54). Another study using T1, T1c, T2 modalities correlated semantic features with epigenetic status in GBM (55). A comprehensive review and tabulation of radiogenomics studies can be found in Bai et al. (51). Diffusion weighted imaging (DWI) and perfusion MR (MRP) variants (Figure 4) provide additional tumor characteristics at the tissue level. Varied combinations of MR modalities have been used to construct radiological feature combinations that highlight distinct portions of the tumor and surrounding tissue. Magnetic resonance spectroscopy imaging (MRSI) proved useful in guiding radiotherapy by defining a molecular signature distinguishing tumor and non-tumor brain tissue (56).
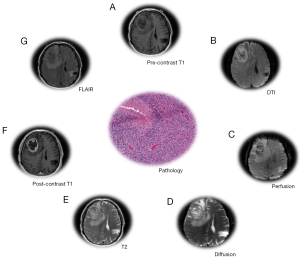
Semantic and computational (57) feature sets have been derived from CT and PET. Like MR, they can be performed using contrast agents or tracer compounds to gain additional physiological information. When CT is performed using a contrast-enhancement, the GBM tumor region presents as an area of low density ringed by an enhancing region (58). PET scans can measure metabolic activity in GBM, but it has not yet been determined whether combined PET/MRI improves diagnostics (59). A number of variants of PET use molecular tracers to derive additional information based on metabolic activity quantified by glucose uptake. FDG PET, which utilizes the fluorodeoxyglucose tracer, has been investigated as an alternative to gadolinium enhancement, but reports conflict as to its efficacy in diagnosing recurrent GBM (60,61). FDG is the most widely used PET tracer (62), and several studies have developed semantic and computational feature sets using FDG-PET (63,64). Computational features from FDG PET/CT images were used to stage lymphoma (65) and non-small cell lung cancer patients (66), and to predict response to radiation therapy in lung cancer (67). In addition to FDG, a large number of alternative tracer compounds have been investigated (62), although there have been few radiomic studies from these less commonly used contrast methods. The FDOPA PET technique, which is based on the DOPA-decarboxylase pathway and amino acid transport, has not been used in radiomics studies to-date, but has been shown to have predictive value in the recurrence of LGG (68,69). Thymidine and FLT PET (70) and amino acid PET have not been studied using the radiomics approach, but these variants of the imaging technique may provide additional prognostic and predictive information. FET PET, which uses a 18-F-fluoroethyl-tyrosine tracer, has been used to determine the extent of invasion of glioma cells into the surrounding brain matter (71). In PET scans with 18F-FMISO, GBM is characterized by higher uptake values relative to the grey matter at the boundary of the tumor, and the central necrotic tissue of GBM tumors will accumulate less tracer (72,73). Compared to other imaging modalities, PET better differentiates recurrent or residual GBM tumor from edema and scar tissues after resection (74). However, for initial diagnosis of GBM, PET scans in general have low sensitivity and specificity (74). As a result, the availability of pre-treatment GBM PET images is also limited for radiomic analysis.
Challenges and opportunities
Medical Image acquisition and standardization
Medical image acquisition is routine for standard MR sequences, but acquisition/scanning protocols vary among institutions, leading to challenges in comparing or combining data gathered in multi-center clinical trials. There are several components of the image acquisition process that lead to variation in the data. These include scanner variability, variation in the specifications of an imaging device involved, and procedures followed by a particular radiologist or imaging physicist/technician. The protocol defined by the physicist/radiologist can vary in terms of image resolution, slice thickness, cut angles and washout period for the contrast imaging. Hence, standardization of image acquisition is central to the integrity of the entire radiomics pipeline. If common standards of acquisition cannot be achieved, the imaging pipeline should incorporate methods standardizing the imaging prior to computational/semantic/volumetric feature extraction. There are some solutions in this space, such as intensity normalization, registering multi-parametric data to a specific anatomical plane, isotropic pixel or voxel re-slicing. Standardized image formats allow data to be readily processed across all steps of the radiomics pipeline. The Neuroimaging Informatics Technology Initiative (NIFTI) format provides support for functional MRI, a coordinate system linked to voxel indices, and dual file storage (75). The Digital Imaging and Communications in Medicine (DICOM) engineering standard provides a system facilitating communication between medical imaging devices and software from multiple vendors (76). DICOM objects contain metadata with patient identification, acquisition device information and printing parameters (77). The Nearly Raw Raster Data NRRD format support multiple compression algorithms and data represented as integer and floating point variants (http://teem.sourceforge.net/nrrd/index.html). These measures must be taken during image acquisition to maintain consistency in image preprocessing, feature extraction and classification to ensure that the acquired images are comparable. Standardizing signal intensity prior to image registration prevents the registration from being distorted by intensity variations (78).
Modalities examined on the basis of the intensity of multi-parametric MR data are combined to classify GBM patients based on clinical and genomic data (24,47). Most radiomic studies in glioma have most routinely centered on two MR modalities, FLAIR and T1-post contrast. Advancements in MRI and PET technologies have allowed radiomics analyses to gain further biological insight: diffusion-weighted MRI measures cellular density, whereas PET and MRS reveal metabolic activity and vascular proliferation (79,80). Dynamic susceptibility, contrast-enhanced T2 MR imaging measuring relative cerebral blood volume, predicted overall survival of GBM patients (81). Including the non-enhancing region and EGFR mutational status improved prognostics in a retrospective study of 45 The Cancer Genome Atlas (TCGA) patients (82). When combining different modalities, an inter-modality registration algorithm with respect to one anatomical plane is required to ensure proper alignment (83), as motion during acquisition can cause distortions (84). Inter-modality spatial alignment is straightforward for modalities such as T1, T1-post contrast, FLAIR, while for perfusion and diffusion images, the algorithms cause geometric distortions to the regions of interest (80). These errors increase when registering an MR image to CT image or registering a histopathology to radiology image. Thus, developing registration algorithms that are suitable across multiple MR-modalities would significantly improve the pipeline by reducing registration error (85,86). Applying Pearson correlation (87), voxel-based registration with thresholding and volumetrics (88), and constraining errors in high-similarity regions improved registration of multi-modality imaging sets (89).
Tumor segmentation
Following acquisition, the tumor images can be segmented through a manual, semi-automated or fully-automated procedure. Since there is no defined ground truth, this process will not be perfectly accurate. Consistency of segmentation process plays a significant role in the radiomics pipeline, as variations in processing steps such as thresholding can affect segmentation (90) by altering how the tumor is delineated (91). Fully-automated segmentation pipelines are available for GBM (92), and are under development for LGG. Random forest classifiers were applied to segment GBM tumor volumes for feature extractions (93). Automation improves reproducibility and concordance, since feature generation and the further downstream analysis are dependent on the quality of the initial segmentation. Manual delineation is time-consuming and further increases the chances of inter-observer variability. When manual contouring was compared to a semi-automated approach using 3D-Slicer, the semi-automated approach (94) improved reproducibility and robustness. Pattern recognition software and techniques reduce observer effects by automating the segmentation pipeline, increasing the robustness of derived results.
Feature Interpretation and analysis
High-throughput feature extraction is at the core of the radiomics process. As described above, there are two types of features—semantic and computational. The definition of these features is dependent on the hypothesis of the project. The numerous methods to obtain the features result in thousands of complex descriptions of the region of interest. Moving from manual to automated methods of feature extraction, automated feature extraction complements manual analysis and reduces variation in scoring semantic features. However, automated feature extraction is still vulnerable to site-specific variations in image acquisition, and any automated method may require modifications when implemented at a study site. Due to different methodologies, reproducibility and robustness of these extracted features is vital as these features will directly determine the correlation drawn to tumor genomic, expression, and microenvironment phenotypes. For example, differences in thresholding stringency can alter the number and attributes of extracted features (95). Outcome modeling based on computational features is a complex, multi-step process starting with pre-processing, followed by feature estimation, feature selection, classification, and finally evaluation by validation studies (96). Robustness of features can be analyzed on the basis of factors such as geometric transformations of the regions of interest and intensity variability (97). We analyzed the robustness of texture features through 8 different geometric transformations of ROIs (horizontal translation by 2 pixels, horizontal and vertical translation by 2 pixels, rotation by 1-degree, rotation by 5-degree, moving each point on the outline on the horizontal and vertical axes by a zero-median random number with a 0.1 and 0.5 pixel standard deviation, shrinking the ROI by 1 pixel and dilating the ROI by 1 pixel) (37). Eighty-two TCGA GBM cases were used, using the original as well as the transformed ROI for texture analysis. A set of thirteen Haralick feature ratios with 2 filters [Laplacian of Gaussian (LOG) (98) and Gaussian (99)] at 5 filter widths (0.2, 0.4, 1.5, 2.5, 5) and 4 pixel distances (1, 2, 4 and 8 mm) (32) were computed. Besides this, histogram-based features (uniformity, mean-intensity and entropy) were computed. Additionally, some regional properties such as area, minimum, mean and maximum intensity were computed from the tumor region. All of these features (2,236 for T1-post contrast and 2,236 features for FLAIR) were computed for each of the 9 ROIs (original and 8 transformations) per case in the 82 dataset. Feature extraction was followed by assessment of robustness via intraclass correlation (ICC), with ICC computed between the various features. The correlation cut-off was set as 0.6 and 22 FLAIR and 26 T1-post contrast features were found to be robust. Heatmaps were generated to depict this high correlation. Figure 5 summarizes the results of this study. Tables 1 and 2 show the interpretation of these robust features in the context of the GBM radiomics problem. Image-derived features classify the target hypothesis (outcome). Classification represents the greatest computational challenge, utilizing machine learning algorithms such as neural networks, support vector machines, decision trees, and logistic regression. During data analysis and predictive modeling, it is important to perform cross-validation (96) to evaluate the predictive models, or preferably, to evaluate their performance on a clinically-matched independent (test) cohort. Cross-validation assesses the generalizability of the analysis pipeline for image-biomarker identification. The pipeline can be then used with higher reliability in multicenter/multi-institutional settings. To minimize type I errors caused by multiple comparisons, methods such as the Benjamini-Hochberg correction (102) and bootstrap-based correction (101) have been proposed. When the sample size is relatively small, methods have been developed to minimize false-positive results (103).
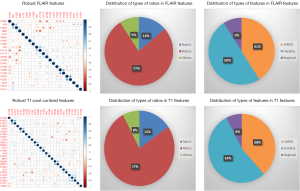
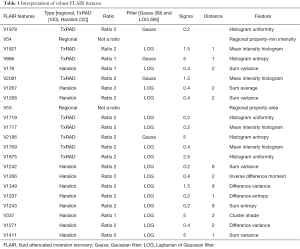
Full table
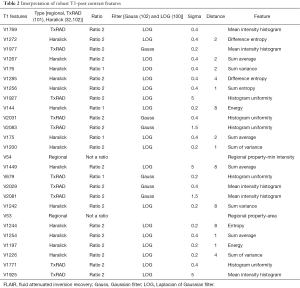
Full table
Besides generating automated features on the ROIs in a high-throughput manner, it is important to discuss their reporting structure. A lexicon for clinical reporting is recommended for radiomics studies, made especially necessary in multi-institutional settings. Improvements have been made in this area through creation of reporting data systems across different disease sites: such as Liver Imaging Reporting and Data System (LI-RADS™ ACR), Prostate Imaging Reporting and Data System (PI-RADS™), Head Injury Imaging Reporting and Data System (HI-RADS, ACR), and Lung Reporting and Data System (LungRADs™, ACR). A universal reporting system with standard terminology and syntax plays a significant role during the feature interpretation and data analysis (104).
Creating mineable data
While performing classification with high-dimensional radiomics data (as might be obtained by large-scale feature extraction), there is potential risk of overfitting to the data. The risk of overfitting increases when the number of instances is much fewer than the number of computationally-extracted features. This “curse-of-dimensionality” can be addressed by minimizing the number of features using principal components analysis (PCA) (105), sparse PCA (sPCA), partial least squares regression (PLS) (106), non-linear PCA (107), and auto-encoders (108), in addition to cross-validation for generalized model construction. Some of these feature selection methods can be supervised (using the label information from each instance), or unsupervised (based on exploiting variance in the data). Such dimension reduction methods make interpretation of the reduced feature set more difficult as features are mathematically-combined into composite features. Aside from relating radiomics (imaging-phenotype) with genetic characteristics, there is also a need for modeling formalisms that integrate measurements across these diverse modalities to drive decision-making in the clinical realm.
Conclusions
Radiomics promises to improve the characterization of radiological datasets and provide further insight to guide patient care in the era of personalized medicine. GBM is one of the most genetically heterogeneous tumor types, exhibiting remarkable inter- and intra-patient variability. Substantial progress has already been made in solving many of the technical hurdles inherent in the radiomics process in GBM. Advances in genome sequencing, expression profiling and machine learning have increased the resolution of datasets and the sensitivity and specificity of the computational methods used to analyze them. Statistical models are needed which relate imaging features to GBM molecular status with high specificity/sensitivity to make the approach useful in practice. More studies correlating radiomic features with disease outcomes and molecular attributes will illuminate the underlying tumor biology of imaging features and treatment responses. Large-scale decision algorithms that fuse features obtained across imaging, genomic and clinical modalities can enable multi-modal decision making in the personalized medicine arena.
Acknowledgments
Funding: A. Rao was supported by CCSG Bioinformatics Shared Resource P30 (CA01667), an Institutional Research Grant from The University of Texas MD Anderson Cancer Center (MD Anderson) and a Career Development Award from the MD Anderson Brain Tumor SPORE.
Footnote
Provenance and Peer Review: This article was commissioned by the editorial office, Translational Cancer Research for the series “Radiomics in Radiation Oncology”. The article has undergone external peer review.
Conflicts of Interest: All authors have completed the ICMJE uniform disclosure form (available at http://dx.doi.org/10.21037/tcr.2016.06.31). The series “Radiomics in Radiation Oncology” was commissioned by the editorial office without any funding or sponsorship. Rao A served as the unpaid Guest Editor of the series. The authors have no other conflicts of interest to declare.
Ethical Statement: The authors are accountable for all aspects of the work in ensuring that questions related to the accuracy or integrity of any part of the work are appropriately investigated and resolved.
Open Access Statement: This is an Open Access article distributed in accordance with the Creative Commons Attribution-NonCommercial-NoDerivs 4.0 International License (CC BY-NC-ND 4.0), which permits the non-commercial replication and distribution of the article with the strict proviso that no changes or edits are made and the original work is properly cited (including links to both the formal publication through the relevant DOI and the license). See: https://creativecommons.org/licenses/by-nc-nd/4.0/.
References
- Shapiro WR, Green SB, Burger PC, et al. Randomized trial of three chemotherapy regimens and two radiotherapy regimens and two radiotherapy regimens in postoperative treatment of malignant glioma. Brain Tumor Cooperative Group Trial 8001. J Neurosurg 1989;71:1-9. [Crossref] [PubMed]
- Surawicz TS, McCarthy BJ, Kupelian V, et al. Descriptive epidemiology of primary brain and CNS tumors: results from the Central Brain Tumor Registry of the United States, 1990-1994. Neuro Oncol 1999;1:14-25. [PubMed]
- Howlader N, Noone AM, Krapcho M, et al. editors. SEER Cancer Statistics Review, 1975-2011, National Cancer Institute. Bethesda, MD, based on November 2013 SEER data submission, posted to the SEER web site, April 2014.
- Weber RG, Sabel M, Reifenberger J, et al. Characterization of genomic alterations associated with glioma progression by comparative genomic hybridization. Oncogene 1996;13:983-94. [PubMed]
- Brennan CW, Verhaak RG, McKenna A, et al. The Somatic Genomic Landscape of Glioblastoma. Cell 2013;155:462-77. [Crossref] [PubMed]
- Patel AP, Tirosh I, Trombetta JJ, et al. Single-cell RNA-seq highlights intratumoral heterogeneity in primary glioblastoma. Science 2014;344:1396-401. [Crossref] [PubMed]
- Verhaak RG, Hoadley KA, Purdom E, et al. Integrated Genomic Analysis Identifies Clinically Relevant Subtypes of Glioblastoma Characterized by Abnormalities in PDGFRA, IDH1, EGFR, and NF1. Cancer Cell 2010;17:98-110. [Crossref] [PubMed]
- Patel M, Vogelbaum MA, Barnett GH, et al. Molecular targeted therapy in recurrent glioblastoma: current challenges and future directions. Expert Opin Investig Drugs 2012;21:1247-66. [Crossref] [PubMed]
- Fearon ER, Vogelstein B. A genetic model for colorectal tumorigenesis. Cell 1990;61:759-67. [Crossref] [PubMed]
- Nelson WG, De Marzo AM, Isaacs WB. Prostate cancer. N Engl J Med 2003;349:366-81. [Crossref] [PubMed]
- Louis DN, von Deimling A, Chung RY, et al. Comparative study of p53 gene and protein alterations in human astrocytic tumors. J Neuropathol Exp Neurol 1993;52:31-8. [Crossref] [PubMed]
- Sturm D, Witt H, Hovestadt V, et al. Hotspot Mutations in H3F3A and IDH1 Define Distinct Epigenetic and Biological Subgroups of Glioblastoma. Cancer Cell 2012;22:425-37. [Crossref] [PubMed]
- Sturm D, Bender S, Jones DT, et al. Paediatric and adult glioblastoma: multiform (epi)genomic culprits emerge. Nat Rev Cancer 2014;14:92-107. [Crossref] [PubMed]
- Nobusawa S, Watanabe T, Kleihues P, et al. IDH1 mutations as molecular signature and predictive factor of secondary glioblastomas. Clin Cancer Res 2009;15:6002-7. [Crossref] [PubMed]
- Hartmann C, Hentschel B, Wick W, et al. Patients with IDH1 wild type anaplastic astrocytomas exhibit worse prognosis than IDH1-mutated glioblastomas, and IDH1 mutation status accounts for the unfavorable prognostic effect of higher age: implications for classification of gliomas. Acta Neuropathol 2010;120:707-18. [Crossref] [PubMed]
- Schonberg DL, Bao S, Rich JN. Genomics informs glioblastoma biology. Nat Genet 2013;45:1105-7. [Crossref] [PubMed]
- Malmstrom A, Gronberg BH, Marosi C, et al. Temozolomide versus standard 6-week radiotherapy versus hypofractionated radiotherapy in patients older than 60 years with glioblastoma: the Nordic randomised, phase 3 trial. Lancet Oncol 2012;13:916-26. [Crossref] [PubMed]
- Wick W, Platten M, Meisner C, et al. Temozolomide chemotherapy alone versus radiotherapy alone for malignant astrocytoma in the elderly: the NOA-08 randomised, phase 3 trial. Lancet Oncol 2012;13:707-15. [Crossref] [PubMed]
- Parker NR, Khong P, Parkinson JF, et al. Molecular heterogeneity in glioblastoma: potential clinical implications. Front Oncol 2015;5:55. [Crossref] [PubMed]
- Fidler IJ. Tumor heterogeneity and the biology of cancer invasion and metastasis. Cancer Res 1978;38:2651-60. [PubMed]
- Fidler IJ. Biological heterogeneity of cancer: implication to therapy. Hum Vaccin Immunother 2012;8:1141-2. [Crossref] [PubMed]
- Lambin P, Rios-Velazquez E, Leijenaar R, et al. Radiomics: Extracting more information from medical images using advanced feature analysis. Eur J Cancer 2012;48:441-6. [Crossref] [PubMed]
- Yamamoto S, Maki DD, Korn RL, et al. Radiogenomic analysis of breast cancer using MRI: a preliminary study to define the landscape. AJR Am J Roentgenol 2012;199:654-63. [Crossref] [PubMed]
- Lee J, Narang S, Martinez JJ, et al. Associating spatial diversity features of radiologically defined tumor habitats with epidermal growth factor receptor driver status and 12-month survival in glioblastoma: methods and preliminary investigation. J Med Imaging (Bellingham) 2015;2:041006 [Crossref] [PubMed]
- Hatt M, Majdoub M, Vallières M, et al. 18F-FDG PET uptake characterization through texture analysis: investigating the complementary nature of heterogeneity and functional tumor volume in a multi-cancer site patient cohort. J Nucl Med 2015;56:38-44. [Crossref] [PubMed]
- Sottoriva A, Spiteri I, Piccirillo SG, et al. Intratumor heterogeneity in human glioblastoma reflects cancer evolutionary dynamics. Proc Natl Acad Sci U S A 2013;110:4009-14. [Crossref] [PubMed]
- Moton S, Elbanan M, Zinn PO, et al. Imaging Genomics of Glioblastoma: Biology, Biomarkers, and Breakthroughs. Top Magn Reson Imaging 2015;24:155-63. [Crossref] [PubMed]
- Aerts HJ, Velazquez ER, Leijenaar RT, et al. Decoding tumour phenotype by noninvasive imaging using a quantitative radiomics approach. Nat Commun 2014;5:4006. [PubMed]
- Gutman DA, Cooper LA, Hwang SN, et al. MR imaging predictors of molecular profile and survival: multi-institutional study of the TCGA glioblastoma data set. Radiology 2013;267:560-9. [Crossref] [PubMed]
- Eisenhauer EA, Therasse P, Bogaerts J, et al. New response evaluation criteria in solid tumours: revised RECIST guideline (version 1.1). Eur J Cancer 2009;45:228-47. [Crossref] [PubMed]
- Wahl RL, Jacene H, Kasamon Y, et al. From RECIST to PERCIST: Evolving Considerations for PET response criteria in solid tumors. J Nucl Med 2009;50:122S-50S. [Crossref] [PubMed]
- Haralick RM, Shanmuga K, Dinstein I. Textural features for image classification. IEEE Trans Syst Man Cybern B Cybern 1973;SMC3:610-21. [Crossref]
- Bernasconi A, Antel SB, Collins DL, et al. Texture analysis and morphological processing of magnetic resonance imaging assist detection of focal cortical dysplasia in extra-temporal partial epilepsy. Ann Neurol 2001;49:770-5. [Crossref] [PubMed]
- Chen DR, Chang RF, Kuo WJ, et al. Diagnosis of breast tumors with sonographic texture analysis using wavelet transform and neural networks. Ultrasound Med Biol 2002;28:1301-10. [Crossref] [PubMed]
- Cook GJ, Yip C, Siddique M, et al. Are pretreatment 18F-FDG PET tumor textural features in non-small cell lung cancer associated with response and survival after chemoradiotherapy? J Nucl Med 2013;54:19-26. [Crossref] [PubMed]
- Wibmer A, Hricak H, Gondo T, et al. Haralick texture analysis of prostate MRI: utility for differentiating non-cancerous prostate from prostate cancer and differentiating prostate cancers with different Gleason scores. Eur Radiol 2015;25:2840-50. [Crossref] [PubMed]
- Gevaert O, Mitchell LA, Achrol AS, et al. Glioblastoma Multiforme: Exploratory Radiogenomic Analysis by Using Quantitative Image Features. Radiology 2014;273:168-74. [Crossref] [PubMed]
- Jamshidi N, Diehn M, Bredel M, et al. Illuminating radiogenomic characteristics of glioblastoma multiforme through integration of MR imaging, messenger RNA expression, and DNA copy number variation. Radiology 2014;270:1-2. [Crossref] [PubMed]
- Cui Y, Tha KK, Terasaka S, et al. Prognostic imaging biomarkers in glioblastoma: development and independent validation on the basis of multiregion and quantitative analysis of MR images. Radiology 2016;278:546-53. [Crossref] [PubMed]
- Lacroix M, Abi-Said D, Fourney DR, et al. A multivariate analysis of 416 patients with glioblastoma multiforme: prognosis, extent of resection, and survival. J Neurosurg 2001;95:190-8. [Crossref] [PubMed]
- Pope WB, Sayre J, Perlina A, et al. MR imaging correlates of survival in patients with high-grade gliomas. AJNR Am J Neuroradiol 2005;26:2466-74. [PubMed]
- Zacharaki EI, Morita N, Bhatt P, et al. Survival analysis of patients with high-grade gliomas based on data mining of imaging variables. AJNR Am J Neuroradiol 2012;33:1065-71. [Crossref] [PubMed]
- Tesar L, Smutek D, Shimizu A, et al. 3D extension of Haralick texture features for medical image analysis. Proceedings of the Foerth IASTED Inter-national Conference on Signal Processing, Pattern Recognition, and Applications, SPPRA 2007. Anaheim, CA, USA, 2007:350-5.
- Tesar L, Shimizu A, Smutek D, et al. Medical image analysis of 3D CT images based on extension of Haralick texture features. Comput Med Imaging Graph 2008;32:513-20. [Crossref] [PubMed]
- Lee J, Narang S, Martinez J, et al. Spatial habitat features derived from multiparametric magnetic resonance imaging data are associated with molecular subtype and 12-month survival status in glioblastoma multiforme. PloS One 2015;10:e0136557 [Crossref] [PubMed]
- Zhou M, Hall LO, Goldgof DB. editors. Exploring Brain Tumor Heterogeneity for Survival Time Prediction. 2014 22nd International Conference on Pattern Recognition (ICPR). DC, USA: Computer Society Washington, 2014.
- Zhou M, Hall L, Goldgof D, et al. Radiologically defined ecological dynamics and clinical outcomes in glioblastoma multiforme: preliminary results. Transl Oncol 2014;7:5-13. [Crossref] [PubMed]
- Kelly PJ, Daumas-Duport C, Kispert DB, et al. Imaging-based stereotaxic serial biopsies in untreated intracranial glial neoplasms. J Neurosurg 1987;66:865-74. [Crossref] [PubMed]
- Zinn PO, Colen RR. Imaging genomic mapping in glioblastoma. Neurosurgery 2013;60:126-30. [Crossref] [PubMed]
- Pavlisa G, Rados M, Pavlisa G, et al. The differences of water diffusion between brain tissue infiltrated by tumor and peritumoral vasogenic edema. Clin Imaging 2009;33:96-101. [Crossref] [PubMed]
- Bai HX, Lee AM, Yang L, et al. Imaging genomics in cancer research: limitations and promises. Br J Radiol 2016;89:20151030 [Crossref] [PubMed]
- Colen RR, Vangel M, Wang J, et al. Imaging genomic mapping of an invasive MRI phenotype predicts patient outcome and metabolic dysfunction: a TCGA glioma phenotype research group project. BMC Med Genomics 2014;7:30. [Crossref] [PubMed]
- Yang D, Rao G, Martinez J, et al. Evaluation of tumor-derived MRI-texture features for discrimination of molecular subtypes and prediction of 12-month survival status in glioblastoma. Med Phys 2015;42:6725-35. [Crossref] [PubMed]
- Naeini KM, Pope WB, Cloughesy TF, et al. Identifying the mesenchymal molecular subtype of glioblastoma using quantitative volumetric analysis of anatomic magnetic resonance images. Neuro Oncol 2013;15:626-34. [Crossref] [PubMed]
- Carrillo JA, Lai A, Nghiemphu PL, et al. Relationship between tumor enhancement, edema, IDH1 mutational status, MGMT promoter methylation, and survival in glioblastoma. AJNR Am J Neuroradiol 2012;33:1349-55. [Crossref] [PubMed]
- Parra NA, Maudsley AA, Gupta RK, et al. Volumetric Spectroscopic Imaging of Glioblastoma Multiforme Radiation Treatment Volumes. Int J Radiat Oncol Biol Phys 2014;90:376-84. [Crossref] [PubMed]
- Parmar C, Leijenaar RT, Grossmann P, et al. Radiomic feature clusters and prognostic signatures specific for Lung and Head & Neck cancer. Sci Rep 2015;5:11044. [Crossref] [PubMed]
- Zinn PO, Mahmood Z, Elbanan MG, et al. Imaging Genomics in Gliomas. Cancer J 2015;21:225-34. [Crossref] [PubMed]
- Fink JR, Muzi M, Peck M, et al. Multimodality Brain Tumor Imaging: MR Imaging, PET, and PET/MR Imaging. J Nucl Med. 2015;56:1554-61. [Crossref] [PubMed]
- Nihashi T, Dahabreh IJ, Terasawa T. Diagnostic accuracy of PET for recurrent glioma diagnosis: a meta-analysis. AJNR Am J Neuroradiol 2013;34:944-50. [Crossref] [PubMed]
- Pötzi C, Becherer A, Marosi C, et al. [11C] methionine and [18F] fluorodeoxyglucose PET in the follow-up of glioblastoma multiforme. J Neurooncol 2007;84:305-14. [Crossref] [PubMed]
- Smith G, Carroll L, Aboagye EO. New frontiers in the design and synthesis of imaging probes for PET oncology: current challenges and future directions. Mol Imaging Biol 2012;14:653-66. [Crossref] [PubMed]
- Leijenaar RT, Carvalho S, Velazquez ER, et al. Stability of FDG-PET Radiomics features: an integrated analysis of test-retest and inter-observer variability. Acta Oncol 2013;52:1391-7. [Crossref] [PubMed]
- Henriksson E, Kjellen E, Wahlberg P, et al. 2-Deoxy-2-[18F] fluoro-D-glucose uptake and correlation to intratumoral heterogeneity. Anticancer Res 2007;27:2155-9. [PubMed]
- Lartizien C, Rogez M, Niaf E, et al. Computer-aided staging of lymphoma patients with FDG PET/CT imaging based on textural information. IEEE J Biomed Health Inform 2014;18:946-55. [Crossref] [PubMed]
- van Gómez López O, García Vicente AM, Honguero Martínez AF, et al. Heterogeneity in [18F]fluorodeoxyglucose positron emission tomography/computed tomography of non-small cell lung carcinoma and its relationship to metabolic parameters and pathologic staging. Mol Imaging 2014;13:1-12. [PubMed]
- Lovinfosse P, Janvary ZL, Coucke P, et al. FDG PET/CT texture analysis for predicting the outcome of lung cancer treated by stereotactic body radiation therapy. Eur J Nucl Med Mol Imaging 2016;43:1453-60. [Crossref] [PubMed]
- Chen W, Silverman DH, Delaloye S, et al. 18F-FDOPA PET imaging of brain tumors: comparison study with 18F-FDG PET and evaluation of diagnostic accuracy. J Nucl Med 2006;47:904-11. [PubMed]
- Karunanithi S, Sharma P, Kumar A, et al. 18F-FDOPA PET/CT for detection of recurrence in patients with glioma: prospective comparison with 18F-FDG PET/CT. Eur J Nucl Med Mol Imaging 2013;40:1025-35. [Crossref] [PubMed]
- Aarntzen EH, Srinivas M, De Wilt JH, et al. Early identification of antigen-specific immune responses in vivo by [18F]-labeled 3'-fluoro-3'-deoxy-thymidine ([18F]FLT) PET imaging. Proc Natl Acad Sci U S A 2011;108:18396-9. [Crossref] [PubMed]
- Vees H, Senthamizhchelvan S, Miralbell R, et al. Assessment of various strategies for 18F-FET PET-guided delineation of target volumes in high-grade glioma patients. Eur J Nucl Med Mol Imaging 2009;36:182-93. [Crossref] [PubMed]
- Bruehlmeier M, Roelcke U, Schubiger PA, et al. Assessment of hypoxia and perfusion in human brain tumors using PET with 18F-fluoromisonidazole and 15O-H2O. J Nucl Med 2004;45:1851-9. [PubMed]
- Swanson KR, Chakraborty G, Wang CH, et al. Complementary but distinct roles for MRI and 18F-fluoromisonidazole PET in the assessment of human glioblastomas. J Nucl Med 2009;50:36-44. [Crossref] [PubMed]
- la Fougère C, Suchorska B, Bartenstein P, et al. Molecular imaging of gliomas with PET: opportunities and limitations. Neuro Oncol 2011;13:806-19. [Crossref] [PubMed]
- NIH. NifTI Documentation 2007 (cited 2016 03/24). Available online: http://nifti.nimh.nih.gov/nifti-1
- Bidgood WD, Horii SC, Prior FW, et al. Understanding and using DICOM, the data interchange standard for biomedical imaging. J Am Med Inform Assoc 1997;4:199-212. [Crossref] [PubMed]
- Flanders AE, Carrino JA. Understanding DICOM and IHE. Semin Roentgenol 2003;38:270-81. [Crossref] [PubMed]
- Bagci U, Udupa JK, Bai L. editors. The influence of intensity standardization on medical image registration. Conference on Medical Imaging 2010: Visualization, Image-Guided Procedures, and Modeling. San Diego, California, USA, 2013.
- Dhermain F. Radiotherapy of high-grade gliomas: current standards and new concepts, innovations in imaging and radiotherapy, and new therapeutic approaches. Chin J Cancer 2014;33:16-24. [Crossref] [PubMed]
- Narayana A, Chang J, Thakur S, et al. Use of MR spectroscopy and functional imaging in the treatment planning of gliomas. Br J Radiol 2007;80:347-54. [Crossref] [PubMed]
- Jain R, Poisson L, Narang J, et al. Genomic mapping and survival prediction in glioblastoma: molecular subclassification strengthened by hemodynamic imaging biomarkers. Radiology 2013;267:212-20. [Crossref] [PubMed]
- Jain R, Poisson LM, Gutman D, et al. Outcome prediction in patients with glioblastoma by using imaging, clinical, and genomic biomarkers: focus on the nonenhancing component of the tumor. Radiology 2014;272:484-93. [Crossref] [PubMed]
- Pappas IP, Styner M, Malik P, et al. Automatic method to assess local CT-MR imaging registration accuracy on images of the head. AJNR Am J Neuroradiol 2005;26:137-44. [PubMed]
- Li G, Miller RW. Volumetric Image Registration of Multi-modality Images of CT, MRI and PET. Bethesda, Maryland, USA: National Cancer Institute, National Institutes of Health: 2010.
- Ellingson BM, Cloughesy TF, Lai A, et al. Nonlinear registration of diffusion-weighted images improves clinical sensitivity of functional diffusion maps in recurrent glioblastoma treated with bevacizumab. Magn Reson Med 2012;67:237-45. [Crossref] [PubMed]
- Hawkes DJ, Hill DL, Hallpike L, et al. Coregistration of Structural and Functional Images. Springer-Verlag London Ltd 2003:181-97.
- Saad ZS, Glen DR, Chen G, et al. A new method for improving functional-to-structural MRI alignment using local Pearson correlation. Neuroimage 2009;44:839-48. [Crossref] [PubMed]
- Rohlfing T, Beier J. Improving reliability and performance of voxel-based registration by coincidence thresholding and volume clipping 1999. King’s College 1999:165-8.
- Gass T, Szekely G, Goksel O. Detection and Correction of Inconsistency-based Errors in Non-Rigid Registration. Conference on Medical Imaging Image Processing 2014. San Diego, 2014:16-8.
- Zaidi H, El Naqa I. PET-guided delineation of radiation therapy treatment volumes: a survey of image segmentation techniques. Eur J Nucl Med Mol Imaging 2010;37:2165-87. [Crossref] [PubMed]
- Lee JA. Segmentation of positron emission tomography images: Some recommendations for target delineation in radiation oncology. Radiother Oncol 2010;96:302-7. [Crossref] [PubMed]
- Porz N, Bauer S, Pica A, et al. Multi-Modal Glioblastoma Segmentation: Man versus Machine. Plos One 2014;9:e96873 [Crossref] [PubMed]
- Zhang J, Barboriak DP, Hobbs H, et al. A fully automatic extraction of magnetic resonance image features in glioblastoma patients. Med Phys 2014;41:042301 [Crossref] [PubMed]
- Parmar C, Rios Velazquez E, Leijenaar R, et al. Robust Radiomics feature quantification using semiautomatic volumetric segmentation. PLoS One 2014;9:e102107 [Crossref] [PubMed]
- Hatt M, Tixier F, Le Rest CC, et al. Robustness of intratumour F-18-FDG PET uptake heterogeneity quantification for therapy response prediction in oesophageal carcinoma. Eur J Nucl Med Mol Imaging 2013;40:1662-71. [Crossref] [PubMed]
- Kassner A, Thornhill RE. Texture analysis: a review of neurologic MR imaging applications. AJNR Am J Neuroradiol 2010;31:809-16. [Crossref] [PubMed]
- Balagurunathan Y, Kumar V, Gu Y, et al. Test-retest reproducibility analysis of lung CT image features. J Digit Imaging 2014;27:805-23. [Crossref] [PubMed]
- Haralick RM. Statistical and structural approaches to texture. Proceedings of the IEEE 1979;67:786-804. [Crossref]
- Haralick R, Shapiro L. Computer and Robot Vision. 1st. Boston, MA, USA: Addison-Wesley Longman Publishing Co, 1992.
- Ganeshan B, Miles KA, Young RC, et al. Three-dimensional textural analysis of brain images reveals distributed grey-matter abnormalities in schizophrenia. Eur Radiol 2010;20:941-8. [Crossref] [PubMed]
- Efron B. 1977 Rietz Lecture: Bootstrap methods —another look at the jackknife. Ann Stat 1979;7:1-26. [Crossref]
- Benjamini Y, Hochberg Y. Controlling the false discovery rate: a practical and powerful approach to multiple testing. J R Stat Soc Series B Stat Methodol 1995;57:289-300.
- Kim S, Dougherty E, Barrera J, et al. Strong feature sets from small samples. J Comput Biol 2002;9:127-46. [Crossref] [PubMed]
- Duong PA, Pastel DA, Sadigh G, et al. The Value of Imaging Part II: Value beyond Image Interpretation. Acad Radiol 2016;23:23-9. [Crossref] [PubMed]
- Mishra D, Dash R, Rath AK, et al. Feature selection in gene expression data using principal component analysis and rough set theory. Adv Exp Med Biol 2011;696:91-100. [Crossref] [PubMed]
- Wold S, Ruhe A, Wold H, et al. The collinearity problem in linear-regression - the partial least-squares (PLS) approach to generalized inverses. SIAM J Sci Stat Comput 1984;5:735-43. [Crossref]
- Kramer MA. Nonlinear principal component analysis using autoassociative neural networks. AIChE J 1991;37:233-43. [Crossref]
- Kumar D, Wong A, Clausi D. Lung nodule classification using deep features in CT images. Computer & Robot Vision 2015;327:110-6.